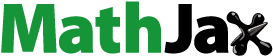
ABSTRACT
This paper addresses the problem of integrating sustainability and business issues in manufacturing through the implementation of Industry 4.0 and process management. The objective of this work is to propose a novel methodology to guide companies in benefitting the so-called Twin Transition (digital and sustainable) drivers at the operation level for reaching business and strategic objectives. The proposed methodology results in a unique tool to address both strategic and business objectives and sustainability through practical managerial actions and technological implementations. The roadmapping methodology is divided into five phases that, starting from the definition of corporate objective and strategy, provide a comprehensive, multi-dimensional, cross-functional plan of Industry 4.0 technology implementation and related changes in terms of processes and Information & Communication Technologies (ICT) architectures. The methodology is presented through an application case performed on an Aerospace company site devoted to the assembly, integration, and test of satellites.
1. Introduction
Nowadays, the industry is called to face a multitude of disruptive macro-trends and contingent external events that are contributing to harming the industrial context in its core principles (Jeffrey et al., Citation2021; Okorie et al., Citation2020).
All these factors suggest that a transition toward more responsible and efficient utilization of resources is now an imperative and a source of competitive advantage. Consequently, companies and even governments are devoting more and more effort to this direction (United Nations, Citation2020). In this context, a responsible solution must also meet the economic needs of the actors who undertake it, especially in the business context. However, the conciliation between economic and environmental sustainability and the lack of knowledge are major barriers hampering sustainability in manufacturing (European Sustainable Business Federation, Citation2019; Rizos et al., Citation2016).
Nevertheless, if sustainability represents a trend that shapes the objectives of companies and society, on the other hand, a technological wave, referred to as Industry 4.0 (I4.0), can support manufacturers to undertake a sustainable transition (Rosa et al., Citation2020).
Although the concept of I4.0 is clear (Ford & Despeisse, Citation2016; Hopkins, Citation2021; Kagermann et al., Citation2013; Mubarik et al., Citation2021; Schmidt et al., Citation2021), when it comes to practice, the application strategies of I4.0 technologies appear foggy and costly to companies (Spaltini et al., Citation2022). The same considerations apply to sustainable or circular transition (Leila et al., Citation2021).
Hence, these two major trends represent an inescapable way to operate competitively today but show a dual source of uncertainty and challenges to be addressed together. The authors refer to these twofold and indivisible transitions, sustainable and technological, as Twin Transition (TT) (Rehman et al., Citation2023).
The relationship between I4.0 technologies and their impact on Sustainability is well known in academia (Rosa et al., Citation2020). Focusing on CE (Spaltini et al., Citation2023), mapped the main contributions that identified relationships between the I4.0 technologies and the CE principles. They also highlighted the main opportunities that I4.0 might provide by enabling CE approaches.
The literature suggests that the reasons for poor adoption of I4.0 technologies to improve processes and foster sustainability are not due to a lack of structured and practical models to merge I4.0 and CE that consider the variables characterizing real manufacturing firms (Kumar et al., Citation2020). In this direction, Bag et al., (Citation2022) noted that manufacturers often struggle as they cannot align strategic objectives (economic and environmental) and technological needs. Trevisan et al., (Citation2023) noted that manufacturers face challenges in balancing short- and long-term focus, thus adopting ineffective TT strategies. Agrawal et al., (Citation2023) showed many TT initiatives field because of the inability or difficulty in planning and setting priorities. Overall, the literature agrees upon the idea that a methodology to foster TT in manufacturing is still missing (Abdul-Hamid et al., Citation2020; Bag et al., Citation2021).
Hence, the following research is intended to fill such gap by proposing a novel methodology designed to guide manufacturers in exploiting I4.0 to foster economic and environmental sustainability. To pursue this objective, the researchers focused on roadmapping models and methodologies and investigated the Technology Roadmap (TRM) field of research for TT in manufacturing. The authors opted to focus on the TRM field as it is designed to provide dynamic and structured plans based on advanced technology implementation. It ranges from the identification of goals to the definition of the action plan before its implementation (Bernal et al., Citation2009; Park et al., Citation2020). The study proposes a methodology to develop and implement a TRM designed to support operations and business process reengineering, under a process and a technological perspective, by considering both financial-economic and sustainability objectives of the stakeholder. The research aims at developing a unique tool able to address both strategic and business objectives and sustainability through practical actions of a management nature and technological implementations enabled by I4.0. Indeed, the literature has provided fruitful TRM methodologies addressing TT-related challenges in manufacturing, but with a limited focus on specific industries like cosmetics (Perossa et al., Citation2023), or domains like ICT only (Harmon et al., Citation2012). A holistic method able to cover operation process from a technological, organizational and process standpoint is still missing.
The paper is structured as follows: in section 2 the adopted research methodology is presented, section 3 is devoted to the analysis of the literature on TRM, section 4 is focused on presenting the developed methodology, in section 5 the results of an application case in a manufacturing company are exposed. In section 6, discussions and conclusions are provided.
2. Research methodology
The following research originates in the design science domain applied to operation management research field. Indeed, it mainly aims at bridging a gap between practice and theory in the TRM for TT domain by producing artifacts (Holmström et al., Citation2009). Thus, the authors relied on Design Research Methodology (DRM) as it aims at developing a new procedure that could be significantly exploitable in industrial cases (Blessing & Chakrabarti, Citation2009). The authors adopted an interpretivist approach involving both qualitative methods and inductive reasoning (Williamson, Citation2002).
The following research is then categorized as applied engineering based as it iteratively involves theory building and practice testing (Lamperti et al., Citation2023; Pezzotta et al., Citation2018; Potts, Citation1993; Sassanelli & Terzi, Citation2022; Sassanelli et al., Citation2019).
Starting from the analysis of the literature, a reiterative process encompassing a cyclical collection of feedback from experts (companies and academics) was followed (Denyer & Tranfield, Citation2009; Greenhalgh, Citation1997). The collection of feedback from industry experts was managed through direct and in-depth interactions (e.g. workshops) with managers and operators of a multinational aerospace manufacturing company.
Concerning the theory building, the authors conducted a Systematic Literature Review (SLR) which allows to collect seminal relevant studies, assess their quality, and synthesize their findings using scientific method (Johnson et al., Citation2013). The SCOPUS database has been selected due to its high coverage of academic journals and being the main search database in both the fields addressed (Culot et al., Citation2020; Kirchherr et al., Citation2017).
The analysis of academic contribution was divided into two blocks. First, the authors reviewed academic contributions related to the use of a roadmap to enable CE transition. Similarly, the second block focused on the analysis of roadmaps designed to address Circular Manufacturing practices and strategies through I4.0 technologies.
The SLR was structured by the authors according to the protocol shown in .
Table 1. Research protocol.
The literature search resulted in 615 documents from the sole keywords selected and the eligibility criteria introduced (Greenhalgh, Citation1997). The authors did not include other eligibility criteria as the number of documents identified resulted sufficient.
Therefore, the authors focused of the identification of the relevant papers for the aim of the research. This step was conducted in two phases which encompassed an analysis of title, abstract and, eventually, full text.
In the first phase, the authors examined both titles and abstract of the documents collected. They excluded only those documents which resulted undoubtedly not related to the topics addressed. In this phase, 141 documents were deemed relevant to be further investigated. The second phase consisted in the analysis of the full body of the remaining documents. Out of those, the authors had access to 58 papers and kept 43 of them. Additionally, the authors included 14 papers through snowball sampling. The overall sample of papers used for the analysis, including previously known contributions of the authors, resulted in 87 academic papers ().
The conceptualization of the methodology relied on the outcomes generated from the SLR and followed a cyclical interaction with experts from the manufacturing and academia (Dawson, Citation2009; Jenney et al., Citation2010).
Given the high heterogeneity in the size of manufacturing companies, the authors adopted the single plant as the unit of analysis of the research. This choice is motivated by the need for the researcher to refer to comparable environments to build the tool. Indeed, the distinction among large, medium and small companies adopted by the European Commission is used to compare companies at the corporate level (European Commission, Citation2003). On the other hand, both academia and empirical evidence from the authors’ experience suggest that by focusing on a single plant level such distinctions among manufacturers turn out to be shrunk.
3. Literature review
Although a shared definition of TRM is still missing (Kerr & Phaal, Citation2022), defined it as ‘a method and management tool, which is underpinned by a generalizable framework, the approach is completely scalable to any unit of analysis and customizable to any strategic context (and) can clearly and coherently portray and present the dynamic linkages (including highlighting discontinuities) between resources and capabilities, product/service solutions, organizational objectives and business drivers, market characteristics, and the changing environment.’
The definition highlights some relevant characteristics. It is somehow related to technology used by the company, it must be linked with strategy, and it links resources, products, and markets.
However, Garcia & Bray, (Citation1997) argued that TRM is not necessarily intended to support entities in introducing new products or services. They distinguished three main typologies, namely: Product technology roadmaps (PTR) focused on product, Emerging technology roadmaps (ETR) focused on depicting given scenarios where emerging technologies may apply, and Issue-oriented roadmaps (IOR) which are focused on improving processes and solve problems that are not explicitly stated.
Overall, TRM is a tool exploited for planning to better contextualize strategy and the role of technology in a competitive environment (Mauro & Amaral, Citation2011). The objective of TRM is to depict, describe and plan a set of activities to overcome barriers that might be known or hidden (Hilary & Daim, Citation2012).
Eventually, TRM is a visual representation, enriched by additional documents, that makes order among the many variables and constraints that a company may consider and provide a strategic overview (Cotrino et al., Citation2020). It represents only the outcome of a backgrounded process, the roadmapping, which encapsulates the very value of the tool and starts from the identification of a need or a desired state of maturity (Bernal et al., Citation2009; Garcia & Bray, Citation1997; Park et al., Citation2020).
Focusing on TT in the manufacturing, the needs expressed by practitioners belong to two macro-clusters:
The need for introducing or renewing a given product portfolio: this is the case of PTRs and ETRs in which companies are interested in making their product connected, greener or they are willing to shift towards a Product-Service System paradigm (Robert Phaal, Farrukh, and Probert Citation2004);
The need for improving the operation processes: this is the case of IORs and ETRs in which companies are willing to redesign their processes or intervene on their resources and asset to achieve a goal using Industry 4.0 technologies (Gerdsri et al., Citation2019)
Regardless of the field of intervention for TT, literature and empirical evidence suggest that companies, especially Small-Medium Enterprises (SMEs), still face several challenges (Mittal et al., Citation2018). In addition to this (Schöggl et al., Citation2023), stressed that, despite I4.0 technologies are finding their applications in manufacturing, there is a limited view of how these might foster sustainability (Lerman et al., (Citation2022) extended the scope to a Supply chain and operation perspective. In the study, they showed that more intensive use of I4.0 is positively related to higher sustainability performances, thus underlying the need for tools supporting digitalization of manufacturers under a TT standpoint.
(Schmitt et al., Citation2020) highlighted that SMEs struggle to complete or start I4.0-related initiatives since they lack a categorization of proceeding, an identification of core phases, and a structuring of the main elements that characterize the projects themselves. Thus, TRM turns out to be a useful, yet essential, method to overcome such barriers.
Literature provides several reasons that support the need for TRM in the industrial context Dalenogare et al., (Citation2018) noted that the I4.0 transition in manufacturing contexts requires a substantial change in strategies, organizational models and infrastructures, manufacturing operations and technologies, human resources, and management of practices. Hence, the risk of a manufacturer being overwhelmed by all those changes is high if not properly guided by roadmaps (Butt, Citation2020). da Silveira Junior et al., (Citation2018) highlighted that TRM is beneficial to anticipating technology trends, supporting decision-making and resource allocation, carrying out risk analysis, and identifying technologies and competencies to enhance. Chih Jen and Daim, (Citation2017) added that TRMs support companies in several other aspects like the alignment of technology investments to strategy, the possibility to benchmark and measure the success of the transition, the capability to define and meet future performance targets and the generation of a proper baseline for technology development.
So far roadmapping and technology planning models have been proposed for many industries and different levels of detail. Focusing on manufacturing-related sectors and Industry 4.0 transition, many models mostly refer to new product introduction and new market access, thus highlighting the very high strategic role of TRM within companies. In this regard (Hilary & Daim, Citation2012), presented a multi-perspective (technology and business) conceptual roadmapping framework based on the Delphi method, AHP, and QFD. This resulted in a seminal contribution as it showed the adaptability of TRM to existing managerial decision-support instruments. Jeon et al., (Citation2011) developed a PTR which investigated multi-criteria decision-making supplier selection dimension in the semiconductor industry. (Ching et al., (Citation2022) proposed a seven-step theoretical framework to link sustainable manufacturing and Industry 4.0 technology and clustered 15 manufacturing sustainability functions of I4.0. Such a framework encompasses both experts’ opinions and matrix structures to establish relationships and hierarchical structures, which in turn resulted in fully theoretical research. Kumar et al., (Citation2021) developed a framework based on Modified Stepwise Weight Assessment Ratio Analysis and Weighted Aggregated Sum Product Assessment to enable multi-criteria decision-making problems for prioritizing I4.0 strategies. Thus, they identified 18 main strategies for I4.0 Transition aimed at overcoming 23 comprehensive barriers faced by manufacturers and proposed a methodology to give priority to challenges. Sufian et al., (Citation2021) presented and implemented, through an industrial use case, a cross-function roadmapping methodology based on 6 stages (gears) and 18 substages to drive manufacturers by highlighting common features of models, frameworks, and transformation strategies and initiatives. This work highlighted the necessity to adopt a cross-department perspective to address strategic changes toward TT (Rafael et al., Citation2018). argued that one of the main issues related to TRM in an industrial context is related to the difficulty of updating them. Hence, they developed a three-step methodology to continuously update existing roadmaps toward digitalization based on agile iterative cycles (Verma et al., Citation2022). opened the path to solution development through TT-oriented roadmapping methodology by investigating 30 impediments for companies to rely on I4.0 to enhance sustainability and prioritizing them through AHP. In the study, the main impediments to TT were clustered and prioritized although no roadmapping methodology was proposed. In the same direction (Khan et al., Citation2020), identified 16 challenges and relied on Best-Worst Method to establish a hierarchy among them (Arman et al., Citation2014). developed a software-based framework, STAR, which integrated aspects of TRM with project management and portfolio analysis to drive decision-making in the R&D domain. Hence, it successfully integrated new managerial tools into PTRs in a single-function domain (Sajjad et al., Citation2022). designed a feature-based roadmap toward I4.0 transition only in manufacturing scenarios using AHP and TOPSIS (Cortés et al., Citation2021). presented a taxonomy, S3, to characterize manufacturing processes, assess them, and identify points to improve them. The model was specifically tested and validated in a real manufacturing firm for the deployment of an automated micromachining tool. Nevertheless, the model was specifically designed to address a specific need and suffered from limited generalizability (Garza Ramos et al., Citation2022). developed a TRM that integrated other managerial tools like SWOT analysis, and Porter’s 5 forces specifically designed to increase TRL from 3 to 8 of a 3D cell culture workstation.
More devoted to sustainability (Howard et al., Citation2022), conducted a mixed-tool method to assess CE level of 6 manufacturing companies and detect potential areas of improvement although it did not integrate a proper roadmap to achieve the desired result (Urbán et al., Citationn.d..). developed a sector-specific TRM aimed at facilitating TT for final-energy generator product manufacturing companies. Such a model was based on sustainability and technology layers and measured outcomes according to 4 high-level dimensions, Customer, Economy, Politics, and Ecology. The extant literature also presents some circular-oriented roadmapping tools which provide a preliminary tentative to direct manufacturing companies toward more sustainable operations (Lopes de Sousa Jabbour et al., Citation2018) (Perossa et al., Citation2023). compared 19 roadmapping frameworks to develop a TRM methodology specifically addressing TT in cosmetic industry. Although this limited the range of applicability of the model, this allowed to adapt to manufacturers more rapidly. Concerning industry-specific tools (Talla & McIlwaine, Citation2022), proposed a roadmap supporting the design stage of products for the construction sector, while (Siripongpreda et al., Citation2021) studied a method to reuse the cellulose waste considering it as a good biodegradable resource useful especially for the electrochemical sensors. The core idea of researchers is to move towards the enhancement of factories’ circular performances. Nevertheless, in this circular-oriented context, the focus at the factory level only needs to be extended into an ecosystem perspective where more than one entity at a time is involved (Mogos & Fragapane, Citation2022). Indeed, specific roadmaps are studied to establish a proper reverse logistics network supported by the right I4.0 technologies (Dev et al., Citation2020). This ecosystem perspective leads sometimes to an additional extension reaching a regional level (Sautter, Citation2016), as the case of (Lai et al., Citation2022) focused on plastic waste management in Singapore and India, and the case of (Siddique et al., Citation2022) still focused on plastic management and circularity but in Bangladesh. Moreover, focused on the wood sector, with an eye on the Philippines, a proposal was made by (Balanay et al., Citation2022).
In most cases, the analysis dimensions both referred to as I4.0 and CE are employed to ensure the pursuit of a TT (Lopes de Sousa Jabbour et al., Citation2018; Talla & McIlwaine, Citation2022). Regarding I4.0, usually, the enabling technologies are explored as key drivers toward circular business models (Prakash & Ambedkar, Citation2022). Indeed, either the CE pillars or ReSOLVE framework are used as analysis dimensions limiting the employment of an operative perspective which instead should be considered by manufacturing companies when the strategic plans need to be transformed into level-headed actions. An operative perspective is adopted by (de Mattos Nascimento et al., Citation2022) in their study which is focused on a 3D printing factory while broader perspectives are still missing (Contini & Peruzzini, Citation2022). focused the attention on how sustainability enhanced by I4.0 can be measured and, in this regard, identified 107 sustainability KPIs based on the TBL concept, which refers to the three sustainability areas (social: 48 KPIs, economic: 39 KPIs, and environmental: 30 KPIs).
The extant literature already presents several contributions providing an integrated view of I4.0 and CE to create roadmaps. Nevertheless, they mostly refer to theoretical frameworks whose applicability has not been validated in real contexts. Hence, the gap found in literature concerning the lack of a tool able to support manufacturers in TT still appears open or only partially solved (Acerbi et al., Citation2021). Specifically, an operative roadmap that supports the TT starting from the strategic objectives of an established company to reach higher levels of circularity supported by technological advancement is still lacking in literature. CE adoption can be highly supported by specific technologies and this opportunity can be further exploited considering that huge technological investments have been already pursued by manufacturing companies in the last years also thanks to the incentives by governments (De Carolis et al., Citation2017). Summing up, a multi-dimensional model should be developed covering different CE-related performances by also integrating the technological perspective.
4. Proposal of a TRM methodology for TT
As per other roadmapping methodologies, this lays its foundations on three main questions to answer, namely: a) Where do we want to go?, b) Where are we now? and c) How can we get there? (Hansen et al., Citation2016).
Consequently, literature and empirical evidence suggest that TRM is usually composed of 3 macro-steps which are (Gerdsri & Vatananan, Citation2007; Kerr et al., Citation2012; Park et al., Citation2020; Robert et al., Citation2004):
Assessment: which aims at defining the goals and understanding the current level of I4.0 and sustainability maturity level within the subject of analysis;
Roadmapping: which aims at defining the necessary transformation journey to support organizations to achieve the stated goals;
Control: which aims at monitoring the outcomes of the projects proposed and validating the roadmap or, on the contrary, adjusting it.
According to this general fragmentation, the authors have mostly devoted their research toward the roadmapping macro-step. The assessment phase is usually carried out through other methodologies and models which belong to Maturity Model (MM) domain (de Bruin et al., Citation2005). In this direction, since literature on MMs has already proposed a multitude of assessments, the authors aimed to design a methodology able to take the outcomes of existing MMs focused on I4.0 and Sustainability Transition (ST) and exploit them in roadmapping process ().
Hence, the TRM proposed in this contribution is composed of 5 main steps ().
The TRM proposed is compiled together with the organization (Chih Jen & Daim, Citation2017; Kerr & Phaal, Citation2019; Kerr et al., Citation2018; Oliveira et al., Citation2019). The involvement of the organization is ensured through specific workshops with managers, middle-management and operators (Chih Jen & Daim, Citation2017; de Alcantara & Martens, Citation2019; Farrukh et al., Citation2014; Kerr & Phaal, Citation2019; Kerr et al., Citation2013, Citation2018; Oliveira et al., Citation2019; Robert et al., Citation2003).
The next paragraphs describe in detail the mentioned steps.
4.1. Step 1: goals and KPIs
The first step, ‘Goals and KPIs’, aims to identify the main strategic objectives that the organization wants to achieve. Setting the right strategic goals and extracting their core elements is indeed key for manufacturers to first understand where to focus (Lichtenthaler, Citation2008). The aim is to give structure and solidity to the abovementioned TT by defining clear targets to achieve. Reportedly, this phase may involve heterogeneous stakeholders. Thus, the collection and understanding of the objectives from the beginning is beneficial to detect potential synergies and causal relationships. Therefore, a second and side takeaway of this phase consists of a prior rationalization of core needs to focus on.
The identification of strategic objectives could be difficult to determine as it must interpolate future needs of the market, the behaviour of competitors and incumbent entrants, and the available resources that the companies will be called to manage (Porter, Citation2008). This process may represent a first barrier to manufacturers which could be unaware of some of these abovementioned elements. In this direction, step 1 has been conjectured to guide the whole process of goal identification through a preliminary understanding of the main trends affecting the industry of reference and the main technologies exploitable by the company. However, circumscribing objectives in a structured way is not enough to set the basis for a strategic transformation. Those must indeed be translated into measurable units to establish a given target to achieve and KPIs to monitor throughout the whole TT. Thus, the conclusive task of this phase consists of the joint definition of KPIs that will allow assessing the correctness of the TRM and its necessary capability to be aligned with corporate strategy (Cotrino et al., Citation2020). To achieve such objective, the authors developed a 2-level approach. This encompasses a top-down phase in which the main strategic goals are given by company’s decision-makers and a bottom-up phase to identify the main goals at operational level. Such approach is designed to ensure consistency with strategy (Cotrino et al., Citation2020) as well as increasing commitment at different organizational levels (Kerr et al., Citation2012). Furthermore, the bottom-up phase also aims at detecting the first areas of improvement and collecting the first feedback about the actual needs of the plants (Farrukh et al., Citation2014). In this regard, a dedicated session neutrally facilitated by TRM experts shall guide a first brainstorming (Oliveira et al., Citation2019).
4.2. Step 2: criticalities
Once defined goals, available technologies, and strategic KPIs, step 2 is dedicated to the identification of criticalities and particularly their prioritization. This step is designed to be carried out after the understanding of the current level of maturity of the system. In this regard, the authors did not develop a specific MM rather they designed the methodology to be modularly integrated into existing models in operations management domain such as (De Carolis et al., Citation2017; Sassanelli et al., Citation2020) MMs’. Indeed, regardless of the level of granularity, the TRM covers the following processes: Design and Engineering; Production; Quality management; Maintenance management; Logistics (internal and external), and Supply chain management (De Carolis et al., Citation2017).
The methodology is designed to distinguish criticalities in terms of severity and of process on which the criticality impacts.
As suggested by (Acerbi et al., Citation2022), all the criticalities identified are assigned to the process in which they originate (different colours of the squares). Regarding the identification of the severity of the single criticalities, the authors have conjectured 2 different approaches. The selection of the proper approach depends on the evaluation of 2 main challenges of firms: the time available to conduct the analysis (Halse & Jæger, Citation2019; Kumar et al., Citation2021) and the data availability of the organization (Geum et al., Citation2015; Han & Geum, Citation2022).
The first approach encompasses a qualitative definition of the severity of the criticality on a 3-level scale: High, Medium and Low (). Such a distinction shall be jointly determined within the workshop.
The second approach, the most time-consuming of the two but the deepest in terms of analysis, lays its foundations on system dynamics theory, multi-objective decision model and multi-attribute decision models. It includes a preliminary phase in which all the presumed criticalities highlighted are sorted into actual criticalities (squares) and effects (hexagons) (Sterman, Citation2001). The effects are those presumed criticalities that are generated by the actual or core criticalities, subsequently, the methodology requires setting the criticality-effect and effect–effect linkages to create a map of dependence and depict where and how the identified criticalities impact the organization.
Afterwards, each effect is assigned to each of the strategic goals stated in Step 1. Then, each direct criticality-effect and effect–effect link is assigned to a severity index calculated as a function of the probability of occurrence and absolute impact in case of occurrence while each effect is assigned to a score calculated as a function of the impact it has on the strategic goals.
All these steps lead to prioritizing criticalities considering the absolute severity, the impact on the different processes, and the relevance for the achievement of the goals (last column of the matrix in ).
4.2.1. Analysis of the impacts
The quantitative approach focuses on numerically depicting both direct and indirect relationships among the criticalities identified during the assessment phase and the consequential problems that they generate along the processes under analysis.
Examples of similar methodologies are already available in many fields of research and practice like Quality management through QFD (Jeon et al., Citation2011).
Hence, the authors exploited the main principles that rule these methodologies to develop a similar one to be applied in the TRM field.
Hence, the first phase of this quantitative approach encompasses a classification of problems into two main categories, namely:
Criticality: root problems that affect specific functions and processes;
Effect: generated problems that affect functions and processes due to the propagation of not addressed criticalities.
Given the need for TRM to be visual (de Alcantara & Martens, Citation2019; Kerr et al., Citation2012; Robert et al., Citation2003; Cotrino et al., Citation2020; Farrukh et al., Citation2014; Oliveira et al., Citation2019; Phaal & Muller, Citation2009), once classified the problems (entities), the authors shall identify the links among criticalities and effect (C-E relationships) as well as the links among effects (E–E relationships) and depict them in a map ().
The map reported in shows how the different criticalities (squares) directly generate the effects (hexagons) and how the effects are linked to the others. In particular, the cause (either effect or criticality) is located at the tail of the arrows while the effects are pointed by the head of the arrows.
Depending on the complexity, the scope of the analysis and the level of maturity of the case analysed, it can be noticed that the readability of the map might be seriously threatened thus harming the possibility to support decision-making. To grant external simplicity and the understanding of the output (Hansen et al., Citation2016; Kerr et al., Citation2013; Robert et al., Citation2003), the mapped links are translated into a matrix through the allocation of a binary attribute to all the direct C-E and E-E relationships.
Starting from these two matrices populated with Boolean variables, the tool analyses the existence of indirect relationships with increasing degrees of ‘kinship’. The variables, respectively, are:
1: There is a relationship from A (rows) to B (columns);
0: There is no relationship.
As an example, at the first comparison of the input tables, it was possible to distinguish analytically the existence of a criticality–effect relationship in cases where these were linked together by a single common effect.
Like the approach followed by (Ching et al., Citation2022), the identification of further indirect relationships among entities follows a cascade approach.
To generalize the methodology, the number of relationships identified by each iteration of the toll is quantified by the formula below (Ghobakhloo et al., Citation2021).
where
: Identifies the relation of a criticality (x) on a direct effect (e) at step i;
: Identifies the total number of steps.
This preliminary process of quantitative mapping of criticality–effect relationships, at present, highlights the existence or not of a correlation between different factors but does not define their impact. Therefore, to obtain a reliable prioritization of interventions, it is necessary to assign a weight to the individual relationships.
4.2.2. Quantitative assessment of cause-effect impacts
The authors opted for the introduction of a unique scoring system for all the elements of the model to quantify the linkages described. Specifically, a scale from 1 to 4 has been selected where 1 indicates the lowest value (i.e. lower frequency, lower impact, lower relevance) and 4 indicates the maximum value.
The first phase of the quantification process consisted in associating the different effects identified with a degree of relationship (i.e. an impact) for the company objectives.
As supported by many TRM methodologies, the relevance of the declared objectives must be jointly defined with the company since they will dramatically affect the whole prioritization process (Chih Jen & Daim, Citation2017; Kerr & Phaal, Citation2019; Kerr et al., Citation2018; Oliveira et al., Citation2019; Phaal et al., Citation2011; Robert et al., Citation2003; Spaltini et al., Citation2022).
Then, the overall impact of each effect was then normalized to fall within a score scale between 1 and 4.
The formula used for this purpose is:
where
: Identifies the overall impact (Relevance) of the generic effect
;
: Identifies the company’s perceived relevance of the generic objective
;
: Identifies the number of goals whose perceived relevance has been measured to define impact
.
A scheme of this process is provided in .
Table 2. Impacts of individual effects on corporate objectives.
The second phase consists in defining the specific weight of each criticality to the direct effects generated (Criticality–Effect relationships) and of each effect to the effect immediately impacted (Effect–Effect relationships). Both the cases follow the same process. First, the magnitude of the overall impacts is divided according to two basic criteria: Absolute impact and frequency.
The absolute impact is the magnitude, from 1 to 4, that a factor x (criticality or effect) can pour its impact on a factor y (effect). It is necessary to specify that the absolute impact has the sole objective of measuring how much a criticality impacts a given effect but does not have consider the magnitude of the criticality itself.
The frequency index instead identifies the possibility, from 1 to 4, of factor x (criticality or effect) to impact a factor y (effect). The result of this process is reported in .
4.3. Step 3: solution proposal
Taking as input the technologies identified in step 1 and the criticalities detected in step 2, stage 3 focuses on the development of solutions.
In this sense, the authors have clustered 3 main typologies of solutions based on the findings from the extant literature: Process-related actions focused on process and organizational redesign; Digital Backbone-related actions focused on implementing or updating ICT technologies, I4.0-related actions focused on implementing or updating I4.0 technologies.
Addressing all criticalities that emerged in step 2 could be unfeasible for manufacturers for a lack of resources or capabilities (Yang et al., Citation2018) or inappropriate compared to the achievable benefits (V. Kumar et al., Citation2021). Hence, the first task consists of the selection of a threshold area of the matrix in which determines the criticalities and effects to focus on based on the priority rate allocated.
Afterwards, the identification of solutions and their categorization is carried out through a first ad hoc analysis of the problems and a subsequent iteration with company and process experts for validation.
It is worth highlighting that TT enabled by I4.0 technology adoption requires a deep background transformation of processes and organization (Marcucci et al., Citation2021). Accordingly, it also requires a careful deployment of enabling technologies including ICT systems (Acerbi & Taisch, Citation2020; Ciliberto et al., Citation2021; Garcia-Muiña et al., Citation2018). Hence, each solution proposed must include a proportionate evaluation of all the three solution categories to guarantee self-standing and modularity (Lee & Park, Citation2005).
4.4. Step 4&5: TRM
Finally, TRM phase takes as input the solutions designed in previous phases and maps them in the visual TRM. This phase has a twofold aim. First, it allows planning the interventions and related investments and resources throughout a given timespan. Second, it is the moment in which the results are formally communicated to stakeholders.
Hence, the authors developed an approach able to satisfy to facilitate both aspects by introducing a multi-layered framework. The choice of a multi-layer TRM is motivated by the necessity to rely on a bi-dimensional structure thus increasing the ease of readiness and the understanding of the visual result. Indeed, TRM has the ultimate role of supporting the decision-making process and strategic planning; thus, they need to be easily understandable by decision-makers (Kerr et al., Citation2013) as well as ensure flexibility in its implementation (Robert et al., Citation2003).
In particular, the first layer aims to link the individual strategic objectives of the firm to the solutions proposed along a timeframe. Looking at the scheme in , this first dimension determines the horizontal axis. The second dimension, represented by the vertical axis, identifies the expected effort for the design and deployment of the given solution. As suggested by (Hansen et al., Citation2016), such choice is motivated by the need of the stakeholder to clearly understand the actions to undertake to reach a specific goal.
Regarding the actions for improvements already detected in phase 3 of the whole roadmapping process, they keep their macro distinctions into Process, Digital Backbone and Industry 4.0 and cross-category solutions; although a general rule about the allocation of projects throughout the timescale is not a priori providable, some guidelines might be applied.
For each area of projects, the projects should be assigned based on the priority level of the criticality solved (determined in phase 2) and according to the logical sequencing of different projects. Regarding this latter, the roadmap should tend to anticipate process-related actions and then, once defined an optimized process, intervene on the supporting technology side.
5. Application case
The actual usefulness and effectiveness of the TRM methodology were tested through diverse application cases. In this paper, the authors report one case that involved a multinational European manufacturer operating in the aerospace industry. The choice of such a case is due to the high complexity of the processes and products managed, the high-quality requirements imposed by the market, the high propensity toward I4.0 investments, and the growing concern toward sustainability aspects of the business.
The focus of the analysis was on the Italian plant devoted to the Assembly, Integration, and Test (AIT) of satellites for off-earth communication and space exploration. The facility analyzed was dedicated to the assembly, integration, and testing of small-medium class and high-volume satellites. It aimed at design and build a state-of-the-art Digital Factory utilizing I4.0 technologies. The objective of the site redesign, which involves the reorganization of flows, tools, work methods, and technologies, was aimed at optimizing and streamlining satellite production, thus reducing their lead times. In general, the designed solution had to meet the need for increased production volumes, transforming production from ‘bespoke’ to ‘mass customization.’ The company deals with three major product categories that differentiate by sizes and weights: micro-satellites (around 150 kg), medium satellites (around 1000 kg), and large satellites (around 2000 kg). The objective of this digitalization initiative was to find an I4.0-oriented solution adaptable to all three product families without requiring significant modifications to the plant whenever there is a switch in the product family being processed. Similarly, the identified solution had to achieve a time and OPEX savings of approximately 20%. In line with this, the management emphasized their willingness to implement an update process for the supporting ICT systems to ensure effective data and information collection.
As the market is growing, the company needed to increase production. Hence, apart from the need for process optimization, it wanted to expand the available area for AIT activities from 4000 m2 to 10,000 m2 within the next 5 years. The facility could produce 4 to 5 satellites per month, but the objective was to increase production to up to 30 satellites per month. This goal included the introduction of micro-satellite constellations.
Moreover, given the high cost and time to certificate procedures (European Customer Specifications-ECSs), the company was also willing to minimize the changes in processes for large satellites. On the other hand, no constraints were provided on the introduction of new technologies applied to the process. Finally, the application case regarded exclusively the operation processes of the AIT departments and ICT architecture, no interventions at supply chain nor product level were analyzed. For the operation processes, the authors refer to New Product Development & Engineering (NPD), Production (i.e. Assembly), Maintenance, Quality (i.e. Test), and Internal logistics.
The identification of criticalities and solutions was conducted through a 3 full days’ workshop on site, and 1 hands-on experience that involved 17 main managers and specialists of each of the processes abovementioned (Annex 1).
Additionally, a 2 full days training session on the digital solutions proposed was provided to an extended team of the company.
5.1. Criticalities
Overall, the methodology allowed the authors to detect 22 criticalities. These were detected through dedicated interviews with the abovementioned managers and specialists. Each interview was conducted by following the methodology proposed by (De Carolis, Citation2017; De Carolis et al., Citation2017) and encompassing the 6 operations processes.
Regarding NPD, synergies among programs by reusing data and information from similar products already made were rarely achieved thus extending the whole time to market needed to deliver a satellite. This effect was further exacerbated by the limited reliance on design methods aimed at facilitating AIT procedures throughout the operations phases. Another critical point referred to the strict certification specifications (i.e. ECSs) hampering the possibility to investigate disruptive process innovations. On the project management side, the firm was not able to properly foresee budgeted times and costs thus leading to problems in the subsequent planning phases.
The assembly area proved to suffer most of the criticalities highlighted. Overall, 10 main criticalities were identified. First, the Bill of Processes (BoP) was printed and compiled manually, and the Manufacturing Execution System (MES) was updated manually and asynchronously at the end of the shift. Moreover, limited data and events happening on the shopfloor were registered automatically on ICT systems and no lead times were recorded.
The manual activities in the A&D sector must often be performed by certified operators. In this regard, no autonomous system to monitor the expiring date of the certification was implemented thus exposing the company to the risk of generating non-conformances. Other criticalities referred to a wrong layout of the shopfloor which forced operators to leave their workplace to reach the tools/components and the reliance on inappropriate tools (e.g. Excel sheets to manage the stock).
Finally, the test phase underlined five additional criticalities which regarded a lack of planning, an onerous reporting system to register quality and test parameters, and a strong reliance on manual reporting and checking activities.
Such criticalities were linked to generated effects (20) and then prioritized accordingly. The identification of weight and links involved all the company stakeholders above mentioned and were neutrally facilitated by the authors as suggested by (Kerr et al., Citation2012).
The analysis permitted the identification of 74 causal relationships (Annex 2).
Each block was then translated into a matrix to determine quantitatively how many times a criticality impacted each effect. Following the methodology presented, the authors conducted another workshop to determine the weight of each link in terms of absolute impact and frequency and to define the impact of each effect on the objectives stated (Annex 2).
From the resulting analysis, shows the most impacting criticalities and effects.
Table 3. Most impacting criticalities and effects and their cumulated impact.
5.2. Solutions and roadmap
After the prioritization phase, 21 solutions were proposed and clustered into 3 areas, namely Process, Digital Backbone, and Industry 4.0. A description of the solutions proposed is available in Annex 3. These allowed the company to eliminate 18 out of the 22 criticalities and further mitigate 19 out of the 20 effects.
Given the high number of solutions proposed, the company, supported by the authors, opted to initially implement process-related solutions and then align the digital backbone. I4.0 technologies were catalogized into A-priority and B-priority based on the macro-business objective that they were supposed to tackle (see and Annex 4).
Accordingly, an analysis of the achievable benefits was provided for both scenarios (see Annex 5).
After the generation of the final TRM and the presentation to the management team, the authors conducted a last workshop to communicate the output to the main stakeholders affected by the transformation and collect feedback regarding the whole methodology followed. To this extent, approximately 30 company experts with different backgrounds and roles were involved. Miró web platform and PowerPoint were used to guide and facilitate the discussion. All the participants agreed with the structure of the roadmapping process, logical links, and level of detail adopted. In this direction, no further comments nor suggestions were provided.
Regarding the TRM layout, the discussion was guided by the following questions:
What criteria would you rely on to chronologically distribute projects?
What criteria would you adopt to define the expected effort required for a project?
From the analysis of the feedback received, emerged the need for integrating the process with an intermediate step (step 5.3b) consisting of a 2nd layer classification computed through a weighted scorecard encompassing ‘time distribution’ and ‘effort definition’ drivers. Among them, it emerged the necessity to identify the logical causality among projects, estimate financial resources, departments, skills and partners involved in each project, distinguishing between internal and outsources activities. Additionally, the participants agreed about the usefulness of introducing a distinction of Education projects into ‘Learning-oriented’ and ‘Training-oriented’ and define a priori the complexity, format, granularity, quantity, and quality of the expected necessary data to run the projects. The detailed list of answers provided is available in Annex 6.
The findings resulting from the employed methodology were subsequently harnessed by the company to formulate a comprehensive project proposal. This resulted in the company’s triumph in a publicly solicited tender (PNRR – Sub investment MIC2.I4.3-Space Factory) initiated by the Italian Space Agency (ASI) and EU. The tender specifically pertained the establishment of a novel aerospace manufacturing facility. Consequently, the company is currently in the process of implementing the proposed solutions put forth by the authors.
6. Discussions and conclusions
Although the relationship between I4.0 technology and sustainability is extensively investigated in literature and proven to be virtuous, manufacturing companies still struggle to apply such concepts and make TT real. Among these causes, the authors identified 4 barriers tackled by this research that refer to a lack of practical models, evaluations, and examples able to consider the specific and real needs of practitioners (Abdul-Hamid et al., Citation2020; Bag et al., Citation2022; Trevisan et al., Citation2023). Over time, many tools were proposed and TRM proved to be among the most effective.
This paper presents a TRM methodology resulting in a unique tool able to address both strategic business objectives and sustainability ones, through practical actions of a management nature and technological implementations to support the TT of manufacturing companies.
The authors conducted a SLR that highlighted the relevance of TRM in I4.0-enabled transformation processes and clustered TRM into PTR, ETR, and IOR (Garcia & Bray, Citation1997). Then, a critical review of the currently available TRMs enhancing TT was performed. The literature highlighted the lack of a multi-perspective and multi-objective but operative roadmap able to tangibly support TT based on specific strategic objectives seemed to be missing.
The methodology presented by the authors is divided into five steps, namely: Goal and KPIs, Criticalities, Solution Proposal, TRM, and Project management. Each step was described in terms of content and sub-phases. Depending on the time and resources available, the authors developed a double, mutually exclusive criticality identification step that could be based either on a qualitative or a quantitative approach.
Most of the methodologies presented in the literature are devoted to product-related TRM (PTR) or TRM aiming at introducing or updating products and exploit technologies as enablers. In this work, the methodology proposed adopts a different perspective thus being focused on manufacturing processes.
In addition, the methodology originates from a twofold analysis that encompasses a study of existing TRM available literature and the analysis of companies’ actual needs and barriers faced while addressing TT. The methodology developed was tested and validated in multiple industrial cases and the results from an Aerospace manufacturing company were presented.
Regarding the contribution to the theory, the paper clusters and describes the main typologies of TRM available in the literature. Therefore, it provides an analysis of the main TRM methodologies/tools available in the literature and presents the possible managerial tools and decision-support techniques that might synergically be integrated with TRM. Multiple contributions addressed TRM under a I4.0 perspective with no focus on sustainability (Hilary & Daim, Citation2012; Jeon et al., Citation2011; Kerr et al., Citation2006; Sufian et al., Citation2021). The authors contributed to theory by introducing an additional dimension considering environmental sustainability as a driving objective of the whole methodology. TRM has been effectively integrated with several managerial and decision-making tools, including QFD (Hilary & Daim, Citation2012), SWOT (Ramos et al., Citation2022), AHP (Verma et al., Citation2022), TOPSIS (Sajjad et al., Citation2022), Delphi (da Silveira Junior et al., Citation2018) and others, which were chosen based on the specific scope of TRM and the corresponding domain of investigation. Nevertheless, a deficiency is observed in the rationale for establishing linkages and quantifying relationships among criticalities and their corresponding effects (Phaal & Muller, Citation2009; Valério et al., Citation2020). Consequently, this deficiency undermines the robustness of prioritizing actions aimed at enhancing the roadmap’s efficacy and performance (Valério et al., Citation2020). To overcome such deficiency, the proposed methodology integrates system dynamics theory, multi-objective decision models and multi-attribute decision models to TRM domain.
Hence, this article proposes a methodology to integrate I4.0 and economic and environmental sustainability in manufacturing, reviews the academic literature of TRM for TT and provides empirical evidence from an Italian manufacturer. Different from other domain-specific or industry-specific methodologies (Harmon et al., Citation2012; Perossa et al., Citation2023), the proposed one addresses TT from a holistic perspective. To achieve this, the research proposes a methodology to identify and prioritize impediments to TT. Consequently, it integrates the results into a complete TRM methodology to foster Sustainable and Digital Transition in the manufacturing domain.
The research also underlines the multi-perspective nature of TT, emphasising the role of Process and Digital Backbone optimisation.
The methodology also combines aspects functional to the achievement of strategic and operational performances, such as good management practices, and the functionality of digital technologies in a single framework.
Regarding the contribution to practice and the managerial implications, this document provides manufacturing companies with a practical tool to undertake a TT in a facilitated way thus supporting managers in identifying strategic investments and planning actions and resources in a defined timeframe. Thanks to the use of the methodology, it was possible to ascertain various advantages in the company involved as an application case. The main ones include 1) the ability to integrate long-term strategic objectives into practical and operational activities, 2) the ability to orchestrate functional and departmental objectives of the various managers in a single operational plan, 3) the ability to generate awareness of drawbacks of the critical issues generated within a function in downstream activities, 4) the ability to link business and sustainability objectives through actions that directly affect both positively, and 5) the ability to generate awareness of the activities to plan and execute both earlier than during digital technology implementation projects. Also, the research emphasizes of relying on a holistic approach by considering operations’ physical and information flow and investigating possible synergies with other functions. Thus, the methodology aims to foster commitment within the organizations through the engagement of employees ranging from top management to shopfloor operators.
The following research presents some limitations and boundaries. First, the methodology does not consider project risk management dimensions, thus limiting its applicability in the high uncertainty sector. Furthermore, no quantitative evaluation of economic and sustainability aspects has been developed yet. Future studies will be devoted to further validating the methodology proposed and refining it based on the insights collected, extending it by introducing a risk management-related step, further consolidating the KPI definition phase as well as applying the model in a multi-sector manufacturing application case.
Disclosure statement
No potential conflict of interest was reported by the author(s).
References
- Abdul-Hamid, A. Q., Ali, M. H., Tseng, M. L., Lan, S., & Kumar, M. (2020). Impeding challenges on industry 4.0 in circular economy: Palm oil industry in Malaysia. Computers & Operations Research, 123(November), 105052. Elsevier Ltd. https://doi.org/10.1016/j.cor.2020.105052
- Acerbi, F., Järnefelt, V., Martins, J. T., Saari, L., Valkokari, K., & Taisch, M. (2021). Developing a qualitative maturity scale for circularity in manufacturing. In A. Dolgui, A. Bernard, D. Lemoine, & G. von Cieminski. and D. Romero (Eds.), Department of management, Economics and Industrial Engineering, Politecnico Di Milano, Piazza Leonardo da Vinci 32. Springer Science and Business Media Deutschland GmbH. https://doi.org/10.1007/978-3-030-85906-0_42
- Acerbi, F., Spaltini, M., & Taisch, M. (2022). Developing a roadmap towards the digital transformation of small & medium companies: A case study analysis in the a & D sector roadmap. 3–35.
- Acerbi, F., & Taisch, M. (2020). Information flows supporting circular economy adoption in the manufacturing sector. Springer. https://doi.org/10.1007/978-3-030-57997-5_81
- Agrawal, R., Yadav, V. S., Majumdar, A., Kumar, A., Luthra, S., & Garza-Reyes, J. A. (2023). Opportunities for disruptive digital technologies to ensure circularity in supply chain: A critical review of drivers, barriers and challenges. Computers & Industrial Engineering, 178(April), 109140. Elsevier Ltd. https://doi.org/10.1016/j.cie.2023.109140
- Arman, H., Gindy, N., Kabli, M., & Cavin, S. (2014). Integrated technology roadmapping tool to aid the decision-making of r&d investments. International Journal of Innovation and Technology Management, 11(2), 1450006. World Scientific Publishing Co. Pte Ltd. https://doi.org/10.1142/S0219877014500060
- Bag, S., Gupta, S., & Kumar, S. (2021). Industry 4.0 adoption and 10R advance manufacturing capabilities for sustainable development. International Journal of Production Economics, 231(January), 107844. Elsevier B.V. https://doi.org/10.1016/j.ijpe.2020.107844
- Bag, S., Sahu, A. K., Kilbourn, P., Pisa, N., Dhamija, P., & Sahu, A. K. (2022). Modeling barriers of digital manufacturing in a circular economy for enhancing sustainability. International Journal of Productivity & Performance Management, 71(3), 833–869. Emerald Group Holdings Ltd. https://doi.org/10.1108/IJPPM-12-2020-0637
- Balanay, R. M., Varela, R. P., & Halog, A. B. (2022). Circular economy for the sustainability of the wood-based industry: The case of Caraga region, Philippines. In Circular economy and sustainability (pp. 447–462). Elsevier. https://doi.org/10.1016/B978-0-12-821664-4.00016-9
- Bernal, L., Dornberger, U., Torres, O., & Byrnes, T. (2009). Technology roadmapping handbook. International SEPT Program, (June), 1–17. https://d1wqtxts1xzle7.cloudfront.net/53460543/Handbook_Roadmapping-libre.pdf?1497130487=&response-content-disposition=inline%3B+filename%3DTechnology_Roadmapping_International_SEP.pdf&Expires=1704727177&Signature=N~ZZH3W95nFCvNy~VetdWE2SOnmg3bkpaaibTafRLARCuersNvzqm1owImPpi7AUsc~GR7f~ci7uYOYM7CRF7ZNse-SSLedwfMYRij8UqD9xjqkXtjywiN6eLGNM1HPjb87jBANMZxsQfnMalrIjhxYRhuTpEB-wXv2Wi9eUs9K~pY-blMlbxqw3hZC6jhq9wilja6vD8kBkVIKGdl1-mizch5Orl~1Y3U~qkDOosWCX5pFvq09PC-z51qkqyKBydw22VEPRwxpAbI5taVuGgBvNguOIWjOohKGnOKeI4pzhQv6Dp026HExkPXqxovP-5ILssoMquHjMdCLnRc4vUA__&Key-Pair-Id=APKAJLOHF5GGSLRBV4ZA
- Blessing, L. T. M., & Chakrabarti, A. (2009). DRM, a Design Research Methodology. Springer London. https://doi.org/10.1007/978-1-84882-587-1
- Butt, J. (2020). A strategic roadmap for the manufacturing industry to implement industry 4.0. Designs, 4(2), 1–31. https://doi.org/10.3390/designs4020011
- Chih Jen, Y., & Daim, T. (2017, January). Benchmarking of technology roadmapping process in energy sector: A literature review. PICMET 2017 - Portland International Conference on Management of Engineering and Technology: Technology Management for the Interconnected World, Proceedings (pp. 1–10). https://doi.org/10.23919/PICMET.2017.8125438.
- Ching, N. T., Ghobakhloo, M., Iranmanesh, M., Maroufkhani, P., & Asadi, S. (2022). Industry 4.0 applications for sustainable manufacturing: A systematic literature review and a roadmap to sustainable development. Journal of Cleaner Production, 334(February), 130133. Elsevier Ltd. https://doi.org/10.1016/j.jclepro.2021.130133
- Ciliberto, C., Szopik-Depczyńska, K., Tarczyńska-Łuniewska, M., Ruggieri, A., & Ioppolo, G. (2021). Enabling the circular economy transition: A sustainable lean manufacturing recipe for industry 4.0. Business Strategy and the Environment, 30(7), 3255–3272. John Wiley and Sons Ltd. https://doi.org/10.1002/bse.2801
- Contini, G., & Peruzzini, M. (2022). Sustainability and industry 4.0: Definition of a set of key performance indicators for manufacturing companies. Sustainability (Switzerland). MDPI. https://doi.org/10.3390/su141711004
- Cortés, D., Ramírez, J., Ponce, P., & Molina, A. (2021, July). S3 manufacturing process taxonomy. Journal of Manufacturing Processes, 67, 579–610. Elsevier Ltd. https://doi.org/10.1016/j.jmapro.2021.04.069
- Cotrino, A., Sebastián, M. A., & González-Gaya, C. (2020). Industry 4.0 Roadmap: Implementation for Small and Medium-Sized Enterprises. Applied Sciences (Switzerland), 10(23), 1–17. https://doi.org/10.3390/app10238566
- Culot, G., Nassimbeni, G., Orzes, G., & Sartor, M. (2020). Behind the definition of industry 4.0: Analysis and open questions. International Journal of Production Economics, 226(October 2019), 107617. Elsevier B.V.: 107617. https://doi.org/10.1016/j.ijpe.2020.107617
- Dalenogare, L. S., Benitez, G. B., Ayala, N. F., & Frank, A. G. (2018). The expected contribution of industry 4.0 technologies for industrial performance. International Journal of Production Economics, 204(December 2017), 383–394. Elsevier B.V. https://doi.org/10.1016/j.ijpe.2018.08.019
- da Silveira Junior, B., Luiz, A., Vasconcellos, E., Guedes, L. V., Costa, R. M., & Guedes, L. F. A. (2018). Technology roadmapping: A methodological proposition to refine delphi results. Technological Forecasting & Social Change, 126(August 2017), 194–206. https://doi.org/10.1016/j.techfore.2017.08.011
- Dawson, C. (2009). Introduction to research methods: A practical guide for anyone undertaking a research project (4th ed., Edited by, Robinson.). Hoe to books.
- de Alcantara, D. P., & Martens, M. L. (2019). Technology roadmapping (TRM): A systematic review of the literature focusing on models. Technological Forecasting & Social Change, 138(July 2018), 127–138. Elsevier. https://doi.org/10.1016/j.techfore.2018.08.014
- de Bruin, T., Freeze, R., Kulkarni, U., Rosemann, M., de Bruin, Sydney de Bruin, Ronald Freeze, & Carey, W. P. (2005). Understanding the main phases of developing a maturity assessment model. 16th Australasian Conference on Information Systems (ACIS), Sydney. 109. http://aisel.aisnet.org/acis2005/109
- De Carolis, A. (2017). A methodology to guide manufacturing companies towards digitalization. Politecnico di Milano. https://www.politesi.polimi.it/handle/10589/136972?mode=complete
- De Carolis, A., Macchi, M., Negri, E., & Terzi, S. (2017). A maturity model for assessing the digital readiness of manufacturing companies. IFIP Advances in Information and Communication Technology, 513, 13–20. https://doi.org/10.1007/978-3-319-66923-6_2
- de Mattos Nascimento, D. L., Quelhas, O. L. G., Moyano-Fuentes, J., Tortorella, G. L., & Maqueira, J. M. (2022). Circular value stream mapping 4.0: Proposed general model and application to a digital 3D printing recycling factory. Sustainable Production and Consumption, 34(November), 600–612. https://doi.org/10.1016/j.spc.2022.10.012
- Denyer, D., & Tranfield, D. (2009). Producing a systematic review. In D. A. Buchanan & A. Bryman (Eds.), The sage handbook of organizational research methods (pp. 671–689). SAGE.
- Dev, N. K., Shankar, R., & Qaiser, F. H. (2020). Industry 4.0 and circular economy: Operational excellence for sustainable reverse supply chain performance. Resources, Conservation and Recycling, 153(February), 104583. https://doi.org/10.1016/j.resconrec.2019.104583
- European Commission. (2003). COMMISSION RECOMMENDATION of 6 May 2003 concerning the definition of micro, small and medium-sized enterprises. Official Journal of the European Union. L124/36. https://doi.org/10.1093/nq/s10-I.5.88-c
- European Sustainable Business Federation. (2019). Final Report. Circular Economy Update: Overview of Circular Economy in Europe.
- Farrukh, C., Kerr, C., Phaal, R., Athanassopoulou, N., & Routley, M. (2014). Light-weighting innovation strategy: A roadmap-portfolio toolkit. Proceedings of PICMET ‘14 Conference: Portland International Center for Management of Engineering and Technology; Infrastructure and Service Integration, Kanazawa, Japan (pp. 97–101).
- Ford, S., & Despeisse, M. (2016). Additive manufacturing and sustainability: An exploratory study of the advantages and challenges. Journal of Cleaner Production, 137, 1573–1587. https://doi.org/10.1016/j.jclepro.2016.04.150
- Garcia, M. L., & Bray, O. H. (1997). Fundamentals of technology roadmapping.
- Garcia-Muiña, F., González-Sánchez, R., Ferrari, A., & Settembre-Blundo, D. (2018). The paradigms of industry 4.0 and circular economy as enabling drivers for the competitiveness of businesses and territories: The case of an Italian ceramic tiles manufacturing company. Social Sciences, 7(12), 255. https://doi.org/10.3390/socsci7120255
- Gerdsri, N., Puengrusme, S., Vatananan, R., & Tansurat, P. (2019). Conceptual framework to assess the impacts of changes on the status of a roadmap. Journal of Engineering and Technology Management - JET-M, 52(January 2018), 16–31. Elsevier. https://doi.org/10.1016/j.jengtecman.2017.12.001
- Gerdsri, N., & Vatananan, R. S. (2007). Dynamics of technology roadmapping (TRM) implementation. Portland International Conference on Management of Engineering and Technology (pp. 1577–1583). https://doi.org/10.1109/PICMET.2007.4349482.
- Geum, Y., Jeong Lee, H., Lee, Y., & Park, Y. (2015). Development of data-driven technology roadmap considering dependency: An ARM-Based technology roadmapping. Technological Forecasting & Social Change 91, 264–279. Elsevier Inc. https://doi.org/10.1016/j.techfore.2014.03.003
- Ghobakhloo, M., Iranmanesh, M., Grybauskas, A., Vilkas, M., & Petraitė, M. (2021). Industry 4.0, innovation, and sustainable development: A systematic review and a roadmap to sustainable innovation. Business Strategy and the Environment, 30(8), 4237–4257. https://doi.org/10.1002/bse.2867
- Greenhalgh, T. (1997). How to read a paper: Papers that summarise other papers (systematic reviews and meta-analyses). BMJ, 315(7109), 672–675. https://doi.org/10.1136/bmj.315.7109.672
- Halse, L. L., & Jæger, B. (2019). Operationalizing industry 4.0: Understanding barriers of industry 4.0 and circular economy. IFIP Advances in Information and Communication Technology, 567(October), 135–142. https://doi.org/10.1007/978-3-030-29996-5_16
- Han, M., & Geum, Y. (2022). Roadmapping for data: Concept and typology of data-integrated smart-service roadmaps. IEEE Transactions on Engineering Management, 69(1), 142–154. IEEE. https://doi.org/10.1109/TEM.2020.3013295
- Hansen, C., Daim, T., Ernst, H., & Herstatt, C. (2016). The future of rail automation: A scenario-based technology roadmap for the rail automation market. Technological Forecasting and Social Change, 110, 196–212. Elsevier Inc. https://doi.org/10.1016/j.techfore.2015.12.017
- Harmon, R. R., Demirkan, H., Raffo, D., & Daim, T. (2012). Roadmapping the next wave of sustainable IT. Foresight, 14(2), 121–138. https://doi.org/10.1108/14636681211222401
- Hilary, M., & Daim, T. U. (2012). Technology roadmap development process (TRDP) for the service sector: A conceptual framework. Technology in Society, 34(1), 94–105. https://doi.org/10.1016/j.techsoc.2012.01.003
- Holmström, J., Ketokivi, M., & Hameri, A.-P. (2009). Bridging practice and theory: A design science approach. The Author Journal Compilation C, 40(1), 65–87. Decision Sciences Institute. https://doi.org/10.1111/j.1540-5915.2008.00221.x
- Hopkins, J. L. (2021). An investigation into emerging industry 4.0 technologies as drivers of supply chain innovation in Australia. Computers in Industry 125, 103323. Elsevier B.V.: 103323. https://doi.org/10.1016/j.compind.2020.103323
- Howard, M., Yan, X., Mustafee, N., Charnley, F., Böhm, S., & Pascucci, S. (2022). Going beyond waste reduction: Exploring tools and methods for circular economy adoption in small-medium enterprises. Resources, Conservation & Recycling, 182(July), 106345. Elsevier B.V. https://doi.org/10.1016/j.resconrec.2022.106345
- Jeffrey, V., Kshetri, N., & DeFranco, J. F. (2021). Scarcity and global insecurity: The semiconductor shortage. IT Professional, 23(5), 78–82. https://doi.org/10.1109/MITP.2021.3105248
- Jenney, J., Gangl, M., Kwolek, R., Melton, D., Ridenour, N., & Coe, M. (2010). Modern methods of systems engineering: With an introduction to pattern and model based methods. CreateSpace Independent Publishing Platform.
- Jeon, J., Lee, H., & Park, Y. (2011). Implementing technology roadmapping with supplier selection for semiconductor manufacturing companies. Technology Analysis and Strategic Management, 23(8), 899–918. https://doi.org/10.1080/09537325.2011.604156
- Johnson, B. T., Low, R. E., & LaCroix, J. M. (2013). Systematic reviews to support evidence-based medicine (2nd edition) by Khalid Khan, Regina Kunz, Jos Kleijnen and Gerd Antes: A review. Research Synthesis Methods, 4(1), 102–108. https://doi.org/10.1002/jrsm.1071
- Kagermann, H., Wahlster, W., & Helbig, J. (2013). Recommendations for implementing the strategic initiative INDUSTRIE 4.0 – securing the future of German manufacturing industry. https://www.bibsonomy.org/bibtex/25c352acf1857c1c1839c1a11fe9b7e6c/flint63%0Ahttp://forschungsunion.de/pdf/industrie_4_0_final_report.pdf.
- Kerr, C., Farrukh, C., Phaal, R., & Probert, D. (2013). Key principles for developing industrially relevant strategic technology management toolkits. Technological Forecasting and Social Change, 80(6), 1050–1070. Elsevier Inc.: 1050–70. https://doi.org/10.1016/j.techfore.2012.09.006
- Kerr, C., & Phaal, R. (2019). Defining the scope of a roadmapping initiative: A checklist-based template for organizational stakeholders. 2019 Portland International Conference on Management of Engineering and Technology (PICMET), Portland, USA (pp. 1–10). https://doi.org/10.23919/PICMET.2019.8893851
- Kerr, C., & Phaal, R. (2022). Roadmapping and roadmaps: Definition and underpinning concepts. IEEE Transactions on Engineering Management, 69(1), 6–16. IEEE. https://doi.org/10.1109/TEM.2021.3096012
- Kerr, C., Phaal, R., & Probert, D. (2006). A framework for strategic military capabilities in defence transformation. 11th International Command and Control Research and Technology Symposium: Coalition Command and Control in the Networked Era, 44, 0–20. http://www.dodccrp.org/events/11th_ICCRTS/html/papers/061.pdf
- Kerr, C., Phaal, R., & Probert, D. (2012). Cogitate, articulate, communicate: The psychosocial reality of technology roadmapping and roadmaps. R and D Management, 42(1), 1–13. https://doi.org/10.1111/j.1467-9310.2011.00658.x
- Kerr, C., Phaal, R., & Probert, D. (2018). Addressing the cognitive and social influence inhibitors during the ideation stages of technology roadmapping workshops. Technology Roadmapping, 655–686. IEEE. https://doi.org/10.1142/9789813235342
- Khan, S., Imran Khan, M., & Haleem, A. (2020). Prioritisation of challenges towards development of smart manufacturing using BWM method. In Internet of things (IoT): Concepts and applications (pp. 409–426). Springer International Publishing. https://doi.org/10.1007/978-3-030-37468-6_22
- Kirchherr, J., Reike, D., & Hekkert, M. (2017). Conceptualizing the circular economy: An analysis of 114 definitions. Resources, Conservation and Recycling, 127(April), 221–232. https://doi.org/10.1016/j.resconrec.2017.09.005
- Kumar, R., Kr Singh, R., & Kr Dwivedi, Y. (2020). Application of industry 4.0 technologies in SMEs for ethical and sustainable operations: Analysis of challenges. Journal of Cleaner Production, 275, 124063. Elsevier Ltd. https://doi.org/10.1016/j.jclepro.2020.124063
- Kumar, P., Kr Singh, R., & Kumar, V. (2021). Managing supply chains for sustainable operations in the era of industry 4.0 and circular economy: Analysis of barriers. Resources, Conservation & Recycling, 164(January), 105215. Elsevier B.V. https://doi.org/10.1016/j.resconrec.2020.105215
- Kumar, V., Vrat, P., & Shankar, R. (2021). Prioritization of strategies to overcome the barriers in industry 4.0: A hybrid MCDM approach. OPSEARCH, 58(3), 711–750. Springer. https://doi.org/10.1007/s12597-020-00505-1
- Lai, W. L., Sharma, S., Roy, S., Maji, P. K., Sharma, B., Ramakrishna, S., & Goh, K. L. (2022). Roadmap to sustainable plastic waste management: A focused study on recycling PET for triboelectric nanogenerator production in Singapore and India. Environmental Science and Pollution Research, 29(34), 51234–51268. https://doi.org/10.1007/s11356-022-20854-2
- Lamperti, S., Cavallo, A., & Sassanelli, C. (2023). Digital Servitization and Business Model Innovation in SMEs: A Model to Escape From Market Disruption. IEEE Transactions on Engineering Management Institute of Electrical and Electronics Engineers Inc. 1–15. https://doi.org/10.1109/TEM.2022.3233132
- Lee, S., & Park, Y. (2005). Customization of technology roadmaps according to roadmapping purposes: Overall process and detailed modules. Technological Forecasting and Social Change, 72(5), 567–583. https://doi.org/10.1016/j.techfore.2004.11.006
- Leila, S., Vafa, J., Katri, V., Jorge, T. M., & Federica, A. (2021). Towards sustainable manufacturing through collaborative circular economy strategies. In L. M. Camarinha-Matos, X. Boucher, & H. Afsarmanesh (Eds.), Smart and sustainable collaborative networks 4.0 (pp. 362–373). Springer International Publishing.
- Lerman, L. V., Benitez, G. B., Müller, J. M., de Sousa, P. R., & Frank, A. G. (2022). Smart green supply chain management: A configurational approach to enhance green performance through digital transformation. Supply Chain Management, 27(7), 147–176. Emerald Group Holdings Ltd. https://doi.org/10.1108/SCM-02-2022-0059
- Lichtenthaler, U. (2008). Opening up strategic technology planning: Extended roadmaps and functional markets. Management Decision, 46(1), 77–91. https://doi.org/10.1108/00251740810846752
- Lopes de Sousa Jabbour, A. B., Jabbour, C. J. C., Godinho Filho, M., & Roubaud, D. (2018). Industry 4.0 and the circular economy: A proposed research agenda and original roadmap for sustainable operations. Annals of Operations Research, 270(1–2), 273–286. https://doi.org/10.1007/s10479-018-2772-8
- Marcucci, G., Antomarioni, S., Ciarapica, F. E., & Bevilacqua, M. (2021). The Impact of Operations and IT-Related industry 4.0 key technologies on organizational resilience. Production Planning & Control, 1–15. Taylor & Francis. https://doi.org/10.1080/09537287.2021.1874702
- Mauro, C., & Amaral, D. C. (2011). Roadmapping for technology push and partnership: A contribution for open innovation environments. Technovation, 31(7), 320–335. Elsevier. https://doi.org/10.1016/j.technovation.2011.01.005
- Mittal, S., Ahmad Khan, M., Romero, D., & Wuest, T. (2018). A Critical Review of Smart Manufacturing & Industry 4.0 Maturity Models: Implications for Small and Medium-Sized Enterprises (SMEs). Journal of Manufacturing Systems, 49(October), 194–214. Elsevier. https://doi.org/10.1016/j.jmsy.2018.10.005
- Mogos, M. F., & Fragapane, G. (2022). Ways to circular and transparent value chains. In D. Y. Kim, G. von Cieminski, & D. Romero (Eds.), Advances in production management systems. Smart manufacturing and logistics systems: Turning ideas into action. APMS 2022 (pp. 390–398). https://doi.org/10.1007/978-3-031-16411-8_45
- Mubarik, M. S., Naghavi, N., Mubarik, M., Kusi-Sarpong, S., Ahmed Khan, S., Imran Zaman, S., & Kazmi, S. H. A. (2021). Resilience and cleaner production in industry 4.0: Role of supply chain mapping and visibility. Journal of Cleaner Production 292, 126058. Elsevier Ltd: 126058. https://doi.org/10.1016/j.jclepro.2021.126058
- Okorie, O., Subramoniam, R., Charnley, F., Patsavellas, J., Widdifield, D., & Salonitis, K. (2020). Manufacturing in the time of COVID-19: An assessment of barriers and enablers. IEEE Engineering Management Review, 48(3), 167–175. https://doi.org/10.1109/EMR.2020.3012112
- Oliveira, M. G., Routley, M., Phaal, R., & Mendes, G. H. S. (2019, August). The concept of “roadmapping service”: Exploring customer perspectives of roadmapping through the service lens. Proceedings of the International Conference on Engineering Design, ICED (pp. 3101–3110). https://doi.org/10.1017/dsi.2019.317.
- Park, H., Phaal, R., Jae Yun, H., & O’Sullivan, E. (2020). Twenty years of technology and strategic roadmapping research: A school of thought perspective. Technological Forecasting & Social Change, 154(February), 119965. Elsevier. https://doi.org/10.1016/j.techfore.2020.119965
- Perossa, D., Acerbi, F., Rocca, R., Fumagalli, L., & Taisch, M. (2023, December). Twin transition cosmetic roadmapping tool for supporting cosmetics manufacturing. Cleaner Environmental Systems, 11, 100145. Elsevier Ltd. https://doi.org/10.1016/j.cesys.2023.100145
- Pezzotta, G., Sassanelli, C., Pirola, F., Sala, R., Rossi, M., Fotia, S., Koutoupes, A., Terzi, S., & Mourtzis, D. (2018). The product service system lean design methodology (PSSLDM): Integrating product and service components along the whole PSS lifecycle. Journal of Manufacturing Technology Management, 29(8), 1270–1295. Emerald Group Holdings Ltd. https://doi.org/10.1108/JMTM-06-2017-0132
- Phaal, R., & Muller, G. (2009). An architectural framework for roadmapping: Towards visual strategy. Technological Forecasting and Social Change, 76(1), 39–49. Elsevier Inc. https://doi.org/10.1016/j.techfore.2008.03.018
- Phaal, R., O’Sullivan, E., Routley, M., Ford, S., & Probert, D. (2011). A framework for mapping industrial emergence. Technological Forecasting & Social Change, 78(2), 217–230. IEEE. https://doi.org/10.1016/j.techfore.2010.06.018
- Porter, M. E. (2008). The five competitive forces that shape strategy. Special Issue on HBS Centennial. Harvard Business Review, 86(1), 78–93.
- Potts, C. (1993). Software-engineering research revisited. IEEE Software, 10(5), 19–28. https://doi.org/10.1109/52.232392
- Prakash, G., & Ambedkar, K. (2022, October). Digitalization of manufacturing for implanting value, configuring circularity and achieving sustainability. Journal of Advances in Management Research, 20(1), 116–139. https://doi.org/10.1108/JAMR-01-2022-0010
- Rafael, C., Amaral, D. C., & Caetano, M. (2018). Framework for continuous agile technology roadmap updating. Innovation and Management Review, 15(3), 321–336. Emerald Group Holdings Ltd. https://doi.org/10.1108/INMR-05-2018-0030
- Ramos, A. G., Daim, T., Gaats, L., Hutmacher, D. W., & Hackenberger, D. (2022). Technology roadmap for the development of a 3D cell culture workstation for a biomedical industry startup. Technological Forecasting & Social Change, 174(January), 121213. Elsevier Inc. https://doi.org/10.1016/j.techfore.2021.121213
- Rehman, S. U., Giordino, D., Zhang, Q., & Alam, G. M. (2023, March). Twin transitions & industry 4.0: Unpacking the relationship between digital and green factors to determine green competitive advantage. Technology in Society, 73, 102227. https://doi.org/10.1016/j.techsoc.2023.102227
- Rizos, V., Behrens, A., van der Gaast, W., Hofman, E., Ioannou, A., Kafyeke, T., Flamos, A., Rinaldi, R., Papadelis, S., Hirschnitz-Garbers, M., & Topi, C. (2016). Implementation of circular economy business models by small and medium-sized enterprises (SMEs): Barriers and enablers. Sustainability, 8(11), 1212. https://doi.org/10.3390/su8111212
- Robert, P., Clare, J. P. F., Mills, J. F., & Probert, D. R. (2003). Customizing the Technology Roadmapping Approach. Portland International Conference on Management of Engineering and Technology (pp. 361–369). https://doi.org/10.1109/picmet.2003.1222814.
- Robert, P., Clare, J. P. F., & Probert, D. R. (2004). Technology roadmapping - a planning framework for evolution and revolution. Technological Forecasting and Social Change, 71(1–2), 5–26. https://doi.org/10.1016/S0040-1625(03)00072-6
- Rosa, P., Sassanelli, C., Urbinati, A., Chiaroni, D., & Terzi, S. (2020). Assessing relations between circular economy and industry 4.0: A systematic literature review. International Journal of Production Research, 58(6), 1662–1687. Taylor & Francis. https://doi.org/10.1080/00207543.2019.1680896
- Sajjad, A., Ahmad, W., & Hussain, S. (2022). Decision making process development for industry 4.0 transformation. Advances in Science and Technology Research Journal, 16(3), 1–11. Politechnika Lubelska. https://doi.org/10.12913/22998624/147237
- Sassanelli, C., Pezzotta, G., Pirola, F., Rossi, M., & Terzi, S. (2019). The PSS design GuRu methodology: Guidelines and rules generation to enhance PSS detailed design. Journal of Design Research, 17(2–4), 125–162. https://doi.org/10.1504/JDR.2019.105756
- Sassanelli, C., Rossi, M., & Terzi, S. (2020). Evaluating the smart maturity of manufacturing companies along the product development process to set a PLM project roadmap. International Journal of Product Lifecycle Management, 12(3), 185–209. https://doi.org/10.1504/IJPLM.2020.109789
- Sassanelli, C., & Terzi, S. (2022). The D-BEST reference model: A flexible and sustainable support for the Digital transformation of small and medium enterprises. Global Journal of Flexible Systems Management, 23(3), 345–370. Springer. https://doi.org/10.1007/s40171-022-00307-y
- Sautter, B. (2016). Futuring European industry: Assessing the ManuFuture road towards EU re-industrialization. European Journal of Futures Research, 4(1), 25. https://doi.org/10.1007/s40309-016-0100-6
- Schmidt, M.-C., Veile, J. W., Müller, J. M., & Voigt, K.-I. (2021). Ecosystems 4.0: Redesigning global value chains. The International Journal of Logistics Management, 32(4), 1124–1149. https://doi.org/10.1108/IJLM-03-2020-0145
- Schmitt, P., Schmitt, J., & Engelmann, B. (2020). Evaluation of proceedings for SMEs to conduct I4.0 projects. Procedia CIRP, 86, 257–263. Elsevier B.V. https://doi.org/10.1016/j.procir.2020.01.007
- Schöggl, J. P., Rusch, M., Stumpf, L., & Baumgartner, R. J. (2023, January). Implementation of digital technologies for a circular economy and sustainability management in the manufacturing sector. Sustainable Production and Consumption, 35, 401–420. Elsevier B.V. https://doi.org/10.1016/j.spc.2022.11.012
- Siddique, S., Bhuson Roy, B., Zaman, S., Khan, A., Al Alam, M. A., Chowdhury, R. B., Biswas, K. F., Hossain, M. M., Murakami, S., & Sujauddin, M. (2022). Discerning the circularity of the plastic industry in bangladesh through the lens of material flow analysis. Sustainable Production and Consumption, 33(September), 700–715. https://doi.org/10.1016/j.spc.2022.08.001
- Siripongpreda, T., Rodthongkum, N., & Ummartyotin, S. (2021). A critical review on cellulose wastes as the novel substrates for colorimetric and electrochemical sensors. Current Research in Green and Sustainable Chemistry, 4, 100190. https://doi.org/10.1016/j.crgsc.2021.100190
- Spaltini, M., Acerbi, F., Pinzone, M., Gusmeroli, S., & Taisch, M. (2022). Defining the roadmap towards industry 4. 0: The 6Ps maturity model for manufacturing SMEs. In 29th CIRP Life Cycle Engineering Conference, Leuven, Belgium.
- Spaltini, M., Acerbi, F., & Taisch, M. (2023). Development of an industry 4.0-oriented tool supporting circular manufacturing: A systematic literature review. Product Lifecycle Management PLM in Transition Times: The Place of Humans and Transformative Technologies, 609–619. https://doi.org/10.1007/978-3-031-25182-5_59
- Sterman, J. D. (2001). System dynamics modeling: Tools for learning in a complex world. California Management Review, 43(4), 8–25. https://doi.org/10.2307/41166098
- Sufian, A. T., Abdullah, B. M., Ateeq, M., Wah, R., & Clements, D. (2021). Six‐gear roadmap towards the smart factory. Applied Sciences (Switzerland), 11(8), 3568. MDPI AG. https://doi.org/10.3390/app11083568
- Talla, A., & McIlwaine, S. (2022, September). Industry 4.0 and the circular economy: Using design-stage digital technology to reduce construction waste. Smart & Sustainable Built Environment. https://doi.org/10.1108/SASBE-03-2022-0050
- Trevisan, A. H., Lobo, A., Guzzo, D., de Vasconcelos Gomes, L. A., & Mascarenhas, J. (2023). Barriers to employing digital technologies for a circular economy: A multi-level perspective. Journal of Environmental Management, 332(April), 117437. Academic Press. https://doi.org/10.1016/j.jenvman.2023.117437
- United Nations. (2020). The Sustainable Development Goals Report 2020. 10.29171/azuackupamphletk3240s8782016
- Urbán, K., Bíró-Szigeti, S., & Pataki, B. (n.d.). Technology road-mapping of final-energy generator technologies, for the industrial sector, in developed european economies. Acta Polytechnica Hungarica, 19(4), 65–84. https://doi.org/10.12700/APH.19.4.2022.4.4
- Valério, O., Giuliani de, K., da Silva, C. E. S., & Neves, S. M. (2020). ‘Overview on the technology roadmapping (TRM) literature: Gaps and perspectives. Technology Analysis & Strategic Management, 33(1), 58–69. Taylor & Francis. https://doi.org/10.1080/09537325.2020.1787976
- Verma, P., Kumar, V., Daim, T., Sharma, N. K., & Mittal, A. (2022, July). Identifying and prioritizing impediments of industry 4.0 to sustainable digital manufacturing: A mixed method approach. Journal of Cleaner Production, 356, 131639. Elsevier Ltd. https://doi.org/10.1016/j.jclepro.2022.131639
- Williamson, K. (2002). Research methods for students, academics and professionals: Information management and systems. Chandos Publishing Second End. https://doi.org/10.1108/00242530410526664
- Yang, S., Aravind Raghavendra, M. R., Kaminski, J., & Pepin, H. (2018). Opportunities for Industry 4.0 to Support Remanufacturing. Applied Sciences (Switzerland), 8(7), 1177. https://doi.org/10.3390/app8071177
Appendices Annex 1
list of interviewees involved in the application case
Director of the AIT centre
Head of the Organizational Unit (U.O) ‘Industrialization, Innovation, and R&D’
Head of ‘Avionics and Electrical Product Line’
Head of ‘Thermo-mechanical structure and product line’
Head of ‘Platforms Product Line’
Head of Industrial Supply Chain
Head of ‘AIT and Test Operations Management’
Head of the ‘Thermo-Mechanical Systems’ Unit
Head of the ‘Quality Control Inspectors’ Unit
Logistics Manager
Head of ‘Logistics & Clean Rooms’
Head of Technical and Industrialization Office
Head of AIT for a reference product
Quality Inspector
Specialist in the Quality Management System
Specialist in the Quality Assurance AIT/Product Assurance AIT/Industry Maturity Leader
Head of Information System and Product Lifecycle Manager (PLM)
Annex 2
C-E relationship map and Matrices obtained during the C-E quantification workshop