ABSTRACT
The Operator 5.0 concept calls for the self-resilience of operators in Industry 5.0, including the cognitive aspect. Despite attempts to develop supporting technologies, achieved results are loosely connected without a comprehensive approach. Looking for novel expectations, this study seeks inspiration from a chaotic environment, where cognitive resilience is a firm standard: military operations. A systematic literature review in Scopus investigated how technology-enabled cognitive resilience is achieved in this context. Extracted details show vast technology support from field operations to control space, against the single or corporate effect of stressors from the work environment, context, content, or users themselves. These technologies generate indirect and direct influence from physical, mental, and cognitive aspects, creating a cognitive resilience effect. The concept of human-machine symbiosis is proposed, with a framework from technology development to resilience training, to inspire developers to define a broader scope, and engineers to facilitate comprehensive adaptation of Operator 5.0 solutions.
1. Introduction
The Operator 4.0 (O4.0) initiative (Romero et al., Citation2016) promotes the human-centric usage of Industry 4.0 (I4.0) technologies to enhance the capabilities and competencies of human operators (Caldarola et al., Citation2018; Kaasinen et al., Citation2020; Longo et al., Citation2017; Ruppert et al., Citation2018), including cognitive aspect (Madonna et al., Citation2019; Zolotová et al., Citation2020). With the support of technologies in workload analysis, situation awareness, and performance accomplishment advisement (Chacón et al., Citation2020; Mattsson et al., Citation2020; Pacaux-Lemoine et al., Citation2022), the Cognitive O4.0 can interact and communicate with surrounding systems ergonomically, comfortably, and efficiently through diverse technologies, process a large quantity of information and high-velocity data (Fallaha et al., Citation2020; Guerin et al., Citation2019; Thorvald et al., Citation2021).
A technologically enabling work environment that allows a diverse and changing workforce rather than a fixed standard for physical fitness and youth (Romero et al., Citation2020) is advocated in the Industry 5.0 (I5.0) (Breque et al., Citation2021). The Operator 5.0 (O5.0) concept proposed built-in resilience from the human operators and human-machine systems (self-resilience and system-resilience, respectively) (Romero & Stahre, Citation2021). Regarding cognitive resilience as the ability to withstand the effect of stress and rebound from adversity (Staal et al., Citation2008), an ideal technology-supported human cognitive performance is expected within scenarios of abruption, disturbance, unexpected factors, high-risk, safety-critical, and complex operational context (Aljaberi & Abd Rahman, Citation2023; Bakhshandeh & Liyanage, Citation2023; Brocal et al., Citation2019; Grabowski et al., Citation2018; Kaasinen et al., Citation2022; Peng et al., Citation2023; Rankin et al., Citation2014), aiming to maintain mental ability and avoid human-errors (Romero & Stahre, Citation2021). However, despite how exploratory the simulated conditions are, they rather have characteristics of individual innovation and lack a comprehensive vision of resilience and corporate requirements for human-technology symbiosis (Hancock, Citation2017). This fact can be explained as industrial studies aimed at closed and stable environments, repetitive tasks, and predictable workloads, which limit innovative technology use. Therefore, this study seeks inspiration from a more cognitive readiness- and preparedness-demanding field (Fletcher & Wind, Citation2013; Nindl et al., Citation2018): military operation. In such context, human operators are always under a great amount of physiological stress that reduces their functioning at both individual and group levels (Kavanagh, Citation2005).
Resilient cognitive performance is a strict standard during military operations (Bartone, Citation2006; Hodgetts et al., Citation2017; Kelley et al., Citation2011; Martin et al., Citation2020; McKendrick et al., Citation2014; Nindl et al., Citation2018; Staal et al., Citation2008). Many research explored the effect of the stimulus, disturbances, and psychological stressors (hereinafter, these factors are mentioned as ‘stressors’) on military personnel (Fincannon et al., Citation2013; Irgens-Hansen et al., Citation2015; Pomeroy, Citation2013), thus developed supporting technologies and training toward a resilient performance (Flood & Keegan, Citation2022; Goldberg, Citation2012; Reivich et al., Citation2011; M. K. Taylor et al., Citation2008; Zueger et al., Citation2023). With the support of science (e.g. neuroscience, psychology, physiology (Geyer, Citation2011; Stokes et al., Citation2023; Trochowska, Citation2018)) and I4.0 technology (e.g. robotics, information technology, neurotechnology, and artificial intelligence (Billing et al., Citation2021; Feltman et al., Citation2020; Nørgaard & Linden Vørnle, Citation2021)), a ‘human-machine symbiosis’ is envisioned (Malish, Citation2017). This skillful integration of human and machine enhances the human sensing, presenting, processing, and communicating with situational information in extreme environments, demand, and conditions (Merlo, Citation2018), alleviating the mismatch between human limitation and system (DC CSAFW, Citation2010). With the technology support, military personnel are trained to perform complex operations and process vast data in the current information age (Hatfield & Haufler, Citation2018; Martin et al., Citation2020; McFarlane, Citation2006; Reddy, Citation2021), while maintaining an operational level of cognitive readiness (Grier, Citation2012) under negative stimuli or intense stress (Brunyé et al., Citation2020; Pallavicini et al., Citation2016). These efforts improve human resilience as the weakest link within advanced military technology and weaponry systems (Puscas, Citation2018), preventing breakdown, or facilitating quick recovery after breakdown (O’malley, Citation2010).
Since cognitive resilience is a positive adaptation of cognitive functioning despite stressors (Luthar & Cicchetti, Citation2000), understanding stressors and their characteristics is an important step. Relevant review studies (Billing et al., Citation2021; Brunyé et al., Citation2020) only focused on reporting cognitive-enabled technologies without analyzing in-depth the characteristics of stressors and the resilience aspect, thus hindering later technology development. To extend this body of knowledge, and consider how such lessons can be applied to the development of O5.0 solutions, this paper scrutinizes the human-centric technologies that are developed for military operations, what stressors and human factors were considered, and how they improve cognitive resilience of operators/soldiers/human users (henceforward, these subjects will be mentioned as ‘human users’) along with the overall performance. The main research questions of this study are:
What are the characteristics of military operations and deployed technologies to support human users performing assigned tasks?
What are the stressors that were considered, and how the cognitive performance of human-technology symbiosis can be improved?
How these lessons of technology-enabled cognitive resilience can be applied in the industrial environment?
The results from this paper yield two-way contributions in both fields:
Developers in the military field can have an insight from the O4.0 concept with a process improvement approach to assess the efficiency of technology usage. These measures are useful in the evolution of hybrid or remote and electronic warfare (DC CSAFW, Citation2010) when users perform repetitive tasks in a controlled environment similar to industrial operators in a control room. In this scenario, boundaries need to be established to avoid the negative consequences of misused technology.
Inspired by military standards and expectations, industrial developers and engineers can have innovative expectations about cognitive resilience-enabled technologies, and contemplate different initiatives for self- and system-resilience enhancement in the transition toward I5.0 (Gladysz et al., Citation2023), as production tasks can be considered as challenges for personal training and development, aiming at mental toughness of human users.
The paper is structured as follows: Section 2 describes the search strategy and methods, with results and findings from included studies. The discussion and suggestion for human-technology resilience symbiosis are mentioned in Section 3. The conclusion is drawn in Section 4, along with the future research agenda.
2. Evidence of technological-support-based cognitive resilience in military operations
In this section, subsection 2.1 explains the review strategy with selection criteria and the scanning process. Subsection 2.2 describes the characteristics of military operations mentioned in the included studies, with the deployed technologies discussed in subsection 2.3. Subsection 2.4 categorized the characteristics of stressors as the main actors requiring resilience. As not many studies are intrinsically aimed at cognitive resilience, firstly the technology supports for cognitive functioning are described in subsection 2.5, and then the cognitive resilience effect will be scrutinized in subsection 2.6. The influencing factors that affect the efficiency of supporting technologies are mentioned in subsection 2.7, along with associated side effects, with the tested user population are discussed in subsection 2.8.
2.1. Review strategy and selection criteria
As this research focuses on the human-centricity of supporting technologies, the authors want to capture the detailed interaction between human users and technology given certain missions, not only the updates from newly developed functions or technologies. Therefore, technology reports and white-papers are not considered sufficient for the intended purpose, but scientific research or experimentation with the involvement of human subjects is the most expected input. Consequently, the Scopus database is selected, as it is the most commonly used for literature searches (Joshi, Citation2016), and has some advantages compared to Web of Science such as more records (Stahlschmidt & Stephen, Citation2022) with good quality (Powell & Peterson, Citation2017). Besides, Scopus includes in-press papers that are important for emerging and multidisciplinary topics (Visser et al., Citation2021). Aligned with the Preferred Reporting Items for Systematics Reviews and Meta-Analyses (PRISMA) guidelines (Page et al., Citation2021), the search was conducted with the following setup:
Database: Scopus;
Type: articles in scientific journals or conference proceedings;
Language: English;
Publication date: from January 2011 to December 2023;
The constructed search query is illustrated in Figure S1, with detailed descriptions provided in Section S.I of the Supplementary Materials. The milestone of 2011 was chosen as the beginning of the I4.0 era (Dais, Citation2017; Xu et al., Citation2021), and to avoid the inclusion of technologies that may have indirect connections to the O4.0 concept.
The results from the search were handled, and duplicates were removed in the Mendeley software (Reiswig, Citation2010). The study selection was carried out in two stages: Title and Abstract screening and Full-text screening. Each stage was performed independently by the first and the third authors. Occurred discrepancies were discussed among all authors. The eligibility criteria applied for the selection of studies were as follows:
Participants: technology- or machinery-equipped soldiers or military operators, who are enlisted personnel, including military police, disciplinary force, law enforcement officers, first responders (except paramedics) such as police and firefighters, including recruits during training periods and other participants during operation-related technology development and testing phases.
Intervention/exposure: Technology support was deployed when the participants performed individual tasks in military operations. The participants are exposed to stress and unexpected factors from the work environment, work content, and work-context factors that are associated with the tasks. Other long-term and not task-associated stressors such as salary, motivation, and chronic stress are excluded. Simulated tasks that are not put into the work content of military operation (e.g. general language cognition (Fertonani et al., Citation2010), verbal identity (Trumbo et al., Citation2016) or letter recognition (Andrews et al., Citation2011), non-threat visual task (Chi et al., Citation2010)) are excluded.
Comparison: any.
Outcome: The improvement, optimization, or enhancement in cognitive functioning of participants against stress, fatigue, and unexpected factors during the technology usage period.
Setting: Simulated or real-world environment.
Study types: Both observational studies and experimental studies were included. Concerning publication types, original articles, conference papers, and reviews published in English available in full-text were included, while study protocols, abstracts, and letters to the editor were excluded.
The PRISMA-based flowchart of the selection process is illustrated in . A total of 67 studies were selected to be included in this review. In the following section, their characteristics will be mentioned with extracted details to facilitate further discussion.
2.2. General characteristics of included studies
Technological supports were developed for many military operations with various characteristics. Environmental effects, physical and physiological influences are usually considered besides the human factors and the technology itself. As psychological stress is the main actor on cognitive function (McEwen & Sapolsky, Citation1995), the characteristics from included studies are categorized into groups of stressors (Lazarus, Citation2020): the ‘work environment’, ‘work context’ and the ‘work content’ (Thayer et al., Citation2010; Vischer, Citation2007; WHO, Citation2020). Details of the work characteristics from included studies can be found in Table S1, Section S.II of the Supplementary Materials.
In traditional warfare, most military operations are characterized by activities in the open field, with unpredictable and uncontrollable environmental stressors that impair cognitive performance (Martin et al., Citation2019). Extreme performance is usually associated with extreme environmental conditions (e.g. desert, arctic, high altitude, or underwater (Militello et al., Citation2015)). Surrounding conditions such as the availability of troops, civil presentation, etc., heavily contributed to the expectation of user performance, along with intensive requirements for physical activities. Different work environments create different requirements even for similar tasks (e.g. spatial processing during on-foot navigation in a quiet environment (Weisberg et al., Citation2018) vs. in a chaotic scenario of burning house (Weidinger et al., Citation2019)). These operational environments pose additional risks and uncertainties, causing spatial disorientation and loss of environmental cues (Lim et al., Citation2018). Details of these factors as relevant stressors to human users will be discussed in detail in the next section.
In the transition to modern warfare with remote weaponry and advanced military systems-of-systems, many activities merely require cognitive ability and are conducted in enclosed spaces such as in-vehicle, or enclosed space of a control room. Thus, the demand for cognitive-physical balance on human combatants will evolve with a significant reduction of physical performance (Billing et al., Citation2021). The similar requirement of task but performed in different spaces (e.g. on-foot patrol (Bequette et al., Citation2020) vs. in-vehicle patrol (Drnec et al., Citation2018), Unmanned Ground Vehicles (UGV) control in dismounted operation vs. remotely (Chen, Citation2018)) may lead to different perception on the user. The operations in these conditions are associated with the intangible requirement of processing huge amounts of information, while a distance from the battlefield causes cognitive dissonance (Guo et al., Citation2017).
Based on the surrounding environment, the activities that human users need to perform in those operations can be categorized into a broad spectrum illustrated in : start from an open field with a dynamic scene, to a closed environment with static scene and dynamic information flow. Typical tasks within manufacturing systems are also illustrated to emphasize the different scopes between the military and factory domains. Factory operations are conducted in a smaller range, within a stable environment with control conditions, consisting of standardized tasks with a well-designed workload. Due to these natural constraints, developed O4.0 solutions may not cope with cognitive resilience requirements as broadly as in military operations.
Figure 2. The work characteristics and human-centric technologies from included studies. To the left are the field operations, while to the right are the activities performed in a control space. The Operator 4.0 concept is formulated within a smaller and limited context, with the out-station tasks on the left, while the works in control stations are placed to the right.
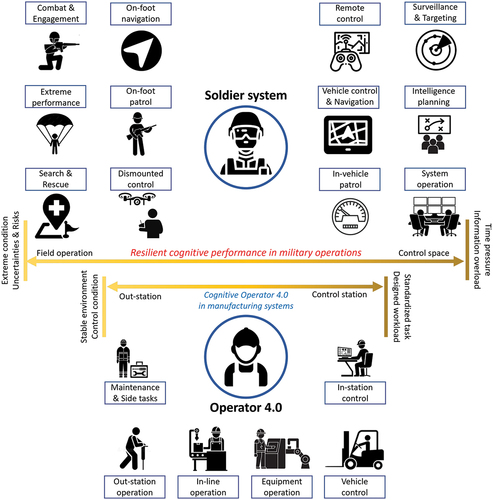
To approach intended operational conditions, some studies tested their technologies in real-life conditions (Chmielewski, Citation2017), while the others were conducted in a safe and controlled digital environment due to its dangerous nature (e.g. fire (J. H. Park et al., Citation2015), high altitude (Hartley et al., Citation2022)). There are extreme scenarios (e.g. casualties (Lipp et al., Citation2021)) that can only be simulated. Participants were exposed to additional factors to simulate the necessary effect (e.g. decreased proportion of oxygen (Rice et al., Citation2019), obstacles on terrain (Bequette et al., Citation2020)). The combination of Virtual Reality (VR), Augmented Reality (AR), and live sessions can bring a more accumulated realistic effect (Ogden et al., Citation2015), building up the tension and stressors.
The work context factors define how the user is supposed to perform the assigned task. The specific task should be considered with the consideration of the work environment and work content. A task can have shared characteristics from many missions. For instance, the navigation task with a guided robot (Elyounnss et al., Citation2016) can be a navigation task but belong to a search and rescue mission, which is done in an extreme environment of an on-fired building. Tasks can be well-described and instructed in standard procedure (Lipp et al., Citation2021), drill scripts (Hardy & Hiller, Citation1985; Hiller et al., Citation1985; D. M. Johnson & Hiller, Citation1985) with given conditions, used machinery and technology (e.g. ARTEP 7–8-DRILL and FM 3–21.8), along with required output and temporal demand (Department of the Army, Citation2009). However, due to natural characteristics of high risk and uncertainties, the use context of technology also varies according to the current situational demand. Only during the technology test or prototyping phase, participants are strictly instructed to act on fixed conditions (i.e. kneeling posture (Berka et al., Citation2010), fixed route (Elliott et al., Citation2010)). In further training or adaptation, assigned tasks need to be performed even with or without supporting technology, or mixed conditions when the technology is malfunctioning (Bequette et al., Citation2020). The same work content, such as using navigation cues from technology, can be addressed differently within different contexts. For example, navigation in unfamiliar terrain with a secondary auditory task, or at night with a secondary visual task (Elliott et al., Citation2010), under temporal pressure with stress (Zhou et al., Citation2023), or in deadly situation (J. H. Park et al., Citation2015) require different supporting functions. As this study focused on individual performance with only support from technology, no social support from comrades or colleagues was included.
The work content includes factors that are specifically and technically related to the exact movements, manipulations should be done within the operation. Predominantly complex task components with improvised focus are required. Repetitive tasks are rarely encountered, only if the technology is specifically designed or tested with a single function (e.g. information processing (Allen et al., Citation2013)) or manipulation (e.g. interactive gesture (Zocco et al., Citation2015)). As time is considered a valuable resource, time pressure always exists in intended use, even incorporated as a critical technical requirement (Weidinger et al., Citation2019), or a performance measure (Brunyé et al., Citation2021).
Besides the cognitive load in completing the tasks and operating the technology, death or life consequences always result in a higher value of cognitive load and enormous stress, than the normal industrial users using the same technology. This load fuels a high availability for decision-making activities and thus requires a significant level of Situational Awareness (SA), which creates a constant stress status.
2.3. Characteristics of deployed human-centric technologies
The assisting technologies can be hardware (Bequette et al., Citation2020; Vatsal & Hoffman, Citation2017) and software (Buchler et al., Citation2014; Özyurt et al., Citation2014), or even a cognitive digital clone model as a decision-maker (Golovianko et al., Citation2023). Software needs to be incorporated into hardware (a system or subsystems of sensors and effectors (Özyurt et al., Citation2014)) to place an effect. Assistant software can be embedded into weapon systems such as aircraft (Godé & Lebraty, Citation2013), after a careful reliability prediction and estimation process (Ryu & Jeong, Citation2018).
Mostly the technologies focused on generating the SA about surrounding environments, the scope of required tasks, and the changes that affect task requirements. These human-centric technologies either read signals from users (e.g. physiological (Brunyé et al., Citation2021; Davila & Hourani, Citation2018), neural signal (R. McKinley et al., Citation2012)) or introduce signals to users through sensory input channels (e.g.: visual (seeing) (Hartley et al., Citation2022; Lipp et al., Citation2021), auditory (hearing) (Hartley et al., Citation2022; Lipp et al., Citation2021), tactile (touch) (Elliott et al., Citation2010; Elyounnss et al., Citation2016), olfactory (smell) (Rice et al., Citation2019)), and one special channel of brain stimulation (Feltman et al., Citation2020; Puscas, Citation2018), thanks to advancements in neuroscience (Berka et al., Citation2010). Its combination with I4.0 technologies such as VR and AR helps to improve the effective use or reduce the negative effect while operating in virtual environments (Brunyé et al., Citation2019; Teo et al., Citation2016). Neurocognitive technology and brain-computer networks can be integrated into human users, merging human and machine cognition into a cyborg (Nørgaard & Linden Vørnle, Citation2021). Noticeably, the ‘gustatory’ (taste) channel was not deployed in any included studies.
A large part of the suggested technologies is developed for operation with specific tasks (Bequette et al., Citation2020; Chen, Citation2018; Elyounnss et al., Citation2016; J. H. Park et al., Citation2015). A smaller part is developed to create the environment for training purposes (From et al., Citation2011; Hartley et al., Citation2022; Lackey et al., Citation2014; Lipp et al., Citation2021; Ogden et al., Citation2015). Different intended usage will have different cognitive loads and characteristics. The training phase has resilience requirements supporting the learning to understand new knowledge, while the operation phase has a much more significant requirement to prepare for sudden engagement and contact. Some technologies facilitate a specific elementary task (e.g. in-vehicle observation (Metcalfe et al., Citation2011), route planning (Brown et al., Citation2020), plate checking while driving (Zahabi & Kaber, Citation2018)). In contrast, the others aim to develop an assistant system supporting complex tasks (Buchler et al., Citation2014; Godé & Lebraty, Citation2013). Some technologies serve for general purposes without specific context (Berka et al., Citation2010; Davila & Hourani, Citation2018; Nørgaard & Linden Vørnle, Citation2021; Paul, Citation2023; Puscas, Citation2018, Citation2018; Vatsal & Hoffman, Citation2017).
The technologies in the included studies are reported during different phases. Some studies are in the concept phase (Allen et al., Citation2013; Nørgaard & Linden Vørnle, Citation2021; Puscas, Citation2018), based on analyzing the user needs (Brandão & Pinho, Citation2017; Paul, Citation2023; Weidinger et al., Citation2019), or aroused requirement during practices or trials (van Beurden & Roijendijk, Citation2019), or suggesting a computational model for promising technology innovation (Brunyé et al., Citation2021). Some studies have their prototypes to test and adapt according to feedback from users (Berka et al., Citation2010; Ogden et al., Citation2015; Seoane et al., Citation2013; Vatsal & Hoffman, Citation2017). Due to the harsh requirement for technological readiness, and perhaps the confidential nature, no studies were reported on technology in the operation phase.
2.4. Stressors in the included studies
Resilience can only be analyzed under the presence of stress (Luthar & Cicchetti, Citation2000). Besides environmental factors, military operations are full of uncertainty, risk, stimulus, and disturbances, which pose additional unexpected loads on human users. They can all be considered stressors (both physical and physiological (Pomeroy, Citation2013)). This subsection is dedicated to describing these stressors and factors, and how these stressors were introduced, integrated, or tested their effect in the included studies. More details can be found in Table S2, Section S.III of the Supplementary Materials.
categorized the stressors based on coming sources: work environment, work context, work content, and even supporting technologies. Noticeably, the stressors can originate from the perception of human users themselves, extracted from interviews with the main performer (Lipp et al., Citation2021) or operational experts (Hartley et al., Citation2022). These stressors can be grouped by their characteristics:
Unusual stressors: that are not usually considered from a stable and repetitive task viewpoint but can be expected beforehand, therefore requiring attention during the mission, but have less impact and surprise than the other factors.
Unexpected stressors: that are extremely unusual and cannot be fully predicted and can cause surprise.
Overburdened stressors: factors that exceed the normal working capability and expectation.
Detrimental stressors: that significantly affect the human factors and can leave them with serious consequences or long-term effects.
From an environmental aspect, conditions such as ‘complex terrain’, and ‘poor lighting’ (Elliott et al., Citation2010; Lim et al., Citation2018) conditions are considered unusual but foreseeable stressors. The ‘terrain obstacles’, ‘crowded traffic’ with ‘road hazard’ (Metcalfe et al., Citation2010) and ‘weather changes’ can be considered unexpected. Overburden stressors such as ‘multi-tasking’ (Elliott et al., Citation2010; Zahabi & Kaber, Citation2018) and technology ‘breakdown’ (Bequette et al., Citation2020) push the task over the normal limit (physically, mentally, temporally or emotionally). Detrimental factors such as ‘on-fire’ buildings (Weidinger et al., Citation2019) and heavy ‘smoke’ (J. H. Park et al., Citation2015) can result in severe injury or death, thus contributing to more demanding requirements. There are stressors that can have both characteristics: unexpected and overburdened, such as ‘appearing object’ (e.g. vehicles and human entities (Metcalfe et al., Citation2010)). Stressors such as ‘on-air’ condition (Hartley et al., Citation2022) can be both overburdened and detrimental. The environmental stressor can inherently pose another stressor from another domain. For example, a high-altitude working environment of parachute operation (Militello et al., Citation2015) or on-fire building of rescue mission (Weidinger et al., Citation2019) induces great time pressure in the work content.
Considering the work context as the general requirement to decide the specific tasks and activities, there are unusual stressors from the viewpoint of the user. These are ‘enclosed space’ within vehicles (Godé & Lebraty, Citation2013; van Weelden et al., Citation2021), ‘complex indoor environment’ (Weisberg et al., Citation2018), and ‘unfamiliar space’ (Weisberg et al., Citation2018). ‘Disrupted coordination’ during a team mission (Militello et al., Citation2015) also causes higher attention to maintain SA. Unexpected stressors in the work context are ‘low-quality data’ (Bishop, Citation2015), ‘passive role’ when following the task (Drnec et al., Citation2018) and leading others, or ‘switching roles’ with another team member (Militello et al., Citation2015).
Within the work content as the required specific activities, some stressors come from the natural characteristics of the task and add up the difficulties. Unusual factors are the ‘technology unfamiliarity’, ‘intangible object’ that requires imagination (Boyce et al., Citation2019; Mao et al., Citation2018), ‘moving target’, and ‘fast tempo’ (Bequette et al., Citation2020). Unexpected factors are the ‘task change’ or the introduction of a ‘new task element’. ‘Irrelevant information’ and ‘additional requirement’ can have both unexpected and overburdened characteristics. ‘Multitasking’ and ‘demanding overload’ are factors that exceed the normal working capacity of humans. The combination of demanding work-content elements, such as long tasks and increased difficulty (Tiwari et al., Citation2009), can also result in another stimulus, such as vigilance decrement. When conducting a combination of main and secondary tasks, the secondary task is an extra stressor (Elliott et al., Citation2010; Zahabi & Kaber, Citation2018). Stress is not only associated with difficult tasks but also the abrupt changes between normal and difficult tasks. In this case, the change is the stressor (Rao et al., Citation2020).
In specific scenarios, technology can pose additional distractions, physical or cognitive workloads on the user (Bequette et al., Citation2020) due to their natural ‘unfamiliarity’, ‘complexity’ or ‘intensive interaction’ as unusual factors. The use of an improved interface for a mobile computer terminal in the secondary task leads to a significant decrease in the main driving task and a higher perceived workload (Zahabi & Kaber, Citation2018). In these cases, the assistant technology itself is the stressor and should be tested when it is not deployed (Vatsal & Hoffman, Citation2017). Unexpected stressors from the technology are the ‘malfunction’, ‘disruption’, ‘passive state’ or ‘system failures’ (Kapalo et al., Citation2019). Additional load from both intentional usage (e.g. saving energy, reducing noise), or unintentional ones (e.g. ‘damaged’, and ‘break down’) should also be tested to avoid future negative consequences (Bequette et al., Citation2020).
From the human user aspect, the stressors can be stemmed from the physical, mental, or physiological state of himself. Unusual factors during task execution are ‘reduced capability’ such as vision (J. H. Park et al., Citation2015; Weidinger et al., Citation2019) and ‘divided attention’ (J. Nelson et al., Citation2016). Unexpected factors are ‘degraded capability’, or a ‘surprise’ state that requires quick response (Brandão & Pinho, Citation2017; Zahabi & Kaber, Citation2018), or the status of ‘information overload’, which can either be the work context stressor in the case of an information processing task (Zocco et al., Citation2015). The ‘sensory overload’ (Metcalfe et al., Citation2010) and ‘mental fatigue’ (Zhou et al., Citation2023) are overburdening stressors. Detrimental stressors such as Traumatic Brain Injury (TBI), concussion, and Post-traumatic Stress Disorder (PTSD) are mentioned (Cukor et al., Citation2015; Edwards et al., Citation2014; Rizzo et al., Citation2009, Citation2011; Scherer et al., Citation2018), but rarely the main study focus, as they lay in the extreme region that will be mentioned in the next subsection of cognitive resilience.
2.5. Cognitive support from technology
There are different I4.0 technologies/functions to assist human cognitive performance, or preventive measures that are integrated with the technology used to prepare for resilience against the above-mentioned stressors. This subsection discusses the technology supports, and most importantly, the resilient effects or interventions that are imposed on human users.
There are direct and indirect approaches to yield effects on human users as a symbiosis with technology, though the distinction between them can be tenuous (Brunyé et al., Citation2020). Included technology support can be categorized into different measures, such as:
Training the user (indirect mental influence) to get used to the abnormal working conditions, and stressors, while practicing stress relaxation and possible solutions.
Enhancing the SA of the user (direct cognitive influence) by supporting synthesizing the surrounding and mission information; and introducing the collected information to the users through different modalities, based on the status of the user to ensure efficient reception.
Relieving the cognitive load of the user (indirect physical influence) by easing the effect of stressors from the work environment or context, supporting physical workload.
Relieving the cognitive load of the user (direct cognitive influence) by adjusting the task that human users need to process, or enhance the human cognitive capability.
The supporting functions and role of technologies are categorized in . The ‘direct cognitive influence’ group is illustrated by the steps of the Observe, Orient, Decide, and Act (OODA) loop, to emphasize the driving role of human users in the command-and-control decision-making process during operation (J. Johnson, Citation2023). The ‘indirect physical influence’ and ‘indirect mental influence’ are indicated as supporting foundations that can take place before, and during the operation. Details of the supporting technologies and functions can be found in Table S1, Section S.II of the Supplementary Materials.
Figure 4. Technical supports from included studies. The supports can yield indirect physical or mental influence, or direct cognitive influence within the observe, orient, decide and act loop.
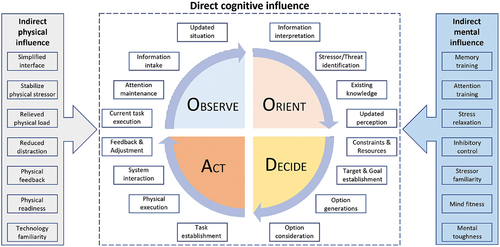
Resilience performance starts from training the human user to become resilient (Hallal-Kirk et al., Citation2019) before the intended operation, from both physical and mental aspects. ‘Indirect mental influence’ includes training for ‘mind fitness’ (Jha et al., Citation2015; Stanley et al., Citation2011), targeted learning (Brunyé et al., Citation2020), and accelerated learning (Berka et al., Citation2010). Other training for cognitive resilience (Blacker et al., Citation2019) fall into this group, such as stress relaxation with biofeedback (Davila & Hourani, Citation2018), recognition capabilities enhancement such as attention, vigilance, and memory training (Daly et al., Citation2017; Keebler et al., Citation2011; Puscas, Citation2018). Feedback with human signals (e.g. neural, physiological (van Weelden et al., Citation2021)) was utilized to facilitate skill acquisition, long-term memory, and skill transfer, creating a resilient mindset and supporting the users to get used to introduced stressors. VR and MR are proven to have significantly superior effects on the training of risky tasks (Rettinger & Rigoll, Citation2022). Both mental and physical training with extreme conditions can be generated by AR headsets in VR environment (Zhou et al., Citation2023), such as emergency parachute operation (Hartley et al., Citation2022), emergency search and rescue (Lackey et al., Citation2014). Specifically designed stimulus and effect are employed to evoke negative emotions and stress (Lipp et al., Citation2021; Ogden et al., Citation2015).
As the ultimate purpose is cognitive performance, many technologies aim at software, algorithm, or Human-Machine Interface (HMI) design, however, hardware can also be counted. Since the physical activity level is an important factor in moderating individual cognitive performance and fatigue (van Beurden & Roijendijk, Citation2019), cognitive performance should not be studied in isolation from the physical aspect. The ‘indirect physical influence’ starts with training for physical readiness with optimal performance (Berka et al., Citation2010), even in case of technology malfunction (Bequette et al., Citation2020). Besides rich multimedia with enhanced realism and vividness from AR and VR, additional physical conditions (such as vibration, noise (Chen, Citation2018; Metcalfe et al., Citation2010), reduced oxygen (Rice et al., Citation2019)) were added to make the testing VR environment more realistic. Advanced user models and input technologies (Emond et al., Citation2010) were utilized to produce effective training outcomes. The physical influence can be deployed through the simplified human-machine interface that optimizes human interaction (Fay et al., Citation2019; Zahabi & Kaber, Citation2018), architecture that supports interactive with intangible information (Abich et al., Citation2018), mechanical structure that relieves the physical burden and movements (Bequette et al., Citation2020; Vatsal & Hoffman, Citation2017), or the protection equipment that stabilizes the work environment (O’Hara et al., Citation2016).
Inspired by the expansion of the Orientation phase in the information warfare (Brumley et al., Citation2006), this study creates elemental steps for every OODA phase to analyze in-depth how the proposed technologies support cognitive performance. The ‘Observe’ phase makes use of technologies that support the human user to keep track of their ‘current task execution’, by giving the status of ongoing tasks and current weapon status (Brandão & Pinho, Citation2017; Chmielewski, Citation2017) with dynamic visualization (Mao et al., Citation2018). Multiple tasks or tasks with divided attention can be monitored during ‘attention maintenance’, with the support from a well-designed interface (J. Nelson et al., Citation2016; J. M. Nelson et al., Citation2019), surveillance system (Metcalfe et al., Citation2011). The collected data and information can be filtered based on the current context (Allen et al., Citation2013; Kim, Citation2016). Utilizing the Multiple Resource Theory (Wickens, Citation2002), the incoming information can be presented to the human user by distribution through multi-sensory channels. This approach can yield the most effective ‘information intake’ (Elliott et al., Citation2010; Kim, Citation2016; Weisberg et al., Citation2018) with a reduced overall cognitive load. High-level data can be presented with a spontaneous insight (Lavigne & Gouin, Citation2014) or deliberated method (Kim, Citation2016) to reduce further processing. The SA of the user will be refreshed with an ‘updated situation’ on time with a simplified presentation (Godé & Lebraty, Citation2013) that supports the Military Decision Making Process (MDMP) and Rapid Decision-making and Synchronization Process (RDSP).
The ‘Orient’ phase starts with ‘information interpretation’ in which support systems help to analyze the acquired data from mixed sources to find useful information that affects the next tasks (Allen et al., Citation2013; Cummings et al., Citation2013). The cognitive load that the human users need to process (e.g. tactical calculation, threat evaluation (Chmielewski et al., Citation2019)) can be reduced thanks to the ‘stressor/threat identification’ that supports the recognition ability (Buchler et al., Citation2014; Daly et al., Citation2017; J. H. Park et al., Citation2015). By linking this information with the ‘existing knowledge’ of the mission (Godé & Lebraty, Citation2013; Militello et al., Citation2015), an ‘updated perception’ will be ready for creating the possible decisions in the next phase. Noticeably, the user status should also be updated as a part of the system, with a physiological computing system deployed to perform a real-time assessment based on physiological data (Stokes et al., Citation2023) and cognitive sensing such as pupilometer, gaze measurement (Zhou et al., Citation2023).
The ‘Decide’ phase considers the ‘constraints and resources’ of the current context, vehicle or equipment (Fay et al., Citation2019; Militello et al., Citation2015) and then makes the ‘target and goal establishment’ such as destinations (Weidinger et al., Citation2019), target (Alicia et al., Citation2018). ‘Option generation’ such as trajectory (Hew, Citation2017), asset distribution, and route plan (Buchler et al., Citation2014) can be made with the distributed cognition between human and machine, and visualized for easy perception (Abich et al., Citation2018; Godé & Lebraty, Citation2013; Özyurt et al., Citation2014). Different criteria and information (From et al., Citation2011; Lim et al., Citation2018) can be offered timely to support the ‘option consideration’ process. Enhancing human capability with improved brain functions can directly result in resilient performance (Stikic et al., Citation2015). Neurotechnology can be used as supporting tools for information processing or used as neuro weapons, to provide its user with ‘decision superiority’ in multi-domain warfare (Nørgaard & Linden Vørnle, Citation2021).
During the ‘Act’ phase, ‘task establishment’ of specific tasks can be made between humans and supported software (Buchler et al., Citation2014; Lafond et al., Citation2010). The ‘physical execution’ is conducted with the support equipment with ‘indirect physical influence’ such as exoskeleton (Bequette et al., Citation2020), wearable hand (Vatsal & Hoffman, Citation2017). The ‘system interaction’ can be made through a multi-modal interactive robot for communication (Billing et al., Citation2021), cognitive ergonomically-designed interface and console (Metcalfe et al., Citation2011; Redden et al., Citation2011) with natural interaction (Zocco et al., Citation2015). The system can offer to take control of the shared tasks when needed (Drnec et al., Citation2018). Finally, the ‘feedback and adjustment’ function takes input from the real world (Elyounnss et al., Citation2016; White et al., Citation2018) and helps users to continue the ‘Observe’ phase of the next iterative loop.
These above-mentioned supporting functions from technologies can be used together to create a collective effect. Under the presence of stressors, how they contribute to the cognitive resilience of the human user is discussed in the next subsection.
2.6. Cognitive resilience effect
Since not all of the included studies originally aimed at cognitive resilience, the authors took a closer look to recognize its potential in each scenario. To analyze the resilient performance, the technology support is put into the context of stressors presence as exhibited in . Inspired by the early work on General Adaptation Syndrome (GAS) (Selye, Citation1936), and considering cognitive resilience as positive adaptation under significant adversity (Luthar & Cicchetti, Citation2000), the technology support can be applied in different operational phases: ‘training’, ‘normal operation without stress’, and stress ‘alarm’, ‘resistance’, ‘adaptation’, and ‘recovery’. Throughout these phases, the cognitive load on human users is expected to be within the regions of the ‘perceived stress threshold’ and the ‘stress tolerance threshold’. With the presence of stress, the cognitive performance may show affected behavior such as narrowing of focus (Militello et al., Citation2015), and a significant degradation when it exceeds the personal ‘stress tolerance threshold’ (Endsley, Citation1993). The stress exhaustion phase, and the functioning above the ‘stress tolerance threshold’ are not considered for two reasons: there is no technology developed for these conditions, and a counterproductive decision in these regions easily results in death, which is not the desired goal of cognitive resilience (Puscas, Citation2018).
Task analysis (Hartley et al., Citation2022) and naturalistic decision-making techniques (Militello et al., Citation2015) are deployed to understand the stress effect. Though cognitive load is not frequently measured directly, different cognitive performance indicators were presented to indirectly examine its value, associated with a wide range of cognitive tasks. Some studies used subjective physiological parameters (e.g. eye tracking, electroencephalography (EEG) (Metcalfe et al., Citation2010), Heart Rate Variability (HRV) (Davila & Hourani, Citation2018), pupillary size and gaze data (Alicia et al., Citation2018; Zhou et al., Citation2023), respiration rate and Galvanic Skin Response (GSR) (Seoane et al., Citation2013)) to predict the overload status of the user. Subjective parameters such as emotional (Lipp et al., Citation2021) or verbal response (Elliott et al., Citation2010), perceived workload (i.e. NASA Task Load Index (NASA-TLX) (Bequette et al., Citation2020; Boyce et al., Citation2022; Rao et al., Citation2020)), behavioral measures (e.g. reaction time to a stressor (Bequette et al., Citation2020; Metcalfe et al., Citation2010; Zahabi & Kaber, Citation2018), the completion time of a task (Brandão & Pinho, Citation2017; Weidinger et al., Citation2019; Zahabi & Kaber, Citation2018; Zocco et al., Citation2015), interaction comfort (Zocco et al., Citation2015)), or performance result on a specific task (i.e. number of killed enemy and accuracy index (Rao et al., Citation2020), detection accuracy (Berka et al., Citation2010; Metcalfe et al., Citation2010), the possible processed amount of information (J. Nelson et al., Citation2016; J. M. Nelson et al., Citation2019), safe driving (Zahabi & Kaber, Citation2018), flight control (Rice et al., Citation2019), focusing attention (Mao et al., Citation2018), accuracy of acquired knowledge or SA (Boyce et al., Citation2022; Metcalfe et al., Citation2010; Zhou et al., Citation2023; Zocco et al., Citation2015)) or on a standardized cognitive test (e.g. Stroop test (T. D. Parsons et al., Citation2011), Visual Sequence Comparison (Rice et al., Citation2019)). These indicators can be measured based on the performance in the main task (Zocco et al., Citation2015) or in the secondary task (Elliott et al., Citation2010; Zahabi & Kaber, Citation2018) in case of multitasking. The ultimate goal of the experiment in the included studies is the improvement of these indicators under the presence of stressors, without any mental mistake (e.g. wrong threat detection, friendly fire, civilian casualties, etc.).
During ‘training’, many measures can be performed to increase the ‘perceived stress threshold’, such as relaxation training (Davila & Hourani, Citation2018), stressor familiarity (Daly et al., Citation2017), with physical endurance and malfunction scenario practice (Bequette et al., Citation2020). On the other hand, extreme exposure and mental toughness training aim to increase the ‘stress tolerance threshold’. During ‘normal operation without stress’, built-in technology functions such as permanent and convenient information access (Chmielewski, Citation2017; Weidinger et al., Citation2019), specialized interface (Fay et al., Citation2019; Lim et al., Citation2018), natural human-system interaction (Zocco et al., Citation2015) also help to expand this threshold. Technical functions that deliver pre-processed information (Kim, Citation2016) through multi-channel (Elliott et al., Citation2010; Elyounnss et al., Citation2016) reduce the amount of cognitive requirement demanded on the user.
The appearance of stressors can take the form of a momentary stressful event (e.g. appearing objects (Bartlett et al., Citation2013), casualty witnessing (Ogden et al., Citation2015), technology malfunction (Militello et al., Citation2015)) or a long period of disturbance (e.g. night condition with heavy fog (Elliott et al., Citation2010), noisy sound (White et al., Citation2018)), or the combination of both types (e.g. the appearance of obstacles in no light conditions of heavy smoke (J. H. Park et al., Citation2015)). Once the stressor appears, early detection of stressors or threats (J. H. Park et al., Citation2015), or the acute physiological response from the user (Brunyé et al., Citation2021; Seoane et al., Citation2013) can be helpful for the later stress-coping steps. Options to reduce distraction from secondary task (Zahabi & Kaber, Citation2018), calm down, or stabilize the situation (Davila & Hourani, Citation2018) help to shorten the ‘alarm’ period. During the ‘resistance’ phase, timely and cognition-driven efforts such as reduced calculation amount with relevant information and instruction (Zhou et al., Citation2023), distributed cognition (Hew, Citation2017) will cut down the reaction time. If the cognitive load is still increasing, aided functioning (e.g. voice recognition (Kim, Citation2016), driving (Drnec et al., Citation2018), target recognition (Chen, Citation2018), decision making (Buchler et al., Citation2014; Godé & Lebraty, Citation2013)) can be activated. Automated operation can be deployed if the user is under a critical amount of cognitive load (Drnec et al., Citation2018).
Once the cognitive load is mitigated during the ‘adaptation’ phase, adaptive control, auto-adaptation (Zhou et al., Citation2023), or cooperation (Elyounnss et al., Citation2016) between humans and the supporting system can take place, to gradually stabilize the cognitive load under the stressor presence. After this phase, the ‘recovery’ phase will set up a new cycle with a stable cognitive load, with new stressors appearing. Noticeably, during operation or after exposure to stressors (e.g. navigation at night with secondary visual task (Elliott et al., Citation2010)), the ‘perceived stress threshold’ and the ‘stress tolerance threshold’ can be degraded to a lower value.
However, the supporting and enhancing effects of technology are imposed on users differently, as individual differences and backgrounds play a significant role in predicting the expected efficiency. These effects are discussed in the next subsection.
2.7. Influencing factors - side effect
Since technology support yields different influences on individuals in each specific context, it is necessary to investigate the factors affecting effective usage and possible side effects.
Many influencing factors include individual differences such as brain morphology, knowledge, skills, and abilities (Brunyé et al., Citation2014, Citation2019). The post-training distress during training is reduced in individuals lacking prior experience (Lackey et al., Citation2014). The military training background offers an advantage with necessary skills, such as overview interaction due to spatial training (Gardony et al., Citation2021), and military-related experience affects the perceived stress. By training, the performance of military members can yield better accurate performance than civilians during higher workload conditions in some circumstances (Harden et al., Citation2014). The task-related experience plays a great role (Lackey et al., Citation2014), as the users are familiar with the expected operating conditions. Natural skills of users show heterogeneous effects, as sometimes they affect or do not affect the technology-support improvement (Weisberg et al., Citation2018). An appropriate level of trust from the users in the supporting functions plays a significant role in the overall performance (Drnec et al., Citation2018).
The influencing factors can come from the technology itself. Lack of prior training or familiarity with the technology or mechanical part can cost the performance (Bequette et al., Citation2020), as the users need to pay more attention to adapt to and adjust the technology during operation. If the technology is not fully developed, with un-ergonomic design, low dexterity, and lack of autonomy and human-close-loop feedback, then it causes more physical workload and divided attention for the user (Vatsal & Hoffman, Citation2017).
Side effects are mainly negative, which can behave as additional stressors that further degrade human-technology performance. However, they are not initially considered in the study, therefore their effects have no countermeasure during usage. Side effects stemmed from characteristics of a user group (Brunyé et al., Citation2019), or an intrinsic effect from the supporting function (Mao et al., Citation2018; Zocco et al., Citation2015). Operating in a VR environment induced higher mental demand than in live conditions (Lackey et al., Citation2014), as VR technology requires intensive interaction and involvement. If the technology is too intrusive, the user can be overwhelmed with the received information, which leads to a reversed effect (Boyce et al., Citation2022). Lack of knowledge about intended users can lead to deterioration of their original ability (Brunyé et al., Citation2014). In some studies, the enhancement of one skill set comes at the cost of another (R. A. McKinley et al., Citation2012). During multi-tasking with the supporting technology for the secondary task, the SA and performance of the main task are still affected, such as visual attention and environment awareness (Zahabi & Kaber, Citation2018). If the users were well-trained under more realistic conditions (Zhou et al., Citation2023), or the stressor was too weak (Bequette et al., Citation2020), then no effect was observed. The frequency and severity of side effects (i.e. sickness, dizziness, and lightheadedness) may hinder military applications (Feltman et al., Citation2020).
Regarding these influencing factors and side effects, some studies gave recommendations to prevent, limit, or reduce their effects, while others only discovered and reported them without any suggestion. More details can be found in Table S3, Section S.IV of the Supplementary Materials.
2.8. Study population
The study populations in the included studies are heterogeneous. Some studies tested their technology on end-users as soldiers and militants (Abich et al., Citation2018; Bequette et al., Citation2020; Cummings et al., Citation2013; Elliott et al., Citation2010), firefighters (Lipp et al., Citation2021; Zhou et al., Citation2023), army operators (Alicia et al., Citation2018), police and military officers (Mao et al., Citation2018; Zahabi & Kaber, Citation2018; Zocco et al., Citation2015), navy experts (Özyurt et al., Citation2014), or military students (Boyce et al., Citation2022; Cummings et al., Citation2013; Rice et al., Citation2019). The tested populations with military backgrounds (i.e. prior training and experience) are well aware of their operating condition, the associated risks, and the unexpectedness of the tasks (Zahabi & Kaber, Citation2018). Due to their prior possession of physical readiness and mental toughness, the outcome of the experiment and the generalizability of later technology deployment might be affected. Their served experience varied significantly (e.g. from one to 19 years of service (Lipp et al., Citation2021)), indicating different levels of effect are included.
On the contrary, some studies performed experiments on civilians (Boyce et al., Citation2019; Drnec et al., Citation2018; Kim, Citation2016; J. H. Park et al., Citation2015; Rao et al., Citation2020; Weidinger et al., Citation2019; Weisberg et al., Citation2018), who are in lack of prepared capacity and knowledge for military task and context. Therefore, their overall performance is far lower than military expectations and does not reflect the true technology efficiency during operation. However, testing on this population can reveal other resilience factors that are more obvious to a layman such as information overload (Boyce et al., Citation2019). This discovery can be beneficial for further technical development and adaptation before introducing them to military users. Other studies are tested on mixed populations between soldiers and students (Brown et al., Citation2020; Gardony et al., Citation2021), therefore the collected results reflect a broader viewpoint. The variety can avoid the effect of factors such as age, technology competency, etc.
Another factor that harms the generalization is the varied tested populations with a significant unbalance in age, and gender (e.g. all male (Bequette et al., Citation2020; Lackey et al., Citation2014; Mao et al., Citation2018; Zhou et al., Citation2023), 59 male and one female (Lipp et al., Citation2021)). Only a few studies have an adequate balanced number of female participants (Rice et al., Citation2019)). More details can be found in Table S1, Section S.II of the Supplementary Materials.
3. Discussions
This section discusses the current drawbacks of reported technologies (subsection 3.1) and suggests the concept of a human-technology resilient symbiosis system (subsection 3.2), how this resilience preparation can be applied from a comprehensive perspective (subsection 3.3). The promising lessons for industrial application are proposed in subsection 3.4.
3.1. Current drawbacks of reported technologies in included studies
This subsection discusses the shortcomings of the included studies and their reported technologies. Firstly, the heterogeneity of intended usage indicates missing support in some fields, only a few technologies are built for practical operation with descriptive details of work content and work context. Universal tools can be intended for operation (Bequette et al., Citation2020; Vatsal & Hoffman, Citation2017), but more description of possible tasks are necessary. Other technologies such as real-time memory aids, implantable neural interfaces, and brain computers show promising cognitive support (Puscas, Citation2018; P. Taylor et al., Citation2015), but need to be tested within a specific context. Within an operation, many technologies are built for navigation missions, but not many are developed for combat. This fact can be due to the classified nature of military technology, but can also be due to the fast temporal and flexible characteristic, which makes it harder to define the needs of users. On the contrary, developed technologies for specific tasks may lack generalization to apply in a normal context or a complex cognitive process (Brunyé et al., Citation2020).
Secondly, not all technology has been deployed or tested during real operations. Though some tasks are supposed to be carried out in extreme environments such as on-air (Hartley et al., Citation2022; Militello et al., Citation2015), or on-fire building (J. H. Park et al., Citation2015), the supporting technologies are tested in an enclosed environment without realistic physical effects (e.g. heat, free-falling). The meaningful transfer of knowledge and skills from training to operation is hindered by the limited amount of realism with live weapon and combat (Blacker et al., Citation2019; Brunyé et al., Citation2020), reduced amount of physical workload (Lackey et al., Citation2014). Some technologies intended for training (i.e. marksmanship (Berka et al., Citation2010)) also should be tested within different training environments and contexts. As both work content and context during training play a vital effect on the trained outcome (Blacker et al., Citation2019), the difference between the testing and the intended environments raises ambiguity in realistic deployment, insufficient assessment, or even life/death difference during real situations (Bequette et al., Citation2020). Trained tasks in game-based and virtual environments have doubtful skill transfer into real-life activity (Zahabi et al., Citation2022). Similarly, technologies tested with virtual, augmented, and simulated environments may not yield the expected results in operation.
Thirdly, most of the proposed technologies are still in the immature phase with unclear effects and low stability. Inconsistent, reverse or negative effects posed more additional cognitive load on the users (Bequette et al., Citation2020; Boyce et al., Citation2016; Vatsal & Hoffman, Citation2017). There is an ambiguous threshold for supporting technology. Participants who experienced a higher supporting technology level sometimes showed worse accuracy and response time (Boyce et al., Citation2022). Users declared more perceived cognitive benefits but still yielded reduced effectiveness (Lafond et al., Citation2010). The effect of technology on certain task types (e.g. transcranial Direct Current Stimulation (tDCS) in multitasking performance) comes in a delayed manner (Hsu et al., Citation2015), or technical trade-offs are not fully discovered in both the short- and long-term, in operation and daily life (i.e. brain stimulation (Brunyé et al., Citation2020)). The effect can be complicated in the case of multi-tasking, as the technology support for the secondary task leads to a significant decrease in the main task and higher perceived workload (Zahabi & Kaber, Citation2018). Therefore, developers should determine how to improve technology based on the baseline condition of using normal and enhanced technology (e.g. reduced interaction during tasks and off-road glances (Zahabi & Kaber, Citation2018)). Lack of human factors in the control and feedback loop also leads to uncomfortable and awkward movements (Vatsal & Hoffman, Citation2017). If the technology is early in the development cycle and is new to the users, then the users may have low trust and convenience for using them, and opt to perform the assigned task manually (Brewer et al., Citation2022). Against these hard-to-predict behaviors and low stability, there should be a possibility to suggest and switch between which scenario the technology or the user will be the decision maker, and which condition the user should rely on technology (Drnec et al., Citation2018). This is the concept of cognitive and cooperative automation of an assistant system (Schulte & Meitinger, Citation2016), that builds mutual trust from the human side.
Fourthly, individual differences including natural cognitive flexibility (Rademacher et al., Citation2023) show a heavy impact on technology-enabled efficiency. Naturally resilient individuals can cope with extreme situations and environments more easily (Paulus et al., Citation2018). Therefore, the natural cognitive, physical, and cognitive-motor capability of the user should be determined before applying technology, in both non-working and specific working contexts (Games et al., Citation2015). Background diseases such as mild TBI (mTBI), and post-traumatic growth (Linder et al., Citation2019) affect cognitive performance and reduce technology sensitivity. Knowing the user profile (e.g. skills, experience, sleep, and health condition, etc. (Brunyé et al., Citation2021) facilitates individualized enhancement approaches (Brunyé et al., Citation2020). Examples of this individualization are preferred attaching body part (Bequette et al., Citation2020), targeted brain regions, electrode positioning and sizing, stimulation intensity, timing, and duration of Transcranial Direct Current Stimulation (tDCS) (Brunyé et al., Citation2019)) and customizable predictive models (e.g. pupil-lamen formula (Zhou et al., Citation2023)). Consequently, the usability of new technology should be tested with individual variability and response carefully examined, especially with the technology that serves individual practice (Bequette et al., Citation2020).
The assessment of the resilience effect is ambiguous. For active mission or field applications, objective parameters become difficult to assess (Seoane et al., Citation2013; van Beurden & Roijendijk, Citation2019), or both the subjective and objective workload measures can become inconsistent. Even considered by the users as a helpful instrument, technology did not generate any difference in perceived stress, generated SA, and task performance when used and not used (Özyurt et al., Citation2014). Though the users perceived the same workload, their performance still deteriorated due to the fatigue effect (Bequette et al., Citation2020), or when reaching the cognitive threshold (Endsley, Citation1993). During prototype testing and adaptation, the enhanced resilient performance can show no effect, with one possible reason is the introduced cognitive stimuli were too easy. Therefore the expected effect to test the technology was not generated (Bequette et al., Citation2020). Besides the ambiguous technology effect, not a clear perspective of resilience was mentioned. Agility in task performance is not quantified and measured (Bequette et al., Citation2020), and there is an ambiguous distinction between psychological flexibility (Doorley et al., Citation2020) and cognitive resilience, though both concepts aim at better goal achievement. To avoid misconception, a more detailed resilience model analyzing more specific low- and high-order cognitive processes under the presence of stressors should be elaborated. In such a model, psychological flexibility can be an input factor or model component from the user (S. Parsons et al., Citation2016). The resilience model should be considered in more extreme cases, such as how to support the human user to get back to normal functioning from over the tolerance threshold, or functioning not only in the presence of physiological stressors but also physical pain (Yao & Hsieh, Citation2019). Besides, developers, engineers, and researchers should clarify more criteria such as what are the expected signs of cognitive resilience, what should be the duration and amplitude of the technical-supported effect, how flexible and measurable the resilience is, etc.
Another question is raised in the validity of included studies. In different phases of training, different applying times and schedules may affect the enhancement (Parasuraman & McKinley, Citation2014). Most of the development is tested with single-time use, which does not grant effect on continuous or long-term use (Feltman et al., Citation2020). The tested populations do not support the generalization of study results. The cognitive effect has diverse directions and amplitude, depending on the individual user (Bequette et al., Citation2020), or gender (Rice et al., Citation2019). This leads to an incomparable effect of supporting technology within user groups. For instance, young people with better technology proficiency may have more advantages when getting used to new equipment. In general, there is a lack of evidence to claim that aided resilience comes from cognitive enhancement or optimization (Brunyé et al., Citation2020), or from the natural capability of the user. Consequently, the mass application on a larger population is limited.
Though some supporting technologies can make decisions with their own rationale and interfere when they consider it necessary, unfortunately, insignificant attention was paid to the moral dimension. As military operations usually require hard moral choices (Jensen, Citation2013), the ethical aspect of increased capacity (Caron, Citation2018) and enhanced cognitive ability are rising concerns (Bostrom & Sandberg, Citation2009). The moral dimension should be considered even from an early phase in technology development, training, adaptation, and deployment. It is even more important in the case of networked, remote, or virtual operation (Burken & van Bezooijen, Citation2015; Coleman, Citation2013; Singer, Citation2016; Strawser, Citation2013). Ethical rules should be governed by human-machine teaming to ensure the control of human users and their responsibilities during operation (Nørgaard & Linden Vørnle, Citation2021). This requirement is extremely important in remote weaponry control with lethal decision-making, where the lack of realistic feeling about the battlefield creates cognitive dissonance (Guo et al., Citation2017). Too much available information from ubiquitous technology may also potentially blur normative structures (Boshuijzen van Burken, Citation2019). In certain circumstances, this moral dimension also determines what kind of technology should be used (Strawser, Citation2010). Even if not supported by technology, technology can intensify the moral aspect by informing the users of consequences with increased magnitude, and keeping them aware of their perceived distance (Burken & van Bezooijen, Citation2015). A controversial question is whether the moral dimension or even from a legal viewpoint should be applied to the enhanced individual or the enhancement itself (Puscas, Citation2018). Suggestions for an ethical Artificial Intelligence (AI) integrated into a remote air defense system are proposed by Koch (Koch, Citation2022). According to the suggestions of authors, AI only supports its users with context information, enhanced data, and relevant details to facilitate the decision-making process. On the other hand, the human user needs to understand the factors that affect the classification of AI, check moral compliance, and decide the final action.
As supporting technologies may exist in a close connection with human users for a long duration, there are psycho-social concerns that raise several controversial questions. To ensure effective and efficient interaction with human users, there are behavioral features that technology artifacts should have to achieve their goals (e.g. autonomy, adaptation, and interaction), which can be considered agency properties and agenthood (Garcia et al., Citation2002). On the other hand, appropriate design and human-technology interface play an important role, considering the technology is an integrated entity with the human symbiosis. Not only a well-designed interface with simplified interactions can reduce the stress of its users (Fay et al., Citation2019; Zahabi & Kaber, Citation2018), but its outlook changes from the hardware of supporting technologies should be considered from societal norms. The development of invasive technology such as brain-computer interfaces may distort the concept of personhood on the users (O’Brien, Citation2011; Sample et al., Citation2019), which possibly raises rejections within the society, similar to the case of cochlear implants (Sparrow, Citation2005). Since these aspects of human-machine interface design were not mentioned within the included studies, we call for more serious considerations in future technology development, to avoid negative psycho-social impacts.
Last but not least, as more and more technologies are integrated into human symbiosis, their physical form, and technological reliability should be considered for field operation (Militello et al., Citation2015). There are concerns about the energy source to power up modern equipment, which increases the physical carrying load on dismounted and infantry soldiers. Renewable energy with flexible wearable photovoltaic technology is a promising solution (Paraskevopoulos & Tsekleves, Citation2014a, Citation2014b). With an increased ratio of software, cybersecurity should be integrated similarly to a weapon system (Chaplain, Citation2018). However, this paper will not dive into this aspect.
3.2. Concept of a human-technology resilient symbiosis system
Though the included studies aim at establishing resilient cognitive performance, however, the usage is either too focused on an elementary task or too general without the applicable work environment and context. Though cognitive enhancement is mentioned, the resilience aspect is not well-defined. In this subsection, a comprehensive picture of cognitive resilience is suggested in . A human-technology symbiosis is discussed in the following paragraphs.
Figure 6. The resilient symbiosis system from human and supporting technologies. The human user is the center of this resilient symbiosis and receives updated information and support from five main constituent sources or topics, namely the “Operator 4.0”, the “work environment and context”, the “work content”, the “user capability” and the “technology capability”.
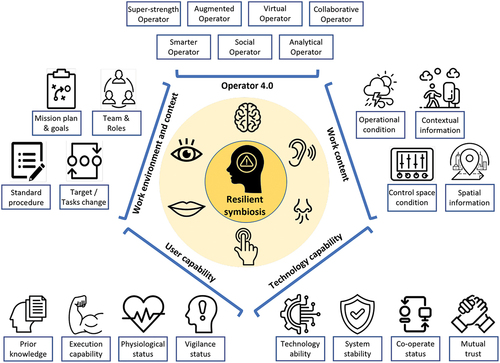
The human-centric technologies from the O4.0 concept play an important role in supporting this symbiosis, to benefit human users with technology enhancement. However, this resilient symbiosis should be aware of the work content factors, from the overall intelligence such as ‘mission goals and plan’, to current ‘team and roles’ cooperation which define the assigned performance and tasks. This function also supports the performance in case of multi-users or in team missions with socially interactive (van Weelden et al., Citation2021), preparing for possible role switch during operation (Militello et al., Citation2015). The ‘standard procedure’ represents the normal expectation of how the symbiosis conducts the tasks. The possible ‘target and tasks change’ reflects the updated context that heavily affects the next tasks.
Once the work content is defined based on the above-mentioned factors, this symbiosis should get updated about the ‘operational condition’ and ‘contextual information’, which reflects the possible terrain, weather, obstacles, and other foreseeable objects in the mission. Because in-vehicle and remote control are becoming popular in modern warfare, this symbiosis should also be aware of the ‘control space condition’ which is the surrounding space. The actual space where the mission is carried out is updated as ‘spatial information’.
As cognitive and physical aspects of an individual have a tight connection (e.g. an increased physical load leads to a decrement in cognitive performance (Knapik et al., Citation2004)), the current cognitive state, physical conditions, and capability of the users should be collected as the operational context (Allen et al., Citation2013), along with changes in work environment, context, and content factors. The ‘prior knowledge’ includes background knowledge and experience of the users such as training on relevant tasks, technology familiarity, etc. This information helps to identify their point of need during later usage. The recorded natural capability on a trained task also serves as the baseline performance. The ‘execution capability’ and ‘physiological status’ reflect the current execution readiness. Adjusting the physical condition or enhancing physical fitness in the long term can help to reduce the impact of acute stress (M. K. Taylor et al., Citation2008). As a result, the recommendation from a suggesting system can help the symbiosis adjust its condition. In harsh environments, technology that can improve working conditions (O’Hara et al., Citation2016), or support cognitive performance against environmental changes. The ‘vigilance status’ is an important indicator of SA, and serves as an attention management tool for the recovery of human users when their task is interrupted (Hairston et al., Citation2012). With the support of multi-modal body sensor networks including wearable EEG sensors, advanced machine learning algorithms can be used for real-time state recognition, cognitive and physical performance prediction, and mitigation of performance degradation during adaptation or operation (Saikia, Citation2023). A cognitive state recognition can utilize the concept of Human Asset Administration Shell from the industrial field (Eesee et al., Citation2024).
As technology malfunction can become a stressor, the symbiosis should be aware of its current ‘technology capability’. The technically designed functions can be categorized as ‘technology ability’, including both physical information (e.g. fuel level) and functional information (e.g. possible movement range) of the system (Fay et al., Citation2019). The technology capability should be adjusted with tuned parameters according to human physical performance (Bequette et al., Citation2020). The more complex the physical task, the more requirements for the tuned parameters. The ‘system stability’ reflects the current functioning of technology in symbiosis. During operation, as inspired by the work of (Allen et al., Citation2013), the technology should be aware of the operational context with leverage points for decision support (Militello et al., Citation2015) similar to a task analysis approach, and the condition of the war-fighter himself. Then the automation level (Özyurt et al., Citation2014) and UI (Cummings et al., Citation2013) can be updated, presenting the collected information according to the user requirement (Daly et al., Citation2017). ‘Co-operate status’ is an indicator in case humans and technology make a common decision or shared control (Drnec et al., Citation2018; Elyounnss et al., Citation2016; Nørgaard & Linden Vørnle, Citation2021), with adaptive triggering based on cognitive and physical states from human user (Brunyé et al., Citation2020; Zhou et al., Citation2023). Based on the recognized workload and performance of the user, the objective mission effectiveness and adaptation mechanisms can be designed and triggered to arrange the workload between human and system (S. Park et al., Citation2018), re-planning the tasks (Lim et al., Citation2018). Such an intervention or support is trivial during critical events that are important to the mission goals (Wilson & Russell, Citation2007), or when the human or system performance is possibly degrading the most (Inagaki, Citation2000). Psychological experiments suggested that technology should support the users to manage their cognitive resources and capacity efficiently, such as workload transition to improve multitasking performance (Devlin et al., Citation2020). Therefore, cooperating does not only mean operating the technology but also operating the physical activities from the human user side.
The ‘mutual trust’ reflects the agreement rate of users on suggestions from technology. These features help to establish trust that encourages the user to offload their cognitive load (Dorneich et al., Citation2004). A similar indicator, the Technology Acceptance Measure (TAM) was utilized in some studies (Abich et al., Citation2018; Keebler et al., Citation2011), indicating how well a system is accepted by its user (Lee et al., Citation2003). Explainable AI (XAI) principles can be deployed to support this interaction (Core et al., Citation2006). This approach helps to reduce the lack of transparency in AI-based processes and semantics, and build trust in military personnel (Nørgaard & Linden Vørnle, Citation2021). During the operation, the trust value can be changed due to the current context, therefore, a trust calibration tool that directs the flexible automation is necessary (Hairston et al., Citation2012), especially in case the human and machine co-operate (Drnec et al., Citation2018). Otherwise, if the users place a high level of trust in technology-operated outputs, cognitive biases (i.e. representativeness, availability, and anchoring) can occur (Krebs, Citation2023).
The more information provided causes more risk of cognitive overload (Bawden & Robinson, Citation2009). This situational information can be updated to the user via sensory channels: visual, auditory, tactile, gustatory (which is still unexplored), olfactory, and brain stimulation, to avoid overload in one channel. In parallel, it offers more chances to adjust the amount of incoming information in each channel (Allen et al., Citation2013; Wickens, Citation2002). Under the condition of high cognitive load, reliable aid with appropriate information accuracy and volume can improve cognitive performance (Mao et al., Citation2018). The associating difficulty is that the user needs to get used to multi-modal sensory signals. The same principle can be used to design multi-modal communication for human-robot interaction during dismounted operations (Barber & Bendell, Citation2019).
3.3. Preparation for cognitive resilience by human-technology symbiosis
As resilient cognitive performance is a collective effect of human-technology symbiosis, an approach with relevant measures in different phases to facilitate overall efficiency is important (Brunyé et al., Citation2020). A comprehensive preparation is illustrated in , starting from developing the ‘technology capability’, selecting the personnel, and examining their ‘individual capability’. The ‘collective capability’ is determined through training and adaptation with supporting technology. In contrast, the ‘symbiotic capability’ is improved during advanced training or execution, aiming at better human-technology synchronization. ‘Extreme capability’ can be predicted and analyzed after an extreme performance, and ‘capability recovery’ is the last phase of rehabilitation.
The technology capability can be prepared for cognitive resilience according to the steps and measures mentioned in previous subsections. From the technology side, the human-technology interface should be designed with well-defined interactions that do not create redundant stress during usage. Designers can evaluate and differentiate possible interfaces along the two dimensions of usability and potential for stress (Moraveji & Soesanto, Citation2012) while considering symbiosis factors inspired by natural symbiotic relationships (Barravecchia et al., Citation2023). The physical and mental aspects of user needs during operation can be analyzed with different stressful scenarios to develop the technical functions (Hartley et al., Citation2022; Militello et al., Citation2015). To prepare for technology development, a method to collect real-life data sets from a realistic experiment (Weidinger et al., Citation2019), or from soldier performance during augmented training under the effect of stressors or in arousal status (Napier et al., Citation2016) can be utilized. These data can be used to develop technological functions according to human physiological reactions under specific circumstances. The stability of technology under different operational conditions needs to be examined, while human factors such as expected reactions and behaviors should be considered. As technology requires necessary infrastructures, secure connectivity, suitable network protocol, or cybersecurity measures can be deployed.
Before thinking of using technology, the training for cognitive resilience can start with training the personnel (Hallal-Kirk et al., Citation2019) with cognitive basic function, capability, and execution skills, such as improving working memory, inhibitory control, and mental set shifting (Blacker et al., Citation2019). Even without supporting technology, human performance during military operations is expected to have the least impaired performance during stress (Steinberg et al., Citation2018). Individual characteristics such as overindulgence, impulsive behaviors, etc. should be discovered and managed with appropriate training (Blacker et al., Citation2019). Besides the use of interactive multimedia instruction, personalization can further encourage generative processing (Blankenbeckler et al., Citation2014). During this phase, the natural capability in both cognitive and physical aspects of the individual should be measured in different environmental conditions without the technical support to predict the psycho-physiological and cognitive response (Mekjavic et al., Citation2023; L. Taylor et al., Citation2016; Wallace, Citation2023). Mitroff et al. provide an example of measuring visual search competency within distractors (Mitroff et al., Citation2018). The user profile, prior knowledge, and personal physiological states and traits that influence cognitive performance (Martin et al., Citation2020) should also be studied to tailor the technology to the selected personnel. Selecting a person based on the unique brain function can be done with wearable functional near-infrared spectroscopy with a machine learning algorithm (Saikia, Citation2023). Individual variables such as technology familiarity and bias that affect the training and adaptation efficiency with technology should be assessed to predict the possible adeptness.
With the popularity of mobile device technology, it is possible to assess personal capability by personal convenience. To assess the efficiency of supporting technologies on different groups of users, cognitive load can be aroused by a computer task or mobile game (Davila & Hourani, Citation2018) in a training room or home environment to establish the baseline behavior. For instance, stress relaxation training with blue-tooth-based heart rate monitoring, and heart rate variability biofeedback can positively affect users with positive re-framing and active coping style (Davila & Hourani, Citation2018). Based on these results, the resilience performance of the technology users can be predicted. In this early phase, individual moral assessment should be considered with rules and norms for hiring personnel (Burken & van Bezooijen, Citation2015), as a basis to anticipate the decisions that are later made by the symbiosis.
After selecting the personnel, the intended technology can be adjusted according to the individual ergonomic, physical, and physiological characteristics (Bequette et al., Citation2020; Elliott et al., Citation2010), even according to specific physical body features. More technical adaptations can be made according to the initial result during the usage of the prototype (Vatsal & Hoffman, Citation2017; Zocco et al., Citation2015). Individual training with integrated physical and cognitive exercises can be carried out (Fischer et al., Citation2015). This initiative will enhance the collective capability of this symbiosis, and facilitate a natural interaction and adaptation between physical fitness and cognitive function. As inspired by (Sottilare et al., Citation2017), a virtual environment with the fusion of visual and haptic feedback can be used for training and exercising cognitive processes with support from adaptive instruction. Since multi-modal human-technology interaction is established, the need to keep track of the different channels arose, to avoid information overload or unfamiliarity of the user on each and all channels.
Before elaborate complex training scenario, targeted training for a specialized domain (e.g. rig a cargo bag, maintain a weapon, inspect a parachute, or shoot and azimuth during navigation) (Brunyé et al., Citation2020) with repetitive elemental tasks can be conducted without any stimulus or stressors. This method gradually introduces technology functions with human users (Vatsal & Hoffman, Citation2017). Once the users learn what to do in a given situation first, they will be able to respond correctly in an improvised situation. The elemental tasks during familiarization should contain the most basic components (Bequette et al., Citation2020) with high psychological fidelity, to make a successful training transfer (Blacker et al., Citation2019; Hartley et al., Citation2022). The technology capability should be tested again after training and during the stable region of the learning curve, to measure the enhanced effect on collective capability. Mathematical models that aim at predicting human cognitive performance under stress effect can be utilized for prediction purpose (Brunyé et al., Citation2021). Anthropomorphism attribution of supporting technology is proposed by (Cappuccio et al., Citation2022), to facilitate the explicit and implicit attentional, behavioral responses with war-fighters during human-machine team operation. The gestural and behavioral changes of the users after getting used to technology should also be examined to prevent long-term malfunction during later operation, such as the gait parameters (Bequette et al., Citation2020), or relying behavior on technology. An experiment can combine the primary and secondary tasks with different stressors to analyze the trade-off in performance (Lenné et al., Citation2014). Throughout these steps, mutual trust can be established, with a technology trade-off that can be discovered early. This step can generate ideas in design and functions for further technology improvement.
The stimulus, stressors, and disturbance from the above-mentioned ‘unusual’, ‘unexpected’, and ‘overburdened’ groups are introduced in this phase. The user should understand more about the technology, and when, which context to rely on it, under the expanded context of possible stressors and disturbances during operation. The appearance of these factors may alter the previously learned standard procedures and tasks, and force the users to come up with flexible solutions. Based on a detailed task analysis (Blacker et al., Citation2019) of required knowledge, skills, and abilities (Hartley et al., Citation2022), the resilience stimuli should be introduced from every aspect: work environment, content, and context. This step helps human users understand the limits of technology and avoid over-reliance, which is an important principle to make complex decisions in military settings (Cook et al., Citation2007). VR can help to create the stimuli from environmental factors to test the cognitive stress experienced by soldiers (Bartlett et al., Citation2013). With the use of VR to replicate a realistic task during operation, the cognitive ability of a soldier under normal and stimulated scenarios can be conducted, such as VR Stroop Task (T. D. Parsons et al., Citation2011). The unexpected events can also be simulated qualitatively, in which the human attrition and will to fight can be predicted with a system dynamic approach (Richmond et al., Citation2021).
Besides physical training, mental training also contributes to higher physical readiness, therefore, a holistic and comprehensive fitness program is necessary (Boykin & Rice, Citation2019). Training for in-depth technology adaptation will be considered with physical-mental coordination.
The symbiotic capability in different stages of the operation should be considered, even during change over of remote control, as many possibilities of interruption and disruption can happen during this sensitive time, and the cognitive load increases dramatically with a huge wave of new information (Machuca et al., Citation2012). For operations and tasks that require complex decision-making, technology can be developed to facilitate the sharing of SA (Lyreskog et al., Citation2023), diving the decision into smaller tasks that two or more individuals can sense, think and make decisions together (Jiang et al., Citation2019; Salmon et al., Citation2017).
Technology should be tested in an environment that is as close to reality as possible (Rice et al., Citation2019), using the combination of VR, AR, and mixed reality with live training. The case of technology malfunction or breakdown needs to be considered as another extreme condition and should be carried out in parallel with the scenario of no tech support, to increase the resiliency of human users in these scenarios.
In this phase, to prepare for extreme conditions operation, more advanced training should be conducted with the appearance of ‘detrimental’ stressors. As creating crisis scenarios in a VR environment is easier in real life, more technology-based and technology-distributed training programs can be deployed (Baltos et al., Citation2018). Extreme conditions with mission-based training can be carried out with lethal decision-making and detrimental effects.
As TBI and post-traumatic stress symptoms can yield deficits in attention, memory, problem-solving (Hampton, Citation2011), willing attention disengaging and shifting from threat (Kuester et al., Citation2022), cognitive resiliency should also be re-test for users after traumatic events. Though this rehabilitation phase is not designed in the initial search strategy of this paper, the included studies also suggested the use of proposed technologies to facilitate this process and support the human soldiers in gaining their prior cognitive skills (for Disease Control C and Prevention, Citation2003).
Many of the deployed technologies from the included studies can be applied to this phase. Outside of the mission, recovery can be facilitated with just-in-time adaptive intervention with the support of wearable technology that enhances treatment personalization and effectiveness (Pulantara et al., Citation2018), or a therapy system with VR exposure to realistic mission environment (Rizzo et al., Citation2011). Military tasks or simulators by VR can be used to provide a rehabilitation environment or to assess the cognitive ability of soldiers with traumatic brain injury (Edwards et al., Citation2014). AR with Microsoft HoloLens is used to develop a platform to detect the mTBI from a soldier and assess return-to-duty readiness under basic and complex scenarios (Scherer et al., Citation2018). With active duty service members who suffer combat-related PTSD, VR-supported therapy can offer an environment with manageable stimuli both on screen and in the real world (Cukor et al., Citation2015; Rizzo et al., Citation2009). However, this application is different as it only focuses on environmental exposure while disposing of action encouragement for revenge.
3.4. Lessons for technology-supported cognitive resilience in Industry 5.0
From military examples discussed in previous sections, it can be seen that technology development should have been through careful adaptation and detailed consideration before coming into application. Developing such a human-centric technology with resilience requirements demands a multi-disciplinary approach, and besides the detailed usage context definition, physiological and psychological mechanisms are important to define the prediction model (Brunyé et al., Citation2021).
Despite a large difference between the two fields, the authors tried to make a comparable ground for technology-enabled human performance in industrial and military operations, as illustrated in . The industrial work environment is usually a closed space and well-controlled, has a stable work context and work content that requires mostly repetitive tasks, consistent from the training phase into the execution phase. The initiatives from military fields such as individual selection, training with stressors, and execution under uncertainty are not commonplace in industrial standards. The users in the military field were trained with excellent skills before using technology, while the users in the industrial environment were normal civilians, without special knowledge or physical and cognitive skills. Given the fact that military operation usually requires human users to perform under a great level of stress above their stress threshold, industrial applications that take place below an acceptable stress level may not require such strict protocol.
Figure 8. The comparison of human-technology symbiosis performance between industrial and military operation.
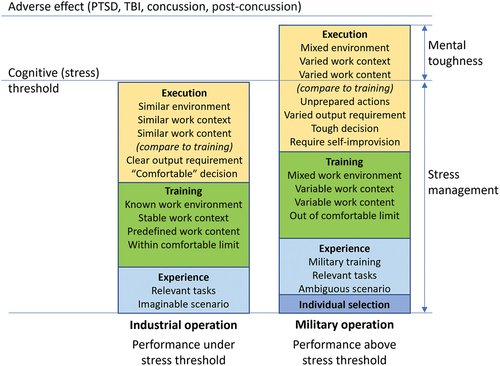
However, in the transition towards an O5.0 model operating under a Volatility, Uncertainty, Complexity, and Ambiguity era (Romero & Stahre, Citation2021), a similar approach can also be applied for the industrial setting, improve the adaptation of human users with their technology to yield better and resilient performance. There are several bases for this bold initiative, such as many similar individual capability enhancements that have been deployed in industrial environments (e.g. biofeedback training (Purwandini Sutarto et al., Citation2012; Sutarto et al., Citation2013), stress management programs (Landsbergis, Citation2018), stress monitoring (Leone et al., Citation2020)). In the case of remote control and system operation from a closed control room in modern warfare, the boundary between using the same technology for military and industrial operations becomes blurred, except for hard decision-making, expected results, and cognitive resilience levels that are incomparable. Through included studies, there are military technologies were tested with normal civilian populations, and proved that they can have similar positive resilient effects on critical situations. Though some of them are intended for military use, their work setting suggests a similar usage for civilian use (Brunyé et al., Citation2021). On the contrary, some developed technologies from industrial sectors are prepared for the same uncertainty and dangerous situations, which are similar to military settings (Aljaberi & Abd Rahman, Citation2023).
Resilient human-machine symbiosis in an industrial environment can be built with the same architecture similar to that illustrated in , with the use of O4.0 technologies remaining the same role as a supporting pillar. Factors from the work environment and work context can be simplified with only indoor environmental factors, such as noise, temperature, airflow, etc., without the updated details of other elements in military settings such as civilians, and hostages. The work content factors can contain only fixed production goals with predefined repetitive tasks and motions. The user capability and technology capability tracking can have a similar function, to ensure smooth cooperation between humans and technology. The information exchange and support from technology can be also signaled to human users via different sensory channels.
To prepare for resilience, similar stimulus or disturbances from different groups such as ‘unusual’, ‘unexpected’, and ‘overburdened’ in should be introduced into technology development, training, and testing. The human-technology symbiosis in an industrial environment can be trained to operate under the appearance of stressors, such as multi-tasking with divided attention, or normal operating when powered down (Bequette et al., Citation2020). The ‘detrimental’ stressors are rare in industrial environments, however, they can be replicated during the context of special safety-critical operations.
A comprehensive approach to preparing for cognitive resilience as illustrated in can also be adjusted to apply to an industrial context. Technology development can be carried out based on risk analysis with process analysis methods. To analyze the human-technology interaction during critical events, activity diagrams can be used to decompose the cognitive and physical activity of human users and machines, therefore predicting the possible excessive cognitive activity and necessary technology support (LaMonica et al., Citation2022). Military approaches such as red teaming can be applied to analyze critical situations or processes to discover more uncertainty and risk within an environment of multiple agents from available big data (Abbass & Abbass, Citation2015a, Citation2015b). Individual capability with natural performance without the technology support can be measured in a similar method, to predict the later technology efficiency. Instead of the moral dimension, safety awareness can be assessed. Training and adaptation with technology, and execution and improvement can be carried out, with the gradual introduction of stressors, and mental training, aiming at a better synchronization between humans and technology. During testing and in the operation phase, disturbance can be replicated in the manufacturing line and system as a practice/drill, to test the resiliency and readiness, from productivity, quality, and safety aspects. The aftermath of extreme performance may not appear in an industrial environment, therefore it is not necessary to replicate them and perform a capability recovery step unless there is a specific requirement for dangerous tasks, such as operation in a nuclear power plant.
4. Conclusions
This study took an ambitious step toward the preparation for cognitive resilience with O5.0 solutions. Different studies reporting the technology-enabled performance of human users in military fields were collected and analyzed considering the cognitive aspect. The results reflect how the human factors were incorporated into different phases of technology development, fabrication, testing, and operation, with consideration of stressors, stimulus, and disturbances from different sources. Based on the collected evidence on influencing factors and side effects of these technology usage scenarios, a human-technology symbiosis is constructed, with a comprehensive approach for operation preparation. This effort suggests a new perspective for O5.0 solutions and applicable initiatives that can be deployed in the industrial field.
From a military perspective, how and when to apply technology is still under concern. Before implementing the operation model, theoretical and mathematical models that are integrated with relevant physiological relationships are necessary. Though wearable technology now enables a real-time measuring of human physiological parameters, however, some specialized measures are hard to conduct in open field setup, or impractical to interpret during special conditions such as thermoregulatory sweating and intense movement, thus are neglected in theoretical model development (Brunyé et al., Citation2021). Portability is important for technology development, as it enables more mobility during technology deployment (Rice et al., Citation2019). Though controversially, some researchers declared that supported information from technology (i.e. opaque alarm system) has no relationship with the trust and therefore, the performance of its user (Harden et al., Citation2014). The authors call for attention in future research, that examines the impact of user reliability on performance with technological support.
Due to much of the relevant research being conducted in defense laboratories without dissemination to the scientific literature (Brunyé et al., Citation2020), researchers are not able to be aware of all resilience requirements during the performance of soldiers and militants, as well as the detailed characteristics of the tested population. There can be more resilience requirements in other operations that are not incorporated with technology (Fincannon et al., Citation2013), therefore not included in the synthesis of this study.
Defying the odds, this study captured an overview of the current research direction aiming at technology-enabled cognitive resilience in military operations. The preparation for cognitive resilience in this context gave a comprehensive approach, with detailed steps and demanding requirements. This big picture helps to imagine how a resilient symbiosis in an industrial environment should be, taking into consideration the difference between the work environment, and expected performance between both fields. By taking benchmarks from military fields, more attention should be paid during technology development, individual and collective training with technologies.
Supplemental Material
Download PDF (417.3 KB)Disclosure statement
No potential conflict of interest was reported by the author(s).
Supplementary material
Supplemental data for this article can be accessed online at https://doi.org/10.1080/21693277.2024.2368232
Additional information
Funding
References
- Abbass, H. A., & Abbass, H. A. (2015a). Analytics of risk and challenge. Computational Red Teaming: Risk Analytics of Big-Data-To-Decisions Intelligent Systems (pp. 47–48). Cham: Springer International Publishing. https://doi.org/10.1007/978-3-319-08281-3_2
- Abbass, H. A., & Abbass, H. A. (2015b). Big-data-to-decisions red teaming systems. Computational Red Teaming: Risk Analytics of Big-Data-To-Decisions Intelligent Systems (pp. 105–158). Cham: Springer International Publishing. https://doi.org/10.1007/978-3-319-08281-3_2
- Abich, J., Eudy, M., Murphy, J., Garneau, C., Raby, Y., & Amburn, C. (2018). Use of the augmented reality sandtable (ares) to enhance army cbrn training. HCI International 2018–Posters’ Extended Abstracts: 20th International Conference, HCI International 2018 (pp. 223–230). Springer, Las Vegas, NV, USA. July 15–20, 2018
- Alicia, T. J., Taylor, G. S., Turpin, T. S., & Surana, A. (2018). Removing the bottleneck: Utilizing autonomy to manage multiple uas sensors from inside a cockpit. In E. K. Robert, W. G. Douglas, M. S. Charles, & G. N. Hoa (Eds.), Unmanned systems technology XX (Vol. 10640, pp. 130–148). SPIE.
- Aljaberi, S. A. A., & Abd Rahman, N. I. (2023). Development of operator training simulator (ots) in refining process for atmospheric distillation column. Journal of Engineering Science and Technology, 18(4), 2221–2237.
- Allen, J. P., Regli, S. H., Stibler, K. M., Craven, P., Gerken, P., & Tremoulet, P. D. (2013). The information exoskeleton: Augmenting human interaction with information systems. Foundations of Augmented Cognition: 7th International Conference, AC 2013, Held as Part of HCI International 2013 (pp. 553–561). Springer, Las Vegas, NV, USA. Proceedings 7. July 21–26, 2013.
- Andrews, S. C., Hoy, K. E., Enticott, P. G., Daskalakis, Z. J., & Fitzgerald, P. B. (2011). Improving working memory: The effect of combining cognitive activity and anodal transcranial direct current stimulation to the left dorsolateral prefrontal cortex. Brain Stimulation, 4(2), 84–89. https://doi.org/10.1016/j.brs.2010.06.004
- Bakhshandeh, M., & Liyanage, J. (2023). Dealing with abnormalities and deviations to enhance resilience in engineering assets: A critical review from human factors and decision-making perspectives under complex operational contexts. IOP Conference Series: Materials Science and Engineering (Vol. 1294. pp. 012037). IOP Publishing.
- Baltos, G. C., Chomata, F. S., Vidakis, I. G., & Balodis, J. (2018). Modern learning and training tools which can be properly adapted and designed to reinforce specialized courses on effective crisis management. Journal of Educational and Social Research, 8(3), 27. https://doi.org/10.2478/jesr-2018-0028
- Barber, D., & Bendell, R. (2019). Design approach for investigating multimodal communication in dismounted soldier-robot interaction. HCI International 2019–Late Breaking Papers: 21st HCI International Conference, HCII 2019 (pp. 3–14). Springer, Orlando, FL, USA. Proceedings 21, July 26–31, 2019.
- Barravecchia, F., Bartolomei, M., Mastrogiacomo, L., & Franceschini, F. (2023). Redefining human–robot symbiosis: A bio-inspired approach to collaborative assembly. The International Journal of Advanced Manufacturing Technology, 128(5–6), 2043–2058. https://doi.org/10.1007/s00170-023-11920-1
- Bartlett, J. L., Sessoms, P. H., & Reini, S. A. (2013). Strength through science: Using virtual technology to advance the warfighter. Aviation, Space, and Environmental Medicine, 84(2), 165–166. https://doi.org/10.3357/ASEM.3578.2013
- Bartone, P. T. (2006). Resilience under military operational stress: Can leaders influence hardiness? Military Psychology, 18(sup1), S131–S148. https://doi.org/10.1207/s15327876mp1803s_10
- Bawden, D., & Robinson, L. (2009). The dark side of information: Overload, anxiety and other paradoxes and pathologies. Journal of Information Science, 35(2), 180–191. https://doi.org/10.1177/0165551508095781
- Bequette, B., Norton, A., Jones, E., & Stirling, L. (2020). Physical and cognitive load effects due to a powered lower-body exoskeleton. Human Factors, 62(3), 411–423. https://doi.org/10.1177/0018720820907450
- Berka, C., Behneman, A., Kintz, N., Johnson, R., & Raphael, G. (2010). Accelerating training using interactive neuro-educational technologies: Applications to archery, golf and rifle marksmanship. International Journal of Sport & Society, 1(4), 87. https://doi.org/10.18848/2152-7857/CGP/v01i04/54040
- Billing, D. C., Fordy, G. R., Friedl, K. E., & Hasselstrøm, H. (2021). The implications of emerging technology on military human performance research priorities. Journal of Science and Medicine in Sport, 24(10), 947–953. https://doi.org/10.1016/j.jsams.2020.10.007
- Bishop, J. (2015). The role of affective computing for improving situation awareness in unmanned aerial vehicle operations: A US perspective. In V. Jordi (Ed.), Handbook of research on synthesizing human emotion in intelligent systems and robotics (pp. 404–414). IGI Global. https://doi.org/10.4018/978-1-4666-7278-9
- Blacker, K. J., Hamilton, J., Roush, G., Pettijohn, K. A., & Biggs, A. T. (2019). Cognitive training for military application: A review of the literature and practical guide. Journal of Cognitive Enhancement, 3(1), 30–51. https://doi.org/10.1007/s41465-018-0076-1
- Blankenbeckler, P. N., Graves, T. R., & Wampler, R. L. (2014). Designing interactive multimedia instruction to address soldiers’ learning needs. In Research report 1979. US Army Research Institute for the ….
- Boshuijzen van Burken, C. (2019). Modern military operations: A normative practice approach to moral decision making. In J. d. V. Marc & J. Henk (Eds.), The normative nature of social practices and ethics in professional environments (pp. 115–133). IGI Global. https://doi.org/10.4018/978-1-5225-8006-5
- Bostrom, N., & Sandberg, A. (2009). Cognitive enhancement: Methods, ethics, regulatory challenges. Science and Engineering Ethics, 15(3), 311–341. https://doi.org/10.1007/s11948-009-9142-5
- Boyce, M. W., Gardony, A. L., Shorter, P., Horner, C., Stainrod, C. R., Flynn, J., Brunyé, T. T., & Amburn, C. R. (2019). Characterizing the cognitive impact of tangible augmented reality. Virtual, Augmented and Mixed Reality. Multimodal Interaction: 11th International Conference, VAMR 2019, Held as Part of the 21st HCI International Conference, HCII 2019 (pp. 416–427). Springer, Orlando, FL, USA. Proceedings, Part I 21, July 26–31, 2019.
- Boyce, M. W., Reyes, R. J., Cruz, D., Amburn, C. R., Goldberg, B., Moss, J. D., & Sottilare, R. A. (2016). Effect of topography on learning military tactics–integration of generalized intelligent framework for tutoring (gift) and augmented reality sandtable (ARES) (Report No. ARL-TR-7792). Washington: Army Research Laboratory. https://apps.dtic.mil/sti/tr/pdf/AD1017876.pdf
- Boyce, M. W., Thomson, R. H., Cartwright, J. K., Feltner, D. T., Stainrod, C. R., Flynn, J., Ackermann, C., Emezie, J., Amburn, C. R., & Rovira, E. (2022). Enhancing military training using extended reality: A study of military tactics comprehension. Frontiers in Virtual Reality, 3, 754627. https://doi.org/10.3389/frvir.2022.754627
- Boykin, G. L., & Rice, V. J. (2019). Physical readiness is more than physical fitness: Relationships between army physical fitness test scores and self-reports of physical and psychological fitness. International Conference on Applied Human Factors and Ergonomics (pp. 176–183). Springer.
- Brandão, W. L., & Pinho, M. S. (2017). Using augmented reality to improve dismounted operators’ situation awareness. 2017 IEEE Virtual Reality (VR) (pp. 297–298). IEEE.
- Breque, M., De Nul, L., & Petridis, A. European Commission, Directorate-General for Research and Innovation. (2021). Industry 5.0: Towards asustainable, human-centric and resilient European industry (Report No. KI-BD-20-021-EN-N). Publications Office of the European Union. https://data.europa.eu/doi/10.2777/308407
- Brewer, R., Baker, A., Krausman, A., Neubauer, C., Forster, D., Scharine, A., Berg, S., Davis, K., & Schaefer, K. E. (2022). Evaluation of human-autonomy team trust for weaponized robotic combat vehicles [ master’s thesis]. Faculty of Applied Health Sciences, Brock University,
- Brocal, F., González, C., Komljenovic, D., Katina, P. F., & Sebastián, M. A. (2019). Emerging risk management in industry 4.0: An approach to improve organizational and human performance in the complex systems. Complexity, 2019, 1–13. https://doi.org/10.1155/2019/2089763
- Brown, N., Coyne, J., Sibley, C., & Foroughi, C. (2020). Human performance in the simulated multiple asset routing testbed (smart): An individual differences approach. Advances in Human Error, Reliability, Resilience, and Performance: Proceedings of the AHFE 2019 International Conference on Human Error, Reliability, Resilience, and Performance (pp. 95–105). Springer, Washington DC, USA. July 24–28, 2019.
- Brumley, L., Kopp, C., & Korb, K. (2006). The orientation step of the ooda loop and information warfare. Information Warfare and Security Conference (pp. 20). Citeseer.
- Brunyé, T. T., Brou, R., Doty, T. J., Gregory, F. D., Hussey, E. K., Lieberman, H. R., Loverro, K. L., Mezzacappa, E. S., Neumeier, W. H., Patton, D. J., Soares, J. W., Thomas, T. P., & Yu, A. B. (2020). A review of us army research contributing to cognitive enhancement in military contexts. Journal of Cognitive Enhancement, 4(4), 453–468. https://doi.org/10.1007/s41465-020-00167-3
- Brunyé, T. T., Holmes, A., Cantelon, J., Eddy, M. D., Gardony, A. L., Mahoney, C. R., & Taylor, H. A. (2014). Direct current brain stimulation enhances navigation efficiency in individuals with low spatial sense of direction. Neuroreport, 25(15), 1175–1179. https://doi.org/10.1097/WNR.0000000000000214
- Brunyé, T. T., Hussey, E. K., Fontes, E. B., & Ward, N. (2019). Modulating applied task performance via transcranial electrical stimulation. Frontiers in Human Neuroscience, 13, 140. https://doi.org/10.3389/fnhum.2019.00140
- Brunyé, T. T., Yau, K., Okano, K., Elliott, G., Olenich, S., Giles, G. E., Navarro, E., Elkin-Frankston, S., Young, A. L., & Miller, E. L. (2021). Toward predicting human performance outcomes from wearable technologies: A computational modeling approach. Frontiers in Physiology, 12, 738973. https://doi.org/10.3389/fphys.2021.738973
- Buchler, N., Marusich, L. R., & Sokoloff, S. (2014, June 30). The warfighter associate: Decision-support software agent for the management of intelligence, surveillance, and reconnaissance (isr) assets. In Michael A. Kolodny (Ed.), Ground/Air Multisensor Interoperability, Integration, and Networking for Persistent ISR V, USA. (Vol. 9079, p. 907902). SPIE. https://doi.org/10.1117/12.2073085
- Burken, C. B., & van Bezooijen, B. (2015). Morally responsible decision making in networked military operations. In K. Bert-Jaap, O. Ilse, R. Henny, S. Tsjalling, & V. D. H. Jeroen (Eds.), Responsible Innovation 2: Concepts, Approaches, and Applications (Vol. 1, pp. 265–282). Cham: Springer. https://doi.org/10.1007/978-3-319-17308-5_14
- Caldarola, E. G., Modoni, G. E., & Sacco, M. (2018). Enhancing the workforce skills and competences by leveraging a human-centered knowledge-based system in the rise of industry 4.0. International Journal on Advances in Intelligent Systems, 11(3&4), 309–324.
- Cappuccio, M. L., Galliott, J. C., & Sandoval, E. B. (2022). Saving private robot: Risks and advantages of anthropomorphism in agent-soldier teams. International Journal of Social Robotics, 14(10), 2135–2148. https://doi.org/10.1007/s12369-021-00755-z
- Caron, J. F. (2018). The ethics of developing capacity-increasing technologies. A theory of the super soldier: The morality of capacity-increasing technologies in the military (pp. 72–92). Manchester University Press. https://www.jstor.org/stable/j.ctv18b5pmz.8
- Centers for Disease Control and Prevention. (2003). Report to congress on mild traumatic brain injury in the United States: Steps to prevent a serious public health problem (Vol. 45).
- Chacón, A., Angulo, C., & Ponsa, P. (2020). Developing cognitive advisor agents for operators in industry 4.0. In R. M. Luis, A. O. R. Roque, & D. P. Miguel (Eds.), New Trends in the Use of Artificial Intelligence for the Industry (Vol. 4, p. 127). Intechopen. https://doi.org/10.5772/intechopen.86015
- Chaplain, C. (2018). Weapon systems cybersecurity: Dod just beginning to grapple with scale of vulnerabilities. GAO Report No GAO-19-128.
- Chen, J. Y. (2018). Effects of operators’ spatial ability on their performance of target detection and robotics tasks. In M. John, A. Laurel, S-K. Pamela, & L. John (Eds.), Designing soldier systems: Current issues in human factors (human factors in defence) (Vol. 1, pp. 53–67). London: CRC Press. https://doi.org/10.1201/9781315576756
- Chi, R. P., Fregni, F., & Snyder, A. W. (2010). Visual memory improved by non-invasive brain stimulation. Brain Research, 1353, 168–175. https://doi.org/10.1016/j.brainres.2010.07.062
- Chmielewski, M. (2017). Situation awareness tools supporting soldiers and low level commanders in land operations. application of gis and augmented reality mechanisms. Proceedings of the Geographic Information Systems Conference and Exhibition “GIS ODYSSEY (pp. 85–94).
- Chmielewski, M., Sapiejewski, K., & Sobolewski, M. (2019). Application of augmented reality, mobile devices, and sensors for a combat entity quantitative assessment supporting decisions and situational awareness development. Applied Sciences, 9(21), 4577. https://doi.org/10.3390/app9214577
- Coleman, S. (2013). Ethical challenges of new military technologies. In N. Hitoshi & M. Robert (Eds.), New technologies and the law of armed conflict (Vol. 1, pp. 29–41). T.M.C. Asser Press The Hague. https://doi.org/10.1007/978-90-6704-933-7
- Cook, M. J., Noyes, J. M., & Masakowski, Y. (2007). Decision making in complex environments. Ashgate Publishing, Ltd.
- Core, M. G., Lane, H. C., Van Lent, M., Gomboc, D., Solomon, S., & Rosenberg, M. (2006). Building explainable artificial intelligence systems. AAAI.
- Cukor, J., Gerardi, M., Alley, S., Reist, C., Roy, M., Rothbaum, B. O., Difede, J., & Rizzo, A. (2015). In E. C. Ritchie, (Ed.), Posttraumatic Stress Disorder and Related Diseases in Combat Veterans (Vol. 1, pp. 69–83). Cham: Springer. https://doi.org/10.1007/978-3-319-22985-0_7
- Cummings, D., Prasad, M., Lucchese, G., Aikens, C., & Hammond, T. A. (2013). Multi-modal location-aware system for paratrooper team coordination. In E. M. Wendy (Ed.), CHI ‘13: CHI Conference on Human Factors in Computing Systems, Association for Computing Machinery, Paris France, 27 April 2013. (pp. 2385–2388). https://doi.org/10.1145/2468356.2468779
- Dais, S. (2017). Industrie 4.0 – Anstoß, Vision, Vorgehen. In B. Vogel-Heuser, T. Bauernhansl, & M. Hompel (Eds.), Handbuch Industrie 4.0 Bd.4: Allgemeine Grundlagen [Springer Reference Technik]. (2nd ed., Vol. 2, pp. 261–277). Heidelberg: Springer Vieweg Berlin. https://doi.org/10.1007/978-3-662-53254-6
- Daly, T., Murphy, J., Anglin, K., Szalma, J., Acree, M., Landsberg, C., & Bowens, L. (2017). Moving vigilance out of the laboratory: Dynamic scenarios for uas operator vigilance training. Augmented Cognition. Enhancing Cognition and Behavior in Complex Human Environments: 11th International Conference, AC 2017, Held as Part of HCI International 2017 (pp. 20–35). Springer, Vancouver, BC, Canada. July 9–14, 2017
- Davila, M., & Hourani, L. (2018). Evaluation of hrv biofeedback as a resilience building intervention in the reserve component (bart) (Report No. W81XWH-16-1-0346). Fort Detrick, Maryland 21702-5012: U.S. Army Medical Research and Materiel Command. https://apps.dtic.mil/sti/tr/pdf/AD1095180.pdf
- DC CSAFW. (2010). Report on technology horizons: A vision for air force science and technology during 2010-2030 (Vol. 1). Office of the US Air Force Chief Scientist.
- Department of the Army. (2009). Warrior Leader Skills Level 2, 3, and 4. Soldier’s Manual of Common Tasks and Warrior Skills Level 2, 3, and 4 (pp. 1–214). Washington: Pentagon Publishing.
- Devlin, S. P., Moacdieh, N. M., Wickens, C. D., & Riggs, S. L. (2020). Transitions between low and high levels of mental workload can improve multitasking performance. IISE Transactions on Occupational Ergonomics and Human Factors, 8(2), 72–87. https://doi.org/10.1080/24725838.2020.1770898
- Doorley, J. D., Goodman, F. R., Kelso, K. C., & Kashdan, T. B. (2020). Psychological flexibility: What we know, what we do not know, and what we think we know. Social and Personality Psychology Compass, 14(12), 1–11. https://doi.org/10.1111/spc3.12566
- Dorneich, M., Whitlow, S., Ververs, P. M., Carciofini, J., & Creaser, J. (2004). Closing the loop of an adaptive system with cognitive state. Proceedings of the Human Factors and Ergonomics Society Annual Meeting (Vol. 48. pp. 590–594). SAGE Publications Sage CA, Los Angeles, CA.
- Drnec, K., Gremillion, G., Donavanik, D., Canady, J. D., Atwater, C., Carter, E., Haynes, B. A., Marathe, A. R., & Metcalfe, J. S. (2018). The role of psychophysiological measures as implicit communication within mixed-initiative teams. Virtual, Augmented and Mixed Reality: Interaction, Navigation, Visualization, Embodiment, and Simulation: 10th International Conference, VAMR 2018, Held as Part of HCI International 2018 (pp. 299–313). Springer, Las Vegas, NV, USA. Proceedings, Part I 10. July 15–20, 2018.
- Edwards, J., Vess, J., Reger, G., & Cernich, A. (2014). The use of virtual reality in the military’s assessment of service members with traumatic brain injury: Recent developments and emerging Opportunities. Applied Neuropsychology: Adult, 21(3), 220–230. https://doi.org/10.1080/09084282.2013.796554
- Eesee, A. K., Jaskó, S., Eigner, G., Abonyi, J., & Ruppert, T. (2024). Extension of haas for the management of cognitive load. IEEE Access.
- Elliott, L. R., van Erp, J., Redden, E. S., & Duistermaat, M. (2010). Field-based validation of a tactile navigation device. IEEE Transactions on Haptics, 3(2), 78–87. https://doi.org/10.1109/TOH.2010.3
- Elyounnss, M., Holloway, A., Penders, J., & Alboul, L. (2016). Development of an intelligent robotic rein for haptic control and interaction with mobile machines. Conference Towards Autonomous Robotic Systems (pp. 111–115). Springer.
- Emond, B., Fournier, H., & Lapointe, J. F. (2010). Applying advanced user models and input technologies to augment military simulation-based training. Proceedings of the 2010 Spring Simulation Multiconference (pp. 1–7).
- Endsley, M. R. (1993). Situation awareness and workload- flip sides of the same coin. International Symposium on Aviation Psychology (pp. 906–911), Columbus, OH.
- Fallaha, M., Cinar, Z. M., Korhan, O., & Zeeshan, Q. (2020). Operator 4.0 and cognitive ergonomics. Industrial Engineering in the Digital Disruption Era: Selected papers from the Global Joint Conference on Industrial Engineering and Its Application Areas, GJCIE 2019 (pp. 217–228). Springer, Gazimagusa, North Cyprus, Turkey. September 2–3, 2019.
- Fay, D., Stanton, N., & Roberts, A. P. (2019). Exploring ecological interface design for future rov capabilities in maritime command and control. Advances in Human Aspects of Transportation: Proceedings of the AHFE 2018 International Conference on Human Factors in Transportation (pp. 264–273). Loews Sapphire Falls Resort at Universal Studios, Orlando, Florida, USA Springer. July 21–25, 2018.
- Feltman, K. A., Hayes, A. M., Bernhardt, K. A., Nwala, E., & Kelley, A. M. (2020). Viability of tdcs in military environments for performance enhancement: A systematic review. Military Medicine, 185(1–2), e53–e60. https://doi.org/10.1093/milmed/usz189
- Fertonani, A., Rosini, S., Cotelli, M., Rossini, P. M., & Miniussi, C. (2010). Naming facilitation induced by transcranial direct current stimulation. Behavioural Brain Research, 208(2), 311–318. https://doi.org/10.1016/j.bbr.2009.10.030
- Fincannon, T., Keebler, J. R., Jentsch, F., & Curtis, M. (2013). The influence of camouflage, obstruction, familiarity and spatial ability on target identification from an unmanned ground vehicle. Ergonomics, 56(5), 739–751. https://doi.org/10.1080/00140139.2013.771218
- Fischer, M. V., Stone, J., Hawkes, T. D., Eveland, E., & Strang, A. J. (2015). Integrative physical and cognitive training development to better meet airmen mission requirements. Procedia Manufacturing, 3, 1580–1586. https://doi.org/10.1016/j.promfg.2015.07.445
- Fletcher, J., & Wind, A. P. (2013). The evolving definition of cognitive readiness for military operations. In F. O’Neil, Harold, S. P. Ray, & L. B. Eva (Eds.), Teaching and measuring cognitive readiness (1st ed., pp. 25–52). NY: Springer. https://doi.org/10.1007/978-1-4614-7579-8
- Flood, A., & Keegan, R. J. (2022). Cognitive resilience to psychological stress in military personnel. Frontiers in Psychology, 13, 809003. https://doi.org/10.3389/fpsyg.2022.809003
- From, J., Perrin, P., O’Neill, D., & Yen, J. (2011). Supporting the commander’s information requirements: Automated support for battle drill processes using r-cast. 2011-MILCOM 2011 Military Communications Conference (pp. 1523–1528). IEEE.
- Games, K. E., Lakin, J. M., Quindry, J. C., Weimar, W. H., & Sefton, J. M. (2015). Prolonged restricted sitting effects in uh-60 helicopters. Aviation, Space, and Environmental Medicine, 86(1), 34–40. https://doi.org/10.3357/AMHP.4092.2015
- Garcia, A., Silva, V., Chavez, C., & Lucena, C. (2002). Engineering multi-agent systems with aspects and patterns. Journal of the Brazilian Computer Society, 8(1), 57–72. https://doi.org/10.1590/S0104-65002002000100006
- Gardony, A. L., Martis, S. B., Taylor, H. A., & Brunyé, T. T. (2021). Interaction strategies for effective augmented reality geo-visualization: Insights from spatial cognition. Human–Computer Interaction, 36(2), 107–149. https://doi.org/10.1080/07370024.2018.1531001
- Geyer, A. (2011). Cognition: What does it have to do with the brain? In D. Schmorrow & C. Fidopiastis (Eds.), Foundations of augmented cognition. Directing the future of adaptive systems (pp. 55–59). Springer Berlin Heidelberg.
- Gladysz, B., Tran, T., Romero, D., van Erp, T., Abonyi, J., & Ruppert, T. (2023). Current development on the operator 4.0 and transition towards the operator 5.0: A systematic literature review in light of industry 5.0. Journal of Manufacturing Systems, 70, 160–185. https://doi.org/10.1016/j.jmsy.2023.07.008
- Godé, C., & Lebraty, J. F. (2013). Improving decision making in extreme situations: The case of a military decision support system. International Journal of Technology and Human Interaction (IJTHI), 9(1), 1–17. https://doi.org/10.4018/jthi.2013010101
- Goldberg, S. L. (2012). Psychology’s contribution to military training. In H. L. Janice & D. M. Michael (Eds.), The Oxford Handbook of Military Psychology [Oxford Library of Psychology] (1st ed., pp. 241–261). Oxford: Oxford University Press. https://doi.org/10.1093/oxfordhb/9780195399325.013.0080
- Golovianko, M., Gryshko, S., Terziyan, V., & Tuunanen, T. (2023). Responsible cognitive digital clones as decision-makers: A design science research study. European Journal of Information Systems, 32(5), 879–901. https://doi.org/10.1080/0960085X.2022.2073278
- Grabowski, M., Rowen, A., & Rancy, J. P. (2018). Evaluation of wearable immersive augmented reality technology in safety-critical systems. Safety Science, 103, 23–32. https://doi.org/10.1016/j.ssci.2017.11.013
- Grier, R. A. (2012). Military cognitive readiness at the operational and strategic levels: A theoretical model for measurement development. Journal of Cognitive Engineering and Decision Making, 6(4), 358–392. https://doi.org/10.1177/1555343412444606
- Guerin, C., Rauffet, P., Chauvin, C., & Martin, E. (2019). Toward production operator 4.0: Modelling human-machine cooperation in industry 4.0 with cognitive work analysis. IFAC-Papersonline, 52(19), 73–78. https://doi.org/10.1016/j.ifacol.2019.12.111
- Guo, Z., Ma, J., & Wang, X. (2017). On the war ethics of unmanned aerial vehicle. Asian Social Science, 13(2), 134–134. https://doi.org/10.5539/ass.v13n2p134
- Hairston, W. D., Chen, J., Barnes, M., Martinez, I., LaFiandra, M., Binseel, M., Scharine, A., Ericson, M., Vaughan, B., Lance, B., & McDowell, K. (2012). Technological areas to improve soldier decisiveness: Insights from the soldier-system design perspective. US Army Research Laboratory.
- Hallal-Kirk, L., Patton, D. J., Johnston, J., Milham, L., Townsend, L., & Riddle, D. (2019). Training for readiness and resilience: Supplemental findings. International Conference on Applied Human Factors and Ergonomics (pp. 165–175). Springer.
- Hampton, T. (2011). Traumatic brain injury a growing problem among troops serving in today’s wars. JAMA, 306(5), 477–479. https://doi.org/10.1001/jama.2011.1092
- Hancock, P. A. (2017). Mind, machine and morality: Toward a philosophy of human-technology symbiosis. CRC Press.
- Harden, J., Bliss, J., Bernard, A., & Jarsaillon, S. (2014). The influence of mental workload and information reliability on aerial search performance. Proceedings of the Human Factors and Ergonomics Society Annual Meeting (Vol. 58. pp. 568–572). SAGE Publications Sage CA, Los Angeles, CA.
- Hardy, J. G., & Hiller, J. (1985). Drill trainer’s guides for light attack squads and platoons. U. S. Army Research Institute for the Behavioral and Social Sciences.
- Hartley, C. A., Hurley, K. J., Morganson, V. J., Kass, S. J., Wheeler Atkinson, B. F., & Pierce, M. B. (2022). Eject, eject, eject! conducting a cognitive task analysis to assess parachute descent training simulators. Ergonomics in Design, 10648046221124786. https://doi.org/10.1177/10648046221124786
- Hatfield, B. D., & Haufler, A. J. (2018). Brain processes during expert cognitive-motor performance: The impact of mental stress and emotion regulation. In S. Rebecca, K. Steven, & D. M. Michael (Eds.), Neurocognitive and physiological factors during high-tempo operations (pp. 167–192).CRC Press. https://doi.org/10.1201/9781315597850
- Hew, P. C. (2017). Distributed cognition sheds light on munitions trajectories in close air support. Ergonomics in Design, 25(2), 12–14. https://doi.org/10.1177/1064804616675243
- Hiller, J., Meliza, L., Hardy, G., Jones, T. L., & Jackson, E. (1985). Drill training and evaluation guidebook for light motorized platoons and squads. U. S. Army Research Institute for the Behavioral and Social Sciences.
- Hodgetts, H. M., Vachon, F., Chamberland, C., & Tremblay, S. (2017). See no evil: Cognitive challenges of security surveillance and monitoring. Journal of Applied Research in Memory and Cognition, 6(3), 230–243. https://doi.org/10.1016/j.jarmac.2017.05.001
- Hsu, W. Y., Zanto, T. P., Anguera, J. A., Lin, Y.-Y., & Gazzaley, A. (2015). Delayed enhancement of multitasking performance: Effects of anodal transcranial direct current stimulation on the prefrontal cortex. Cortex; a Journal Devoted to the Study of the Nervous System and Behavior, 69, 175–185. https://doi.org/10.1016/j.cortex.2015.05.014
- Inagaki, T. (2000). Situation-adaptive autonomy: Dynamic trading of authority between human and automation. Proceedings of the Human Factors and Ergonomics Society Annual Meeting (Vol. 44. pp. 13–16). SAGE Publications Sage CA, Los Angeles, CA.
- Irgens-Hansen, K., Gundersen, H., Sunde, E., Baste, V., Harris, A., Bråtveit, M., & Moen, B. (2015). Noise exposure and cognitive performance: A study on personnel on board royal Norwegian navy vessels. Noise & Health, 17(78), 320. https://doi.org/10.4103/1463-1741.165057
- Jensen, M. N. (2013). Hard moral choices in the military. Journal of Military Ethics, 12(4), 341–356. https://doi.org/10.1080/15027570.2013.869897
- Jha, A. P., Morrison, A. B., Dainer-Best, J., Parker, S., Rostrup, N., & Stanley, E. A. (2015). Minds “at attention”: Mindfulness training curbs attentional lapses in military cohorts. Public Library of Science ONE, 10(2), e0116889. https://doi.org/10.1371/journal.pone.0116889
- Jiang, L., Stocco, A., Losey, D. M., Abernethy, J. A., Prat, C. S., & Rao, R. P. N. (2019). Brainnet: A multi-person brain-to-brain interface for direct collaboration between brains. Scientific Reports, 9(1), 6115. https://doi.org/10.1038/s41598-019-41895-7
- Johnson, J. (2023). Automating the ooda loop in the age of intelligent machines: Reaffirming the role of humans in command-and-control decision-making in the digital age. Defence Studies, 23(1), 43–67. https://doi.org/10.1080/14702436.2022.2102486
- Johnson, D. M., & Hiller, J. H. (1985). Drill trainer’s guides for assault gun crews and platoons. U. S. Army Research Institute for the Behavioral and Social Sciences.
- Joshi, A. (2016). Comparison between scopus and isi web of science. Journal Global Values, 7(1), 1–11.
- Kaasinen, E., Anttila, A. H., Heikkilä, P., Laarni, J., Koskinen, H., & Väätänen, A. (2022). Smooth and resilient human–machine teamwork as an industry 5.0 design challenge. Sustainability, 14(5), 2773. https://doi.org/10.3390/su14052773
- Kaasinen, E., Schmalfuß, F., Özturk, C., Aromaa, S., Boubekeur, M., Heilala, J., Heikkilä, P., Kuula, T., Liinasuo, M., Mach, S., Mehta, R., Petäjä, E., & Walter, T. (2020). Empowering and engaging industrial workers with operator 4.0 solutions. Computers & Industrial Engineering, 139, 105678. https://doi.org/10.1016/j.cie.2019.01.052
- Kapalo, K. A., Wisniewski, P. J., & LaViola, J. J. (2019). First in, left out: Current technological limitations from the perspective of fire engine companies. ISCRAM.
- Kavanagh, J. (2005). Stress and performance: A review of the literature and its applicability to the military. Rand.
- Keebler, J. R., Jentsch, F., & Hudson, I. (2011). Developing an effective combat identification training. Proceedings of the Human Factors and Ergonomics Society Annual Meeting (Vol. 55. pp. 1554–1558). SAGE Publications Sage CA, Los Angeles, CA.
- Kelley, A. M., Athy, J. R., King, M., Erickson, B., Chiaramonte, J., Vasbinder, M., & Thompson, A. (2011). Think before you shoot: the relationship between cognition and marksmanship (Report No. USAARL 2011-23). United States Aeromedical Research Laboratory - Warfighter Health Division. https://apps.dtic.mil/sti/citations/ADA553803
- Kim, S. (2016). Unmanned aerial vehicle (Uav) operators’ workload reduction: The effect of 3d audio on operators’ workload and performance during multi-aircraft control [ master’s thesis]. Air Force Institute of Technology.
- Knapik, J. J., Reynolds, K. L., & Harman, E. (2004). Soldier load carriage: Historical, physiological, biomechanical, and medical aspects. Military Medicine, 169(1), 45–56. https://doi.org/10.7205/MILMED.169.1.45
- Koch, W. (2022). Elements of an ethical ai demonstrator for responsibly designing defence systems. 2022 25th International Conference on Information Fusion (FUSION) (pp. 1–8). IEEE.
- Krebs, S. (2023). Above the law: Drones, aerial vision and the law of armed conflict–a socio-technical approach. International Review of the Red Cross, 105(924), 1690–1728. https://doi.org/10.1017/S1816383123000413
- Kuester, A., Schumacher, S., Niemeyer, H., Engel, S., Spies, J., Weiß, D., Muschalla, B., Burchert, S., Tamm, S., Weidmann, A., Bohn, J., Willmund, G., Rau, H., & Knaevelsrud, C. (2022). Attentional bias in German armed forces veterans with and without posttraumatic stress symptoms–an eye-tracking investigation and group comparison. Journal of Behavior Therapy and Experimental Psychiatry, 76, 101726. https://doi.org/10.1016/j.jbtep.2022.101726
- Lackey, S., Salcedo, J., Matthews, G., & Maxwell, D. B. (2014). Virtual world room clearing: A study in training effectiveness. Interservice/industry training, simulation, and education conference (Vol. 14045. pp. 1–11).
- Lafond, D., Vachon, F., Rousseau, R., Tremblay, S., Kaber, D. B., & Boy, G. (2010). A cognitive and holistic approach to developing metrics for decision support in command and control. In S. Gavriel & K. Waldemar (Eds.), Advances in Cognitive Ergonomics (1st ed., pp. 65–73). Boca Raton: CRC Press. https://doi.org/10.1201/EBK1439834916
- LaMonica, D. A., Drnec, K., & Miller, M. E. (2022). Employing mbse to assess and evaluate human teaming in military aviation command and control. 2022 IEEE 3rd International Conference on Human-Machine Systems (ICHMS) (pp. 1–7). IEEE.
- Landsbergis, P. A. (2018). Interventions to reduce job stress and improve work organization and worker health. In P. L. Schnall, M. Dobson, E. Rosskam, & R. Elling (Eds.), Unhealthy work: Causes, consequences, cures (1st ed., pp. 193–209). Routledge.
- Lavigne, V., & Gouin, D. (2014). Visual analytics for cyber security and intelligence. The Journal of Defense Modeling and Simulation: Applications, Methodology, Technology, 11(2), 175–199. https://doi.org/10.1177/1548512912464532
- Lazarus, R. S. (2020). Psychological stress in the workplace. In R. Crandall (Ed.), Occupational stress (2nd ed., pp. 3–14). CRC Press. https://doi.org/10.1201/9781003072430
- Lee, Y., Kozar, K. A., & Larsen, K. R. (2003). The technology acceptance model: Past, present, and future. Communications of the Association for Information Systems, 12(1), 50. https://doi.org/10.17705/1CAIS.01250
- Lenné, M. G., Hoggan, B. L., Fidock, J., Stuart, G., & Aidman, E. (2014). The impact of auditory task complexity on primary task performance in military land vehicle crew. Proceedings of the Human Factors and Ergonomics Society Annual Meeting (Vol. 58. pp. 2185–2189). SAGE Publications Sage CA, Los Angeles, CA.
- Leone, A., Rescio, G., Siciliano, P., Papetti, A., Brunzini, A., & Germani, M. (2020). Multi sensors platform for stress monitoring of workers in smart manufacturing context. 2020 IEEE International Instrumentation and Measurement Technology Conference (I2MTC) (pp. 1–5). IEEE.
- Lim, Y., Gardi, A., Sabatini, R., Ramasamy, S., Kistan, T., Ezer, N., Vince, J., & Bolia, R. (2018). Avionics human-machine interfaces and interactions for manned and unmanned aircraft. Progress in Aerospace Sciences, 102, 1–46. https://doi.org/10.1016/j.paerosci.2018.05.002
- Linder, S. M., Koop, M. M., Ozinga, S., Goldfarb, Z., & Alberts, J. L. (2019). A mobile device dual-task paradigm for the assessment of mtbi. Military Medicine, 184(Supplement_1), 174–180. https://doi.org/10.1093/milmed/usy334
- Lipp, N., Dużmańska-Misiarczyk, N., Strojny, A., & Strojny, P. (2021). Evoking emotions in virtual reality: Schema activation via a freeze-frame stimulus. Virtual Reality, 25(2), 279–292. https://doi.org/10.1007/s10055-020-00454-6
- Longo, F., Nicoletti, L., & Padovano, A. (2017). Smart operators in industry 4.0: A human-centered approach to enhance operators’ capabilities and competencies within the new smart factory context. Computers & Industrial Engineering, 113, 144–159. https://doi.org/10.1016/j.cie.2017.09.016
- Luthar, S. S., & Cicchetti, D. (2000). The construct of resilience: Implications for interventions and social policies. Development and Psychopathology, 12(4), 857–885. https://doi.org/10.1017/S0954579400004156
- Lyreskog, D. M., Zohny, H., Savulescu, J., & Singh, I. (2023). Merging minds: The conceptual and ethical impacts of emerging technologies for collective minds. Neuroethics, 16(1), 12. https://doi.org/10.1007/s12152-023-09516-3
- Machuca, J. P., Miller, M. E., & Colombi, J. M. (2012). A cognitive task analysis-based evaluation of remotely piloted aircraft situation awareness transfer mechanisms. 2012 IEEE International Multi-Disciplinary Conference on Cognitive Methods in Situation Awareness and Decision Support (pp. 179–182). IEEE.
- Madonna, M., Monica, L., Anastasi, S., & DiNardo, M. A. (2019). Evolution of cognitive demand in the human–machine interaction integrated with industry 4.0 technologies. WIT Transactions on the Built Environment, 189(1), 13–19. https://doi.org/10.2495/SAFE190021
- Malish, R. G. (2017). The importance of the study of cognitive performance enhancement for us national security. Aerospace Medicine and Human Performance, 88(8), 773–778. https://doi.org/10.3357/AMHP.4795.2017
- Mao, C. C., Tseng, Y. C., & Chen, C. H. (2018). Dynamic information visualization on cognitive ability for intelligence preparation of the battlefield. Proceedings of the 6th International Conference on Information and Education Technology (pp. 263–266).
- Martin, K., McLeod, E., Périard, J., Rattray, B., Keegan, R., & Pyne, D. B. (2019). The impact of environmental stress on cognitive performance: A systematic review. Human Factors, 61(8), 1205–1246. https://doi.org/10.1177/0018720819839817
- Martin, K., Périard, J., Rattray, B., & Pyne, D. B. (2020). Physiological factors which influence cognitive performance in military personnel. Human Factors, 62(1), 93–123. https://doi.org/10.1177/0018720819841757
- Mattsson, S., Fast-Berglund, Å., Li, D., & Thorvald, P. (2020). Forming a cognitive automation strategy for operator 4.0 in complex assembly. Computers & Industrial Engineering, 139, 105360. https://doi.org/10.1016/j.cie.2018.08.011
- McEwen, B. S., & Sapolsky, R. M. (1995). Stress and cognitive function. Current Opinion in Neurobiology, 5(2), 205–216. https://doi.org/10.1016/0959-4388(95)80028-X
- McFarlane, D. C. (2006). Engaging innate human cognitive capabilities to coordinate human interruption: The hail system. In C. Forsythe, M. L. Bernard, & E. G. Timothy (Eds.), Cognitive Systems: Human Cognitive Models in System Design, Part (1st ed., pp. 324). New York: Psychology Press. h ttps://d oi.org/0.4324/9781410617088
- McKendrick, R., Shaw, T., de Visser, E., Saqer, H., Kidwell, B., & Parasuraman, R. (2014). Team performance in networked supervisory control of unmanned air vehicles: Effects of automation, working memory, and communication content. Human Factors, 56(3), 463–475. https://doi.org/10.1177/0018720813496269
- McKinley, R. A., Bridges, N., Walters, C. M., & Nelson, J. (2012). Modulating the brain at work using noninvasive transcranial stimulation. Neuroimage: Reports, 59(1), 129–137. https://doi.org/10.1016/j.neuroimage.2011.07.075
- McKinley, R., Nelson, J., McIntire, L., Nelson, J., & Weisend, W. (2012). Improved skill learning: enhancing formation and retention of non-declarative memories with transcranial direct current stimulation (Report No. 1). New Orleans, LA: Society for Neuroscience.
- Mekjavic, I. B., Norheim, A. J., & Friedl, K. E. (2023). Human performance and medical treatment during cold weather operations–synthesis of a symposium. International Journal of Circumpolar Health, 82(1), 2246666. https://doi.org/10.1080/22423982.2023.2246666
- Merlo, J. L. (2018). Military operations: Humans not machines make the difference. In R. Steinberg, S. Kornguth, & M. D. Matthews (Eds.), Neurocognitive and physiological factors during high-tempo operations (pp. 195–207). London: CRC Press. https://doi.org/10.1201/9781315597850
- Metcalfe, J. S., Larkin, G. B., Johnson, T., Oie, K., Paul, V., & Davis, J. (2010, April 5-9). Experimentation and evaluation of threat detection and local area awareness using advanced computational technologies in a simulated military environment. In G. R. Gerhart, D. W. Gage, & C. M. Shoemaker (Eds.), SPIE Defense, Security, and Sensing, Orlando, Florida, United States. (Vol. 7692, pp. 72–83). SPIE. https://doi.org/10.1117/12.850516
- Metcalfe, J. S., Mikulski, T., & Dittman, S. (2011, April 25-29). Accounting for human neurocognitive function in the design and evaluation of 360 degree situational awareness display systems. In J. J. Guell, K. L. Bernier, J. T. Thomas, & D. D. Desjardins. SPIE Defense, Security, and Sensing, Orlando, Florida, United States. (Vol. 8042, pp. 112–125). SPIE. https://doi.org/10.1117/12.885051
- Militello, L. G., Sushereba, C. E., Branlat, M., Bean, R., & Finomore, V. (2015). Designing for military pararescue: Naturalistic decision-making perspective, methods, and frameworks. Journal of Occupational and Organizational Psychology, 88(2), 251–272. https://doi.org/10.1111/joop.12114
- Mitroff, S. R., Ericson, J. M., & Sharpe, B. (2018). Predicting airport screening officers’ visual search competency with a rapid assessment. Human Factors: The Journal of the Human Factors & Ergonomics Society, 60(2), 201–211. https://doi.org/10.1177/0018720817743886
- Moraveji, N., & Soesanto, C. (2012, May 5 - 10). Towards stress-less user interfaces: 10 design heuristics based on the psychophysiology of stress. CHI ‘12: CHI Conference on Human Factors in Computing Systems, Austin Texas USA, (pp. 1643–1648). CHI EA. https://doi.org/10.1145/2212776.2223686
- Napier, S., Best, C., Patton, D., & Hodges, G. (2016). Using an augmented training event to collect data for future modeling purposes. Foundations of Augmented Cognition: Neuroergonomics and Operational Neuroscience: 10th International Conference, AC 2016, Held as Part of HCI International 2016 (pp. 421–430). Springer, Toronto, ON, Canada, July 17-22, 2016, Proceedings, Part II 10.
- Nelson, J., McKinley, R. A., Phillips, C., McIntire, L., Goodyear, C., Kreiner, A., & Monforton, L. (2016). The effects of transcranial direct current stimulation (tdcs) on multitasking throughput capacity. Frontiers in Human Neuroscience, 10, 589. https://doi.org/10.3389/fnhum.2016.00589
- Nelson, J. M., Phillips, C. A., McKinley, R. A., McIntire, L. K., Goodyear, C., & Monforton, L. (2019). The effects of transcranial direct current stimulation (tdcs) on multitasking performance and oculometrics. Military Psychology, 31(3), 212–226. https://doi.org/10.1080/08995605.2019.1598217
- Nindl, B. C., Billing, D. C., Drain, J. R., Beckner, M. E., Greeves, J., Groeller, H., Teien, H. K., Marcora, S., Moffitt, A., Reilly, T., Taylor, N. A. S., Young, A. J., & Friedl, K. E. (2018). Perspectives on resilience for military readiness and preparedness: Report of an international military physiology roundtable. Journal of Science and Medicine in Sport, 21(11), 1116–1124. https://doi.org/10.1016/j.jsams.2018.05.005
- Nørgaard, K., & Linden Vørnle, M. (2021). Cyborgs, neuroweapons, and network command. Scandinavian Journal of Military Studies, 4(1), 94–107. https://doi.org/10.31374/sjms.86
- O’Brien, M. J. (2011). The future of humanity: Heidegger, personhood and technology. Comparative Philosophy: An International Journal of Constructive Engagement of Distinct Approaches Toward World Philosophy, 2(2), 62–62. https://doi.org/10.31979/2151-6014(2011).020207
- Ogden, P. M., Wollert, T. N., Butler, P., & Salcedo, J. N. (2015). Squad overmatch: Using virtual technology to enhance live training environments. Virtual, Augmented and Mixed Reality: 7th International Conference, VAMR 2015, Held as Part of HCI International 2015 (pp. 300–308). Springer, Los Angeles, CA, USA. Proceedings 7, August 2–7, 2015.
- O’Hara, R., Vojta, C., Henry, A., Caldwell, L., Wade, M., Swanton, S., Linderman, J. K., & Ordway, J. (2016). Effects of a new cooling technology on physical performance in us air force military personnel. Journal of Special Operations Medicine: A Peer Reviewed Journal for SOF Medical Professionals, 16(2), 57–61. https://doi.org/10.55460/67L0-EZBK
- O’malley, P. (2010). Resilient subjects: Uncertainty, warfare and liberalism. Economy and Society, 39(4), 488–509. https://doi.org/10.1080/03085147.2010.510681
- Özyurt, E., Döring, B., & Flemisch, F (2014). Evaluation and extension of the cognitive assistant system (cogas) for user-oriented support of air target identification. 2014 IEEE International Inter-Disciplinary Conference on Cognitive Methods in Situation Awareness and Decision Support (CogSIMA) (pp. 66–72). IEEE.
- Pacaux-Lemoine, M. P., Berdal, Q., Guérin, C., Rauffet, P., Chauvin, C., & Trentesaux, D. (2022). Designing human–system cooperation in industry 4.0 with cognitive work analysis: A first evaluation. Cognition, Technology & Work, 24(1), 93–111. https://doi.org/10.1007/s10111-021-00667-y
- Page M. J., Moher D., Bossuyt P. M., Boutron, I., Hoffmann, T. C., Mulrow, C. D., Shamseer, L., Tetzlaff, J. M., Akl, E. A., Brennan, S. E., Chou, R., Glanville, J., Grimshaw, J. M., Hróbjartsson, A., Lalu, M. M., Li, T., Loder, E. W., Mayo-Wilson, E. … Whiting, P. (2021). Prisma 2020 explanation and elaboration: Updated guidance and exemplars for reporting systematic reviews. BMJ: British Medical Journal, 372, n160. https://doi.org/10.1136/bmj.n160
- Pallavicini F., Argenton L., Toniazzi N., Aceti, L., & Mantovani, F. (2016). Virtual reality applications for stress management training in the military. Aerospace Medicine and Human Performance, 87(12), 1021–1030. https://doi.org/10.3357/AMHP.4596.2016
- Paraskevopoulos I., & Tsekleves E. (2014a). Solar soldier: Virtual reality simulations and guidelines for the integration of photovoltaic technology on the modern infantry soldier. Simulation and Modeling Methodologies, Technologies and Applications: International Conference, SIMULTECH 2012 (pp. 141–154). Springer, Rome, Italy. July 28–31, 2012, Revised Selected Papers.
- Paraskevopoulos I., & Tsekleves E. (2014b). Simulating the integration of photovoltaic technology on the modern infantry soldier using modelling and simulation: Scenarios and guidelines. The Journal of Defense Modeling and Simulation: Applications, Methodology, Technology, 11(2), 155–173. https://doi.org/10.1177/1548512912458194
- Parasuraman R., & McKinley, R. A., (2014). Using noninvasive brain stimulation to accelerate learning and enhance human performance. Human Factors: The Journal of the Human Factors & Ergonomics Society, 56(5), 816–824. https://doi.org/10.1177/0018720814538815
- Park, J. H., Baek I. J., Han S. J., & Moon Y. E., (2015). Saft: Study of sensor unit for fall prevention in blocked vision. Adjunct Proceedings of the 2015 ACM International Joint Conference on Pervasive and Ubiquitous Computing and Proceedings of the 2015 ACM International Symposium on Wearable Computers (pp. 177–180).
- Park S., Endsley T., & Padir T., (2018). Comparisons of adaptive automation conditions for single-operator multiple-agent control systems. 2018 IEEE International Symposium on Technologies for Homeland Security (HST) (pp. 1–6). IEEE.
- Parsons, T. D., Courtney, C. G., Arizmendi, B., & Dawson, M. (2011). Virtual reality stroop task for neurocognitive assessment. In J. D., Westwood, S. W., Westwood, L. Felländer-Tsai, R. S., Haluck, H. M., Hoffman, R. A., Robb, S. Senger, & K. G., Vosburgh (Eds.), Medicine meets virtual reality 18 [Studies in Health Technology and Informatics] (Vol. 163, pp. 433–439). IOS Press. https://doi.org/10.3233/978-1-60750-706-2-433
- Parsons, S., Kruijt, A. W., & Fox, E. (2016). A cognitive model of psychological resilience. Journal of Experimental Psychopathology, 7(3), 296–310. https://doi.org/10.5127/jep.053415
- Paul, S., (2023). A survey of technologies supporting design of a multimodal interactive robot for military communication. Journal of Defense Analytics and Logistics, 7(2), 156–193. https://doi.org/10.1108/JDAL-11-2022-0010
- Paulus M. P., Simmons A.N., Potterat E.G., Van Orden K. F., & Swain J. L., (2018). Systems neuroscience approaches to measure brain mechanisms underlying resilience—towards optimizing performance. In R. Steinberg, S. Kornguth, & M. D. Matthews (Eds.), Neurocognitive and physiological factors during high-tempo operations (1st ed., pp. 133–150). London: CRC Press.
- Peng, C., Zhen, X., & Huang, Y. (2023). A structured approach for resilience-oriented human performance assessment and prediction in offshore safety-critical operations. Ocean Engineering, 287, 115743. https://doi.org/10.1016/j.oceaneng.2023.115743
- Pomeroy D., (2013). The Impact of Stressors on Military Performance (Report No. DSTO-GD-0780). Fishermans Bend VIC 3207: Defence Science and Technology Organisation. https://apps.dtic.mil/sti/citations/ADA602524
- Powell, K. R., & Peterson, S. R., (2017). Coverage and quality: A comparison of web of science and scopus databases for reporting faculty nursing publication metrics. Nursing Outlook, 65(5), 572–578. https://doi.org/10.1016/j.outlook.2017.03.004
- Pulantara, I. W., Parmanto, B., & Germain, A. (2018). Development of a just-in-time adaptive mhealth intervention for insomnia: Usability study. JMIR Human Factors, 5(2), e8905. https://doi.org/10.2196/humanfactors.8905
- Purwandini Sutarto, A., Abdul Wahab, M. N., & Mat Zin, N. (2012). Resonant breathing biofeedback training for stress reduction among manufacturing operators. International Journal of Occupational Safety and Ergonomics, 18(4), 549–561. https://doi.org/10.1080/10803548.2012.11076959
- Puscas I. M., (2018). Military human enhancement. In W. Boothby (Ed.), New technologies and the law in war and peace (pp. 182–229). Cambridge University Press.
- Rademacher L., Kraft D., Eckart C., & Fiebach, C. J., (2023) Individual differences in resilience to stress are associated with affective flexibility. Psychological Research, 87(6), 1862–1879. https://doi.org/10.1007/s00426-022-01779-4
- Rankin A., Lundberg J., Woltjer R., Rollenhagen, C., & Hollnagel, E. (2014). Resilience in everyday operations: A framework for analyzing adaptations in high-risk work. Journal of Cognitive Engineering and Decision Making, 8(1), 78–97. https://doi.org/10.1177/1555343413498753
- Rao A. K., Chahal J.S., Chandra S, & Dutt V. (2020). Virtual-reality training under varying degrees of task difficulty in a complex search-and-shoot scenario. Intelligent Human Computer Interaction: 11th International Conference, IHCI 2019 (pp. 248–258). Springer, Allahabad, India, Proceedings 11, December 12–14, 2019.
- Redden E. S., Elliott L. R., Pettitt R. A., & Carstens, C. B., (2011). Scaling robotic systems for dismounted warfighters. Journal of Cognitive Engineering and Decision Making, 5(2), 156–185. https://doi.org/10.1177/1555343411409315
- Reddy R. (2021). Strategic considerations of industry 4.0 on electronic warfare using technology roadmaps. 2021 IEEE AFRICON (pp. 1–6). IEEE.
- Reiswig, J. (2010). Mendeley. Journal of the Medical Library Association: JMLA, 98(2), 193. https://doi.org/10.3163/1536-5050.98.2.021
- Reivich K. J., Seligman M. E., & McBride S. (2011). Master resilience training in the U.S. Army. American Psychologist, 66(1), 25. https://doi.org/10.1037/a0021897
- Rettinger M., & Rigoll G., (2022). Defuse the training of risky tasks: Collaborative training in xr. 2022 IEEE International Symposium on Mixed and Augmented Reality (ISMAR) (pp. 695–701). IEEE.
- Rice G. M., Snider D., Drollinger S., Greil, C., Bogni, F., Phillips, J., Raj, A., Marco, K., & Linnville, S. (2019). Dry-eeg manifestations of acute and insidious hypoxia during simulated flight. Aerospace Medicine and Human Performance, 90(2), 92–100. https://doi.org/10.3357/AMHP.5228.2019
- Richmond, M., Gary, M., Cao, T., Blumson, D., Kosowski, L., Hock, K., Elsawah, S, and Kempt, N. (2021). Operational effectiveness of future land combat teams: Model conceptualisation. In R. W. Vervoort, A. A. Voinov, J. P. Evans, & L. Marshall (Eds.), 24th International Congress on Modelling and Simulation, Sydney, NSW, Australia, (pp. 813–819). Modelling and Simulation Society of Australia and New Zealand Inc.
- Rizzo A., Parsons T.D., Lange B., Kenny, P., Buckwalter, J. G., Rothbaum, B., Difede, J., Frazier, J., Newman, B., Williams, J., & Reger, G. (2011). Virtual reality goes to war: A brief review of the future of military behavioral healthcare. Journal of Clinical Psychology in Medical Settings, 18(2), 176–187. https://doi.org/10.1007/s10880-011-9247-2
- Rizzo, A., Reger, G., Gahm, G., Difede, J., & Rothbaum, B. O. (2009). Virtual reality exposure therapy for combat-related PTSD. In P. Shiromani, T. Keane, J. E. LeDoux (Eds.), Post-Traumatic Stress Disorder: Basic Science and Clinical Practice (2009th ed. pp. 375–399). Springer Nature: Humana Press. https://doi.org/10.1007/978-1-60327-329-9
- Romero, D., & Stahre, J. (2021). Towards the resilient operator 5.0: The future of work in smart resilient manufacturing systems. Procedia CIRP, 104, 1089–1094. https://doi.org/10.1016/j.procir.2021.11.183
- Romero, D., Stahre, J., & Taisch, M. (2020). The operator 4.0: Towards socially sustainable factories of the future. Computers & Industrial Engineering, 139, 106128. https://doi.org/10.1016/j.cie.2019.106128
- Romero D., Stahre J., Wuest T., Noran O., Bernus P., Fast-Berglund Å., & Gorecky D. (2016). Towards an operator 4.0 typology: A human-centric perspective on the fourth industrial revolution technologies. Proceedings of the international conference on computers and industrial engineering (CIE46) (pp. 29–31), Tianjin, China.
- Ruppert T., Jaskó S., Holczinger T., & Abonyi, J. (2018). Enabling technologies for operator 4.0: A survey. Applied Sciences, 8(9), 1650. https://doi.org/10.3390/app8091650
- Ryu, I. S., & Jeong, S. J. (2018, July 2-5). A study on the weapon system software reliability prediction and estimation process at the software development phase. In O. Gervasi, B. Murgante, S. Misra, E. Stankova, C. M. Torre, A. M. A. C. Rocha, D. Taniar, B. O. Apduhan, E. Tarantino, & Y. Ryu (Eds.), Computational Science and Its Applications – ICCSA 2018, Melbourne, VIC, Australia. (pp. 205–217). Cham: Springer. https://doi.org/10.1007/978-3-319-95174-4
- Saikia M. J., (2023). K-means clustering machine learning approach reveals groups of homogeneous individuals with unique brain activation, task, and performance dynamics using fnirs. IEEE Transactions on Neural Systems and Rehabilitation Engineering.
- Salmon P. M., Stanton N. A., & Jenkins D. P., (2017). Distributed situation awareness: Theory, measurement and application to teamwork. CRC Press.
- Sample M., Aunos M., Blain-Moraes S., Bublitz, C., Chandler, J. A., Falk, T. H., Friedrich, O., Groetzinger, D., Jox, R J., Koegel, J., McFarland, D., Neufield, V., Rodriguez-Arias, D., Sattler, S., Vidal, F., Wolbring, G., Wolkenstein, A., & Racine, E. (2019). Brain–computer interfaces and personhood: Interdisciplinary deliberations on neural technology. Journal of Neural Engineering, 16(6), 063001. https://doi.org/10.1088/1741-2552/ab39cd
- Scherer M. R., Weightman M. M., Radomski M. V., Smith, L., Finkelstein, M., Cecchini, A., Heaton, K. J., & McCulloch, K. (2018). Measuring soldier performance during the patrol-exertion multitask: Preliminary validation of a postconcussive functional return-to-duty metric. Archives of Physical Medicine & Rehabilitation, 99(2), S79–S85. https://doi.org/10.1016/j.apmr.2017.04.012
- Schulte, A., and Meitinger C. (2016). Introducing cognitive and co-operative automation into uninhabited aerial vehicle guidance work systems. In F. Jentsch & M. Barnes (Eds.), Human-robot interactions in future military operations (Vol. 1, pp. 145–170). London: CRC Press. https://doi.org/10.4324/9781315587622
- Selye H., (1936). A syndrome produced by diverse nocuous agents. Nature, 138(3479), 32–32. https://doi.org/10.1038/138032a0
- Seoane F., Ferreira J., Alvarez L., Buendia, R., Ayllón, D., Llerena, C., & Gil-Pita, R. (2013). Sensorized garments and textrode-enabled measurement instrumentation for ambulatory assessment of the autonomic nervous system response in the ATREC project. Sensors, 13(7), 8997–9015. https://doi.org/10.3390/s130708997
- Singer, P. W., (2016). The ethics of killer applications: Why is it so hard to talk about morality when it comes to new military technology? Journal of Military Ethics, 9(4), 5–18. https://doi.org/10.1080/15027570.2010.536400
- Sottilare R., Hackett M., Pike W., & LaViola, J. (2017). Adaptive instruction for medical training in the psychomotor domain. The Journal of Defense Modeling and Simulation: Applications, Methodology, Technology, 14(4), 331–343. https://doi.org/10.1177/1548512916668680
- Sparrow, R. J., (2005). Defending deaf culture: The case of cochlear implants. Journal of Political Philosophy, 13(2), 135–152. https://doi.org/10.1111/j.1467-9760.2005.00217.x
- Staal, M. A., Bolton, A. E., & Yaroush, R. A. (2008). Cognitive performance and resilience to stress. In B. J. Lukey, & V. Tepe (Eds.), Biobehavioral Resilience to Stress (1st ed., Vol. 19, pp. 259–299). New York: Routledge. https://doi.org/10.1201/9781420071788
- Stahlschmidt S., & Stephen D. (2022). From indexation policies through citation networks to normalized citation impacts: Web of science, scopus, and dimensions as varying resonance chambers. Scientometrics, 127(5), 2413–2431. https://doi.org/10.1007/s11192-022-04309-6
- Stanley E. A, Schaldach J. M., Kiyonaga A., & Jha, A. P., (2011). Mindfulness-based mind fitness training: A case study of a high-stress predeployment military cohort. Cognitive and Behavioral Practice, 18(4), 566–576. https://doi.org/10.1016/j.cbpra.2010.08.002
- Steinberg, R. M., Matthews, M. D., & Kornguth, S. (2018). Closing remarks: Realization of existing capabilities in sustaining performance. In R. Steinberg, S. Kornguth, & M. D. Matthews (Eds.), Neurocognitive and physiological factors during high-tempo operations (Vol. 1, pp. 243–254). London: CRC Press. https://doi.org/10.1201/9781315597850
- Stikic, M., Berka, C., & Korszen, S. (2015). Neuroenhancement in tasks, roles, and occupations. In D. Waldman & P. Balthazard (Eds.), In Organizational neuroscience [Monographs in Leadership and Management] (Vol. 7, pp. 169–186). Emerald Group Publishing Limited. https://doi.org/10.1108/S1479-357120157
- Stokes, C. K., Lohani M., DeCostanza A. H., & Loh, E. (2023). Human–machine social systems: Test and validation via military use cases. In G. Fortino, D. Kaber, A. Nurberger, & D. Mendonca (Eds.), Handbook of Human-Machine Systems (1st ed., pp. 71–82). John Wiley & Sons, Ltd). https://doi.org/10.1002/9781119863663
- Strawser B. J., (2010). Moral predators: The duty to employ uninhabited aerial vehicles. Journal of Military Ethics, 9(4), 342–368. https://doi.org/10.1080/15027570.2010.536403
- Strawser B.J., (2013). Killing by remote control: The ethics of an unmanned military. Oxford University Press.
- Sutarto A. P., Wahab M. NA., & Zin N. M., (2013). Effect of biofeedback training on operator’s cognitive performance. Work, 44(2), 231–243. https://doi.org/10.3233/WOR-121499
- Taylor P., Bilgrien N., He Z, & Siegelmann H. T., (2015). Eyeframe: Real-time memory aid improves human multitasking via domain-general eye tracking procedures. Frontiers in ICT, 2, 17. https://doi.org/10.3389/fict.2015.00017
- Taylor M. K., Markham A. E., Reis J. P., Padilla, G. A., Potterat, E. G., Drummond, S.P.A., & Mujica-Parodi, L. R., (2008). Physical fitness influences stress reactions to extreme military training. Military Medicine, 173(8), 738–742. https://doi.org/10.7205/MILMED.173.8.738
- Taylor L., Watkins S. L., Marshall H., Dascombe, B. J., & Foster, J. (2016). The impact of different environmental conditions on cognitive function: A focused review. Frontiers in Physiology, 6, 372. https://doi.org/10.3389/fphys.2015.00372
- Teo W.P., Muthalib M., Yamin S., Hendy, A. M., Bramstedt, K., Kotsopoulos, E., Perrey, S., & Ayaz, H. (2016). Does a combination of virtual reality, neuromodulation and neuroimaging provide a comprehensive platform for neurorehabilitation?–a narrative review of the literature. Frontiers in Human Neuroscience, 10, 284. https://doi.org/10.3389/fnhum.2016.00284
- Thayer, J. F., Verkuil, B., Brosschotj, J. F., Kevin, K., West, A., Sterling, C., & Christie, I. C. (2010). Effects of the physical work environment on physiological measures of stress. European Journal of Preventive Cardiology, I7(4), 431–439. https://doi.org/10.1097/HJR.0b013e328336923a
- Thorvald P., Fast Berglund Å., & Romero D. (2021). The cognitive operator 4.0. 18th International Conference on Manufacturing Research, ICMR 2021, incorporating the 35th National Conference on Manufacturing Research (Vol. 15. pp. 3–8). IOS Press, University of Derby, Derby, UK. 7–10 September. 2021
- Tiwari T., Singh A. L., & Singh I. L., (2009). Task demand and workload: Effects on vigilance performance and stress. Journal of the Indian Academy of Applied Psychology, 35(2), 265–275.
- Trochowska, K., (2018). Cultural neuroscience and the military: Applications, perspectives, controversies. In C. Faucher (Ed.), Advances in Culturally-Aware Intelligent Systems and in Cross-Cultural Psychological Studies [Intelligent Systems Reference Library] (Vol. 134, pp. 283–310). Cham: Springer.
- Trumbo M. C., Matzen L. E., Coffman B. A., Hunter, M. A., Jones, A. P., Robinson, C.S.H., & Clark, V. P., (2016). Enhanced working memory performance via transcranial direct current stimulation: The possibility of near and far transfer. Neuropsychologia, 93, 85–96. https://doi.org/10.1016/j.neuropsychologia.2016.10.011
- van Beurden M., & Roijendijk L. (2019). Towards a mixed reality platform for applied cognitive load evaluation. Engineering Psychology and Cognitive Ergonomics: 16th International Conference, EPCE 2019, Held as Part of the 21st HCI International Conference, HCII 2019 (pp. 123–136). Springer, Orlando, FL, USA. Proceedings 21. July 26–31, 2019,
- van Weelden E., Alimardani M., Wiltshire T. J., & Louwerse M. M., (2021). Advancing the adoption of virtual reality and neurotechnology to improve flight training. 2021 IEEE 2nd International Conference on Human-Machine Systems (ICHMS) (pp. 1–4). IEEE.
- Vatsal V., & Hoffman G., (2017). Wearing your arm on your sleeve: Studying usage contexts for a wearable robotic forearm. 2017 26th IEEE International Symposium on Robot and Human Interactive Communication (RO-MAN) (pp. 974–980). IEEE.
- Vischer J. C., (2007). The effects of the physical environment on job performance: Towards a theoretical model of workspace stress. Stress and Health: Journal of the International Society for the Investigation of Stress, 23(3), 175–184. https://doi.org/10.1002/smi.1134
- Visser M., van Eck N. J., & Waltman L., (2021). Large-scale comparison of bibliographic data sources: Scopus, web of science, dimensions, crossref, and microsoft academic. Quantitative Science Studies, 2(1), 20–41. https://doi.org/10.1162/qss_a_00112
- Wallace P. J., (2023). The effects of heat and cold on cognitive function and endurance capacity [ master’s thesis]. Faculty of Applied Health Sciences, Brock University,
- Weidinger J., Schlauderer S., & Overhage S., (2019). Analyzing the potential of graphical building information for fire emergency responses: Findings from a controlled experiment. 14th International Conference on Wirtschaftsinformatik (pp. 1084–1098). Wirtschaftsinformatik, Siegen, Germany. February 24–27, 2019
- Weisberg, S. M., Badgio, D., & Chatterjee, A. (2018). Feel the way with a vibrotactile compass: Does a navigational aid aid navigation? Journal of Experimental Psychology: Learning, Memory, and Cognition, 44(5), 667. https://doi.org/10.1037/xlm0000472
- White, T. L., Krausman, A. S., & Haas, E. C. (2018). Tactile displays in army operational environments. In J. Martin, L. Allender, P. Savage-Knepshield, & J. Lockett (Eds.), In Designing soldier systems: Current Issues in Human Factors (1st ed., pp. 97–116). London: CRC Press. https://doi.org/10.1097/10.1201/9781315576756
- WHO. (2020). Occupational health: Stress at the workplace. https://www.who.int/news-room/questions-and-answers/item/ccupational-health-stress-at-the-workplace
- Wickens, C. D., (2002). Multiple resources and performance prediction. Theoretical issues in ergonomics science. Theoretical Issues in Ergonomics Science, 3(2), 159–177. https://doi.org/10.1080/14639220210123806
- Wilson G.F., & Russell C. A., (2007). Performance enhancement in an uninhabited air vehicle task using psychophysiologically determined adaptive aiding. Human Factors: The Journal of the Human Factors & Ergonomics Society, 49(6), 1005–1018. https://doi.org/10.1518/001872007X249875
- Xu X., Lu Y., Vogel-Heuser B., & Wang, L. (2021). Industry 4.0 and industry 5.0—inception, conception and perception. Journal of Manufacturing Systems, 61, 530–535. https://doi.org/10.1016/j.jmsy.2021.10.006
- Yao, Z. F., & Hsieh, S. (2019). Neurocognitive mechanism of human resilience: A conceptual framework and empirical review. International Journal of Environmental Research and Public Health, 16(24), 5123. https://doi.org/10.3390/ijerph16245123
- Zahabi, M., & Kaber, D. (2018). Effect of police mobile computer terminal interface design on officer driving distraction. Applied Ergonomics, 67, 26–38. https://doi.org/10.1016/j.apergo.2017.09.006
- Zahabi M., Nasr V, Abdul Razak AM, McCanless, L., Maredia, A., Patranella, B., Wozniak, D., & Shahini, F. (2022). Effect of variable priority training on police officer driving performance and workload. Ergonomics, 65(8), 1057–1070. https://doi.org/10.1080/00140139.2021.2013550
- Zhou T., Xia P., Zhu Q., & Du, J. (2023). Cognition-driven navigation assistive system for emergency indoor wayfinding (cogdna): Proof of concept and evidence. Safety Science, 162, 106100. https://doi.org/10.1016/j.ssci.2023.106100
- Zocco A., Zocco M. D., Greco A., Livatino S., & De Paolis L. T., (2015). Touchless interaction for command and control in military operations. Augmented and Virtual Reality: Second International Conference, AVR 2015 (pp. 432–445). Springer, Lecce, Italy, August 31-September 3, 2015, Proceedings 2.
- Zolotová I., Papcun P., Kajáti E., Miškuf, M., & Mocnej, J. (2020). Smart and cognitive solutions for operator 4.0: Laboratory h-cpps case studies. Computers & Industrial Engineering, 139, 105471. https://doi.org/10.1016/j.cie.2018.10.032
- Zueger R., Niederhauser M., Utzinger C., Annen, H., & Ehlert, U. (2023). Effects of resilience training on mental, emotional, and physical stress outcomes in military officer cadets. Military Psychology, 35(6), 566–576. https://doi.org/10.1080/08995605.2022.2139948