ABSTRACT
In contrast to most Indigenous people in Canada, Inuit appeared until recently to have been protected from type 2 diabetes (T2D) related to obesity. We assessed the associations of metabolites (amino acids, acylcarnitines) with adiposity and biomarkers of T2D in school-aged Inuit children of Nunavik (Canada). Concentrations of metabolite were measured in plasma samples from a cross-sectional analysis of 248 children (mean age = 10.8 years). We assessed associations of plasma metabolites with adiposity measures (BMI, skinfold thicknesses) and T2D markers (insulin, glucose, adiponectin). Plasma concentrations of valine and tyrosine were higher in obese and overweight children compared to those of normal weight children (P < 0.05). An increment of 1-SD in BMI (SD = 3.3 kg/m2) was statistically associated with an increment of 0.21 (95% CI: 0.08, 0.33) for valine, 0.15 (95% CI: 0.02, 0.27) for isoleucine and 0.17 (95% CI: 0.04, 0.29) for tyrosine. Insulin concentration increased with concentrations of all amino acids (P < 0.05) except methionine. None of the acylcarnitines measured were statistically significantly associated with adiposity or T2D biomarkers A signature of metabolites, particularly higher levels of branched-chain amino acids, might allow for early detection of T2D among school-aged Inuit children.
Introduction
Childhood adiposity carries a significant burden of chronic diseases [Citation1,Citation2], psychological disorders [Citation3] and health-care costs [Citation4]. The most prevalent approach of determining obesity – a crude index of adiposity – is to compare the body mass index (BMI) to a reference-standard that takes into accounts child age and sex [Citation5]. The Inuit population of Nunavik (Northern Quebec, Canada) has experienced a low prevalence of obesity until the 1970s, when transition towards a western-style diet and sedentarity started taking its toll. We recently reported that the International Obesity Task Force (IOTF) BMI reference-standard is the most specific for identifying obesity accompanied by higher insulin disturbances in school-aged Nunavik Inuit children [Citation6].
Because T2D has a strong adiposity-dependent component, authors have suggested the use of the term “diabesity” when referring to the common factors of diabetes and obesity [Citation7]. The prevention of T2D, for which obesity is the major contributor, requires a better understanding of the pathophysiological changes that could lead to earlier diagnosis and intervention. Tabák et al. [Citation8] reported that changes in current biomarkers of T2D (insulin-resistance, fasting and 2 h post-load plasma glucose) appear two to four years before its diagnosis. Data from the emerging field of metabolomics – the study of the metabolome, i.e. small molecule chemicals within a biological sample – indicate that circulating concentrations of certain metabolites, in particular amino acids (AA) and acylcarnitines (AC), allow for early detection of diet-induced linking obesity and T2D [Citation9,Citation10].
Metabolomic research has identified branched-chain AA (BCAA: valine, isoleucine, leucine), aromatic AA (AAA: phenylalanine and tyrosine), and short-chain AC (C3 and C5) as the most promising metabolites distinguishing individuals with insulin-resistance or T2D, independently of BMI [Citation9,Citation11,Citation12]. In addition, these metabolites allow differentiating between normal weight and obese individuals [Citation9] and predicting insulin-resistance improvement, regardless of the amount of weight lost [Citation13]. Increased plasma concentrations of BCAA and AAA are also predictive of T2D incidence [Citation14]. Studies among children observed that BCAA are elevated in obese subjects and that this increase is associated with insulin resistance in longitudinal analyses, independently of initial BMI [Citation15–20].
Alongside being the constituents of proteins, AA can serve as energy fuel and give rise to several metabolites; circulating AA levels vary according to different physiological states [Citation21] and at high concentrations are known to modulate insulin resistance [Citation9]. ACs are generated through fatty acid oxidation and AA catabolism that are used as complementary sources of energy in cardiac and skeletal muscle mitochondria [Citation22]. AC profiles can help identify mitochondrial dysfunction [Citation23], which is increasingly being linked to insulin resistance [Citation24].
In Canada, obesity and T2D prevalence are higher in non-Inuit Indigenous compared to non-Indigenous people [Citation25,Citation26]. The Nunavik Inuit Health Survey, Qanuippitaa? 2004, revealed high proportions of obesity (W = 31.8%, M = 25.8%) [Citation27,Citation28] higher than the rest of Canada (2004: W = 23.2%, M = 22.9%) [Citation29]. The prevalence of diabetes among Nunavimmiut (4.7%) [Citation28] is similar to that of the Canadian adult population (2004: 4.8%) [Citation30], despite the younger age structure of the Nunavik population. Among circumpolar Inuit populations, although a low prevalence was reported in studies conducted before the 1980s compared with western populations, results from studies conducted over the last two decades indicate that the prevalence of diabetes is rising [Citation31]. Until recently, no studies had assessed the metabolic profiles of Indigenous children. A recent study conducted in an American Indigenous community revealed that plasma concentrations of several AA are altered in adolescents with obesity and are associated with insulin sensitivity [Citation32]. The present study aims at assessing if Inuit children with either adiposity or insulin resistance can be discriminated using plasma levels of AA and AC previously associated with obesity-related T2D. We access data from the Nunavik Child Development Study (NCDS) to determine the associations between AA and AC in relation with adiposity measures and biomarkers of T2D in school-aged Inuit children.
Materials and methods
Ethics statement
Written informed consent was obtained from the mother of each participating child, and oral consent was obtained from the child. Consent and assent forms were approved by the Nunavik Nutrition and Health Committee and the research ethics review board of Laval and Wayne State Universities.
Study design and participants
The cross-sectional analysis of the NCDS study included 294 school-aged Inuit children from the 14 villages of Nunavik, a region north of the 55th parallel in Arctic Quebec (Canada), who were recruited between September 2005 and February 2010. The design and recruitment have been described in detail elsewhere [Citation33]. Briefly, inclusion criteria were age between 8.5 and 14.5 years, birth weight ≥2.5 kg, gestation duration ≥35 weeks, no major birth defects, and no major neurological or chronic health problems affecting growth.
Anthropometric parameters
Anthropometric parameters were measured by research nurses trained to use standard measurement procedures. Weight was quantified on a digital balance, and height was recorded using a stadiometer. Two measurements were taken for each parameter, and a third was obtained if a discrepancy was noted between the initial measurements for weight (>500 g) and height (>0.5 cm). Final growth parameter values were based on the average of the two closest measurements. BMI was calculated as the ratio of weight (kg) to squared height (m2). The body weight status (normal weight, overweight or obese) of each participant was determined using the IOTF BMI reference-standard [Citation34]. The IOTF system (widely used internationally) is based on data from six large, nationally-representative, cross-sectional surveys on child growth – in Brazil, Great Britain, Hong Kong, the Netherlands, Singapore, and the USA. The cut-off values (for age and sex) are extrapolations of adult BMI cut-off points for overweight (25 kg/m2) and obesity (30 kg/m2).
Triceps skinfold thickness (TST, right arm) and subscapular skinfold thickness (SST) were measured in mm with a skinfold calliper by a research nurse. TST was measured on relaxed right arm, at mid-distance between the hood and the tip of the elbow, and SST from the back at about 1 inch below the lower border of the shoulder blade. Two measurements were performed for each skinfold thickness parameter, and a third was obtained in case of a discrepancy >3 mm between the two initial measurements. The final values were the average of the two closest measurements.
Blood sampling
A non-fasting venous blood sample (20 mL) was collected from each child. Following centrifugation, the plasma was transferred in plastic tubes and kept frozen at −80°C until time of analysis. Samples were shipped on dry ice to the Centre de Toxicologie du Québec (CTQ) for AA and AC analysis and to the Centre de recherche de l’Institut universitaire de cardiologie et de pneumologie de Québec for T2D biomarkers measurements.
Laboratory analyses
Plasma concentrations of insulin and adiponectin were determined using Milliplex kits (Millipore, Billerica, MA) and a Luminex reader (Bio-Rad Lab, Hercules, CA). Glucose concentration was measured with the Amplex-Red Glucose assay kit accordingly to the manufacturer’s instructions (Life Technologies).
Plasma concentrations of AA [arginine (Arg), glutamic acid (Glu), isoleucine (Ile), leucine (Leu), methionine (Met), phenylalanine (Phe), tyrosine (Tyr), valine (Val)] and AC [carnitine (C0), acetyl- (C2), propionyl- (C3), butyryl- (C4), isobutyryl- (IsoC4), glutaryl- (C5-DC), hexanoyl- (C6) and octanoylcarnitine (C8)] were determined by isotope-dilution liquid chromatography/hybrid quadrupole-time-of-flight (QTof) mass spectrometry using the method described by Roy et al. [Citation35]. Inter-day coefficients of variation ranged from 5.2% to 10.9% for AA and 5.0% to 9.8% for AC. Accuracy ranged from −7.3% to 12.8% for amino acids as assessed against SRM 1950 certified values (National Institute of Standards and Technology, Gaithersburg, MD, USA). We substituted plasma metabolite concentrations below the limit of detection (LOD) by a value equal to half the LOD.
Statistical analysis
Characteristics of children according to IOTF weight status were compared using chi-square test for categorical variable (sex) and compared using analysis of variance (ANOVA) for continuous variables. Firstly, arithmetic means (±SEM) of metabolites concentrations according to IOTF weight status were compared using a one-way analysis of variance (ANCOVA) with Tukey’s post-test for continuous variables and adjusted for age (continuous) and sex. Tests for trend across IOTF weight status were assessed using the SAS software PROC GLM CONTRAST. Secondly, we performed adjusted linear regression analyses to assess the association of each metabolite (dependent variables) with different sets of independent variables: measurements of adiposity (BMI, triceps skinfold thickness and subscapular skinfold thickness) as well as biomarkers of obesity (adiponectin) and T2D (insulin, glucose). Age- and sex-adjusted standardised estimates (95% CI) of metabolite concentrations according to a 1-SD increase of each independent variable were plotted on a graph. In order to visually compare metabolite concentrations with independent variables, all plasma metabolite concentrations were standardised (mean = 0, SD = 1) and the standardised mean-difference with 95% confidence interval (CI) was calculated for each metabolite and plotted on a graph. All analyses were performed with SAS software version 9.1.3 (SAS Institute, Cary, NC). P values reported are 2-sided (P < 0.05).
Results
Samples for analysis
Of the 294 available participants, 4 had missing data on height and were excluded. Metabolite concentrations were quantified in plasma samples of 248 participants, due to missing or insufficient volume sample. Therefore, the present cross-sectional analysis comprised 248 children aged 8 to 14 years.
Characteristics of the study participants
Sample characteristics are summarised in . Of the 248 children, 75 (30.3%) were classified as either overweight (22.6%) or obese (7.7%) according to the IOTF criteria. Normal weight, overweight and obese children did not differ significantly in terms of sex, age, non-fasting plasma glucose and adiponectin concentrations. In contrast, height, weight, BMI, BMI z-score, triceps skinfold thickness, and subscapular skinfold thickness as well as non-fasting plasma insulin concentrations were significantly higher in children exhibiting obesity compared to those with overweight, who themselves had higher values than their normal weight peers (all ptrend-values < 0.001).
Table 1. Baseline characteristics of Inuit children according to their IOTF weight status
AA and AC concentrations according to body weight status
AA and AC concentrations according to IOTF BMI status are provided in . None of the AC measured were statistically significantly associated with IOTF BMI status. Among children with obesity and overweight, plasma valine (P = 0.01) and tyrosine (P = 0.03) concentrations were higher than those of their normal weight counterparts. Standardised mean (95% CIs) differences in plasma AA () and AC (supplemental ) concentrations were also calculated and presented for obese or overweight children and their normal weight counterparts. Standardised mean differences were statistically significant for valine (overweight vs. normal weight: 0.35, 95% CI = 0.05 to 0.65; obese vs. normal weight: 0.60, 95% CI = 0.12 to 1.07) and tyrosine (obese vs. normal weight: 0.54, 95% CI = 0.07 to 1.01). No other significant differences were observed between weight status categories.
Table 2. Adjusteda means (±SEM) of metabolite concentrations according to IOTF weight status*
AA and AC concentrations in relation to adiposity and metabolic markers
Plasma AC levels did not vary according to adiposity or T2D biomarkers (see supplemental figures). depicts the age- and sex-adjusted standardised estimates of plasma AA concentrations (95% CI) according to 1-SD increment in markers of adiposity. An increment of 1 SD in BMI (SD = 3.3 kg/m2) was statistically associated with an increment of 0.21 (95% CI: 0.08, 0.33) for Val, 0.15 (95% CI: 0.02, 0.27) for Ile and 0.17 (95% CI: 0.04, 0.29) for Tyr. Increments of 1 SD (SD = 5.8 mm) in triceps skinfold thickness and 1 SD (SD = 6.5 mm) in subscapular skinfold thickness were statistically associated with an increment for Val of 0.21 (95% CI: 0.08, 0.33) and 0.19 (95% CI: 0.07, 0.32), respectively.
Figure 2. Adjusted standardised estimatesa (95% CI) of amino acid concentrations per increment in adiposity measurements (BMI, subscapular skinfold thickness, Triceps skinfold thickness) among Inuit children
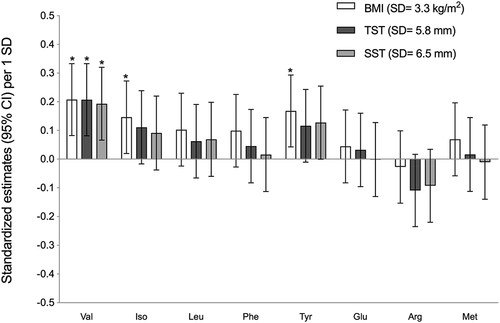
depicts the age- and sex-adjusted standardised estimates of plasma AA concentrations (95% CI) according to 1-SD increment in biomarkers. All AA except Met were significantly associated with non-fasting insulin concentration. An increment of 1 SD for insulin concentration (SD = 143 pmol/L) was accompanied by an increase of AA varying between 0.06 and 0.19. In contrast, an augmentation of 1 SD for insulin was associated with a decrease of −0.14 (95% CI: −0.28, −0.01) of octanoyl carnitine (C8). An increase of 1 SD (SD = 3.9 mmol/L) of plasma glucose was only significantly associated with an increase of 0.07 (95% CI: 0.03, 0.11) Glu, whereas adiponectin was not associated with any metabolite concentrations. All data related to crude metabolite concentrations are presented in Tables S1, S2 and S3 of Appendix (Supplementary materials).
Figure 3. Adjusted standardised estimatesa (95% CI) of amino acid concentrations per increment in biomarkers (glucose, insulin, adiponectin) among Inuit children. Biomarkers where collected in non-fasting state
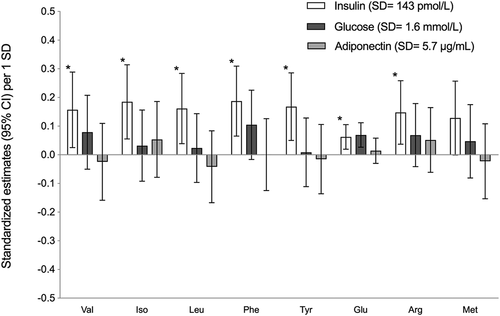
Discussion
We used of a metabolite profiling approach covering BCAA, AAA, and AC to gain insight on obesity-related T2D in Inuit children from Nunavik, an Indigenous population that have adapted to severe cold and a diet comprising predominantly marine foods. Our results suggest that a metabolite signature is associated with obesity and biomarkers of T2D in children from this population. Plasma concentrations of Val, Ile and Tyr increased with BMI, whereas an augmentation of non-fasting plasma insulin level was associated with higher concentrations of all AA except Met.
Some metabolic features noted in these school-aged children are similar to those observed in children experiencing obesity or overweight from other populations. In a cross-sectional study involving 69 US children aged 8 to 18 years, McCormack et al. [Citation15] reported that obesity was significantly associated with an elevation in fasting concentrations of circulating BCAA (Val, Ile, Leu). In their 18-month longitudinal analyses of a subsample of children (n = 17), the authors noted that baseline BCAA concentrations were associated with a higher insulin resistance, independently of the initial BMI. Using an untargeted metabolomic approach and a principal component analysis (PCA), Perng et al. [Citation19] observed among 262 US children (aged 6–10) that a factor dominated by BCAA and short-chain AC was associated with adiposity and elevated cardiometabolic biomarkers (HOMA-IR, insulin, hs-CRP, IL-6, and leptin), but was not linked to adiponectin levels [Citation19]. In a follow-up study in which the authors characterise metabolomic profiles of four overweight/obesity and metabolic risk phenotypes among 524 adolescents aged approximately 13 years, a principal component analysis revealed that a factor representing mainly BCAA (Val, C3 and C5 AC) was highest in participants belonging to the overweight/obese and high metabolic risk group [Citation36]. A serum amino acid signature including BCAA and AAA was found to be associated with obesity and serum triglycerides among 6–12 years Mexican children [Citation37]. In a longitudinal cohort followed by the same researchers, the amino acid signature was associated with serum triglycerides and with the risk of hypertriglyceridaemia after 2 years of follow-up. In the only study on this topic conducted among Indigenous children, Short et al. [Citation32] examined if differences in plasma concentrations of several amino acids and their metabolites were present between 11–17 year-old boys and girls with obesity (n = 58) and those of normal weight (n = 36). Compared with the normal weight group, participants in the obese group had significantly higher plasma levels of 17 AA including BCAA (+110% to 16%) and AAA (+115% to 32%). Concentrations of all AA were positively correlated with body fat and negatively correlated with insulin sensitivity [Citation32].
In contrast, Mihalik et al. [Citation38] observed no evidence of alteration in AA and AC metabolism in obese (BMI ≥ 95th for age and sex) or obese with T2DM adolescents compared to their normal-weight counterparts [Citation17]. Similarly, Michaliszyn et al. [Citation16] reported lower fasting plasma concentrations of several AA (Leu/Ile, Phe, Met and Arg) in obese adolescents with and without dysglycemia compared to normal-weight adolescents [Citation16]. They also observed that an increment in the glycaemic status was associated with a reduction of circulating AA levels. Unfortunately, because school-aged of children did not fast prior to blood collection in the present study, we could not categorise them according to their glycaemic status.
The reasons behind such inconsistency in the literature are unclear. Both Mihalik et al. [Citation17] and Michaliszyn et al. [Citation16] suggested that obese adolescents might exhibit different metabolic adaptations than adults, for whom the disease progression is spread over a longer period of time. They also argue that plasticity and growth during childhood and adolescence might have a major impact on metabolism (increased anabolism or decreased catabolism), which makes comparisons difficult with adult metabolomic profiles.
The differences observed between obese and normal weight school-aged of Inuit children concerning plasma concentrations of Val and Tyr might represent an early manifestation of the adverse metabolic consequences of nutritional transition [Citation39]. This Westernisation of diet (regimen rich in refined sugars, saturated animal fats, and engineered foods, but low in fruits, vegetables and fibre) [Citation40] has been proposed to explain the obesity/T2D epidemic in Indigenous populations [Citation41,Citation42] whose traditional diet was based on local food gathered from fishing, hunting, wild plant- and fruit-picking [Citation43–48]. An effect of diet through an alteration of gut microbiota is also likely. Ridaura et al. [Citation49] observed in obese mice that the gut exhibits a higher level of expression of microbial genes involved in the biosynthesis of essential AA (Phe, Lys, Val, Leu and Ile) and nonessential AA (Arg, Cys and Tyr), which contributes to the elevation of their circulating concentrations. Alternatively, the increase in circulating Val and Tyr levels in obese compared with normal weight children could be due to a decrease in the expression of the large neutral AA transporter (LAT1) in obese children [Citation50].
Of mention is the lack of an association between plasma AC levels and biomarkers of adiposity and glucose homeostasis in the present study. This is in contrast to the above-mentioned study of Perng et al. which found that the highest levels of a BCAA-related factor (Val, C3 and C5) were present in adolescents belonging to the overweight/obese and high metabolic risk group [Citation36]. Hence, although Val is increased in obese Inuit children, it is not accompanied by a similar increase in propionylcarnitine (C3), a product of BCAA catabolism, in particular valine and isoleucine catabolism [Citation9]. Higher levels of C3 (and C5) have been noted in metabolically unhealthy individuals, independently of body mass index [Citation11], suggesting that these AC may represent promising biomarkers for discriminating metabolic wellness among obese individuals. Hence, obesity among Inuit children may not exhibit the hallmarks of the unhealthy metabolic phenotype that may lead to obesity-related T2D in the long term. Using criteria developed for other populations, obesity has been on the rise in circumpolar Inuit populations over the past decades, especially among women [Citation51,Citation52]. While the impact of obesity on metabolic indicators has been lower than in other populations, better quality epidemiological data are required and community based-health interventions urgently needed to prevent future consequences of the emerging obesity epidemics [Citation52].
Our study has limitations and therefore results should thus be interpreted with caution. Firstly, because of limitations inherent to cross-sectional investigations, no causal relationship can be ascertained. Secondly, our participants were Inuit children aged between 8.5 and 14.5 years, and therefore generalisation of our results to other populations and other age groups is limited. Thirdly, our participants provided a non-fasting blood sample for laboratory analysis. This non-fasting condition is an obstacle to the use of the homeostasis model assessment (HOMA) to assess insulin resistance (HOMA-IR) and beta-cell function (HOMA-β) [Citation53] or associations of metabolites with other established cardiometabolic risk biomarkers. Furthermore, metabolite concentrations are influenced by dietary exposure [Citation54] and are therefore more variable then they would have been under fasting conditions. It is therefore remarkable that significant associations were noted between amino acids and adiposity as well as metabolic biomarkers, despite the non-fasting condition of participants. Fourthly, we did not collect information pertaining to the stage of puberty. Increased secretion of growth hormone (GH) and changes in protein metabolism during puberty can interfere with the dynamics of BCAA at the muscle cell level. As discussed above, in contrast to adults, associations between plasma concentrations of some metabolites and obesity in youth are not well established and studies showed controversial results. A systemic review indicated that several potential factors could interfere with these associations, including age and sex in relation to the hormonal and metabolic changes associated with puberty and rapid growth during this phase of life [Citation55].
Finally, we measured traditional markers of adiposity that may not be optimal in this population. Wells et al. observed that adiposity tends to be greater in populations inhabiting colder environments [Citation56]. Therefore, inclusion of surrogate markers of fatness localisation such as waist circumference or visceral fat might provide more insight regarding the associations between obesity and T2D, and metabolite concentrations. Of interest, a recent report identified a BCAA-based metabolic score that can predict steatosis grade in a group of young patients aged 9 to 19 years (n = 68) with severe obesity [Citation57]. In the future, this score may provide a feasible alternative to expensive imaging tests or biopsy to identify obese children and adolescents with severe steatosis, a key metabolic event in the pathogenic sequence leading to T2D.
In conclusion, adiposity or insulin-resistance among children can be discriminated by using metabolites, especially those related to amino acid metabolism. Data from the present study indicate that metabolites such as Val, Tyr and likely isoleucine might have the ability to metabolically discriminate excess adiposity among children. Although overweight/obesity increases the risk of T2D, levels of metabolites such as BCAA in individuals with excessive weight might be clinically useful to discern which children are at higher risk for T2D. However, do higher levels of these metabolites allow us to better predict and identify future risks of T2D for overweight/obese children? Unfortunately, cross-sectional studies cannot adequately and accurately answer this question. Longitudinal studies among children are warranted to investigate if increased plasma levels of these putative metabolites allow for early identification and better predict individuals at higher risk of developing T2D.
Abbreviations
AAA: aromatic amino acid; BCAA: branched-chain amino acid; IOTF: International Obesity Task Force; T2D: type 2 diabetes
Authorship
G.M. participated in the data collection; T.C.M.M., P.A., M.L. designed the analysis; T.C.M.M., C.R., P.Y.T., P.A., M.L contributed to the data analysis; T.C.M.M., P.A., M.L. conducted the analysis and interpretation, and wrote the first draft of the manuscript; T.C.M.M., C.R., P.Y.T., A.SJ., S.M., G.M., P.A., M.L. revised critically the manuscript for important intellectual content; P.A. and M.L. had primary responsibility for the manuscript final content. All authors read and approved the final version of the manuscript.
Ethical standards disclosure
This study was conducted according to the guidelines laid down in the Declaration of Helsinki and all procedures involving human subjects/patients were approved by the Nunavik Nutrition and Health Committee and the research ethics review board of Laval and Wayne State Universities. Written informed consent was obtained from the mother of each participating child, and oral consent was obtained from the child.
Supplemental Material
Download MS Word (26.3 MB)Acknowledgments
The authors are grateful to the Nunavik population, particularly the parents and children who participated in this study. We also thank the Nunavik Nutrition and Health Committee for their collaboration and input.
Disclosure statement
No potential conflict of interest was reported by the authors.
Supplementary material
Supplemental data for this article can be accessed https://doi.org/10.1080/22423982.2020.1858605 here.
Additional information
Funding
References
- Cali AM, Caprio S. Obesity in children and adolescents. J Clin Endocrinol Metab. 2008;93:S31–9.
- Craig LC, Love J, Ratcliffe B, et al. Overweight and cardiovascular risk factors in 4- to 18-year-olds. Obes Facts. 2008;1:237–242.
- Gouveia MJ, Frontini R, Canavarro MC, et al. Quality of life and psychological functioning in pediatric obesity: the role of body image dissatisfaction between girls and boys of different ages. Qual Life Res. 2014;23:2629–2638.
- Hampl SE, Carroll CA, Simon SD, et al. Resource utilization and expenditures for overweight and obese children. Arch Pediatr Adolesc Med. 2007;161:11–14.
- Katzmarzyk PT. Anthropometric indicators in relation to the gold standards. In: Bray GA, Bouchard C, editors. Handbook of obesity ─ Epidemiology, etiology, and physiopathology. Vol. 1. Third Ed ed. Boca Raton: Taylor & Francis Group; 2014. p. 37–46.
- Medehouenou TCM, Ayotte P, St-Jean A, et al. Overweight and obesity prevalence among school-aged Nunavik Inuit children according to three BMI classification systems. J Adolesc Health. 2015;57:31–36.
- Astrup A, Finer N. Redefining type 2 diabetes: ‘diabesity’ or ‘obesity dependent diabetes mellitus’? Obes Rev. 2000;1:57–59.
- Tabák AG, Jokela M, Akbaraly TN, et al. Trajectories of glycaemia, insulin sensitivity, and insulin secretion before diagnosis of type 2 diabetes: an analysis from the Whitehall II study. Lancet. 2009;373:2215–2221.
- Newgard CB, An J, Bain JR, et al. A branched-chain amino acid-related metabolic signature that differentiates obese and lean humans and contributes to insulin resistance. Cell Metab. 2009;9:311–326.
- Bene J, Márton M, Mohás M, et al. Similarities in serum acylcarnitine patterns in type 1 and type 2 diabetes mellitus and in metabolic syndrome. Ann Nutr Metab. 2013;62:80–85.
- Batch BC, Shah SH, Newgard CB, et al. Branched chain amino acids are novel biomarkers for discrimination of metabolic wellness. Metabolism. 2013;62:961–969.
- Huffman KM, Shah SH, Stevens RD, et al. Relationships between circulating metabolic intermediates and insulin action in overweight to obese, inactive men and women. Diabetes Care. 2009;32:1678–1683
- Shah SH, Crosslin DR, Haynes CS, et al. Branched-chain amino acid levels are associated with improvement in insulin resistance with weight loss. Diabetologia. 2012;55:321–330.
- Wang TJ, Larson MG, Vasan RS, et al. Metabolite profiles and the risk of developing diabetes. Nat Med. 2011;17:448–453.
- McCormack SE, Shaham O, McCarthy MA, et al. Circulating branched-chain amino acid concentrations are associated with obesity and future insulin resistance in children and adolescents. Pediatr Obes. 2013;8:52–61.
- Michaliszyn SF, Sjaarda LA, Mihalik SJ, et al. Metabolomic profiling of amino acids and beta-cell function relative to insulin sensitivity in youth. J Clin Endocrinol Metab. 2012;97:E2119–2124.
- Mihalik SJ, Michaliszyn SF, de Las Heras J, et al. Metabolomic profiling of fatty acid and amino acid metabolism in youth with obesity and type 2 diabetes: evidence for enhanced mitochondrial oxidation. Diabetes Care. 2012;35:605–611.
- Newbern D, Gumus Balikcioglu P, Balikcioglu M, et al. Sex differences in biomarkers associated with insulin resistance in obese adolescents: metabolomic profiling and principal components analysis. J Clin Endocrinol Metab. 2014;99:4730–4739.
- Perng W, Gillman MW, Fleisch AF, et al. Metabolomic profiles and childhood obesity. Obesity (Silver Spring). 2014;22:2570–2578.
- Reinehr T, Wolters B, Knop C, et al. Changes in the serum metabolite profile in obese children with weight loss. Eur J Nutr. 2014;54:173–181.
- Bröer S, Palacín M. The role of amino acid transporters in inherited and acquired diseases. Biochem J. 2011;436(2):193–211.
- Flanagan JL, Simmons PA, Vehige J, et al. Role of carnitine in disease. Nutr Metab (Lond). 2010;7:30.
- Reuter SE, Evans AM, Chace DH, et al. Determination of the reference range of endogenous plasma carnitines in healthy adults. Ann Clin Biochem. 2008;45(Pt 6):585–592.
- Fiorentino TV, Monroy A, Kamath S, et al. Pioglitazone corrects dysregulation of skeletal muscle mitochondrial proteins involved in ATP synthesis in type 2 diabetes. Metabolism. 2021 Jan;114:154416. doi:https://doi.org/10.1016/j.metabol.2020.154416. Epub 2020 Oct 31.
- Dyck R, Osgood N, Lin TH, et al. Epidemiology of diabetes mellitus among first nations and non-first nations adults. CMAJ. 2010;182:249–256.
- Green C, Blanchard JF, Young TK, et al. The epidemiology of diabetes in the Manitoba-registered first nation population: current patterns and comparative trends. Diabetes Care. 2003;26:1993–1998.
- Ngueta G, Laouan-Sidi EA, Lucas M. Does waist circumference uncorrelated with BMI add valuable information? J Epidemiol Community Health. 2014;68(9):849–855.
- Chateau-Degat ML, Dewailly E, Louchini R, et al. Cardiovascular burden and related risk factors among Nunavik (Quebec) Inuit: insights from baseline findings in the circumpolar Inuit health in transition cohort study. Can J Cardiol. 2010;26(6):190–196.
- Shields M, Tjepkema M. Trends in adult obesity. Health Rep. 2006;17(3):53–59.
- Public Health Agency of Canada. Report from the national diabetes surveillance system: diabetes in Canada, 2009. [cited Mar 2020]. Available from: http://www.phac-aspc.gc.ca/publicat/2009/ndssdic-snsddac-09/pdf/report-2009-eng.pdf.
- Tvermosegaard M, Dahl-Petersen IK, Nielsen NO, et al. Cardiovascular disease susceptibility and resistance in circumpolar inuit populations. Can J Cardiol. 2015;31:1116–1123.
- Short KR, Chadwick JQ, Teague AM, et al. Effect of obesity and exercise training on plasma amino acids and amino metabolites in American Indian adolescents. J Clin Endocrinol Metab. 2019 Aug 1;104(8):3249–3261.
- Pirkle CM, Lucas M, Dallaire R, et al. Food insecurity and nutritional biomarkers in relation to stature in Inuit children from Nunavik. Can J Public Health. 2014;105:e233–238.
- Cole TJ, Bellizzi MC, Flegal KM, et al. Establishing a standard definition for child overweight and obesity worldwide: international survey. BMJ. 2000;320:1240–1243.
- Roy C, Tremblay PY, Bienvenu JF, et al. Quantitative analysis of amino acids and acylcarnitines combined with untargeted metabolomics using ultra-high performance liquid chromatography and quadrupole time-of-flight mass spectrometry. J Chromatogr B Analyt Technol Biomed Life Sci. 2016;1027:40–49.
- Perng W, Rifas-Shiman SL, Sordillo J, et al. Metabolomic profiles of overweight/obesity phenotypes during adolescence: a cross-sectional study in project viva. Obesity (Silver Spring). 2020 Feb;28(2):379–387.
- Moran-Ramos S, Ocampo-Medina E, Gutierrez-Aguilar R, et al. An amino acid signature associated with obesity predicts 2-year risk of hypertriglyceridemia in school-age children. Sci Rep. 2017;7:5607.
- Mihalik SJ, Goodpaster BH, Kelley DE, et al. Increased levels of plasma acylcarnitines in obesity and type 2 diabetes and identification of a marker of glucolipotoxicity. Obesity (Silver Spring). 2010;18:1695–1700.
- Popkin BM. Contemporary nutritional transition: determinants of diet and its impact on body composition. Proc Nutr Soc. 2011;70:82–91.
- Popkin BM. Global nutrition dynamics: the world is shifting rapidly toward a diet linked with noncommunicable diseases. Am J Clin Nutr. 2006;84:289–298.
- Young TK. Are subarctic Indians undergoing the epidemiologic transition? Soc Sci Med. 1988;26:659–671.
- Popkin BM, Adair LS, Ng SW. Global nutrition transition and the pandemic of obesity in developing countries. Nutr Rev. 2012;70:3–21.
- Bernard L, Lavallee C, Gray-Donald K, et al. Overweight in Cree schoolchildren and adolescents associated with diet, low physical activity, and high television viewing. J Am Diet Assoc. 1995;95:800–802.
- Kuhnlein HV, Receveur O, Chan HM. Traditional food systems research with Canadian Indigenous Peoples. Int J Circumpolar Health. 2001;60:112–122.
- Blanchet C, Dewailly É, Chaumette P et al. Chapter 2. Diet profile of Circumpolar Inuit. In: Sustainable Food Security in the Arctic: State of Knowledge. Groupe d’études inuit et circumpolaires (GÉTIC), Université Laval, in cooperation with the Canadian Circumpolar Institute Press (CCI Press), University of Alberta. Canada. 2002:33–46.
- Kuhnlein HV, Receveur O, Soueida R, et al. Arctic indigenous peoples experience the nutrition transition with changing dietary patterns and obesity. J Nutr. 2004;134:1447–1453.
- Torrie J, Kishchuk BE, Webster A The evolution of health status and health determinants in the cree region (Eeyou Istchee): eastmain-1-A powerhouse and rupert diversion sectoral report. Cree Board of Health and Social Services of James Bay, 2005: p. 1–373.
- Kuhnlein HV, Receveur O, Soueida R, et al. Unique patterns of dietary adequacy in three cultures of Canadian Arctic indigenous peoples. Public Health Nutr. 2008;11:349–360.
- Ridaura VK, Faith JJ, Rey FE, et al. Gut microbiota from twins discordant for obesity modulate metabolism in mice. Science. 2013;341:1241214.
- Christensen HN. Role of amino acid transport and countertransport in nutrition and metabolism. Physiol Rev. 1990;70:43–77.
- Kellett S, Poirier P, Dewailly E, et al. Is severe obesity a cardiovascular health concern in the Inuit population? Am J Hum Biol. 2012;24(4):441–445.
- Young TK. Are the circumpolar Inuit becoming obese? Am J Hum Biol. 2007;19(2):181–189.
- Matthews DR, Hosker JP, Rudenski AS, et al. Homeostasis model assessment: insulin resistance and beta-cell function from fasting plasma glucose and insulin concentrations in man. Diabetologia. 1985;28:412–419.
- Thompson DK, Sloane R, Bain JR, et al. Daily variation of serum acylcarnitines and amino acids. Metabolomics. 2012;8:556–565.
- Frohnert BI, Rewers MJ. Metabolomics in childhood diabetes. Pediatr Diabetes. 2016;17(1):3–14.
- Wells JC. Ecogeographical associations between climate and human body composition: analyses based on anthropometry and skinfolds. Am J Phys Anthropol. 2012;147:169–186.
- Lischka J, Schanzer A, Hojreh A, et al. A branched-chain amino acid-based metabolic score can predict liver fat in children and adolescents with severe obesity. Pediatr Obes. 2020 Oct 14;e12739. doi: https://doi.org/10.1111/ijpo.12739.