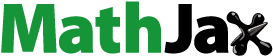
Abstract
Recently introduced regret-based choice models in transportation research have mainly adopted the assumption of identically and independently distributed unobserved regrets. The central argument underlying this paper is that this assumption is difficult to defend considering the fundamental nature of the concept of regret, which states that regret is generated through the comparison of choice alternatives on an attribute-by-attribute basis. To support this stance, we identify and diagnose specification errors in classic regret-based choice models, and provide an alternative specification. Results show that relaxing the assumption of identically and independently distributed error terms and applying the proposed error components Frechit structure leads to a substantial improvement in model performance of the regret-based models for the data used.
1. Introduction
Chorus, Arentze, and Timmermans (Citation2008) introduced regret-based choice models in transportation research as an alternative to expected/random utility models. The fundamental proposition of regret theory is that individuals minimize the amount of regret they (expect to) experience when choosing among choice alternatives. Regret is defined as the negative emotion that individuals experience in situations where a non-chosen alternative turns out to outperform the chosen one after all. Several regret functions have been suggested in the literature. The different specifications of the deterministic part of the regret function can be summarized as follows:
(1)
(1)
(2)
(2)
(3)
(3)
Equation (1) is the original regret formulation proposed by Chorus, Arentze, and Timmermans (Citation2008). Attribute-level regret is a function of objective attribute differences between chosen alternative i and non-chosen alternative j. Attribute-level regret is then summed across attributes. Finally, the total regret for chosen alternative i is defined to depend only on the best non-chosen alternative in the individual’s choice set, motivated by the principle of Irrelevance of Statewise Dominated Alternatives (ISDA) (Quiggin Citation1994).
To avoid the non-smooth likelihood function, caused by max the operators, Chorus (Citation2010) proposed an approximation of the original regret specification using a logarithmic function as shown in Equation (2). This specification has two issues: First, it over-represents regret (e.g. regret is ln (2) at the reference point) for smaller attribute differences (Rasouli and Timmermans Citation2014). Chorus (Citation2014) suggested subtracting ln (2) from the logarithmic function leading to no change in the choice probabilities and estimated parameters. However, the implication of this operation is that the model is no longer a regret-only model as the original model was. The model de facto introduces rejoice. Moreover, it automatically assumes that rejoice has an effect on the decision-making process for all attributes, converging to ln (2) for larger attribute-differences. Second, the model define regret against all non-chosen alternatives in the choice set, instead against the best non-chosen alternative. Theoretically, particularly in decision making under uncertainty, the ISDA property is an appealing property and for that reason alone, specification (1) may be preferable. From an applied perspective, however, the specification of the regret function in multi-alternative choice sets may be viewed as an empirical matter (Rasouli and Timmermans Citation2017, Citation2018; Hensher, Rose, and Green Citation2015; Chorus and Van Cranenburgh Citation2018), and may depend on the decision context in terms of complexity of the choice set, cognitive effort, distinctiveness of the choice alternatives, long-term consequences of the decision, etc.
The third specification of the regret function (Equation (3)) was used in Rasouli and Timmermans (Citation2014, Citation2017) to combine and keep the properties of the original specification of the regret function and the possibility of comparing regret against all other alternatives in the choice set. It allows a more systematic comparison of the effects of the change in functional form and the best only versus the all comparisons assumption.
Similar to utility-based choice models, regret-based choice models assume that total regret is stochastic, which is captured by adding an error term to the total regret function.
(4)
(4)
where
is a scale factor, which is inversely proportional to the variance of the error term. If it is normalized and assumed to be equal to one, then the variance of the error terms is
. Central to our argument then is that while Chorus, Arentze, and Timmermans (Citation2008) provided strong arguments for the specification of the regret function, the specification of the error terms received less motivation in their seminal publication. They simply assumed, following the derivation of the MNL model, that the (negatives of) the error terms are identically and independently Gumbel distributed (IID-Gumbel), so that
(5)
(5)
Later, Chorus (Citation2012) argued that measurement error results in negative correlations between random errors while omitted variables cause positive correlations. Although it seems unlikely that the two sources of correlation will completely cancel out each other, it also seems unlikely that one of the two sources will strongly dominate. Furthermore, he argued that the third source of random error (random behavior by decision-makers) generates i.i.d. errors and as such will dampen the effect of any correlation resulting from the combination of correlations caused by measurement error and omitted variables.
After their introduction, applications of random regret choice models have almost invariably adopted the assumption of independently and identically distributed error terms (e.g. Chorus, Arentze, and Timmermans Citation2009; De Moraes Ramos, Daamen, and Hoogendoorn Citation2011; Kaplan and Prato Citation2012; Beck et al. Citation2013; Chorus, Van Cranenburgh, and Dekker Citation2014; Hess, Beck, and Chorus Citation2014; Leong and Hensher Citation2015; Sharma, Hickman, and Nassir Citation2017; Li et al. Citation2018). Consequently, most applications involve Equation (5) in combination with Equation (2); a few studies explored taste variation (e.g. Boeri and Masiero Citation2014; Hensher, Greene, and Ho Citation2016). The behavioral validity of independently and identically distributed unobserved regrets has not found much attention in transportation research.
In two previous publications, we argued that all above regret specifications differ distinctly from the classical utility specifications with respect to the behaviorally inherent pairwise comparisons of alternatives. Such comparative judgment processes may cause non-IID errors in regret based choice models. We formally showed (Jang, Rasouli, and Timmermans Citation2017) that measurement error causes heteroskedastic unobserved regrets, and, therefore, the assumption of IID error terms is more difficult to defend in random regret choice models, compared to the classic random utility choice models. Simulation results of arbitrarily generated measurement error supported the formal results by showing that the consideration of the heteroskedasticity results in estimated parameters that are closer to the true values. In a sequel (Jang, Rasouli, and Timmermans Citation2020), we formally showed that omitted variables result in correlation between unobserved regrets due to the definition of regret which is based on the pairwise comparison of attribute levels. By arbitrarily omitting variables, we empirically showed that it is necessary to consider correlation between unobserved regrets to obtain robust parameters.
Since the previous studies were based on artificial settings, a question that still remains is how the implied process of comparative judgment in regret choice models can be better reflected in the specification of the error terms.Footnote1 To answer this question, in this study, we first suggest an alternative general expression for the specification of the error terms in regret choice models. Then, we compare the new specification against the commonly applied specification. We use two empirical data sets: The first data set is based on revealed preference survey and the second data set is based on a stated preference survey.
2. Error specification for regret-based choice models
Based on this diagnosis, we contend that regret-based choice models are more sensitive to sources of errors because compared to the (linear-additive) utility models. The assumption of identically distributed errors is less appropriate to represent unobserved regrets if measurement error occurs, while the assumption of independently distributed errors is less appropriate when significant variables are excluded from the analysis. Therefore, in this section, we discuss an alternative specification of the error terms in random regret choice models.
2.1. Allowing for non-identically distributed error terms – multiplicative error terms
To relax the assumption of independently distributed error terms, in this study, we specify multiplicative error terms instead of additive error terms. If we assume that unobserved utilities are independently distributed following the Weibull distribution, a closed form expression for the choice probabilities can be obtained (Castillo et al. Citation2008; Fosgerau and Bierlaire Citation2009), leading to the so-called multinomial Weibit (Weibull probability unit) modelFootnote2:
(6)
(6)
where
are multiplicative errors, assumed to be independently Weibull distributed, and the
is the shape parameter for the distribution
. The choice probability of the Weibit model was proved for the travel time (general cost) in the stochastic user equilibrium problem. In that case, utility (general cost) is always positive. In this study, we derive it for the utility. If
, the choice probability in the Weibit model can be determined by
(7)
(7)
For the choice probability derivation, we referred Castillo et al. (Citation2008) and Kitthamkesorn and Chen (Citation2013). In general, choice probability can be obtained from
(8)
(8)
where,
is the partial derivate of the joint survival function with respect to
. The cumulative distribution function of
is
(9)
(9)
This leads the survival function of
as
(10)
(10)
Then, the joint survival function can be derived, based on the independent assumption of
.
(11)
(11)
Therefore,
(12)
(12)
Considering Equation (7), the Equation (12) can be expressed as
(13)
(13)
The Equation (13) can be reformulated using Equation (8).
(14)
(14)
For more detail, see Castillo et al. (Citation2008) and Kitthamkesorn and Chen (Citation2013).
Alternatively, the choice probability with multiplicative error terms can be derived using a simple assumption of substitution (Fosgerau and Bierlaire Citation2009). They assumed multiplicative error terms following a certain distribution satisfying
. If
, the choice proabilities can be obtained as:
(15)
(15)
If we define
(16)
(16)
where
is an error terms identical and independently following Gumbel distribution, and
is a specific parameter for distribution
. Then, Equation (15) can be restated as:
(17)
(17)
Therefore, the choice probability can be obtained as same as in classical multinomial logit models.
(18)
(18)
Although they did not mention about specific distribution for the multiplicative error terms
, the choice probability in Equation (18) shows the same mathematical form as shown in Equation (14). In addition, we should note that
follows a certain distribution which is exponential of the nagative of Gumbull distribution based on Equation (16) which indicates the Weibull distribution (For more detail, see Mattsson, Weibull, and Lindberg Citation2014). Therefore, both approaches obtained choice probability in different ways, their results show the same equation
.
Whereas previous studies focused on derivation of choice probability when , in this study, we show the choice probability when
. Let assume that the reverse of the multiplicative error terms follow the Weibull distribution
. Since the inverse of the Weibull distribution is mathematically equivalent to the Frechet distribution (Khan, Pasha, and Pasha Citation2008), we can say that the multiplicative errors in this condition are independently distributed following the Frechet distribution. In this study, we call this structure the Frechit (Frechet probability unit) structure. Then, choice probabilities can be defined as:
(19)
(19)
As in same manner from Equation (8) to Equation (14), the choice probability can be derived as:
(20)
(20)
The choice probabilities also can be obtained from the logit structure:
(21)
(21)
If we define
(22)
(22)
Note that
is a certain distribution which is exponential of the Gumbull distribution. It is mathematically defined as Frechet distribution (Mattsson, Weibull, and Lindberg Citation2014)
.
Then the Equation (21) can be restated as:
(23)
(23)
Therefore,
(24)
(24)
Note that the choice probability function in Equation (24) is equivalent with Equation (20).
We apply the multiplicative error terms to regret models. Theoretically, the domain of regret should not include any non-negative values (Rasouli and Timmermans Citation2017), we introduce the Frecit structure in the regret models . While the Gumbel distribution may generate negative values for regret, the Frechet distribution, which is only distributed in positive domain, is a better candidate for representing error terms in regret models. Note that in the classical regret models with linear error terms (logit structure), the regret minimization is represented as ‘negaive of regret’ maximization
. In a similar way, to represent the regret minimization with the multiplicative error terms (frechit structure), we use the ‘reverse of regret’ maximization
. Then, as in the same way in Equations (19)–(24), the choice probabilities are consistently derived as:
(25)
(25)
To illustrate how the choice probabilities are differently obtained between logit and frechit structures, assume five alternatives in a choice set. Regrets equals R1=1, R2=2, R3=3, R4=4, R5=5. Therefore, alternative 1 is the best option and alternative 5 is the worst option in this choice set. Assuming the shape parameter is equal to 1, the probability of choosing the best alternative 1 respectively the worst alternative 5, according to the commonly used Logit structure, is respectively:
(26)
(26)
(27)
(27)
However, using the Frechit structure, assuming shape parameter
is one, the choice probabilities equal:
(28)
(28)
(29)
(29)
Therefore, for this example, the Frechit structure predicts a lower probability of the best alternative being chosen, and a higher choice probability for the worst alternative being choaen than the logit structure.
2.2. Allowing for non-independently distributed error terms – multiplicative error components structure
Earlier research regarding the relaxation of the assumption of independently distributed error terms focused on the extension of the logit structure based on generalized extreme value (GEV) distributed error terms (McFadden Citation1978). An example is the paired combinatorial logit model (e.g. Koppelman and Wen Citation2000).
More recently, in line with the development of mixed logit structures, the error components logit model was proposed and has been widely used to capture correlations between choice alternatives. Compared to the GEV-based models, the error components logit model has the advantage of capturing heterogeneous correlations. The error components have only been discussed for the logit structure (additive error terms). In this study, we discuss the error components for the proposed Frechit structure.
The following specification allows the inclusion of possible correlation between the error terms. Note that the error components require two additional parameters to represent correlations between pairs of alternatives. Since regret is defined as the summation of attribute-level regrets, the error components considering omitted variables are also expressed in additive form.
(30)
(30)
where
is an average effect of omitted variable(s) on the comparison of alternative i and j for individual n
, which is assumed to be log-normally distributed with density
,
refers collectively to the parameters of the distribution (generally mean and standard deviations of
). In this study, we apply this structure to multiplicative error terms. Then, the unconditional choice probability equals:
(31)
(31)
in Equation (31) represents the probability of individual n choosing alternative i which can be represented as
(32)
(32)
Since the choice probability
is not expressed in closed form anymore, simulation is required to obtain approximate values to estimate the parameters.
(33)
(33)
where R indicates the number of random draws from the log normal distribution. Then, a simulated log likelihood function is used to obtain optimized values. It can be expressed as
(34)
(34)
3. Empirical evidence
In this section, we will investigate how the proposed error structure performs empirically between regret and utility models. We use two data sets: a revealed preference data set regarding mode choice, expected to include measurement error and omitted variables, and a stated preference data set with labeled mode alternatives, expected to include omitted variables. Of primary interest is the question how differently the proposed error structure reproduces regret minimization behavior, compared to the logit structure. The results for the RRsum (Equation (2)) and utility models are presented in this section while the results for the RRmax and RRlog models can be found in Appendices 1. To empirically figure out the pure effect of the proposed error structure in regret minimizing behavior, we also conducted a similar analysis for the (linear-additive) utility-maximization model. To ensure a fair comparison between the Logit and Weibit structures, we fixed the scale parameter in the Logit structure and the shape parameter
in the Weibit structure as one. The validation test were conducted to support estimation results. Moreover, market shares are compared to better understand the effect of the proposed error structure.
To statistically analyze the difference in goodness-of-fit between (1) additive error terms and multiplicative error terms, (2) independent error terms and the error component structure, and (3) utility-based and regret-based models, we use two statistical criteria: Ben-Akiva and Swait’s test for non-nested choice models (Ben-Akiva and Swait Citation1986). Since the Ben-Akiva and Swait’s test is based on the Akaike Information Criterion (Akaike Citation1974) which is known that the index has an bias to pick the larger parameters model if the sample size gets big enough, we also use the Bayesian Information Criterion (Schwarz Citation1978).
3.1. Case study 1
3.1.1. Data description
The first case study is based on the 2009 MON data (Mobiliteit Onderzoek Nederlands – the Dutch National Travel Survey). This survey was administered among a stratified random sample of Dutch citizen to obtain information about that daily travel. The model estimations are based on a subset of 354 respondents, who reside in the Province of Noord Brabant (Figure ). The data includes two level-of-service variables (travel time and travel distance) for three transportation modes (car, bike, walk). Since the values of variable were derived from network models connecting centroids between two zones, they may include measurement error. In addition, the respondents may consider other variables as well to choose a mode, some relevant variables may be omitted. The travel time ranges from 1 to 134 min, while travel distance varies between 1 and 91 km. The variance of travel time is 56.48, which is larger than the variance of travel distance (50.89).
Figure 1. Location of Noord Brabant Province in the Netherlands. Source: https://nl.wikipedia.org/wiki/Noord-Brabant.
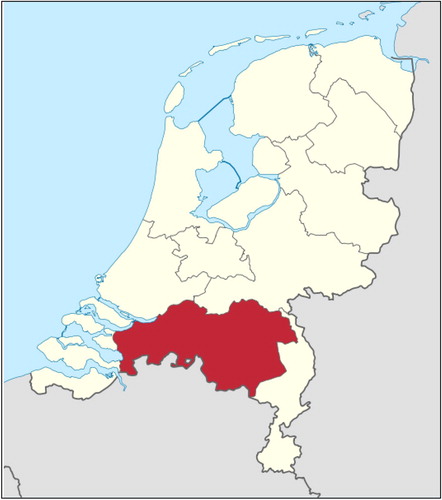
3.1.2. Estimation results
Table shows the estimation results for the different specifications of the error terms. First of all, utility model outperforms to the regret model in logit structure. In case of the logit structure, the probability that the regret-based model outperforms the utility-based model is zero (p ≤ (−6.373)≅0) and the utility-based model has a lower BIC value. This results have been interpreted as that respondents make a decision to maximize utility rather than minimize regret. Second, it demonstrates that multiplicative error terms (Weibit-utility, Frechit-regret) result in a higher predictive power than additive error terms (Logit) for both the utility-based and regret-based choice models, based on both Ben-Akiva and Swait’s test (BS test) and the BIC value. Further, the regret model shows better performance than the utility model with multiplicative error terms. In case of the Weibit (Frechit) structure, the BS test indicates that the probability of the regret-based model being superior to the utility-based model is not zero (p ≤ −1.836 ≅ 0.033), and the regret model has a lower BIC value. These results indicate that relaxing the assumption of identically distributed error terms significantly improves the performance of the regret-based choice model. The absolute value of parameters is commonly decreased in both utility and regret models. Note that whereas the variance of error is constant with the additive error terms (logit structure), depending on the scale factor in the Gumbell distribution, the variance of error with the multiplicative error terms (weibit structure) depends on the deterministic utility (or regret). That is, as increase of deterministic utility, the variance of error is also increasing (for more detail, see Castillo et al. Citation2008). Table also shows that the error components (EC) structure significantly improves the goodness-of-fit of the regret-based models with both the logit and Frechit structure. The BS test shows that the probability that the regret model with the logit structure outperforms the regret model with the Logit-EC structure is zero (p ≤ −7.756≅0). The probability that the regret model with the Frechit structure outperforms the regret model with the Frechit-EC structure is also zero (p ≤ −7.069≅0). In addition, the BIC values are much lower for the EC structure. On the other hand, the effect of the EC structure is unclear in utility-based models: results of the BS test show that the probability that the utility model with the logit structure outperforms the utility model with the Logit-EC structure. The probability that the utility model with the Weibit structure outperforms the utility model with the Weibit-EC structure cannot be calculated because the improvement is too small, compared to the increase in the number of parameters. The BIC values are even higher for the EC structure. When comparing utility-based and regret-based models with the error component structure and the multiplicative error terms, whereas the error component structure statistically improves the predictive power of the regret-based model, it is difficult to say that the predictive power of the utility-based model is improved: the BS test shows that the probability that the utility-based model without the error component structure outperforms the model with the error component structure is not zero (p ≤ −1.043 ≅ 0.852), and even the BIC value becomes higher for the error components structure, implying that the proposed error structure using additional parameters does not lead to improve model performance. Therefore, the error components structure that accounts for possible correlation between alternatives shows better performance for regret than for utility. The absolute value of parameters is commonly decreased by introducing EC structure. This is because the EC structure leads to additional error.
Table 1. Estimation results – first case study.
The results of the other regret specifications (RRmax and RRlog) are shown in Appendix 1. The proposed error components Frechit structure also shows improvement in model performance for all these specifications. However, the improvement is smaller for the RRmax specification. This is probably because the regret for the chosen alternative only depends on the best non-chosen alternative in the RRmax specification, and therefore the heteroskedasticity and correlation between unobserved regrets are smaller than in other specifications. The RRlog specification shows similar (or slightly higher) improvement in this data set, compared to the RRsum specification.
3.1.3. Market shares
To assess the degree of accuracy of the market shares predicted by the models, we compare observed and predicted market shares. The root mean square error (RMSE) is used as an index. A lower value of the RMSE indicates better accuracy. Table shows the results. First, while the weibit/frechit structure leads to improved predictive accuracy for both the utility and regret models the improvement is higher in the regret model. Second, the application of the EC structure in the utility model does not have a significant effect on market shares. With respect to the RMSE value, the difference in the RMSE by applying the EC structure is not different in logit structure and marginal in weibit structure. This implies that the introduction of the studied alternate error structures in the utility-maximizing model does not lead to a better prediction of market shares for this data set. On the other hand, the EC structure significantly improves the predictive ability of the regret model for both the logit and frechit structure. Therefore, at least for the current data set, allowing for heteroskedastic and correlated error terms to better reflect the regret generating process leads to improved results. The results of the other regret specifications are listed in Appendix 2. The proposed error components Frechit structure leads to better accuracy of the predicted market shares in the regret models, and the improvement is smaller for the RRmax specification, and higher for the RRlog specification.
Table 2. Market shares – first case study.
3.1.4. Validation
For the validation tests, the data were randomly split into 6 different subsets. The 5 subsets were used for estimation and a remained set was used for prediction. This procedure was repeated 6 times to use each subset for validation once. The comparison of performance of model in the validation dataset is shown in Table . Akin to the estimation results for the full data set, the validation results show that the proposed error structure is more effective to both utility and regret models than logit structure, and the effect is larger in regret models based on the adjusted rho-square. While the utility model outperforms regret model in logit structure, the dominant relationship is reversed by releasing the IID assumption in errors. Appendix 3 shows the validation results of other regret specifications (RRmax and RRlog). The proposed error structure improves model performance of both RRmax and RRlog specifications, however, as in the estimation, the improvement is smaller for the RRmax specification.
Table 3. Validation – first case study.
3.2. Case study 2
3.2.1. Data description
The data used in the second case study were collected in Beijing, China to analyze travel demand for electronic bikes (Figure ). An orthogonal fractional factorial choice set design was constructed to systematically vary the attributes within and between modes. The attribute levels are shown in Table . Respondents were asked to choose among alternatives labeled as E-bike, Car and Bus. Travel time and cost are only considered in the survey, therefore, some relevant variables may be omitted. The data relate to the stated choice of 297 participants who responded to 9 choice sets.
Figure 2. Location of Beijing in China. Source: https://en.wikipedia.org/wiki/File:China-Beijing.png.
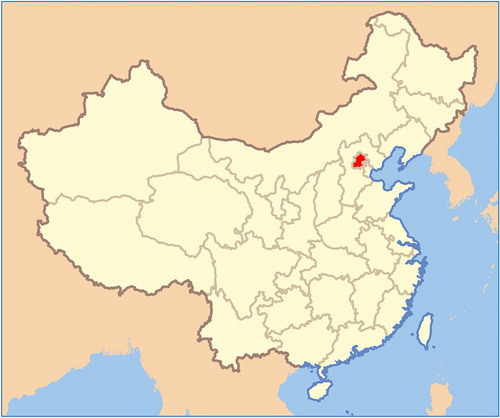
Table 4. Attribute levela – second case study.
3.2.2. Estimation results
The detailed estimation results are shown in Table . Although the EC structure for the utility based model was tested, the estimated parameters were all close to zero, and statistically highly insignificant, indicating no correlation between alternatives. Therefore, we do not report these in the table. On the other hand, in the regret model, while the correlation between error terms of E-bike and car shows value close to zero, the error components have higher values for E-bike and bus and are slightly lower in magnitude but higher in significance between car and bus (Table ). The predictive power of each model improves by replacing the assumption of additive error terms with multiplicative error terms, judged on the basis of the BS test and the BIC value. However, in contrast with the first case study, the improvement does not differ much between the utility-based and regret-based models. This may be explained by the smaller measurement error in stated choice experiments as respondents are informed about the values of the attributes. Moreover, introducing error components improves the goodness-of-fit of the regret-based models.
Table 5. Estimation results – second case study.
These results are similar for the other regret specifications (Appendix 1). Similar to the results of the first case study, the improvement for the RRmax specification is smaller than improvement for the RRsum and RRlog specifications.
3.2.3. Market shares
The results of the comparison between the observed and predicted market shares are reported in Table . It shows that whereas the utility model over-predicts the share of e-bike and car, and under-predicts the share of bus, the regret model over-predicts the share of e-bike and under-predicts the share of car and bus. The logit structure has the worst accuracy for both the utility and regret model. The application of the proposed error components Frechit structure shows the best accuracy for the regret model. Therefore, as in the first case study, the results suggest that the error terms are not identically and independently distributed. For this data set, the use of the logit structure in the regret model leads to a poor model performance in comparison to the proposed error structure.
Table 6. Market shares – second case study.
As for the other regret models, the accuracy improves with the proposed error components Frechit structure (Appendix 2). It is smaller for the RRmax specification, compared to the RRsum and RRlog specifications.
3.2.4. Validation
The validation results are reported in Table . Full data were randomly separated to 9 subsets. The 8 subsets were used to estimate the models, and remained a subset was used for prediction. This process is repeated 9 times by excluding each subset once. To the likeness of estimation results, the proposed error structure consistently improve model performance in regret models. This is common in other regret specifications (Appendix 3). On the other hand, the multiplicative error terms slightly improve model performance in utility models, and the error components are not effective.
Table 7. Validation – second case study.
4. Concluding and discussion
Recently, regret-based choice models have been introduced in the transportation research community as an alternative to expected/random utility models. The popularity of these models has rapidly increased as witnessed by an increasing number of applications in transportation research and other domains. The more fundamental literature has focused primarily on the mathematical specification of the deterministic part of the regret function. The specification of the error terms has been less subject of discussion, and almost all studies have followed the assumption of independently and identically Gumbel distributed error terms, used in the seminal article (Chorus, Arentze, and Timmermans Citation2008).
This study has pointed out that the IID assumption to describe unobserved regrets is more difficult to defend when the process that generates regret is taken into consideration. Therefore, we propose an error components Frechit specification to relax the commonly used assumptions about the error terms underlying random regret mimimization models. To assess this specification, we empirically tested the various specifications using two data sets. Empirical results show that the utility-based model has a better performance than the regret-based model when the logit structure is used. However, the use of the proposed error components Frechit structure leads to the regret-based model outperforming the utility-based model. Therefore, our conclusion is that if the empirical data are free from measurement error and all relevant variables are included in the regret function, currently used model specifications based on the logit structure may be valid. However, if not, the application to logit structure underrates the power of regret-based choice model.
Acknowledgements
The authors thank Qiong Tian (Beihan University) for providing the data used in the second case study.
Disclosure statement
No potential conflict of interest was reported by the author(s).
Notes
1 Since the originally proposed logit formulation of the random regret minimization model has been motivated on the assumption that measurement error and the error introduced by omitted variables cancel each other out (Chorus Citation2012), in this study we only investigate these sources of error. Taste variation also affects the error but we don’t see why it would in a fundamental sense be different between utility and regret.
2 The location parameter of the Weibull distribution is assumed zero.
References
- Akaike, H. 1974. “A New Look at the Statistical Model Identification.” IEEE Transactions on Automatic Control 19 (6): 716–723. doi: 10.1109/TAC.1974.1100705
- Beck, M. J., C. G. Chorus, J. M. Rose, and D. A. Hensher. 2013. “Vehicle Purchasing Behaviour of Individuals and Groups: Regret or Reward?.” Journal of Transport Economics and Policy 47: 475–492.
- Ben-Akiva, M., and J. Swait. 1986. “The Akaike Likelihood Ratio Index.” Transportation Science 20: 133–136. doi: 10.1287/trsc.20.2.133
- Boeri, M., and L. Masiero. 2014. “Regret Minimization and Utility Maximization in a Freight Transport Context: An Application from Two Stated Choice Experiments.” Transportmetrica A: Transport Science 10 (6): 548–560. doi: 10.1080/23249935.2013.809818
- Castillo, E., J. M. Menendez, P. Jimenez, and A. Rivas. 2008. “Closed Form Expressions for Choice Probabilities in the Weibull Case.” Transportation Research Part B: Methodological 42: 373–380. doi: 10.1016/j.trb.2007.08.002
- Chorus, C. G. 2010. “A New Model of Random Regret Minimization.” European Journal of Transport and Infrastructure Research 10 (2): 181–196.
- Chorus, C. G. 2012. Random Regret-based Discrete Choice Modeling: A Tutorial. Dordrecht: Springer.
- Chorus, C. G. 2014. “Benefit of Adding an Alternative to One’s Choice Set: A Regret Minimization Perspective.” Journal of Choice Modeling 13: 1–11. doi: 10.1016/j.jocm.2015.01.003
- Chorus, C. G., T. A. Arentze, and H. J. P. Timmermans. 2008. “A Random Regret-Minimization Model of Travel Choice.” Transportation Research Part B: Methodological 42 (1): 1–18. doi: 10.1016/j.trb.2007.05.004
- Chorus, C. G., T. A. Arentze, and H. J. P. Timmermans. 2009. “Spatial Choice: A Matter of Utility or Regret?” Environment and Planning B: Planning and Design 36 (3): 538–551. doi: 10.1068/b34092
- Chorus, C. G., and S. Van Cranenburgh. 2018. “Specification of Regret-Based Models of Choice Behaviour: Formal Analyses and Experimental Design Based Evidence—Commentary.” Transportation 45 (1): 247–256. doi: 10.1007/s11116-016-9739-x
- Chorus, C. G., S. Van Cranenburgh, and T. Dekker. 2014. “Random Regret Minimization for Consumer Choice Modeling: Assessment of Empirical Evidence.” Journal of Business Research 67 (11): 2428–2436. doi: 10.1016/j.jbusres.2014.02.010
- De Moraes Ramos, G., W. Daamen, and S. Hoogendoorn. 2011. “Expected Utility Theory, Prospect Theory, and Regret Theory Compared for Prediction of Route Choice Behavior.” Transportation Research Record: Journal of the Transportation Research Board 2230: 19–28. doi: 10.3141/2230-03
- Fosgerau, M., and M. Bierlaire. 2009. “Discrete Choice Models with Multiplicative Error Terms.” Transportation Research Part B: Methodological 43: 494–505. doi: 10.1016/j.trb.2008.10.004
- Hensher, D. A., W. H. Greene, and C. Q. Ho. 2016. “Random Regret Minimization and Random Utility Maximization in the Presence of Preference Heterogeneity: An Empirical Contrast.” Journal of Transportation Engineering 142: 1–17. doi: 10.1061/(ASCE)TE.1943-5436.0000827
- Hensher, D. A., J. M. Rose, and W. H. Green. 2015. Applied Choice Analysis. 2nd ed. Cambridge: Cambridge University Press.
- Hess, S., M. J. Beck, and C. G. Chorus. 2014. “Contrasts Between Utility Maximisation and Regret Minimisation in the Presence of Opt Out Alternatives.” Transportation Research Part A 66: 1–12.
- Jang, S., S. Rasouli, and H. J. P. Timmermans. 2017. “Bias in Random Regret Models Due to Measurement Error: Formal and Empirical Comparison with Random Utility Model.” Transportmetrica A: Transport Science 13 (5): 405–434. doi: 10.1080/23249935.2017.1285366
- Jang, S., S. Rasouli, and H. J. P. Timmermans. 2020. “Error By Omitted Variables in Regret-based Choice Models: Formal and Empirical Comparison with RUM Using Orthogonal Design Data.” Transportmetrica A 16: 892–909.
- Kaplan, S., and C. G. Prato. 2012. “The Application of the Random Regret Minimization Model to Drivers’ Choice of Crash Avoidance Maneuvers.” Transportation Research Part F: Traffic Psychology and Behaviour 15 (6): 699–709. doi: 10.1016/j.trf.2012.06.005
- Khan, M. S., G. R. Pasha, and A. H. Pasha. 2008. “Theoretical Analysis of Inverse Weibull Distribution.” WSEAS Transactions on Mathematics 7 (2): 30–38.
- Kitthamkesorn, S., and A. Chen. 2013. “A Path-Size Weibit Stochastic User Equilibrium Model.” Transportation Research Part B: Methodological 57: 378–397. doi: 10.1016/j.trb.2013.06.001
- Koppelman, F. S., and C. H. Wen. 2000. “The Paired Combinatorial Logit Model: Properties, Estimation and Application.” Transportation Research Part B 34: 75–89. doi: 10.1016/S0191-2615(99)00012-0
- Leong, W., and D. A. Hensher. 2015. “Contrasts of Relative Advantage Maximisation with Random Utility Maximisation and Regret Minimization.” Journal of Transport Economics and Policy 49: 167–186.
- Li, D., Y. Gao, R. Li, and W. Zhou. 2018. “Hybrid Random Regret Minimization and Random Utility Maximization in the Context of Schedule-Based Urban Rail Transit Assignment.” Journal of Advanced Transportation, to appear.
- Mattsson, L. G., J. W. Weibull, and P. O. Lindberg. 2014. “Extreme Values, Invariance and Choice Probabilities.” Transportation Research Part B 59: 81–95.
- McFadden, D. 1978. “Modeling the Choice of Residential Location.” In Spatial Interaction Theory and Planning Models, 105–142. Amsterdam: North-Holland.
- Quiggin, J. 1994. “Regret Theory with General Choice Sets.” Journal of Risk and Uncertainty 8 (2): 153–165. doi: 10.1007/BF01065370
- Rasouli, S., and H. J. P. Timmermans. 2014. “Regret-based Models of Consumer Choice of Shopping Center.” Paper presented at the 21th RARCS Conference, Bucharest, Romania.
- Rasouli, S., and H. J. P. Timmermans. 2017. “Specification of Regret-Based Models of Choice Behavior: Formal Analyses and Experimental Design Based Evidence.” Transportation 44 (6): 1555–1576. doi: 10.1007/s11116-016-9714-6
- Rasouli, S., and H. J. P. Timmermans. 2018. “Issues in the Specification of Regret-Only Choice Models: a Rejoinder to Chorus and Van Cranenburgh.” Transportation 45 (1): 257–263. doi: 10.1007/s11116-016-9740-4
- Schwarz, G. E. 1978. “Estimating the Dimension of a Model.” Annals of Statistics 6: 461–464.
- Sharma, B., M. Hickman, and N. Nassir. 2017. “Park-and-ride Lot Choice Model Using Random Utility Maximization and Random Regret Minimization” Transportation, to appear.