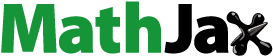
ABSTRACT
Speech perception involves prediction, but how is that prediction implemented? In cognitive models prediction has often been taken to imply that there is feedback of activation from lexical to pre-lexical processes as implemented in interactive-activation models (IAMs). We show that simple activation feedback does not actually improve speech recognition. However, other forms of feedback can be beneficial. In particular, feedback can enable the listener to adapt to changing input, and can potentially help the listener to recognise unusual input, or recognise speech in the presence of competing sounds. The common feature of these helpful forms of feedback is that they are all ways of optimising the performance of speech recognition using Bayesian inference. That is, listeners make predictions about speech because speech recognition is optimal in the sense captured in Bayesian models.
Perception involves prediction. In speech perception this claim is neither novel nor contentious; it has long been known that listeners are sensitive, for example, to the frequency of occurrence of individual words (Howes, Citation1957; Pollack, Rubenstein, & Decker, Citation1959). A word's frequency represents its prior probability and hence constitutes a prediction as to how likely the word is to appear in linguistic experience. Frequency-based predictions about words can even influence the identification of speech sounds: An ambiguous sound is more likely to be reported as forming a higher frequency word (e.g. more “best” responses to ambiguous steps on a “best-pest” continuum; Connine, Titone, & Wang, Citation1993). At the lexical level, listeners given sufficiently constraining context can accurately predict upcoming words in a sentence. The sentence fragment “The cat sat on the … ” will lead most listeners to predict that the next upcoming word is “mat”. Such predictions can also influence the listener's processing of following words. For example, if the fragment “She needs hot water for the … ” is completed by a word that is ambiguous between “bath” and “path”, listeners will be more likely to report the word as “bath” than after hearing “She liked to jog along the … ” (Miller, Green, & Schermer, Citation1984; see also Connine, Citation1987).
Listeners appear to be able to make predictions about the phonological form of spoken words on the basis not only of knowledge about frequency of occurrence (e.g. that “best” occurs more often than “pest”) and conceptual knowledge (e.g. about bathing and jogging), but also on the basis of a variety of other sources of knowledge. These range from knowledge about the vowel inventory of a speaker of a particular dialect (Brunellière & Soto-Faraco, Citation2013), through to lexical knowledge predicting the later sounds within words (Gagnepain, Henson, & Davis, Citation2012), to knowledge about the thematic constraints of verbs (Dahan & Tanenhaus, Citation2004). Form-based predictions about incoming speech are made on the basis of many and varying types of information, including syntax (Magnuson, Tanenhaus, & Aslin, Citation2008; Van Alphen & McQueen, Citation2001), prosody (Cutler, Citation1976), transitional probability (Pitt & McQueen, Citation1998), and visual cues (Van Wassenhove, Grant, & Poeppel, Citation2005). Listeners can also use many types of constraints – semantic, syntactic, and pragmatic – to anticipate upcoming words (e.g. Altmann & Kamide, Citation1999; Arai & Keller, Citation2013; Brouwer, Mitterer, & Huettig, Citation2013; Chambers, Tanenhaus, Eberhard, Filip, & Carlson, Citation2002; Kamide, Scheepers, & Altmann, Citation2003; Van Berkum, Brown, Zwitserlood, Kooijman, & Hagoort, Citation2005).
The critical question to ask, therefore, is not whether listeners make predictions, but exactly how and when those predictions influence perception. The answer to this question, we suggest, is that predictions in speech recognition are based on Bayesian inference (Norris & McQueen, Citation2008). We make the case that the reason listeners make predictions about speech follows from the assumption that they are Bayesian ideal observers, and hence also that they make predictions only to the extent that those predictions help speech perception.
In the literature on speech recognition this question has largely been addressed in the context of a debate contrasting models in which there is feedback of information from higher to lower levels (as instantiated inter alia in the TRACE model; McClelland & Elman, Citation1986), against models without this kind of feedback (e.g. the Bayesian Shortlist B model; Norris & McQueen, Citation2008). Simply put, in the former class of model predictions influence perception through activation feedback from higher processing levels to logically prior processing levels, but in the latter class of models no such reverse information flow is possible and yet predictions can still be made. In what follows, we argue that there are both theoretical and empirical arguments which suggest that perceptual predictions about speech do not require activation feedback; that is, that prediction does not imply this kind of feedback. We then discuss suggested frameworks for how Bayesian prediction might operate, including generative models (such as the analysis-by-synthesis model of Halle & Stevens, Citation1959, Citation1962) and predictive coding (e.g. Rao & Ballard, Citation1999). We conclude that, if insights from the debate about feedback are brought to bear, such frameworks have the potential to drive new developments in the cognitive modelling of speech perception, and to advance understanding of the way listeners make predictions about speech.
1. Definitions
1.1. What is a prediction?
Given our argument that speech perception is Bayesian, it should come as no surprise that our definition of prediction is Bayesian too. A prediction is a belief about the state of the world. That belief may be that grass is green, or that the next word in this sentence will be “cat”. But it need not be a prediction of a specific event or outcome. The prediction might also be a probability distribution over a range of possible outcomes, as in the case of word frequency. Other things being equal, we should predict that words will appear with probabilities given by their frequency of occurrence in the language.
(1)
(1)
But word frequency provides a very weak set of priors. Sentential context such as “He ate a … ” can provide much more constraint (though still not isolate a single word), while “The cat sat on the … ” may generate a very high prior for “mat”. In Bayesian terms, however, all that is happening in all these cases is that the priors are becoming more peaked around the most likely word or words; all of these cases are predictions. This Bayesian notion of prediction is thus more general than any more informal notion of prediction such as making a single forecast about a specific outcome (e.g. which word will come next). Importantly, predictions or beliefs should not be fixed; when the world changes, predictions should change too. Bayes' theorem, as applied to word recognition in Equation (1), shows how to do this. It provides a formal procedure for updating beliefs in the light of new evidence, and hence accommodating to a changing world. In Bayesian terms it tells us how to turn a prior probability (P(Word)) into a posterior probability (P(Word|Evidence)). p(EvidenceWord), in turn, is the likelihood of the presented evidence, if what is being presented is this word.
Bayes' theorem thus shows how to properly update a model of the world as new perceptual data arrive. Indeed, a case can be made that the primary function of perception is to construct the best possible model of the world. A model of the world necessarily contains implicit predictions. If, in your model of the world, walls are harder than people, this leads to the prediction that in a collision between a wall and a person, the person will probably come off second best. But if you enter a Japanese house where the walls are made of paper, this belief must be updated; the wall will probably suffer the most damage. Belief updating is yet more important when moving from houses with paper walls to houses with hard walls.
It is important, particularly in the context of this special issue, to distinguish this Bayesian definition of prediction, in which predictions about words during speech recognition are based on their prior probabilities, from other definitions. As already noted, Bayesian predictions need not concern specific outcomes: multiple words can become more probable at the same time. Also, on the Bayesian account, predictive processing is not limited to situations where anticipatory behaviour is observed. Predictions about a given word can have consequences for behaviour in advance of any perceptual evidence about that word (e.g. anticipatory fixations to the referent of a spoken word with a high prior probability; Altmann & Kamide, Citation1999), but predictions are also playing a role if priors influence behaviour only when the word is being heard (e.g. speeding up of lexical decisions to higher frequency words; Luce & Pisoni, Citation1998).
1.2. What is activation feedback?
Activation feedback can best be defined by reference to the TRACE model. TRACE was derived from the IAM of visual word recognition (Rumelhart & McClelland, Citation1982), and based on the same connectionist principles. In TRACE there are three layers of nodes representing phonetic features, phonemes, and words. Activation necessarily flows from the features to the phoneme layer and on to the word layer. However, nodes representing words also have top-down connections to the phoneme nodes. Activation of phoneme nodes activates corresponding word nodes and these in turn pass activation back down the network to the phoneme nodes. In this way, lexical context alters the activation of the nodes responsible for phoneme identification online (i.e. as the input is being processed). At the phoneme level, feedback from the lexical level thus causes activation to build up faster in phonemes in words than in phonemes in nonwords. To distinguish it from other forms of feedback (including feedback for learning, feedback for attentional control and feedback for binding; see Section 3.4), we refer to the feedback in TRACE as activation feedback.
Activation feedback provides a simple account of how lexical context can influence phoneme identification. For example, ambiguous phonemes tend to be identified so as to form words rather than non-words (the “Ganong effect”; Ganong, Citation1980). A phoneme that could be either /b/ or /p/ is reported as [b] in [?if] (beef-peef), but as [p] in [?is] (beace-peace). Activation feedback accounts for this finding by assuming that lexical feedback (from beef or peace) has activated phonemes consistent with actual words. Other demonstrations of lexical context effects in phonemic decision-making, for instance in phoneme monitoring (Rubin, Turvey, & van Gelder, Citation1976) and phonemic restoration (Samuel, Citation1981), can be accounted for similarly, as can Ganong effects for ambiguous sounds in word-final position (more reports of /s/ than of /ʃ/ after [kı], because kiss is a word but kish is not; McQueen, Citation1991a) and indeed effects of sentence context (such as in the bathing/jogging example above, from Miller et al., Citation1984).
2. Prediction and Bayesian inference
With these definitions in place, we resume our argument that prediction in speech recognition is based on Bayesian inference, and can be achieved without activation feedback.
Bayesian models, whether of speech perception (e.g. Feldman, Griffiths, & Morgan, Citation2009; Kleinschmidt & Jaeger, Citation2015), of spoken-word recognition (e.g. Shortlist B; Norris & McQueen, Citation2008) or of visual word recognition (e.g. the Bayesian Reader; Norris, Citation2006, Citation2009; Norris & Kinoshita, Citation2012) are examples of ideal observer models (see Geisler & Kersten, Citation2002, or Geisler, Citation2003). Such models describe how to make optimal decisions given limited data. Much of the time, after all, our senses receive quite ambiguous data. Given some stored set of stimulus categories (e.g. phonemes, words, or letters) and some such noisy stimulus, the best any perceptual system can possibly then do is to match the input against the stored category representations and select the category that provides the best fit; that is, the category with the highest posterior probability given the input.
Consider the case of visual word recognition operating on a fixed set of letters of known form. (As will be discussed in Section 4.1, the assumption of a fixed set of items is crucial.) Assume for the moment that all letters are equally common. Faced with some degraded representation of a letter, the best a perceptual system can possibly do is to match the input against the stored letter representations and select the letter that matches best (a formal derivation of this can be found in Appendix A of Pelli, Burns, Farell, & Moore-Page, Citation2006). But what if the letters form words, and those words appear with different frequencies? The words plus their frequencies constitute a prediction as to which letters are expected. The optimal decision procedure is given by a Bayesian ideal observer (again see Pelli et al., Citation2006, for derivation). This procedure takes words and their probabilities into account, but does not need to involve activation feedback from a lexical level of representation to some earlier level of letter analysis.
For phonetic categorisation, this optimal Bayesian procedure has been instantiated by Norris and McQueen (Citation2008) in their Merge B model. In Merge B, perceptual evidence is combined with lexical knowledge (probabilities or predictions) to compute the posterior probability of phonemes given the evidence. But lexical knowledge has no influence on the operation of the perceptual processes that deliver that evidence. According to Bayes' theorem (see Equation (1)), the likelihoods (i.e. the evidence) are kept separate from the priors; only the posterior probability is computed. Adding activation feedback could not possibly improve performance of the ideal observer; by definition, the observer is already ideal. (Indeed, as will be discussed later, activation feedback could well make performance worse; as it were, the priors could distort the likelihoods).
For spoken-word recognition, Shortlist B (Norris & McQueen, Citation2008) is likewise based on the assumption that listeners use Bayesian inference and hence act as ideal observers. The model is inherently predictive as it uses the prior probability of words, combined with their likelihood, to compute their posterior probability (Equation (1)). Shortlist B has no activation feedback, since that cannot improve on ideal listener performance. No model can outperform one which instantiates Bayes' theorem.
Shortlist B offers a ready explanation of frequency effects in spoken-word recognition. The lexical priors in the model reflect the frequency of occurrence of words, and simulations show that the model fits the relevant empirical data (Norris & McQueen, Citation2008). A striking feature of these simulations is that they require very few parameters: many aspects of the model's performance derive from the core assumption of Bayesian inference. Frequency biases in Shortlist B are just one form of predictive processing. What counts is not frequency itself, but priors. A more complete account would replace frequency with an estimate of the prior probability of words appearing in particular syntactic or semantic contexts. Frequency and context effects have the same explanation in a Bayesian model.
Discussions of prediction often take it as self-evident that prediction will improve perception. But, as Bayesian models capture, simply making predictions is not enough; those predictions have to be used in the right way, and that means drawing the appropriate inferences from them. Prediction should be Bayesian because in this way perception is indeed improved.
3. Prediction and activation feedback
In cognitive models of recent years, however, the dominant view has not been Bayesian, but has been, rather, that the benefits of prediction can be achieved by activation feedback. TRACE is the standard-bearer of this proposal. It is a flagship model, being both the first to have instantiated the dynamic inter-word competition that is essential for recognising words (due to the structure of vocabularies: McQueen, Cutler, Briscoe, & Norris, Citation1995), and also the only computational model of this type that has been implemented such that it can simulate a wide range of behavioural data on speech recognition. In TRACE, activation feedback is in fact the mechanism underlying all contextual effects; knowledge at a higher level of processing feeds back to affect activation of units at a lower level of processing. In IAMs, the output of one cognitive process feeds back to a logically prior cognitive process and, crucially, alters online the computations performed within that prior process. Thus the feedback connections in TRACE make it possible for contextual information to influence the perception of speech sounds.
Is this kind of information flow evidentially warranted? Note that this question in turn requires agreement on what precisely would count as evidence of such flow, and which levels are involved. The TRACE definition of feedback as connections from one level allowing information flow to a logically prior level excludes, for example, very general top-down effects such as those of attention (there appears to be unanimous agreement that attention can control the engagement of early perceptual processes; the amount of processing resources allocated to a task may be altered, with no necessary consequent alteration of the way those computations are performed). The distinction here resembles Gilbert and Sigman's (Citation2007) contrast of sensory versus behavioural context, where the latter encompasses attentional top-down control and processes involved in reconfiguring networks to perform different tasks.
At the other extreme, it is quite possible that, in a theory stated at what Marr (Citation1982) would term “computational” or “algorithmic” levels of analysis, there might be no need for feedback between different stages of processing, even though the requirement to implement the processing computations in neural tissue might best be served by exploiting recurrent connections between layers of neurons. These implementational details might be undetectable using behavioural measurement alone and hence would not be part of a purely psychological theory. Similarly, an algorithmic account with no feedback would not need to be altered in the light of evidence of the existence of recurrent neural connections, so long as those neurons were just part of the implementation of that algorithm. It is in fact known that there are extensive recurrent connections in the brain (see, e.g. Davis & Johnsrude, Citation2007, for review). These neurobiological facts cannot count as evidence of activation feedback, however, since it is not yet clear what those connections actually do.
3.1. Theoretical considerations
Though it is commonly assumed in the activation feedback literature that such feedback helps perception, especially with noisy or degraded input, in fact this assumption is unwarranted. As we have already discussed, an ideal observer can operate without activation feedback. By definition, adding feedback could not improve the performance of an already ideal observer. But adding feedback can actually make performance worse; it can generate hallucinations. In TRACE, if feedback flows down from a word to its constituent phonemes, it will boost the activation of those phonemes. Those phonemes, in turn, will boost the activation of the word even more, and so this cycle will continue. The problem here is that the activation generated by the input is being reused multiple times, and amplified each time. If feedback increases the activation of predicted input then that increased activation will make the predicted word even more likely. With too much activation feedback, the model will perceive only its own predictions. This will be detrimental when there is a mismatch between those predictions and the acoustic evidence, resulting in a hallucination.
Note, however, that this is not an inherent problem with the use of activation feedback. McClelland (Citation2013) has shown how a variant on an interactive-activation network can be constructed in which lower level nodes subtract out their own contribution to the feedback they receive from higher levels. This model retains activation feedback, but prevents the runaway feedback that can happen in the original model. The reason this network avoids the problem of runaway feedback is that it is constructed to implement Bayes' theorem. What this suggests, therefore, is that the way to get activation feedback to work appropriately is to ensure that it implements Bayesian prediction. Feedback performing Bayesian computations may be considered unobjectionable; however, it is incontrovertibly simpler to perform those same computations without feedback.
The original IAM had no internal noise. When noise is added to these models, feedback boosts both signal and noise equally. Again this means that there is no benefit in terms of increased sensitivity. Feedback is thus not a magic ingredient for improving perception. It is worth noting that the best current automatic speech recognition devices are feed-forward systems (Abdel-Hamid, Deng, & Yu, Citation2013; Hinton et al., Citation2012). Similarly, state-of-the-art image recognition uses feed-forward convolution neural nets (Zhou, Lapedriza, Xiao, Torralba, & Oliva, Citation2014).
Phoneme identification, considered as a form of multidimensional signal detection in which distributions of signal and noise overlap, also highlights the limitations on using feedback. An observer's task would be to judge whether a particular sample comes from the signal distribution or the noise distribution. Given that both distributions are fixed, the only freedom an observer has is in where to place the decision criterion. The observer could decide to maximise detection performance by placing the criterion exactly half way between the two distributions. If missing a signal were to be considered very costly, the criterion would be better placed nearer the noise distribution. In signal detection terms, observers could alter their bias. But now imagine that some high-level information indicates whether the input is signal or noise. If the high-level information is accurate in this, it could be fed back to alter placement of the criterion, and performance would become perfect as a result. The measured sensitivity of the whole system would accordingly increase. But of course the higher-level process has no need at all to feed any information back. It already knows the answer, so altering the processing information in accord with the answer achieves nothing.
The same principle holds even if the predictions of the higher level process are not wholly reliable. Low- and high-level information can be combined at the higher level stage (as in Merge B; Norris & McQueen, Citation2008), and nothing is gained by feeding that information back.
3.2. Behavioural data
Given the force of the theoretical argument that activation feedback cannot improve perception, it should come as no surprise that there is no convincing evidence for this kind of feedback. As Norris, McQueen, and Cutler (Citation2000) argued, almost all of the behavioural data on lexical involvement in phonemic decision-making available at the time of their review was not diagnostic with respect to activation feedback. Such data include demonstrations that lexical knowledge can influence phonemic decision-making across a range of tasks, including phonetic categorisation (Ganong, Citation1980) and phoneme monitoring (Rubin et al., Citation1976), and in the phoneme restoration illusion (Samuel, Citation1981).
The assumption that feedback would help perception led therefore to a substantial accumulation of evidence that was consistent with the idea of activation feedback, but did not prove to be diagnostic of it. All the cited findings are simply explained by purely feed-forward models such as Merge (Norris et al., Citation2000) and its Bayesian successor Merge B (Norris & McQueen, Citation2008). In Merge, phonemes can be identified on the basis of pre-lexical representations and read out of lexical-level representations. Decisions are based on a combination of those two sources of information. So although widely interpreted as evidence for activation feedback, results such as those of Ganong (Citation1980), Rubin et al. (Citation1976), and Samuel (Citation1981) warrant far more restricted inferences.
They suggest that a distinction needs to be made between process interaction and information interaction. The Ganong effect tells us that two different sources of information (lexical and pre-lexical) are combined in making a perceptual decision. It tells us about interaction of information. In itself, these data do not tell us whether lexical information feeds back down to influence pre-lexical processing. That is, it does not tell us whether there is interaction between processes.
3.2.1. So what would count as evidence for activation feedback?
The kind of evidence that would be diagnostic is evidence that feedback from the lexicon influences the internal workings of the pre-lexical processor. Elman and McClelland (Citation1988) attempted to find such evidence. They examined perceptual compensation for fricative-stop coarticulation (the tendency for listeners to perceive ambiguous stops between a [t] and a [k] as [k] after the fricative [s] but as [t] after the fricative [ʃ]; Mann & Repp, Citation1981). This process reflects a perceptual compensation for the acoustic consequences of coarticulating a stop after a fricative, and is generally considered to have a pre-lexical locus (for detailed discussion, see McQueen, Jesse, & Norris, Citation2009). Elman and McClelland showed that the compensation effect on ambiguous stops occurred after ambiguous fricatives placed in lexically disambiguating contexts (e.g. more [k] responses to christma[s/ʃ] [t/k]apes and more [t] responses to fooli[s/ʃ] [t/k]apes). They argued that this was evidence of lexical feedback: Fricatives that are filled in by the lexicon shape the pre-lexical compensation process in the same way as physically unambiguous fricatives.
This result appeared to contradict the feed-forward view. In particular, it cannot be explained in terms of the merging of lexical and pre-lexical information at a phonemic decision stage (Norris et al., Citation2000) because the lexicon provides information about the identity of the fricative, but not about the identity of the stop. At the time this was the most convincing evidence of activation feedback. In fact the logic underlying this experiment still represents a “gold standard” for identifying this kind of feedback. It goes beyond simply demonstrating that there are top-down or predictive effects, and instead looks for evidence that the lexical effect modulates the inner workings of pre-lexical processes.
The tables have since turned, however, such that the evidence from the compensation for coarticulation paradigm currently challenges the claim of activation feedback in speech recognition (McQueen et al., Citation2009). Many other factors turn out to contribute to Elman and McClelland's (Citation1988) finding. Transitional probabilities between fricatives and stops (Magnuson, McMurray, Tanenhaus, & Aslin, Citation2003; Pitt & McQueen, Citation1998), effects of word length and of perceptual grouping (Samuel & Pitt, Citation2003), the replicability of the effect (McQueen, Citation1991b; McQueen et al., Citation2009; Samuel & Pitt, Citation2003); and experiment-induced biases (McQueen, Citation2003; McQueen et al., Citation2009) all have their role to play in accounting for apparent demonstrations of lexical involvement in compensation for coarticulation. To date, there is no convincing evidence from this paradigm showing activation feedback (see McQueen et al., Citation2009, for a more detailed account).
Indeed, there is evidence from the paradigm that directly contradicts activation feedback. Lexical involvement in fricative decisions (i.e. deciding that the final ambiguous sound of christma[s/ʃ] is /s/ rather than /ʃ/) can be observed even in the absence of lexical involvement on the subsequent stops (e.g. no compensatory shift in /t/-/k/ decisions consistent with the lexical bias on the fricative) or even in the presence of effects quite opposite to those predicted by the lexical bias (McQueen et al., Citation2009; Pitt & McQueen, Citation1998). These dissociations between fricative and stop decisions challenge TRACE because the feedback-based account assumes that if the lexicon is influencing pre-lexical processing to cause the effect on fricatives, then an effect on the stops should necessarily follow (at least if the compensation for coarticulation mechanism was operating, as was the case in these studies). In contrast, the dissociations support feed-forward accounts in which the pre-lexical compensation for coarticulation process is impervious to lexical influence, but in which the lexicon can still influence decisions about the fricatives at a post-lexical decision stage, as in Merge (Norris et al., Citation2000).
3.3. Neuroimaging data
The available behavioural data are thus either neutral with respect to whether there is feedback or, in the case of the diagnostic evidence from the compensation for coarticulation paradigm, speaks against it. Neuroimaging data have also been used to address whether there is lexical-pre-lexical feedback in speech perception.
In an fMRI study (Myers & Blumstein, Citation2008), participants provided not only behavioural evidence of a Ganong effect (i.e. a shift of the /k/-/g/ boundary favouring the lexically consistent alternative in kiss-giss vs. kift-gift contexts) but also a reflection of this effect in brain activity (the BOLD signal in the superior temporal gyrus [STG], bilaterally, varied as a function of the ambiguity of the to-be-categorised stop and, critically, of the lexical contexts). Myers and Blumstein argue that since STG is responsible for perceptual processing, lexical modulation of the STG must reflect feedback.
This argument hinges on assumptions about the function of the STG, specifically that it is engaged in pre-lexical speech processing and that it is not engaged in lexical processing. While there is good evidence that STG is involved in mapping the speech signal onto the mental lexicon (Hickok & Poeppel, Citation2007) it is far from clear that there is a distinct division of labour in which the STG is engaged only in pre-lexical processing (DeWitt & Rauschecker, Citation2012; Price, Citation2012; Ueno, Saito, Rogers, & Lambon Ralph, Citation2011). Furthermore, even if it were the case that neural-level feedback from an area dedicated to lexical processing fed to one dedicated to pre-lexical processing, it would still be necessary to determine that feedback's computational function. The TRACE-style activation feedback discussed above is one possible function, but there are many other computations that could be being performed, including feedback for learning, feedback for binding or feedback for attentional control.
Another concern with the Myers and Blumstein (Citation2008) data are that BOLD signals reflect processes spread out over time (in this case over 1200 ms, delayed relative to stimulus offset), and hence may not directly reflect online processing. This concern does not apply to another neuroimaging study, in which Gow, Segawa, Ahlfors, and Lin (Citation2008) also looked at the Ganong effect, but used a combination of MEG, EEG and structural MRI. A lexical effect was again observed behaviourally (a shift of the /s/-/ʃ/ boundary in s/shandal vs. s/shampoo contexts). Time-varying activity in the supramarginal gyrus (SMG) was found to “Granger-cause” later time-varying activity in the posterior STG, 280–480 ms after stimulus onset. If the SMG is associated solely with word-form representation, and the STG is associated solely with pre-lexical processing, this causality effect would be evidence of feedback. But once again, this argument hinges on how clear it is what the SMG and STG do, and there is not yet consensus on this (compare, e.g. DeWitt & Rauschecker, Citation2012; Gow, Citation2012; Price, Citation2012; Ueno et al., Citation2011). Furthermore, these data again leave open the question of what computational function is being served by the connections between these brain regions. In short, neuroimaging data so far are not diagnostic about whether there is activation feedback.
3.4. Other forms of feedback
Our argument so far has been that prediction in speech recognition is not based on activation feedback. Activation feedback is not the best way to compute online predictions; predictions are of little help unless they can be updated in the light of new evidence. In other words, we need to learn from our experience. In fact, feedback has an important role to play in learning. For example, lexical feedback can be used in perceptual learning to retune pre-lexical representations, allowing listeners to adapt to different listening situations, such as when we encounter a speaker with a foreign or regional accent, or someone with an idiosyncratic way of speaking (Norris, McQueen, & Cutler, Citation2003).
There is now substantial evidence for lexically guided retuning of speech perception (for reviews; see, e.g. McQueen, Tyler, & Cutler, Citation2012; Samuel & Kraljic, Citation2009). When a listener hears an ambiguous fricative in a lexical context that biases its interpretation (e.g. [?], midway between [f] and [s], in a gira[?] context) listeners rapidly adapt their category boundaries so as to interpret further instances of that ambiguous fricative in a manner consistent with the earlier lexical information (in the example, they treat [?] as [f]). Furthermore, perceptual retuning transfers to the same sound appearing in new words, suggesting that lexical information has been fed back to retune pre-lexical processing and hence to help in the perception of new words spoken in the same way (Maye, Aslin, & Tanenhaus, Citation2008; McQueen, Cutler, & Norris, Citation2006; Sjerps & McQueen, Citation2010). That is, the listener can make better predictions as to how the phonemes produced by that speaker should be categorised. However, this form of lexical feedback is quite different from activation feedback. Lexical feedback for learning improves future perception, but does not alter immediate online processing. As Norris et al. (Citation2003) argue, feedback for learning can occur in a way that does not influence immediate online processing and therefore does not necessarily entail activation feedback. Comparing the evidence against activation feedback from the compensation for coarticulation paradigm with the evidence for lexically guided retuning underlines that feedback for learning is not based on activation feedback.
A further vital role for feedback is in binding different components of a representation into a coherent whole. In order to construct an integrated percept of the world we need to form a structured representation in which different features of the input are linked together in a coherent representation. Mooney pictures provide a well-known example: A two-tone image that looks to be nothing more than a set of black blobs on a white background will reveal itself as a picture of a Dalmatian if the viewer is first exposed to a full-tone picture of a Dalmatian. When we see such pictures we know which parts of the image correspond to the head, body, and legs; the percept now corresponds to a Dalmatian, and the features of the dog cohere into a whole. Similarly, when listening to speech, we do not just hear some speech and extract an independent representation of a sequence of words. We know which part of the speech signal corresponds to which word. A theory of perception should have a mechanism to bind together representations both within and between levels.
shows a toy connectionist network where the input is the phoneme sequence /haıdıər/. This input will activate hi, hide, dear, and ear. But which phonemes go with the word dear? When we look at we can see lines connecting dear to its constituent phonemes, so the answer seems very straightforward. It is obvious because we can trace the lines in both directions. The word dear, however, does not “know” which phonemes activated it because it cannot “see” back down the forward connections. It just receives activation. If there are no backward connections, then there is no way of forming an integrated percept of the word dear and its constituent phonemes. In order to form such a percept the phonemes need to be bound to the word, and this requires some form of bi-directional communication.
Critically, in contrast to activation feedback, in these non-activation cases the feedback is beneficial. These forms of feedback either are necessary for perception to be successful, or can make perception more efficient. Activation feedback, in contrast, has no such beneficial effects and can even be harmful. These beneficial forms of feedback could underlie purported neuroimaging demonstrations of top-down effects (Gow et al., Citation2008; Myers & Blumstein, Citation2008). More generally, it seems plausible that recurrent connections known to exist in the brain reflect these kinds of beneficial feedback (for attention, learning and binding) rather than online activation feedback.
3.5. Summary
Listeners could use activation feedback to realise predictions about speech sounds during spoken language processing. But instantiating predictions through this form of feedback is of no benefit to speech recognition. That is, predictions about upcoming words, and hence their component sounds, can be made at the lexical level or above, but feeding this information back to the pre-lexical level does not make for better interpretation of the speech signal. Furthermore, the available behavioural and neuroimaging data are either not diagnostic about this form of feedback, or (in the case of the compensation for coarticulation paradigm) contradict the predictions of the feedback-based model TRACE. Activation feedback therefore appears not to be the means by which listeners process speech or make predictions about spoken language.
While our focus has been on feedback between the lexical and pre-lexical levels about lexical contextual effects on the segments of spoken words, we believe that these arguments apply equally forcefully to other levels of speech processing and other types of contextual effect. The theoretical arguments about the lack of benefits of online feedback are not restricted to the lexical–pre-lexical interface (or indeed to spoken language processing). Furthermore, although it is logically possible that data could favour activation feedback in other domains while continuing not to do so in this specific domain, it is surely more parsimonious to assume that, in the absence of evidence to the contrary, conclusions will hold across domains. Importantly, we are not aware of diagnostic evidence for activation feedback (of the type where higher level contextual information modulates the operation of a lower level pre-decisional process) at other levels in speech recognition.
The absence of activation feedback in Shortlist B also means that the model is not challenged by the data on dissociations in lexical involvement in the compensation for coarticulation paradigm (McQueen et al., Citation2009; Pitt & McQueen, Citation1998). The model has feed-forward flow of information from the pre-lexical to the lexical level and, as in the Merge model (Norris et al., Citation2000), phonological decision-making is based on the merging of information from both the pre-lexical and the lexical levels. Simulations reported in Norris and McQueen (Citation2008) show that Merge B can account for the available data on lexical involvement in phonemic judgement tasks. Shortlist B is also consistent with the data that there is lexically guided retuning in speech perception (Norris & McQueen, Citation2008; Norris et al., Citation2003). Note also that it is compatible with the neurobiological evidence that there are recurrent neural connections; such connections could reflect the variety of functions discussed earlier: feedback for learning, for attentional control or for binding.
Given the theoretical arguments for Bayesian inference, it may therefore come as no surprise that proponents of interactive activation have recently begun to reformulate their theories so that interactive-activation networks with feedback connections can perform Bayesian inference (McClelland, Citation2013; McClelland, Mirman, Bolger, & Khaitan, Citation2014). McClelland (Citation2013) admits that:
the original IA model may have failed to carry out proper Bayesian computations on two counts: it distorted these computations due to its basic activation assumptions and it distorted them due to its failure at lower levels to take back out its own contribution to the signals it received from higher levels. (p. 23)
Revisions to the (stochastic; McClelland, Citation1991) IA model in which the pre-lexical nodes do indeed subtract out their own contributions to the feedback allow the model to correctly perform Bayesian inference (McClelland, Citation2013).
Since the development of this new class of models, the debate between IAM and Bayesian model appears to have been resolved. Both sides of this debate now agree that Bayesian optimality is as good as any model can do and hence, at the computational level, speech perception is Bayesian. Some differences do remain, however. In particular, the two frameworks still make different predictions about the compensation for coarticulation paradigm. In contrast to feed-forward models such as Shortlist B, a Bayesian IAM still predicts that lexical feedback should alter phoneme representations and trigger compensation for coarticulation. The data we have already reviewed suggest that this does not happen.
Furthermore, IAM s have the disadvantage that the activation assumptions do not guarantee that speech recognition will be Bayes-optimal. While McClelland (Citation2013) has shown that such models can be made to operate in a Bayesian fashion, this adds an assumption to the core set of interactive-activation assumptions. Interactive activation, with its activation feedback, can thus be viewed as the wrong place to start in model development. All we actually need is Bayes.
The most parsimonious model of speech perception, therefore, is a Bayesian one that does not include activation feedback. This also means that while the model needs mechanisms for learning, attentional control and binding, the way to implement those beneficial functions is not likely to be through activation feedback. As we have argued, for example, learning appears to occur in the absence of online effects. In the Bayesian model, therefore, there is a clear distinction between speech learning and speech recognition: learning occurs without affecting online recognition of the material that induces the learning. This distinction would be effectively lost in a model in which activation feedback somehow had to be switched off for recognition, but on for learning.
4. New approaches to modelling prediction
So far, we have argued that prediction in speech perception is Bayesian, and that it is not based on activation feedback. Several alternative frameworks, including generative models and predictive coding, have recently received considerable attention. Do these frameworks offer more promising accounts than interactive activation does of how Bayesian prediction might operate?
4.1. Generative models
Models such as TRACE and Shortlist A (Norris, Citation1994) can be characterised as performing template matching. The input is a set of symbols representing features, letters or phonemes, and words are represented as sequences of letters or phonemes. Recognition involves selecting the lexical representation that best matches the input. Selection is performed by competition between lexical nodes mediated by inhibitory connections between those nodes. The lexical representations cover the entire range of possible configurations of words in the input. The input need not match exactly to one of the lexical representations; a degree of generalisation to unseen patterns is possible. In these models the feed-forward connections can be seen as embodying an inverse model representing the transformation between sensory input and words in the lexicon.
It will not always be possible, however, to construct an inverse model. Under these circumstances a simple template matching process with a fixed set of templates will fail. This is particularly apparent in the case of visual object recognition where information is lost when the image of a 3D object is projected onto a 2D retina. Any 2D image may have been generated by infinitely many 3D objects. Consider the problem of perceiving the form of a 3D wireframe cube that might appear in any orientation or location. One could try to solve this problem by having many different templates. An alternative, though, is to have a forward generative model of a cube. Using basic geometry one can project a single canonical representation of a cube onto the image and rotate it and expand or contract it. If some combination of those transformations produces an image that matches the input, then the input might be a cube (see Pece, Citation2007, for an introduction to generative models in the context of vision).
A forward generative model thus uses top-down connections to model the sensory input that would be expected (generated) on the basis of some higher level representation. However, these top-down connections perform a very different function to those in an IAM. In TRACE, activation is fed back via top-down connections to simply boost the activation of nodes at an earlier level. In a generative model the forward model is compared to the input via top-down connections and the discrepancy between the two is then passed forward as a “prediction error”. The parameters of the generative model are adjusted so as to minimise prediction error. The process of homing in on the best match is effectively a search operation which minimises the prediction error between the internal hypothesis and the sensory input. Yuille (Citation1991) referred to this kind of processing as a “deformable template” and Mumford (Citation1992) used the term “flexible template”. A generative model will also incorporate prior knowledge – perhaps cubes are more likely to appear in some orientations than others. During learning it will be necessary to adapt the generative model of the input. Similarly, if the environment changes then the model will have to be updated to better “predict” the input. This is what happens in Kleinschmidt and Jaeger's (Citation2015) model of perceptual learning and adaptation.
A generative model requires flow of information from more abstract high-level object representations to lower level sensory process. This type of model and the template matching process represent opposite extremes. The contrast is between storing lots of representations (templates) in memory in the hope that the input will map fairly directly onto one of them, or storing a single representation and having to search through parameter space to see if there is a set of parameters that maps the input onto that single representation. In many cases the best solution will combine the two – a fast forward-matching process with an approximate inverse model that deals with the most probable inputs, which can be adapted with a slower generative process that shows better generalisation to previously unencountered input (c.f. Rao & Ballard, Citation1997, p. 747). The forward process will also provide an informed set of parameters to use as a starting point for a search.
This idea is consistent with a range of data from the visual object recognition literature. For example, Serre, Oliva, and Poggio (Citation2007) examined performance in a masked animal/non-animal classification task and compared their human data with simulations from a neurobiologically plausible model of visual perception. They suggested that a feed-forward system could perform the classification task when there was little visual clutter, but would require a contribution from recurrent connections as clutter increased. (Note that their model should not be taken as placing a limit on the performance of feed-forward processing.)
In speech perception, the most familiar forms of generative models are those in analysis-by-synthesis (Halle & Stevens, Citation1959, Citation1962) and motor theory (Liberman, Cooper, Shankweiler, & Studdert-Kennedy, Citation1967; for a review of motor theory, see Galantucci, Fowler, & Turvey, Citation2006). These models were not generally presented as Bayesian. The original motivation for analysis-by-synthesis was as a way to overcome the invariance problem; it is difficult to discover features of the speech signal that retain invariance over speakers or contexts. Proponents of analysis-by-synthesis suggested that it might be possible to synthesise the signal from articulatory features and adjust the parameters of that synthesis so as to achieve a match to the input. Poeppel and colleagues (Bever & Poeppel, Citation2010; Poeppel, Idsardi, & van Wassenhove, Citation2008; Poeppel & Monahan, Citation2010) have recently tried to revive interest in analysis-by-synthesis as a model of speech perception, and have made the case that this is a Bayesian model.
What situations in speech recognition might cause feed-forward systems to become inadequate and hence make the extra cost of employing structured generative models worthwhile? One situation concerns conditions analogous to occlusion in object recognition. For example, with auditory continuity illusions (Warren, Obusek, & Ackroff, Citation1972), listeners perceive a signal as being continuous even when it is interrupted by noise. This might be explained by assuming that listeners construct a model of both signal and noise which accounts for the input as being potentially produced by a continuous signal masked by noise.
One piece of empirical data suggesting a possible role of generative models in speech recognition comes from a study by Johnsrude et al. (Citation2013). They examined listeners' ability to identify words spoken by one speaker in the presence of a second stream of speech from a different speaker, a task in which success is presumed to be due in good part to the listener's ability to stream out the second speaker. They manipulated whether the second speaker was an unknown speaker or the participant's spouse. Listeners performed better when the speaker was their spouse. While there are many reasons why people may come to ignore what their spouse says, the result is what would be expected if listeners have a more exact model of the more familiar voice. The better the model, the easier it should be to use it to “explain away” the interfering voice and therefore to separate out the two streams. Regardless of whether this particular study can be taken as evidence for generative models, it does suggest an alternative approach to the study of feedback. Instead of trying to manipulate properties of the target stimulus itself, it might be possible to look at ways in which feedback processes might mitigate the effects of interfering stimuli.
The kind of prediction embodied in structured generative models provides an interesting contrast to the predictions implicit in, say, word frequency effects. The latter predictions are effectively precompiled as a result of experience, whereas a prediction about how a particular word form might be manifest under conditions that may never have been encountered before must be constructed online. However, while there are many plausible circumstances where a slower generative process might come to the rescue when a perceiver encounters unusual input, there is still little basis for assuming that such a process plays a significant role in online perception of normal speech.
4.2. Predictive coding
Friston and colleagues (Friston, Citation2003; Kilner, Friston, & Frith, Citation2007) refer to the use of generative models in the ways described above as “predictive coding”. Note that predictive coding is not a way of generating specific predictions as to what will come next. As Kilner et al. (Citation2007) have pointed out, “the prediction addressed in predictive coding is predicting the sensory effects from their cause” (p. 161). Predictive coding has been invoked as an explanation of both behavioural (Sohoglu, Peelle, Carlyon, & Davis, Citation2014) and neuroimaging data (Clos et al., Citation2014; Sohoglu, Peelle, Carlyon, & Davis, Citation2012). So far, however, these are data that can be considered to be consistent with predictive coding, rather than diagnostic of it.
Although recent discussions of predictive coding often emphasise the online perception role of generative models, an equally important part of the predictive coding framework is the learning of efficient codes. Since the seminal paper of Rao and Ballard (Citation1999), the concept of predictive coding has had a significant influence on the development of models of neural computation (for a review, see Huang & Rao, Citation2011). Current conceptions of predictive coding provide schemes for learning efficient codes using generative models. Networks using predictive coding may sometimes need feedback for learning of efficient codes, but once a code has been developed, online processing can be done by the feed-forward connections alone.
Indeed, early work on predictive coding did not use top-down processing at all. The idea of predictive coding dates back to the early 1950s (Harrison, Citation1952; Oliver, Citation1952), and was introduced in the context of radio and television transmission. In the context of speech processing, linear predictive coding forms the basis of a standard speech compression algorithm, used for instance in the GSM phone system. In all of these cases, the aim was to construct an efficient code to allow information to be transmitted over a channel with limited bandwidth. For example, a simple form of predictive coding would be one taking advantage of the fact that, in television signals, very little changes from one frame to the next. Transmission can be made much more efficient if only the difference between successive frames (the prediction error) is transmitted. If the input signal violates the predictions embodied in the transmitter and receiver, the transmitter will need to pass on the error signal – that is, the discrepancy between the prediction and the input. The receiver must know the code used by the transmitter, but need not pass information back to it. It should be clear from this example that neither the transmitter nor the receiver generate specific predictions that anticipate what will come next.
The television example is about predictive coding in the temporal domain, but such coding can also operate in the spatial domain. This is the basis of predictive coding models of the retina (e.g. Hosoya, Baccus, & Meister, Citation2005; Srinivasan, Laughlin, & Dubs, Citation1982). The retina can take advantage of the fact that signals in adjacent locations are highly correlated. That is, the value at one location effectively predicts the value at nearby locations. Instead of transmitting the absolute light levels in individual cells, it is possible to reduce the required bandwidth by transmitting only the difference between each cell and its neighbours. Note that there may be no need to decode the information that is transmitted. As Hosoya et al. (Citation2005) point out, the goal of predictive coding is not to pass on to the brain a veridical representation of the world, “Instead, the system must reduce the onslaught of raw visual information and extract the few bits of information that are relevant to behaviour.”
The main prerequisite for predictive coding, therefore, is to discover the most efficient code. This could be done using feedback to tune the encoder (see Figure 6 of Rao & Ballard, Citation1999), although Rao and Ballard noted that “the equation for the dynamics of the network can be rewritten such that some of the effects of feedback are replaced by recurrent lateral interactions” (p. 84). Huang and Rao (Citation2011) add that “it is not yet clear from neurophysiological experiments whether feedback connections indeed carry predictions, and feed-forward connections the residual errors”.
An encoder might also learn a compressed code autonomously. This code could then be passed to later stages of perception which have to learn how to interpret that code. This has parallels with how deep neural networks used for speech and object recognition are constructed (Hinton, Osindero, & Teh, Citation2006). The initial layers are “stacked” Restricted Boltzmann Machines which perform unsupervised learning. Subsequent layers are trained by supervised learning. Once trained, these networks achieve state-of-the-art recognition performance operating in a purely feed-forward manner. This invokes once again the distinction between online and offline feedback. Feedback may sometimes be necessary to learn a code but, once established, that code can be used without feedback.
As a framework for understanding neural computation, predictive coding has been very productive. The most successful applications of the predictive coding approach have been in modelling low-level vision, and the target has been primarily neurobiological data rather than behavioural data. More recently though, Yildiz, von Kriegstein, and Kiebel (Citation2013) have developed a computational model of speech recognition based on predictive coding, in the sense of a hierarchical generative model. It is, of course, a Bayesian model, and it is an extension of an earlier biologically inspired model of production and recognition of birdsong (Yildiz & Kiebel, Citation2011). Top-down connections play a critical role in both learning and recognition in this model. Recognition is determined by the representation (called a “module”) that leads to the lowest prediction error in generating the sensory input. The fact that this model can also learn to recognise speech gives it an advantage over TRACE and Shortlist. However, it has yet to be applied to the same range of phenomena as either of those models. The current simulation is limited to a small vocabulary, but it can recognise spoken digits rather than simply working on a transcription of the spoken input. Note, however, that even for this approach as a model of birdsong there are competing accounts using feed-forward architectures (Drew & Abbott, Citation2003; Larson, Billimoria, & Sen, Citation2009). Nevertheless, Yildiz et al.'s model is an exciting development. It now remains to be seen whether this model makes novel predictions.
5. Conclusions
There can be no doubt that listeners make predictions when listening to speech. The doubts arise when we consider how, or even whether, these predictions influence lower level perceptual processes. In the behavioural literature, questions about feedback have generally been formulated in the context of simple feedback of activation from lexical to pre-lexical processes. Early behavioural studies collated cases where lexical information had an influence on phoneme detection or classification and presented these as evidence of feedback. However, these effects simply showed that lexical information could bias decisions made about the output of pre-lexical processes; this effect could readily be simulated by feed-forward models. Although IAMs could also simulate these effects, this form of interaction is unable to do anything to improve perception.
The adoption of a Bayesian framework has changed the nature of the debate over prediction and interaction. Improving perception now means performing Bayesian inference, and this can be done using either feed-forward models (Norris & McQueen, Citation2008) or modified IAMs (McClelland, Citation2013). In most behavioural tasks these competing accounts will therefore make exactly the same predictions. Indeed, the compensation for coarticulation paradigm seems to be the only one capable of generating behavioural data that might distinguish between the two accounts. There the data favours the non-interactive view (McQueen at al., Citation2009; Pitt & McQueen, Citation1998). Recent neuroscientific evidence (Travis et al., Citation2013) also suggests that there is an early stage of acoustic-phonetic processing that is uninfluenced by lexical context.
Although, as we have demonstrated, there is little support for simple activation feedback in online processing, other forms of feedback or recurrence can play an important role in perception. In particular, there is ample evidence that feedback makes an important contribution to learning. Such beneficial forms of feedback have yet to receive the attention they deserve. The feedback embodied in generative models serves a useful function and may deliver testable behavioural predictions. We have suggested that binding also involves a form of feedback which would be needed in order to implement even those models we typically think of as feed-forward.
Bayes and prediction are natural bedfellows. Bayes' theorem specifies how to update predictions (beliefs) in the light of new evidence. In a simple Bayesian model such as Shortlist B, the baseline prediction is that words will appear with probabilities determined by their frequency of occurrence in the language. As new spoken input arrives, those probabilities are updated in the light of that new evidence so as to generate revised predictions. Yildiz et al.’s (Citation2013) model is concerned with predicting the perceptual realisation of words. Kleinschmidt and Jaeger (Citation2015) focus on how listeners' predictions adapt as a result of learning. The content of the prediction in these models is subtly different, but they have in common that they all are Bayesian.
When the first computational models of speech recognition were developed it seemed to many inevitable that a task as complex as speech perception would involve activation feedback. Decades later, however, there is still no convincing behavioural evidence that this is the case. Part of the reason for the early enthusiasm for top-down prediction was that the power of bottom-up models was often unappreciated. The performance of an ideal observer cannot be beaten, and Bayesian (ideal observer) models do not require top-down prediction. The value of generative models and predictive coding might also seem obvious, but the scarcity so far of explicit computational models embodying these ideas makes them hard to evaluate. To reiterate: the question is not whether there is prediction but when and how it operates. Without worked-out models it is hard to generate the most important predictions – what should the data look like?
Disclosure statement
No potential conflict of interest was reported by the authors.
References
- Abdel-Hamid, O., Deng, L., & Yu, D. (2013). Exploring convolutional neural network structures and optimization techniques for speech recognition. Proceedings of Interspeech 2013, Lyon (pp. 3366–3370).
- Altmann, G. T. M., & Kamide, Y. (1999). Incremental interpretation at verbs: Restricting the domain of subsequent reference. Cognition, 73, 247–264. doi:10.1016/S0010-0277(99)00059-1
- Arai, M., & Keller, F. (2013). The use of verb-specific information for prediction in sentence processing. Language and Cognitive Processes, 28, 525–560. doi:10.1080/01690965.2012.658072
- Bever, T. G., & Poeppel, D. (2010). Analysis by synthesis: A (re-)emerging program of research for language and vision. Biolinguistics, 4.2–0.3, 174–200. ISSN 1450–3417
- Brouwer, S., Mitterer, H., & Huettig, F. (2013). Discourse context and the recognition of reduced and canonical spoken words. Applied Psycholinguistics, 34, 519–539. doi:10.1017/S0142716411000853
- Brunellière, A., & Soto-Faraco, S. (2013). The speakers’ accent shapes the listeners’ phonological predictions during speech perception. Brain and Language, 125, 82–93. doi:10.1016/j.bandl.2013.01.007
- Chambers, C. G., Tanenhaus, M. K., Eberhard, K. M., Filip, H., & Carlson, G. N. (2002). Circumscribing referential domains during real-time language comprehension. Journal of Memory and Language, 47, 30–49. doi:10.1006/jmla.2001.2832
- Clos, M., Langner, R., Meyer, M., Oechslin, M. S., Zilles, K., & Eickhoff, S. B. (2014). Effects of prior information on decoding degraded speech: An fMRI study. Human Brain Mapping, 35, 61–74. doi:10.1002/hbm.22151
- Connine, C. M. (1987). Constraints on interactive processes in auditory word recognition: The role of sentence context. Journal of Memory and Language, 26, 527–538. doi:10.1016/0749-596X(87)90138-0
- Connine, C. M., Titone, D., & Wang, J. (1993). Auditory word recognition: Extrinsic and intrinsic effects of word frequency. Journal of Experimental Psychology: Learning, Memory and Cognition, 19, 81–94. doi:10.1037/0278-7393.19.1.81
- Cutler, A. (1976). Phoneme monitoring reaction time as a function of preceding intonation contour. Perception & Psychophysics, 20, 55–60. doi:10.3758/BF03198706
- Dahan, D., & Tanenhaus, M. K. (2004). Continuous mapping from sound to meaning in spoken-language comprehension: Immediate effects of verb-based thematic constraints. Journal of Experimental Psychology: Learning, Memory and Cognition, 30, 498–513. doi:10.1037/0278-7393.30.2.498
- Davis, M. H., & Johnsrude, I. S. (2007). Hearing speech sounds: Top-down influences on the interface between audition and speech perception. Hearing Research, 229, 132–147. doi:10.1016/j.heares.2007.01.014
- DeWitt, I., & Rauschecker, J. P. (2012). Phoneme and word recognition in the auditory ventral stream. Proceedings of the National Academy of Sciences, 109, E505–E514. doi:10.1073/pnas.1113427109
- Drew, P. J., & Abbott, L. F. (2003). Model of song selectivity and sequence generation in area HVc of the songbird. Journal of Neurophysiology, 89, 2697–2706. doi:10.1152/jn.00801.2002
- Elman, J. L., & McClelland, J. L. (1988). Cognitive penetration of the mechanisms of perception: Compensation for coarticulation of lexically restored phonemes. Journal of Memory and Language, 27, 143–165. doi:10.1016/0749-596X(88)90071-X
- Feldman, N. H., Griffiths, T. L., & Morgan, J. L. (2009). The influence of categories on perception: Explaining the perceptual magnet effect as optimal statistical inference. Psychological Review, 116, 752–782. doi:10.1037/a0017196
- Friston, K. (2003). Learning and inference in the brain. Neural Networks: The Official Journal of the International Neural Network Society, 16, 1325–1352. doi:10.1016/j.neunet.2003.06.005
- Gagnepain, P., Henson, R. N., & Davis, M. H. (2012). Temporal predictive codes for spoken words in auditory cortex. Current Biology, 22, 615–621. doi:10.1016/j.cub.2012.02.015
- Galantucci, B., Fowler, C. A., & Turvey, M. T. (2006). The motor theory of speech perception reviewed. Psychonomic Bulletin & Review, 13, 361–377. doi:10.3758/BF03193857
- Ganong, W. F. (1980). Phonetic categorization in auditory word perception. Journal of Experimental Psychology: Human Perception and Performance, 6, 110–125. doi:10.1037/0096-1523.6.1.110
- Geisler, W. S. (2003). Ideal observer analysis. In L. Chalupa & J. Werner (Eds.), The visual neurosciences (pp. 825–837). Cambridge, MA: MIT Press.
- Geisler, W. S., & Kersten, D. (2002). Illusions, perception and Bayes. Nature Neuroscience, 5, 508–510. doi:10.1038/nn0602-508
- Gilbert, C. D., & Sigman, M. (2007). Brain states: Top-down influences in sensory processing. Neuron, 54, 677–696. doi:10.1016/j.neuron.2007.05.019
- Gow, D. W. (2012). The cortical organization of lexical knowledge: A dual lexicon model of spoken language processing. Brain and Language, 121, 273–288. doi:10.1016/j.bandl.2012.03.005
- Gow, D. W., Segawa, J. A., Ahlfors, S. P., & Lin, F.-H. (2008). Lexical influences on speech perception: A granger causality analysis of MEG and EEG source estimates. Neuroimage, 43, 614–623. doi:10.1016/j.neuroimage.2008.07.027
- Halle, M., & Stevens, K. N. (1959). Analysis by synthesis. In W. Wathen-Dunn & L. E. Woods. Proceedings of the seminar on speech compression and processing (Vol. 2). AFCRC-TR-59-198. USAF Camb. Res. Ctr. 2: Paper D7.
- Halle, M., & Stevens, K. N. (1962). Speech recognition: A model and a program for research. IRE Transactions on Information Theory, 8, 155–159. doi:10.1109/TIT.1962.1057686
- Harrison, C. W. (1952). Experiments with linear prediction in television. Bell System Technical Journal, 31, 764–783. doi:10.1002/j.1538-7305.1952.tb01405.x
- Hickok, G., & Poeppel, D. (2007). The cortical organization of speech processing. Nature Reviews Neuroscience, 8, 393–402. doi:10.1038/nrn2113
- Hinton, G., Deng, L., Yu, D., Dahl, G. E., Mohamed, A., Jaitly, N., … Kingsbury, B. (2012). Deep neural networks for acoustic modelling in speech recognition. IEEE Signal Processing Magazine, 29(November), 82–97. doi:10.1109/MSP.2012.2205597
- Hinton, G. E., Osindero, S., & Teh, Y. (2006). A fast learning algorithm for deep belief nets. Neural Computation, 18, 1527–1554. doi:10.1162/neco.2006.18.7.1527
- Hosoya, T., Baccus, S., & Meister, M. (2005). Dynamic predictive coding by the retina. Nature, 436(7047), 71–77. doi:10.1038/nature03689
- Howes, D. H. (1957). On the relation between the intelligibility and frequency of occurrence of English words. Journal of the Acoustical Society of America, 29, 296–305. doi:10.1121/1.1908862
- Huang, Y., & Rao, R. P. N. (2011). Predictive coding. Wiley Interdisciplinary Reviews: Cognitive Science, 2, 580–593. doi:10.1002/wcs.142
- Johnsrude, I. S., Mackey, A., Hakyemez, H., Alexander, E., Trang, H. P., & Carlyon, R. P. (2013). Swinging at a cocktail party: Voice familiarity aids speech perception in the presence of a competing voice. Psychological Science, 24, 1995–2004. doi:10.1177/0956797613482467
- Kamide, Y., & Scheepers, C., & Altmann, G. T. M. (2003). Integration of syntactic and semantic information in predictive processing: Cross-linguistic evidence from German and English. Journal of Psycholinguistic Research, 32, 37–55. doi:10.1023/A:1021933015362
- Kilner, J. M., Friston, K. J., & Frith, C. D. (2007). Predictive coding: An account of the mirror neuron system. Cognitive Processing, 8(3), 159–166. doi:10.1007/s10339-007-0170-2
- Kleinschmidt, D. F., & Jaeger, T. F. (2015). Robust speech perception: Recognize the familiar, generalize to the similar, and adapt to the novel. Psychological Review, 122(2), 148–203. doi:10.1037/a0038695
- Larson, E., Billimoria, C. P., & Sen, K. (2009). A biologically plausible computational model for auditory object recognition. Journal of Neurophysiology, 101, 323–331. doi:10.1152/jn.90664.2008
- Liberman, A. M., Cooper, F. S., Shankweiler, D. P., & Studdert-Kennedy, M. (1967). Perception of speech code. Psychological Review, 74, 431–461. doi:10.1037/h0020279
- Luce, P. A., & Pisoni, D. B. (1998). Recognizing spoken words: The neighborhood activation model. Ear & Hearing, 19, 1–36. doi:10.1097/00003446-199802000-00001
- Magnuson, J. S., McMurray, B., Tanenhaus, M. K., & Aslin, R. N. (2003). Lexical effects on compensation for coarticulation: The ghost of Christmash past. Cognitive Science, 27, 285–298. doi:10.1016/S0364-0213(03)00004-1
- Magnuson, J. S., Tanenhaus, M. K., & Aslin, R. N. (2008). Immediate effects of form-class constraints on spoken word recognition. Cognition, 108, 866–873. doi:10.1016/j.cognition.2008.06.005
- Mann, V. A., & Repp, B. H. (1981). Influence of preceding fricative on stop consonant perception. Journal of the Acoustical Society of America, 69, 548–558. doi:10.1121/1.385483
- Marr, D. (1982). Vision. San Francisco, CA: Freeman. ISBN 0-7167-1284-9
- Maye, J., Aslin, R., & Tanenhaus, M. (2008). The Weckud Wetch of the wast: Lexical adaptation to a novel accent. Cognitive Science: A Multidisciplinary Journal, 32, 543–562. doi:10.1080/03640210802035357
- McClelland, J. L. (1991). Stochastic interactive processes and the effect of context on perception. Cognitive Psychology, 23, 1–44. doi:10.1016/0010-0285(91)90002-6
- McClelland, J. L. (2013). Integrating probabilistic models of perception and interactive neural networks: A historical and tutorial review. Frontiers in Psychology, 4, 503. doi:10.3389/fpsyg.2013.00503
- McClelland, J. L., & Elman, J. L. (1986). The TRACE model of speech perception. Cognitive Psychology, 18, 1–86. doi:10.1016/0010-0285(86)90015-0
- McClelland, J. L., Mirman, D., Bolger, D. J., & Khaitan, P. (2014). Interactive activation and mutual constraint satisfaction in perception and cognition. Cognitive Science, 38, 1139–1189. doi:10.1111/cogs.12146
- McQueen, J. M. (1991a). The influence of the lexicon on phonetic categorization: Stimulus quality in word-final ambiguity. Journal of Experimental Psychology: Human Perception and Performance, 17, 433–443. doi:10.1037/0096-1523.17.2.433
- McQueen, J. M. (1991b). Phonetic decisions and their relationship to the lexicon. Ph.D. dissertation, University of Cambridge.
- McQueen, J. M. (2003). The ghost of Christmas future: Didn't scrooge learn to be good? Commentary on Magnuson, McMurray, Tanenhaus and Aslin (2003). Cognitive Science, 27, 795–799. doi:10.1207/s15516709cog2705_6
- McQueen, J. M., Cutler, A., Briscoe, T., & Norris, D. (1995). Models of continuous speech recognition and the contents of the vocabulary. Language and Cognitive Processes, 10, 309–331. doi:10.1080/01690969508407098
- McQueen, J. M., Cutler, A., & Norris, D. (2006). Phonological abstraction in the mental lexicon. Cognitive Science, 30, 1113–1126. doi:10.1207/s15516709cog0000_79
- McQueen, J. M., Jesse, A., & Norris, D. (2009). No lexical–prelexical feedback during speech perception or: Is it time to stop playing those Christmas tapes? Journal of Memory and Language, 61, 1–18. doi:10.1016/j.jml.2009.03.002
- McQueen, J. M., Tyler, M. D., & Cutler, A. (2012). Lexical retuning of children's speech perception: Evidence for knowledge about words’ component sounds. Language Learning and Development, 8, 317–339. doi:10.1080/15475441.2011.641887
- Miller, J. L., Green, K., & Schermer, T. (1984). On the distinction between the effects of sentential speaking rate and semantic congruity on word identification. Perception & Psychophysics, 36, 329–337. doi:10.3758/BF03202785
- Mumford, D. (1992). On the computational architecture of the neocortex – II The role of cortico-cortical loops. Biological Cybernetics, 66, 241–251. doi:10.1007/BF00198477
- Myers, E. B., & Blumstein, S. E. (2008). The neural bases of the lexical effect: An fMRI investigation. Cerebral Cortex, 18, 278–288. doi:10.1093/cercor/bhm053
- Norris, D. (1994). Shortlist: A connectionist model of continuous speech recognition. Cognition, 52, 189–234. doi:10.1016/0010-0277(94)90043-4
- Norris, D. (2006). The Bayesian reader: Explaining word recognition as an optimal Bayesian decision process. Psychological Review, 113, 327–357. doi:10.1037/0033-295X.113.2.327
- Norris, D. (2009). Putting it all together: A unified account of word recognition and reaction-time distributions. Psychological Review, 116, 207–219. doi:10.1037/a0014259
- Norris, D., & Kinoshita, S. (2012). Reading through a noisy channel: Why there's nothing special about the perception of orthography. Psychological Review, 119, 517–545. doi:10.1037/a0028450
- Norris, D., & McQueen, J. M. (2008). Shortlist B: A Bayesian model of continuous speech recognition. Psychological Review, 115, 357–395. doi:10.1037/0033-295X.115.2.357
- Norris, D., McQueen, J. M., & Cutler, A. (2000). Merging information in speech recognition: Feedback is never necessary. Behavioral and Brain Sciences, 23, 299–325. doi:10.1017/S0140525X00003241
- Norris, D., McQueen, J. M., & Cutler, A. (2003). Perceptual learning in speech. Cognitive Psychology, 47, 204–238. doi:10.1016/S0010-0285(03)00006-9
- Oliver, B. M. (1952). Efficient coding. Bell System Technical Journal, 31, 724–750. doi:10.1002/j.1538-7305.1952.tb01403.x
- Pece, A. (2007). On the computational rationale for generative models. Computer Vision and Image Understanding, 106, 130–143. doi:10.1016/j.cviu.2006.10.002
- Pelli, D. G., Burns, C. W., Farell, B., & Moore-Page, D. C. (2006). Feature detection and letter identification. Vision Research, 46, 4646–4674. doi:10.1016/j.visres.2006.04.023
- Pitt, M. A., & McQueen, J. M. (1998). Is compensation for coarticulation mediated by the lexicon? Journal of Memory and Language, 39, 347–370. doi:10.1006/jmla.1998.2571
- Poeppel, D., Idsardi, W. J., & van Wassenhove, V. (2008). Speech perception at the interface of neurobiology and linguistics. Philosophical Transactions of the Royal Society B-Biological Sciences, 363, 1071–1086. doi:10.1098/rstb.2007.2160
- Poeppel, D., & Monahan, P. (2010). Feedforward and feedback in speech perception: Revisiting analysis by synthesis. Language and Cognitive Processes, 26, 935–951. doi:10.1080/01690965.2010.493301
- Pollack, I., Rubenstein, H., & Decker, L. (1959). Intelligibility of known and unknown message sets. Journal of the Acoustical Society of America, 31, 273–279. doi:10.1121/1.1907712
- Price, C. J. (2012). A review and synthesis of the first 20 years of PET and fMRI studies of heard speech, spoken language and reading. Neuroimage, 62, 816–847. doi:10.1016/j.neuroimage.2012.04.062
- Rao, R. P., & Ballard, D. H. (1999). Predictive coding in the visual cortex: A functional interpretation of some extra-classical receptive-field effects. Nature Neuroscience, 2, 79–87. doi:10.1038/4580
- Rao, R. P. N., & Ballard, D. H. (1997). Dynamic model of visual recognition predicts neural response properties in the visual cortex. Neural Computation, 9, 721–763. doi:10.1162/neco.1997.9.4.721
- Rubin, P., Turvey, M. T., & van Gelder, P. (1976). Initial phonemes are detected faster in spoken words than in nonwords. Perception & Psychophysics, 19, 394–398. doi:10.3758/BF03199398
- Rumelhart, D. E., & McClelland, J. L. (1982). An interactive activation model of context effects in letter perception: II. The contextual enhancement effect and some tests and extensions of the model. Psychological Review, 89(1), 60–94. doi:10.1037/0033-295X.89.1.60
- Samuel, A. G. (1981). Phonemic restoration: Insights from a new methodology. Journal of Experimental Psychology: General, 110, 474–494. doi:10.1037/0096-3445.110.4.474
- Samuel, A. G., & Kraljic, T. (2009). Perceptual learning in speech perception. Attention, Perception & Psychophysics, 71, 1207–1218. doi:10.3758/APP.71.6.1207
- Samuel, A. G., & Pitt, M. A. (2003). Lexical activation (and other factors) can mediate compensation for coarticulation. Journal of Memory and Language, 48, 416–434. doi:10.1016/S0749-596X(02)00514-4
- Serre, T., Oliva, A., & Poggio, T. (2007). A feedforward architecture accounts for rapid categorization. Proceedings of the National Academy of Sciences of the United States of America, 104(15), 6424–6429. doi:10.1073/pnas.0700622104
- Sjerps, M. J., & McQueen, J. M. (2010). The bounds of flexibility in speech perception. Journal of Experimental Psychology: Human Perception and Performance, 36(1), 195–211. doi:10.1037/a0016803
- Sohoglu, E., Peelle, J. E., Carlyon, R. P., & Davis, M. H. (2012). Predictive top-down integration of prior knowledge during speech perception. Journal of Neuroscience, 32(25), 8443–8453. doi:10.1523/JNEUROSCI.5069-11.2012
- Sohoglu, E., Peelle, J. E., Carlyon, R. P., & Davis, M. H. (2014). Top-down influences of written text on perceived clarity of degraded speech. Journal of Experimental Psychology. Human Perception and Performance, 40(1), 186–199. doi:10.1037/a0033206
- Srinivasan, M. V., Laughlin, S. B., & Dubs, A. (1982). Predictive coding: A fresh view of inhibition in the retina. Philosophical Transactions of the Royal Society B: Biological Sciences, 216, 427–459. doi:10.1098/rspb.1982.0085
- Travis, K. E., Leonard, M. K., Chan, A. M., Torres, C., Sizemore, M. L., … Halgren, E. (2013). Independence of early speech processing from word meaning. Cerebral Cortex, 23, 2370–2379. doi:10.1093/cercor/bhs228
- Ueno, T., Saito, S., Rogers, T. T., & Lambon Ralph, M. A. (2011). Lichtheim 2: Synthesizing aphasia and the neural basis of language in a neurocomputational model of the dual dorsal-ventral language pathways. Neuron, 72, 385–396. doi:10.1016/j.neuron.2011.09.013
- Van Alphen, P., & McQueen, J. M. (2001). The time-limited influence of sentential context on function word identification. Journal of Experimental Psychology: Human Perception and Performance, 27, 1057–1071. doi:10.1037/0096-1523.27.5.1057
- Van Berkum, J. J. A., Brown, C. M., Zwitserlood, P., Kooijman, V., & Hagoort, P. (2005). Anticipating upcoming words in discourse: Evidence from ERPs and reading times. Journal of Experimental Psychology: Learning, Memory, and Cognition, 31, 443–467. doi:10.1037/0278-7393.31.3.443
- Van Wassenhove, V., Grant, K. W., & Poeppel, D. (2005). Visual speech speeds up the neural processing of auditory speech. Proceedings of the National Academy of Sciences of the United States of America, 102, 1181–1186. doi:10.1073/pnas.0408949102
- Warren, R. M., Obusek, C. J., & Ackroff, J. M. (1972). Auditory induction: Perceptual synthesis of absent sounds. Science, 176, 1149–1151. doi:10.1126/science.176.4039.1149
- Yildiz, I. B., & Kiebel, S. J. (2011). A hierarchical neuronal model for generation and online recognition of birdsongs. PloS Computational Biology, 7, e1002303. doi:10.1371/journal.pcbi.1002303
- Yildiz, I. B., von Kriegstein, K., & Kiebel, S. J. (2013). From birdsong to human speech recognition: Bayesian inference on a hierarchy of nonlinear dynamical systems. PLoS Computational Biology, 9, e1003219. doi:10.1371/journal.pcbi.1003219
- Yuille, A. L. (1991). Deformable templates for face recognition. Journal of Cognitive Neuroscience, 3, 59–70. doi:10.1162/jocn.1991.3.1.59
- Zhou, B., Lapedriza, A., Xiao, J., Torralba, A., & Oliva, A. (2014). Learning deep features for scene recognition using places database. Advances in Neural Information Processing, 27, 487–495.