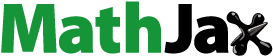
Abstract
Proper use of neuropsychological tests in Indonesia is hindered by a lack of properly adapted neurocognitive tests as well as an absence of normative data. In 2016, we started adapting ten of these tests for use in Indonesia and collected data from healthy participants in Java. Here we introduce and propose a system that will facilitate the proper usage and interpretation of test scores: an online platform and a dynamic database. Newly collected data (492 healthy adults) of the Indonesian version of the Boston Naming Test (I-BNT) were used to illustrate the usefulness of the two functions. Analysis of variances, post-hoc tests, and a simulation study revealed the effects of age and education on the I-BNT, indicating that it is imperative to fine-tune the reference group based on these demographic factors. Putative inadequate sample size issues for obtaining reliable normative scores were overcome by employing regression analyses and the prediction of normative scores. It can be concluded that a flexible online platform is available for the calculation of normative scores either based on the whole population, on fine-tuned reference groups, or on predicted scores. The dynamic database’s growth will allow to obtain even more fine-tuned and more reliable reference data as well as more accurate predictions. Fine-tuned reference data are badly needed for the heterogenous Indonesian population.
Introduction
Normative scores are the key to indicate an individual’s relative standing within a reference population. Traditionally, an individual’s performance on one or series of neuropsychological tests is compared with a published reference group of normative data (Casaletto & Heaton, Citation2017; Zucchella et al., Citation2018). However, normative data may become out-of-date or drawn from another society, country, culture, ethnic group, or continent. An adequate interpretation of test scores demands that normative data be recently collected or recollected from a sample that mimics the respondent’s demographic profile as much as possible.
The rapid development of technology in health practices and research led to the development of large online databases containing various types of clinical data, including normative scores e.g., Meyers (Citation2013). An example of such a database is the one collected by Weintraub et al. (Citation2009). They collected data using the UDS (Uniform Data Set), a neuropsychological test battery for patients with different levels of dementia. The project was continued by the creation of an online normative data calculator with demographic adjustments to facilitate the preclinical diagnosis of Alzheimer’s Disease (Shirk et al., Citation2011). In addition to these examples, there is a newly developed neuropsychological online tool that compares a client’s score with fine-tuned reference scores stored in a dynamic database. This system is currently used in the Netherlands. It is named Advanced Neuropsychological Diagnostics Infrastructure (ANDI), and was developed by researchers from the University of Amsterdam (De Vent et al., Citation2016). It consists of a dynamic database and an online platform that incorporates demographic data and neuropsychological test scores from a growing number of subjects. Its data are obtained from clinical neuropsychologists and researchers investigating neurocognition with a variety of tests in various healthy control groups in the Netherlands.
In the current study, we introduce and illustrate the Indonesian version of ANDI (named I-ANDI hereafter) with its two functions: a dynamic database and an online platform. The dynamic database is an infrastructure to store and incorporate various neuropsychological test scores from a large group of healthy subjects. The online platform allows neuropsychologists and clinicians in Indonesia to define a reference group based on demographic characteristics resembling those of their clients and thereby allow them to interpret their client’s scores efficiently and adequately.
There are two differences between the Dutch-ANDI and I-ANDI. The Dutch-ANDI contains donated data from a variety of tests from various neuropsychologists and practitioners in the Netherlands. We collected data ourselves by collaborating with university partners in various parts of Indonesia (consortium). Secondly, the data obtained with the ten adapted neuropsychological tests stored in I-ANDI’s database were administered as a battery: that is, all subjects received all ten tests and this was not the case in the Dutch ANDI.
Indonesia is an archipelago with different ethnic groups that originate from different parts of the country. Therefore, it is necessary to spread the data collection over islands and different parts of islands in order to make sure that data from different ethnic groups will be stored in the database. These data can then be used for normative scores, for developing normative scores for separate ethnic groups, either alone or in combination with the common demographic factors education, age, and sex. Currently, I-ANDI provides facilities for establishing normative scores for the most common demographic factors: age, sex, and education. I-ANDI also provides the possibility of storing patient data. However, the patient data will remain separate from the healthy controls’ data and will be used only for research purposes.
This paper illustrates the usefulness of I-ANDI for Indonesia’s neuropsychological practices. Data of the Boston Naming Test, adapted for Indonesia (I-BNT) by (Sulastri et al., Citation2019), will be used for that purpose. ANOVA’s and regression analyses will be used to determine the influence of demographic factors on the I-BNT. If there is found to be an influence, then different groups will require different normative data. Finally, a simulation study will illustrate the flexibility of I-ANDI regarding the composition of the reference group for obtaining appropriate normative data.
Methods
The initial I-ANDI data collection
With supports from the European Union (Erasmus Grant outside Europe) and the Indonesian government for the project entitled “Development of I-ANDI,” we established partnerships in 2016 with Radboud University, Nijmegen, the Netherlands and other Indonesian universities within the scheme of the consortium of researchers for our data collection. The consortium members were twelve researchers from Soegijapranata Catholic University in Semarang, Atma Jaya Catholic University in Jakarta, Widya Mandala Catholic University in Surabaya and Radboud University, the Netherlands. We started the data collection by choosing a battery consisting of ten clinically relevant neuropsychological tests covering the domains learning and memory, attention, executive function, and language and then adapted the tests. The ten neuropsychological tests were the Auditory Verbal Learning, Figural Reproduction, Digit Span, Bourdon-Wiersma, Stroop Colour-word, Trail Making, I-Boston Naming Test, Token Test, Verbal Fluency, and Five Point Test. All ten tests were administered to all subjects and the data were stored in the I-ANDI database representing a sample of healthy subjects and further used to develop normative data. In early 2020 we embarked on new collaborations with mental health care providers and with hospitals. Going forward, we anticipate partnerships to grow with other universities on other islands of Indonesia. This will make it possible to increase the number of subjects in the data base and increase its ethnic diversity. The I-ANDI database is intended to contain two categories of subjects: healthy subjects and patients. However, because of Indonesia’s Covid-19 outbreak in March of 2020, we temporarily postponed data collection from different patient categories. Now only the scores from the battery are stored, in the future, I-ANDI will allow data and datasets from single tests as well.
The number of subjects in a subgroup
At the time of the analyses, the database contained 492 subjects. Given that normative data of most cognitive tests are education-, age- and sex-dependent, we analyzed the effects of these factors, although, in our preliminary study reporting normative data on I-BNT (Sulastri et al., Citation2019), sex effects were not found. Given that education and age effects have been previously reported for the BNT (Neils et al., Citation1995), it can be expected that different normative scores for education-age categories will be necessary. Given the number and distribution of subjects in our dataset, we anticipate that not all education-age categories will contain the desired 50 subjects (Bridges & Holler, Citation2007). It is especially likely that the number of subjects with less than seven years of education and the number of older people with various degrees of education will be too low for obtaining reliable normative scores for these subgroups. Alternatively, normative scores could also be calculated with regression-based approaches (Tombaugh, Citation2004). The approaches have the advantage of requiring much smaller sample sizes (Oosterhuis et al., Citation2016) with improved diagnostic precision (Burggraaff et al., Citation2017).
Moreover, a regression-based normative formula that is simultaneously correct for all significant demographic variables might be more desirable for the clinician and more sensitive in detecting cognitive problems. In all, regression-based normative data is most desirable if there are fewer than 50 subjects to calculate the normative scores of a subgroup. Meanwhile, the need for normative scores from adapted neuropsychological tests is high, and Indonesia does not have a neuropsychological battery test database with normative data as yet.
Construction of the indonesian advanced neuropsychological diagnostics infrastructure (I-ANDI)
Inspired by the Dutch ANDI normative database system developed by De Vent et al. (2016), we developed a dynamic database and online platform for Indonesia and named it I-ANDI. illustrates the stepwise construction and design of I-ANDI and how to use it. The I-ANDI user can submit a client’s test score and retrieve his client’s score as it relates to the scores of a reference group.
Figure 1. The stepwise construction and design of I-ANDI. The boxes under the dynamic databases (light grey) illustrate the data flow from data collection to data storage in two separate units. The boxes on the right side (yellow) represent the data flow in the online platform.
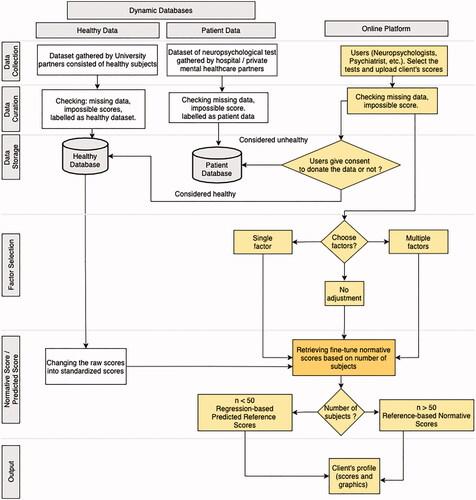
Data collection
I-ANDI provides two kinds of data entry to the dynamic database: (1) data collected by us, or by university, hospital and private mental healthcare providers that are collaborating under a Memorandum of Understanding; and (2) data provided via the online platform that is from other neuropsychologists, psychiatrists, and clinicians throughout the country.
Data gathered by us and by our university partners are from healthy subjects. These data will be used to develop normative scores and will be labeled and stored in the “healthy subjects” database. Data gathered by our university, hospital and mental healthcare partners under a similar collaborative arrangement are from various groups of diagnosed patients. These will be labeled and stored separately as patient data. To gain access to the I-ANDI database, a user will first register on the I-ANDI website. Then after having been approved by the Person in Charge (PIC) of the consortium responsible for the quality and security of the I ANDI database, the user will be able to select the test and upload their client data. I-ANDI will provide templates for the uploading of data in the form of spreadsheet and Comma Separated Values (CSV).
Data curation
This phase is designed to ensure the quality of the data entered. The submitted data are first checked for completeness. When there is incomplete or missing data, the system will alert the user to completes the data before resubmission and continuation of the process. The data are also checked for impossible scores, such as a score on any test that is higher than the test’s maximum. In this manner, coding or typographic errors will be kept from contaminating the data file.
Data storage
I-ANDI has two separate databases: the healthy and the patient database. The on-line available data are stored anonymously. Before entering the data, a clinician or user first determines whether the data originate from a patient or not. In case of doubts, a second opinion expert will be consulted. Next, the user indicates whether one wishes to donate the data to the consortium.
Factor selection
By selecting from among several different demographic factors, users can select the normative score that best mimics the characteristics of his client(s). In other words, users can fine-tune the normative reference score. There are several different demographic choices available: multiple (e.g., age, education, sex), single (e.g., age), or none; the latter implies that the entire dataset will be used to calculate the normative scores.
In the future, when users want to select other or additional demographic factors in order to obtain a more specific or unique normative score, the system is capable of accommodating them. For example, factors such as the client’s “daily spoken language” or the ethnicity of the client’s parents can be added. Such information might be particularly helpful in deriving normative scores that are most suitable for language tests.
Normative score/predicted score
I-ANDI can generate normative scores and/or predictive normative scores from the healthy database. We transformed the raw scores of all tests into standardized scores (z-score). The system provides the fine-tuned normative scores based on the factor(s) chosen by the users. There are two possibilities for calculating normative scores that depend on the number of subjects. If the number of subjects is 50 or more, the system provides an output based on the normative reference-based scores, whereas when the number of subjects is less than 50, the system will recommend the use of regression-based predicted normative scores. The predicted score is obtained from the regression analysis’s weighted score using existing data in the database.
Output
An individual’s test score compared with the normative scores obtained from the chosen dataset is numerically and graphically displayed, next to the number of subjects on which the normative scores are calculated.
Participants
Data from the I-BNT were used to illustrate the newly developed system tools. They were collected during 2017–2019 in the island of Java (Jakarta, representing West Java, N = 193), Semarang (Central Java, N = 197), and Surabaya (East Java, N = 102). Java was chosen considering that it has the highest (57%) percentage of the total Indonesian population (267 million). Participants consisted of 295 females and 197 males, with an age range of 16−80 years old (M = 33.2; SD = 15.2). Participants were categorized into four age-by-decade groups (Palmer et al., Citation1998; Van Den Berg et al., Citation2009): (i) age 20–29 years, (ii) age 30–39 years, (iii) age 40–49 years, (iv) age 50–59 years and two other categories. Additional categories included (v) all persons older than 15 but younger than 20 and (vi) all persons over 60. The years of education varied between 0 and 22 years (M = 13.9; SD = 2.7) and were grouped into five lengths of education corresponding to natural divisions of the Indonesian education system: (i) educated for less than seven years, (ii) education between 7 and 9 years, (iii) education between 10 and 12 years, (iv) education between 13 and 16 years and (v) education over 17 years.
The tests were administered in “Bahasa Indonesia,” the official language of Indonesia that is used nationwide in the educational system, in media, administration and business. Before the test was administered, researchers explained all study procedures and informed the participants that the data were to be used for scientific purposes. Then participants gave their consent to participate in the study. They received seventy-five thousand rupiahs (equal to five US dollars) after finishing the series of tests. The I-BNT was one of the tests administered within the framework of a larger project in which nine other neuropsychological tests were adapted and data from healthy subjects were collected. All included participants with no reported history of psychiatric, neurological diseases, head trauma, drug abuse, or other illnesses that could influence test’ performance. The current research was conducted in compliance with the Helsinki Declaration and the ethics committee of Soegijapranata University gave clearance for this research project (University Ethical Clearance number: 001B/B.7.5/FP.KEP/IV/2018). The design of the database and the transport and storage of private, sensitive information fulfills Indonesia’s regulations as mentioned in ITE Law (Information and Electronic Transactions).
Measures
The BNT, introduced by Kaplan et al. (Citation1983), consists of 60-line drawings graded on levels of difficulty (Alyahya & Druks, Citation2016; Kessel & Hendriks, Citation2016). Subjects have to report the name of the object in the drawing within a limited period of time. The I-BNT was administered using the original procedure of the BNT (Kaplan et al., Citation1983). The response time per item was restricted to 20s. Scores include the number of spontaneously produced correct responses, the number of correct responses given after semantic cueing, and the number of responses given after phonemic cueing. The total number of correct responses is the sum of the number of spontaneous correct responses and the number of correct responses after a stimulus cue is given (Strauss et al., Citation2006; Sulastri et al., Citation2019). In addition, the researcher or test administrator also noted the number of incorrect answers and measured the total time to complete the sixty-item test.
Statistical analysis
An analysis of variance (ANOVA) was used to find the effects of the demographic characteristics, of sex, age, and education on the total scores and total time of the I-BNT. Post-hoc analysis, according to Bonferroni, was used to further delineate main and interaction effects. Uni- and multivariate linear regression analyses were used to obtain the factors and coefficients of the prediction model and formulae for calculating the predicted score; these will be used only when the number of subjects in a chosen reference group is fewer than 50.
Results
provides information about the participants who completed the I-BNT and that were included in the database. The table presents the number of subjects in each age and education category, as well as the distribution of sexes in our current sample.
Table 1. I-BNT Participant demographics (N=492).
shows the effects of age, education and sex on the I-BNT total score and total time. Total score and total time were significantly influenced by age and education as revealed by the outcomes of the three-factor ANOVA (see also ). There was no main effect for sex; only first and second-order interactions between sex and the other factors were found with small effect sizes (Cohen, Citation1988). Therefore, sex was no longer considered as a major factor affecting the performance of the I-BNT; this might be subject to change as more data are collected, or it may be found to differ for different ethnic groups.
Figure 2. Mean and standard deviations of the I-BNT total score. The left side (2a) is education factor effect. Education on X-axis (elementary schools (0–6) to post-graduate (17++)). The graph shows an education-dependent increase, except for the 17++ group. The larger standard deviation in the 0–6 and 17++ groups is indicative of a relatively small number of subjects in combination with large inter-individual differences. The right side (2b) is age factor effect. Age groups are illustrated on the X-axis. Note the small and non-significant age-related effects between the groups <60 years; only the oldest group (≥60) showed a significant decline.
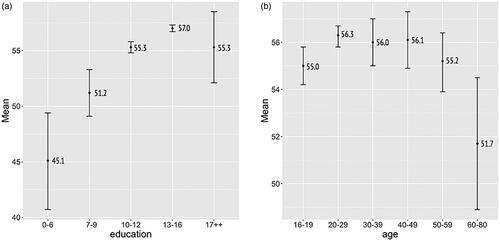
Table 2. Analysis of variance for main effects and interactions between age, education, and sex on the total score and total time.
The effects of demographic factors on the I-BNT total score and total time
The outcomes of the ANOVA showed that education is indeed significant for the total number correct (F (4.446) = 37.23, p < .001, ή2 = .25; ). The effect size was medium (Cohen, Citation1988), 25% of the total variance can be explained by education. shows the means and standard deviations for the different education categories. The highest number of correct items was achieved by people with 13–16 years of education, the lowest score by people in the group with less than 7 years of education. The post-hoc tests showed that all education groups differed from each other on the total number correct, the least educated group scoring significantly lower than other groups. The time to complete the I-BNT (total time) also showed a significant education effect (see ). The fastest was the group that received 13–16 years of education (M = 262.7). The slowest were persons with the lowest education (M = 611.2).
shows the mean (represented by a closed circle) and standard deviation (represented by a vertical line) of the total score based on different age groups; the highest score (56.70) was obtained in the 20–29 years group. The lowest score (51.70), comprised the ≥60 years age group. The main effect of age turned out to be rather small (Cohen, Citation1988) but significant (F (5,446) = 2.32, p < .05, ή2 = .03, ); only 3% of the variance in the total correct number can be ascribed to age. The post-hoc tests showed that all age groups scored better compared to the ≥60 years group. The post-hoc tests for age on the total time shows that the group ≥60 did less well in total time compared with others (M = 431.6). The fastest was the 40–49 years old group (M = 312.6). As mentioned earlier, the system allows the creation of a subset based on more than one demographic factor. The significant main effects, and to a lesser extent the first and second-order interaction effects, as given in , albeit with ή2’s smaller than 7%, already suggested that this might be useful. The second-order interaction’s interpretation is that the effects of sex might be different in the different age and education groups. The interaction between age and education (ή2 = .06) suggests that age effects on the total score of the I-BNT might be different for different education groups. The main and first-order interaction effects on both total number of correct items and on time to complete the test underline the importance of using fine-tuned normative scores for the I-BNT.
The usefulness of different reference groups illustrated by simulation
As previously demonstrated, the demographic factors age and years of education significantly influence the number of correct I-BNT items. These factors have consequences for the comparisons of an individual’s score with the reference group. The user of the online I-ANDI platform enters a client’s demographic data and the raw I-BNT score into the system, and chooses the filters or factors in order to position his client’s score in comparison with the optimally defined normative group. The output of the system is in the form of a graph. The graph shows a person’s raw and z-score score relative to the reference group score as well as the number of subjects (dataset) used for the calculation of the normative score.
The usefulness of I-ANDI, including the choice of different reference groups with different normative scores, is illustrated with the following simulation examples: a score of a single person aged = 68 years with two variants regarding the level of education, either only elementary school (6 years) or senior high (12 years), and I-BNT score of 48.
The results show, as can be seen in , how the client’s z-score relates to the by I-ANDI calculated scores of the clinician’s chosen or preferred reference group. Noticeable differences between the client’s score in relation to the different reference groups can be clearly seen.
Figure 3. An illustration of the internet platform for obtaining client scores concerning different reference groups. The displayed plots are an individual’s standardized z-score compared to (a) all data, (b) education in a 12-year subset, (c) education in a 6-year subset, (d) education in 6 years and ages above 60 years subset. In case the number of subjects is lower than 50, as in , the user is advised to use the predicted normative score. Next, the number of subjects used for the calculation of the normative score is plotted on top of each graph.
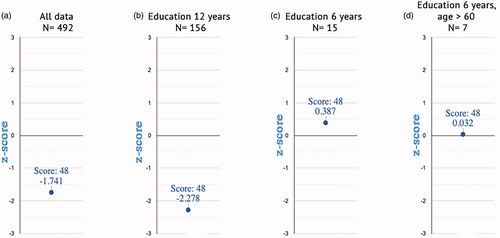
illustrates that the relative standing of a client is highly dependent on the selected reference group. The vertical Y-axes in show the standardized performance scale (z-score) with zero as the mean of the intended reference groups. The numbers 1 and −1, 2 and −2, also 3 and −3 on the Y-axes indicate 1 to 3 standard deviations (SD) from the mean value. From left to right are the score of a client compared to (a) all data in the database (no filters), (b) 12 years of education, (c) 6 years of education (note the large effects of education), and (d) his age (>60) and 6 years of education.
Regression analyses
The data from all subjects were used for hierarchical multiple linear regression analyses in order to identify the contribution of the demographic factors of education, sex, and age, to both the total score and the total time of the I-BNT. Subsequently, a linear model was proposed to predict the normative scores for both variables. The beta weights and its significance scores are presented in and . Two models were analyzed. The first model considered only the education factor and was highly significant: it predicted total scores, F (1,490) = 138.50, p < .001 and total time, F (1,490) = 98.17, p < .001). The R2 were 22% and 17% respectively. Both values show a medium effect size by Cohen’s (Citation1988) standards. This simplest model essentially shows that total score and total time can be predicted by identifying the client’s education level. When the age and sex variables were added to the analysis model of total score and total time, there were no changes of R2 ( and ). This agrees with the reported large ή2 for education and the small ή2 for both variables in the 3-factor ANOVA, as reported in .
Table 3. Hierarchical multiple linear regression analysis predicting I-BNT total score for age, sex, and education (N = 492).
Table 4. Hierarchical multiple linear regression analysis predicting I-BNT total time for age, sex, and education (N = 492).
From the model, the equation of the total score and total time model using education-category (1–5) are:
(1)
(1)
(2)
(2)
The equations for the standardized z-score of the total score and total time are the same:
(3)
(3)
Discussion
The collection of normative scores for any psychological test requires substantial effort, and standardized procedures of data collection. Most psychological tests have been developed in WEIRD-specific (Western, Educated, Industrialized, Rich, and Democratic) cultures (Henrich et al., Citation2010) and monolingual societies. There exists a substantial likelihood that such cognitive tests are not culture free. Additionally, there may be changes in the norm-group demographics as well as changes in health across time that require recently collected data (De Vent et al., Citation2016). The effort to adapt tests to the Indonesian culture and to collect normative data based on the Indonesian population is a relatively recent development. Such efforts are hindered by publishers who claim copyrights, even when the normative scores are collected in another language, a different culture or time periods. Furthermore, middle and low-income countries often are unable to afford academic databases with neuropsychological norm scores. As a consequence, the most important stakeholders (in this case Indonesian neuropsychologists) are then unable to obtain recent and local normative scores by this means.
Another reason for a dynamic database and an internet platform that provides users the choice of fine-tuned reference data is Indonesia’s tremendous cultural and linguistic diversity. This island nation has approximately 600 ethnic groups living on over 17,000 islands, speaking more than 700 native languages and no less than 1,100 dialects (Zein, Citation2020). To say that Indonesia is linguistically diverse is a huge understatement. Although Indonesia’s official language, Bahasa Indonesia, is taught to all children in elementary school, it is not spoken daily by all Indonesians. This variety of languages and ethnic groups makes it even more important to find ways to customize normative scores in a manner that will take this large linguistic and ethnic diversity into account.
The current dataset is only a first step in this direction. We have been able to consider only the three demographic factors that are most often used in international studies: the factors of sex, age and educational level. A great deal remains to be done to further fine-tune the reference data set to more adequately reflect Indonesia’s tremendous linguistic and cultural diversity.
Here we have demonstrated an online, continually evolving data base that provides for the determination and storage of normative scores, and the calculation of a client’s score based on a customized, fined-tuned reference group. The advantages of our platform and dynamic database are that: (a) it has the possibility to obtain reference scores from a sub-dataset, (b) the normative data can be updated toward only recently collected data, (c) it has a procedure to obtain standardized Z
-scores from all data or sub-datasets, and (d) it will allow the data base to grow. It will also offer possibilities for research into the influence that cultural, ethnic and language differences have on the cognitive domains. It will someday truly reflect the tremendous cultural, ethnic and language diversity of the Indonesian people.
However, the development of the normative database demands attention to certain issues. Besides a good and solid infrastructure and a good user-friendly interface, continuous control of the quality of the newly entered data is imperative. Currently the data comes only from the consortium with a quality check, including the outlier test on impossible scores and coding errors, as mentioned in the Methods section. In the future, when the system is fully operational and open for all registered (neuro)psychologists, it will include certain additional quality control procedures. Additional checks on the completeness of all submitted demographic data will be put in place and all neuropsychological tests will be based on appropriate and updated prediction models based on multivariate regression analyses. Only then will neuropsychologists be able to donate data from healthy and/or neuropsychological patients. Whenever there is uncertainty about the quality or the appropriateness of the data, the neuropsychologists of the consortium will decide whether or not to admit the new data to the existing dataset of healthy subjects. A final security measure that simply traces the login history of the system-users will also be added to the log-in monitoring system.
Furthermore, the database can provide a mix of neuropsychological test data. In addition to individual test scores, the database can be extended to provide combinations of test data. It can retrieve cognitive profiles rather than outcomes of just one single test. Finally, the dynamic database can also be used for participants with a clinical diagnosis; the data from these participants will be separated and excluded from the normative sample. The data from various patient groups will be used for research purposes only.
The dynamic database and internet platform facilitate the availability of more reliable normative scores since the user can (through the online platform) define the reference or norm group by choosing different demographic filters (factors). The current system’s version has four filters, and this number can be increased if necessary. The system can dynamically determine the normative score based on the user’s requirements by directly comparing an individual’s score results with normative scores from people who are in the same demographic categories. The same procedures can be applied to data from other neuropsychological tests. We collected normative data from some clinically relevant neuropsychological tests from different areas in Java and also from three other islands which will all be uploaded in the dynamic database as well. The more data that is uploaded, the more relevant the system will be. This includes the possibility to establish the contributing role of linguistic proficiency and type of ethnic group, for example. If these latter factors turn out to explain a statistically determined amount of variance, then the prediction models need to be adapted. It is worth noting that this dynamic database can also serve as an interesting research tool as it contains data from healthy controls’ various cognitive domains since the same tests were administered to all subjects.
The scores that we obtained for the I-BNT agreed well with our recently reported data (Sulastri et al., Citation2019). We found significant and large effects of education on the total score and total time of the I-BNT: the lowest mean score (45) was generated by the group with the lowest level of education. Education’s effects were rather large; other studies also conclude that educational background accounted for the greatest proportion of the variance in BNT data and more variance than age does (Henderson et al., Citation1998; Neils et al., Citation1995; Nicholas et al., Citation1989; Steinberg et al., Citation2005). In the current study, sex effects were not found, and this corresponds with data and conclusions from prior studies (Busch et al., Citation2006). The rather large effects of education and the small effects of age, as found in this study, contribute to the validity of the adapted version of the I-BNT, although a further clinical validation study is necessary with patients with mild forms of dementia or aphasia. Education’s effects underline the importance of having separate normative scores for subjects with different levels of education. The age effects imply different normative scores only for people over 60 years. On the other hand, the first and second order interactions between the three demographic factors suggest that normative data for subgroups are to be preferred, perhaps not now, but in the future when more data will be uploaded in the database. This is illustrated as well by our simulation: large differences in the standardized z-scores were obtained when the score of an individual was compared to the entire dataset or compared with groups with two different levels of education or an age-subgroup with lower levels of education. The z-scores varied from −2.28 to .387 and mainly the level of education showed large effects in the simulation, similar to the relatively large effect size for level of education in the ANOVA. The simulation also shows that the system can be used in a flexible way in determining an individual's score in comparison with this reference group.
A note of caution is that the data presented in this paper should not be considered as normative scores. Normative data for the I-BNT were recently published (Sulastri et al., Citation2019), but it should be noted that in the published analysis only some of the cells were filled with a sufficient number of respondents (Bridges & Holler, Citation2007), and other cells were less or poorly filled. The same issue is present here as well, and this pertains to mostly young persons with less than seven years of education, to persons with the highest level of education, and elderly people in general. Moreover, our current sample may not be representative for the entire Indonesian population, perhaps only for the better-educated population in urbanized parts of Java island. It is imperative that clinicians should consider the characteristics of the normative data, including the representativeness of the sample and the sample size of individual cells in the published normative dataset when they interpret the client’s score to avoid biases (Agelink van Rentergem et al., Citation2017).
In our case most of the cells do not have enough subjects and therefore the option was created to have predicted normative scores based on a statistical prediction model. The use of predicted normative scores may have some benefits (Burggraaff et al., Citation2017) and can be recommended in case the scores of the test are not normally distributed, as is the case for total correct scores of the I-BNT, or when the sample is not fully representative of the whole population. Therefore, the present work should be considered only as an illustrative example regarding the creation and advantages of the dynamic database and the online platform with an extension to predict normative scores; not as a paper presenting normative scores for the Indonesian population at large. In the future, it is expected that more data from other islands and ethnic groups will be added to the database allowing for a better quality or more fine-tuned reference groups. More definitive normative scores for the I-BNT and other tests await a more complete dataset or more efficient norming procedure such as regression-based norming generated from a larger variety of demographic factors (Van Breukelen & Vlaeyen, Citation2005), including parental ethnicity.
Another issue, typical for verbal tests such as the BNT, is that linguistic proficiency in Bahasa, the daily spoken language, and the mother language might play a role in the test performance and consequently influence the normative data. This has not been explored as yet. If our database grows to include a larger variety of subjects, it will allow us to investigate the effects of daily spoken language as this demographic factor is included and stored in the database.
We would like to close with a general reminder against the usage of tests (including the I-BNT) imported from WEIRD and monolingual societies. The presence of normative data for a specific test, does not mean that this test is sufficiently valid in the Indonesian context. Therefore, research toward the validity of the I-BNT remains important. A general remark is that the use of tests from monolingual countries should be discouraged until their full psychometric properties have been explored in the Indonesian context including the role of ethnicity, mother and daily languages spoken. Currently, the test-retest reliability of the whole test battery is being explored, including those of the I-BNT. The preliminary outcome based on a sample of 50 healthy subjects varying in age between 21–64 and 12–22 years of education showed an excellent test-retest reliability for both the total score (.88) and total time (.84); data from other islands were recently collected in order to generate a better applicability to the Indonesian context and they await further analyses. Research to determine the validity of the different tests in the battery, which is considered as their clinical utility (clinical validation), is also foreseen. The data of the I-BNT has established rather large effects of education suggesting a sensitivity for this factor similar to that reported in other cultures and societies. However, until complete psychometrics for the different tests are available, caution is advised in the use and interpretation of these tests.
In all, I-ANDI was developed to assist neuropsychologists. It consists of a dynamic database and an online platform which makes it easy to find fined-tuned normative score based on the user’s chosen demographic factor(s). The significant main and interaction effects, and the outcomes of the simulation, strongly support the use of fine-tuned reference data for the I-BNT. The system provides the capability of calculating the predicted score when the number of subjects in the selected reference dataset is insufficient. An individual’s neuropsychological test performance is shown in the form of informative graphics. In the near future, data from other neuropsychological tests adapted for Indonesian subjects will be included in the database, as well as data from other parts (islands) of Indonesia to better reflect Indonesian’s ethnic, and linguistic diversity. It should be noted that the values of the new instruments increase following their usage, and that internet-enabled methods to compile normative data may help clinicians to efficiently characterize their patient’s neuropsychological test scores.
Acknowledgement
The authors are indebted to Dr. Nathalie De Vent and her team from the University of Amsterdam for introducing Dutch ANDI and providing help with the code. We also thanked collaborating researchers from Radboud University, Nijmegen (Dr M.P.H. Hendriks) for advise throughout the initiating phase of the project, Soegijapranata Catholic University (Dr. Margaretha Sih Setija Utami, M.Kes., Drs. Haryo Guritno, M.Si., Lucia Trisni Widianingtanti, S.Psi., M.Si., CVR Abimanyu, S.Psi., M.Si), Atma Jaya Catholic University of Indonesia (Dr. Angela Oktavia Suryani M.Si., Justinus Budi Santoso, M.Psi., Psikolog, Dr. Magdalena Surjaningsih Halim, Psikolog, Dr. Yohana Ratrin Hestyanti, Psikolog) and Widya Mandala Catholic University of Surabaya (Florentina Yuni Apsari, S.Psi., M.Si., Psikolog), and the assistants who helped collecting the data. Dr. Frans van Haaren and Dr. John V. Keller helped with linguistic corrections.
Disclosure statement
No potential conflict of interest was reported by the author(s).
Additional information
Funding
References
- Agelink van Rentergem, J. A., Murre, J. M. J., & Huizenga, H. M. (2017). Multivariate normative comparisons using an aggregated database. PLoS One, 12(3), e0173218. https://doi.org/10.1371/journal.pone.0173218
- Alyahya, R. S. W., & Druks, J. (2016). The adaptation of the Object and Action Naming Battery into Saudi Arabic. Aphasiology, 30(4), 463–482. https://doi.org/10.1080/02687038.2015.1070947
- Bridges, A. J., & Holler, K. A. (2007). How many is enough? Determining optimal sample sizes for normative studies in pediatric neuropsychology. Child Neuropsychol, 13(6), 528–538. https://doi.org/10.1080/09297040701233875
- Burggraaff, J., Knol, D. L., & Uitdehaag, B. M. J. (2017). Regression-based norms for the symbol digit modalities test in the dutch population: Improving detection of cognitive impairment in multiple sclerosis? European Neurology, 77(5–6), 246–252. https://doi.org/10.1159/000464405
- Busch, R. M., Chelune, G. J., & Suchy, Y. (2006). Using norms in neuropsychological assessment of the elderly. In D. K. Attix & K. A. Welsh-Bohmer (Eds.), Geriatric neuropsychology: Assessment and intervention (p. 133–157). Guilford Publications.
- Casaletto, K. B., & Heaton, R. K. (2017). Neuropsychological assessment: Past and future. Journal of the International Neuropsychological Society, 23(9–10), 778–790. https://doi.org/10.1017/S1355617717001060
- Cohen, J. (1988). Statistical power analysis for the behavioral sciences. Routledge Academic.
- De Vent, N. R., Agelink van Rentergem, J. A., Schmand, B. A., Murre, J. M. J., & Huizenga, H. M. (2016). Advanced Neuropsychological Diagnostics Infrastructure (ANDI): A normative database created from control datasets. Frontiers in Psychology, 7, 1601. https://doi.org/10.3389/fpsyg.2016.01601
- Henderson, L. W., Frank, E. M., Pigatt, T., Abramson, R. K., & Houston, M. (1998). Race, gender, and Educational level effects on Boston Naming Test Scores. Aphasiology, 12(10), 901–911. https://doi.org/10.1080/02687039808249458
- Henrich, J., Heine, S., & Norenzayan, A. (2010). The weirdest people in the world? Behavioral and Brain Sciences, 33 (2–3), 61–83. https://doi.org/10.1017/S0140525X0999152X
- Kaplan, E., Goodglass, H., & Weintraub, S. (1983). The Boston Naming Test. Lea & Febiger.
- Kessel, R. P. C., & Hendriks, M. P. H. (2016). Neuropsychological assessment. Encyclopedia of Mental Health, 2nd Edition, 3, 197–201. https://doi.org/10.1016/B978-0-12-397045-9.00136-1
- Meyers, J. E. (2013). The Meyers neuropsychological system. Retrieved from http://www.meyersneuropsychological.com
- Neils, J., Baris, J. M., Carter, C., Dell’aira, A. L., Nordloh, S. J., Weiler, E., & Weisiger, B. (1995). Effects of age, education, and living environment on Boston naming test performance. Journal of Speech and Hearing Research, 38(5), 1143–1149. https://doi.org/10.1044/jshr.3805.1143
- Nicholas, L. E., Brookshire, R. H., MacLennan, D. L., Schumacher, J. G., & Porrazzo, S. A. (1989). Revised administration and scoring procedures for the Boston Naming test and norms for non-brain-damaged adults. Aphasiology, 3(6), 569–580. https://doi.org/10.1080/02687038908249023
- Oosterhuis, H. E., van der Ark, L. A., & Sijtsma, K. (2016). Sample size requirements for traditional and regression-based norms. Assessment, 23(2), 191–202. https://doi.org/10.1177/1073191115580638
- Palmer, B. W., Boone, K. B., Lesser, I. M., & Wohl, M. A. (1998). Base rates of “impaired” neuropsychological test performance among healthy older adults. Archives of Clinical Neuropsychology, 13(6), 503–511.
- Shirk, S. D., Mitchell, M. B., Shaughnessy, L. W., Sherman, J., Locascio, J., Weintraub, S., & Atri, A. (2011). A web-based normative calculator for the uniform data set (UDS) neuropsychological test battery. Alzheimer’s Research & Therapy, 3(6), 32. https://doi.org/10.1186/alzrt94
- Steinberg, B. A., Bieliauskas, L. A., Smith, G. E., Langellotti, C., & Ivnik, R. J. (2005). Mayo’s older americans normative studies: Age- and IQ-adjusted norms for the Boston Naming Test, the MAE token test, and the judgment of line orientation test. The Clinical Neuropsychologist, 19(3–4), 280–328. https://doi.org/10.1080/13854040590945229
- Strauss, E., Sherman, E. M. S., & Spreen, O. (2006). A compendium of neuropsychological tests: Administration, norms, and commentary, applied neuropsychology. Oxford University Press. 14(1), 62–63. https://doi.org/10.1080/09084280701280502
- Sulastri, A., Utami, M. S. S., Jongsma, M., Hendriks, M., & van Luijtelaar, G. (2019). The Indonesian Boston Naming Test: Normative data among healthy adults and effects of age and education on naming ability. International Journal of Science and Research, 8(11), 134–139.
- Tombaugh, T. N. (2004). Trail Making Test A and B: Normative data stratified by age and education. Archives of Clinical Neuropsychology, 19(2), 203–214. https://doi.org/10.1016/S0887-6177(03)00039-8
- Van Breukelen, G. J., & Vlaeyen, J. W. (2005). Norming clinical questionnaires with multiple regression: The Pain Cognition List. Psychological Assessment, 17(3), 336–344. https://doi.org/10.1037/1040-3590.17.3.336
- Van Den Berg, E., Nys, G. M. S., Brands, A. M. A., Ruis, C., van Zandvoort, M. J. E., & Kessels, R. P. C. (2009). The Brixton Spatial Anticipation Test as a test for executive function: Validity in patient groups and norms for older adults. Journal of the International Neuropsychological Society, 15(5), 695–703. https://doi.org/10.1017/S1355617709990269
- Weintraub, S., Salmon, D., Mercaldo, N., Ferris, S., Graff-Radford, N. R., Chui, H., Cummings, J., DeCarli, C., Foster, N. L., Galasko, D., Peskind, E., Dietrich, W., Beekly, D. L., Kukull, W. A., & Morris, J. C. (2009). The Alzheimer’s Disease Centers’ Uniform Data Set (UDS): The neuropsychologic test battery. Alzheimer Disease and Associated Disorders, 23(2), 91–101. https://doi.org/10.1097/WAD.0b013e318191c7dd
- Zein, S. (2020). Language policy in superdiverse Indonesia (1st ed). Routledge.
- Zucchella, C., Federico, A., Martini, A., Tinazzi, M., Bartolo, M., & Stefano, T. (2018). Neuropsychological testing. Practical Neurology, 18(3), 227–237. https://doi.org/10.1136/practneurol-2017-001743