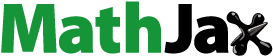
Abstract
The Minimum Risk Equivariant (MRE), estimator is a widely used estimator which has several well-known theoretical and practical properties. It is well known that for the square error and absolute error loss functions, the MRE estimator is a generalized Bayes estimator. This article investigates the potential Bayesianity (or generalized Bayesianity) of the MRE estimator under a general convex and invariant loss function, for estimating the location and scale parameters of an unimodal density function.
Public Interest Statement
This article investigates the potential Bayesianity (or generalized Bayesianity) of the MRE estimator under a general convex and invariant loss function, for estimating the location and scale parameters of an unimodal density function.
1. Introduction
Compare with the uniform minimum variance unbiased estimator, the Minimum Risk Equivariant (MRE) estimator: (1) typically exists not only for convex loss function but also even for non-restricted loss functions and (2) does not need to consider randomized estimators (Lehmann & Casella, Citation1998, p. 156). Moreover, the MRE estimator is a widely used estimator which has several well-known theoretical (such as minimaxity and admissibility under some certain conditions) and practical properties. The MRE estimator has a wide range of applications in Finite sampling framework (Chandrasekar & Sajesh, Citation2013; Ledoit & Wolf, Citation2013), Reliability (Chandrasekar & Sajesh, Citation2013; Wei, Song, Yan, & Mao, Citation2000), Regression and non-linear models (Grafarend, Citation2006; Hallin & Jurečková, Citation2012), Contingency tables (Lehmann & Casella, Citation1998), Economic Forecasting (Elliott & Timmermann, Citation2013), etc.
It is well known that for the square error and the absolute error loss functions, the MRE estimator is a generalized Bayes estimator. This article extends this fact to a class of convex and invariant loss functions for unimodal location (or scale) density functions. This fact has been proven remarkably useful in solving a variety of problems in statistics. Subjects for which the problem is applicable range from truncated data (Manrique-Vallier & Reiter, Citation2014), imputation omitted data due to undercounting (Rubin, Gelman, & Meng, Citation2004, §12), Capture–recapture Estimation (Mitchell, Citation2014), etc.
The problem of finding a prior distribution that its corresponding Bayes estimator under a given loss function coincides with a given estimator started by Lehmann (Citation1951). In his seminal paper, he considered a situation that a Bayes estimator under the squared error loss function is an unbiased estimator. His work has been followed and extended by several authors. For instance, Noorbaloochi and Meeden (Citation1983, Citation2000) expanded Lehmann’s (Citation1951) finding for a general class of prior distributions. Kass and Wasserman (Citation1996) reviewed the problem of selecting prior distributions that their corresponding Bayes estimators are invariance under some sort of transforms. Meng and Zaslavsky (Citation2002) considered a class of single observation unbiased priors (i.e. such priors produced unbiased Bayes estimator under squared error loss function). They showed that under mild regularity conditions, such class of priors must be “noninformative” for estimating either location or scale parameters. Gelman (Citation2006) constructed a non-central t student family of conditionally conjugate priors for hierarchical standard deviation parameters. For restricted parameter space, Kucerovsky, Marchand, Payandeh, and Strawderman (Citation2009) provided a class of prior distributions which their corresponding Bayes estimator under absolute value error loss equal to the maximum likelihood estimator. Ma and Leijon (Citation2011) found a conjugate beta mixture prior such that its corresponding Bayes estimator under the variational inference framework retains some given properties.
This paper provides a class of prior distributions that their corresponding Bayes estimator under general convex and invariant loss function coincides with the MRE estimator for location or scale family of distributions.
Section 2 collects some required elements for other sections. The problem of finding such prior distribution for location and scale parameters have been studied in Sections 3 and 4, respectively.
2. Preliminaries
Bayes estimator for an unknown parameter under a general loss function
has been evaluated from the posterior distribution
. Therefore, to study some specific properties of a Bayes estimator, one has to study posterior distribution
. The following provides a condition which leads to equivalent Bayes estimator under two prior distributions.
Lemma 1
Suppose is a continuous random variable with density function
. Moreover, suppose that
and
are two priors distributions which lead to Bayes estimators
and
under a general loss function
respectively. Then, two Bayes estimators
and
are equivalent estimator (i.e.
) if and only if
for all
.
Proof
Bayes estimators with respect to and
are equivalent if and only if the posterior distribution
under these priors are equivalent, i.e.
The rest of proof arrives from the fact that left hand side of the above equation is a function of while the right hand side is a function of
.
The following from Marchand and Payandeh (Citation2011) recalls the Bayes estimator under generalized loss function for location parameter
.
Lemma 2
(Marchand & Payandeh, Citation2011) Suppose random variable sampled from a location density function
Moreover, suppose that
stands for the Bayes estimator under generalized loss function
and prior distribution
for location parameter
Then,
satisfies
(1)
(1)
Now, we extend the above result for the problem of finding a Bayes estimator for a scale parameter under general loss function
and prior distribution
Lemma 3
Suppose random variable sampled from a scale density function
Moreover, suppose
stands for the Bayes estimator under generalized loss function
and prior distribution
for a scale parameter
Then,
satisfies
(2)
(2)
where
Proof
Marchand and Strawderman (Citation2005) provided a connection between a scale parameter estimation problem, with elements and a location parameter estimation problem with elements
They showed that by choosing
,
,
, and
transformations the problem of finding a Bayes estimator for a scale parameter
under general loss function
and prior distribution
can be restated as a problem of finding a Bayes estimator for location parameter
under general loss function
and prior distribution
Moreover, they showed that the Bayes estimator
of a location parameter
with respect to prior
and the Bayes estimator
of a scale parameter
with respect to prior
satisfies
and
The desired proof arrives from the above observations.
The Fourier transform is an integral transform which defines for an integrable and real-valued by
(3)
(3)
The convolution theorem for the Fourier transforms states that(4)
(4) (see Dym & Mckean, Citation1972 for more details).
Now, we recall definition and some properties of exponential type functions which are used later in proof of Theorem 4.
Definition 1
A function in
is said to be an exponential type
function on the domain
if there are positive constants
and
such that
for
The Paley–Wiener theorem states that the Fourier (or inverse Fourier) transform of an function vanishes outside of an interval
if and only if the function is an exponential type
(Dym & McKean, Citation1972, p. 158). An exponential type function is a continuous function which is infinitely differentiable everywhere and has a Taylor series expansion over every interval (see Champeney, Citation1987, p. 77; Walnut, Citation2002, p. 81). The exponential type functions are also called band-limited functions (see Bracewell, Citation2000, p. 119 for more details).
3. Bayesianity of the MRE estimator for location family of distributions
Suppose has distribution
with respect to Lebesgue density function
where
is known and unknown location parameter
is to be estimated by decision rule
under equivalent loss function
An estimator (decision rule)
of
is location invariant if and only if
for all
The MRE estimator is an estimator which has the smallest risk among all invariant estimators (Shao, Citation2003). It is well known that the MRE estimator for
based upon one observation is
where the value of
will minimize
, or for smooth
satisfies
(Lehmann & Casella, Citation1998).
Now, we consider the problem of finding prior distribution that its corresponding Bayes estimator
under general convex and invariant loss function
coincides with the MRE estimator
i.e.
(5)
(5)
The following studies possible solution of Equation 5 under the absolute value loss function.
Theorem 1
Suppose is a continuous random variable with location density function
Then, the Bayes estimator under the absolute value loss function and prior
coincides with the MRE estimator
whenever
for
Proof
Under the absolute value loss function and prior is a Bayes estimator if and only if
Letting and
The above equation can be restated as
An application of the Fourier transform along with the convolution theorem (Equation 4), then, takeing the Fourier transform back lead to
where and
stand for delta direct function and the Fourier and inverse Fourier transforms, respectively. The desired proof arrives from an application of Lemma 1.
The following theorem using Lemma 2 extends results of Theorem 1 to a general convex and invariant loss function
Theorem 2
Suppose is a continuous random variable with location density function
Moreover, suppose that
stands for a convex and invariant loss function. Then, under prior
the MRE estimator
is a Bayes estimator for a location parameter
Proof
Lemma 2 states that the Bayes estimate satisfies the equation
, for all
Setting
one can reduce solving equation
in
to
where The last equation arrives from that fact that there exist a delta direct function in the nominator of the function insides of the inverse Fourier transform. An application of Lemma 1 warranties that, if there is another prior distribution
such that its corresponding Bayes estimator
Then,
for all
The following two propositions verify findings of Theorem 2 for two class of loss functions.
The MRE estimator for normal distribution under the LINEX loss function is
The following proposition studies Bayes ianity of such MRE estimator.
Proposition 1
Suppose is a random variable which distributed according to a normal distribution with mean
and variance
Then, the Bayes estimator for
with respect to prior distribution
and under the LINEX loss function
coincides with the MRE estimator
Proof
The Bayes estimate, say with respect to prior
and under loss function
finds out by
Setting
Theorem 2 reduces solving equation
in
to
where constance should be chosen such that
for all
The following extends the above result to a convex combination of two LINEX loss functions, say
Proposition 2
Suppose is a random variable which distributed according to a normal distribution with mean
and variance
Then, the Bayes estimator for
with respect to prior distribution
and under convex combination of two LINEX loss functions
coincides with the MRE estimator
Proof
Setting along with result of Theorem 2, one may reduce solving equation
in
to
where and constance
should be chosen such that
for all
The second equality arrives from the fact that
and
4. Bayesianity of the MRE estimator for scale family of distributions
Suppose has distribution
with respect to Lebesgue density function
where
is known and unknown scale parameter
is to be estimated by decision rule
under equivalent loss function
An estimator (decision rule)
of
is scale invariant if and only if
for all
The MRE estimator is an estimator which has the smallest risk among all invariant estimators (Shao, Citation2003). It is well known that the MRE estimator for
based upon one observation is
where the value of
will minimize
, for smooth
satisfies
(Lehmann & Casella, Citation1998).
Now, we consider the problem of finding prior distribution that its corresponding Bayes estimator
under general convex and invariant loss function
coincides with the MRE estimator
i.e.
(6)
(6)
The following studies possible solution of Equation 6 under the absolute value loss function for symmetric-scale distribution functions (see Jafarpour & Farnoosh, Citation2005 for more details on symmetric-scale distribution functions).
Theorem 3
Suppose non-negative and continuous random variable distributed according to a scale density function
Moreover, suppose that
is symmetric about
Then, the Bayes estimator, under the absolute value loss function and prior distribution
coincides with the MRE estimator
Proof
Under the absolute value loss function, solving equation in
can be restated as
From the above equation, one may conclude that, a trivial solution of is to let
be free of
This observation along with an application of Lemma 1 complete the desired proof.
is an obvious example for such symmetric-scale distribution satisfies Theorem 3’s conditions. Since
an expression
does not depend on
Therefore,
is a MRE estimator (see Rohatgi & Saleh, Citation2011, p. 446 for more details).
The following theorem using Lemma 3 extends results of Theorem 3 to a general convex and invariant loss function
Theorem 4
Suppose non-negative and continuous random variable distributed according to a scale family distribution with density function
Then, Bayes estimator, under convex and invariant loss function
and prior distribution
coincides with the MRE estimator
Proof
Using Lemma 3, one may conclude that solving equation in
where
can be reduced to
where and in the second equality
and
Taking the Fourier transform from both sides along with an application of the convolution theorem, the above equation can be restated as
Positivity of arrives from the fact that
is an exponential type 1 function. Now, the Paley–Wiener theorem warranties that its corresponding inverse Fourier transform takes its values inside of an interval
The desired proof arrives by an application of Lemma 1.
It worthwhile mentioning that the above inverse Fourier transform may not evaluated analytically. Therefore, one has to employ some numerical approach, such as the fast Fourier transformation, to handel it.
5. Conclusion and suggestion
This paper provides a class of prior distributions which their corresponding Bayes estimator under general convex and invariant loss function coincides with the MRE estimator for location or scale family of distributions. This problem can be studied for scale-location family of distributions under general convex and invariant loss function.
Acknowledgements
Author would like to thank professor Xiao-Li Meng who introduced the problem and William Strawderman for his constrictive comments. Referees’ comments and suggestions are gratefully acknowledged by author.
Additional information
Funding
Notes on contributors
Amir T. Payandeh Najafabadi
Amir T. Payandeh Najafabadi is an Associate Professor in Department of Mathematics Sciences at Shahid Behashti University, Tehran, Evin. He was born on September 3, 1973. He received his PhD from University of New Brunswick, Canada in 2006. He has published 28 papers and was co-author of two books. His major research interests are: Statistical Decision Theory, Lévy processes, Risk theory, Riemann–Hilbert problem, & integral equations.
References
- Bracewell, R. N. (2000). The Fourier transform and its applications (3rd ed.). New York, NY: McGraw-Hill.
- Champeney, D. C. (1987). A handbook of Fourier theorems. New York, NY: Cambridge University Press.
- Chandrasekar, B., & Sajesh, T. A. (2013). Reliability measures of systems with location-scale ACBVE components. Theory & Applications, 28, 7–15.
- Dym, H., & Mckean, H. P. (1972). Fourier series and integrals. Probability and mathematical statistics. New York, NY: Academic Press.
- (2013). Elliott, G., & Timmermann, A. (Eds.). Handbook of economic forecasting (Vol. 2). New York, NY: Newnes.
- Gelman, A. (2006). Prior distributions for variance parameters in hierarchical models (comment on article by Browne and Draper). Bayesian Analysis, 1, 515–534.
- Grafarend, E. W. (2006). Linear and nonlinear models: Fixed effects, random effects, and mixed models. New York, NY: Walter de Gruyter.
- Hallin, M., & Jurečková, J. (2012). Equivariant estimation. Encyclopedia of environmetrics. New York, NY: Wiley.
- Jafarpour, H., & Farnoosh, R. (2005). Comparing the kurtosis measures for symmetric-scale distribution functions considering a new kurtosis. In Proceedings of the 8th WSEAS International Conference on Applied Mathematics (pp. 90–94). Tenerife: World Scientific and Engineering Academy and Society (WSEAS).
- Kass, R. E., & Wasserman, L. (1996). The selection of prior distributions by formal rules. Journal of the American Statistical Association, 91, 1343–1370.
- Kucerovsky, D., Marchand, É., Payandeh, A. T., & Strawderman, W. E. (2009). On the Bayesianity of maximum likelihood estimators of restricted location parameters under absolute value error loss. Statistics & Risk Modeling, 27, 145–168.
- Ledoit, O., & Wolf, M. (2013). Optimal estimation of a large-dimensional covariance matrix under Stein’s loss ( Working Paper No. 122). Zurich: University of Zurich Department of Economics.
- Lehmann, E. L. (1951). A general concept of unbiasedness. The Annals of Mathematical Statistics, 22, 587–592.
- Lehmann, E. L., & Casella, G. (1998). Theory of point estimation. New York, NY: Springer.
- Ma, Z., & Leijon, A. (2011). Bayesian estimation of beta mixture models with variational inference. IEEE Transactions on Pattern Analysis and Machine Intelligence, 33, 2160–2173.
- Manrique-Vallier, D., & Reiter, J. P. (2014). Bayesian estimation of discrete multivariate latent structure models with structural zeros. Journal of Computational and Graphical Statistics, 23, 1061–1079.
- Marchand, É., & Payandeh, A. T. (2011). Bayesian improvements of a MRE estimator of a bounded location parameter. Electronic Journal of Statistics, 5, 1495–1502.
- Marchand, É., & Strawderman, W. E. (2005). On improving on the minimum risk equivariant estimator of a scale parameter under a lower-bound constraint. Journal of Statistical Planning and Inference, 134, 90–101.
- Meng, X., & Zaslavsky, A. M. (2002). Single observation unbiased priors. The Annals of Statistics, 30, 1345–1375.
- Mitchell, S. A. (2014). Capture–recapture estimation for conflict data and hierarchical models for program impact evaluation ( PhD thesis), Harvard University Cambridge, Cambridge, MA.
- Noorbaloochi, S., & Meeden, G. (1983). Unbiasedness as the dual of being Bayes. Journal of the American Statistical Association, 78, 619–623.
- Noorbaloochi, S., & Meeden, G. (2000). Unbiasedness and Bayes estimators (Technical Report No. 9971331). University of Minnesota. Retrieved from http://users.stat.umn.edu/gmeeden/papers/bayunb.pdf
- Rohatgi, V. K., & Saleh, A. M. E. (2011). An introduction to probability and statistics (Vol. 910). New York, NY: Wiley.
- (2004). Rubin, D. B., & Gelman, A. (Eds.). (2004). Applied Bayesian modeling and causal inference from incomplete-data perspectives ( Vol. 561). New York, NY: Wiley.
- Shao, J. (2003). Mathematical statistics: Springer texts in statistics. New York, NY: Springer.
- Walnut, D. F. (2002). An introduction to wavelet analysis (2nd ed.). New York, NY: Birkhäuser Publisher.
- Wei, J., Song, B., Yan, W., & Mao, Z. (2011, June). Reliability estimations of Burr-XII distribution under Entropy loss function. In The 9th international Conference on IEEE Reliability, Maintainability and Safety (ICRMS) (pp. 244–247). Guiyang, China.