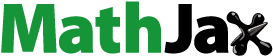
Abstract
Based on a classical true score theory (classical test theory, CTT) equation, indicating that as the observed correlation between two tests increases, the reliability of the difference scores decreases, researchers have concluded that difference scores are unreliable. But CTT shows that the reliabilities of the two tests and the true correlation between them influence the observed correlation and previous analyses have not taken the true correlation sufficiently into account. In turn, the reliability of difference scores depends on the interaction of the reliabilities of the individual tests and their true correlation when the variances of the tests are equal, and on a more complicated interaction between them and the deviation ratio when the variances of the tests are not equal. The upshot is that difference scores likely are more reliable, on more occasions, than researchers have realized. I show how researchers can predict what the reliability of the difference scores is likely to be, to aid in deciding whether to carry through one’s planned use of difference scores.
Public Interest Statement
There are many important issues that depend on difference scores for their elucidation. For example, to find out if an intervention worked, participants might be asked to complete a relevant measure before and after the intervention, with the hope that scores will be more favorable after it than before it. Or participants might complete two or more different measures, such as when high school students take tests in different subject areas to determine relative strengths and weaknesses. Whether the difference of interest pertains to change on a measure, or on the difference between different measures, difference scores are important. However, because of a prevalent belief that difference scores are not reliable, researchers have been discouraged from using them. The present work challenges the blanket assertion that difference scores are inherently unreliable and demonstrates the conditions under which different scores are more reliable or less reliable.
1. Introduction
Basic and applied researchers in education and psychology often have reason to be interested in the differences between people’s scores on two tests. The two tests might have the same items, as in a pretest–posttest design or in other types of within-participants designs. Alternatively, the tests might have different items, such as when a school psychologist identifies that a particular student has a relative weakness in one domain compared to a relative strength in another domain. In either case, difference scores—the difference between scores on two tests—are of interest. But for many decades, psychometric experts have warned that difference scores are not reliable. Bereiter (Citation1963, p. 3) stated the consequence of this warning with admirable clarity:
Although it is commonplace for research to be stymied by some difficulty in experimental methodology, there are really not many instances in the behavioral sciences of promising questions going unresearched because of deficiencies in statistical methodology. Questions dealing with psychological change may well constitute the most important exceptions. It is only in relation to such questions that the writer has ever heard colleagues admit to having abandoned major research objectives solely because the statistical problems seemed to be insurmountable.
Bereiter’s observation has retained its force, despite advances in the intervening decades. For example, Chiou and Spreng (Citation1996) noted that difference scores continue to be criticized. Even very recently, Thomas and Zumbo (Citation2012) stated, “There is such doubt in research practice about the reliability of difference scores that granting agencies, journal editors, reviewers, and committees of graduate students’ theses have been known to deplore their use” (p. 37). Given the expressed negativity throughout the decades, my goal is to investigate the alleged unreliability of difference scores in a better way than has hitherto been done. The stakes are large. If previous criticisms hold up, this would constitute an important reason to avoid doing research that depends on difference scores. In contrast, if previous criticisms do not hold up, an enormous impediment to research can be removed. I will show that the reliability of difference scores depends on the interaction between the true correlation between the tests, individual test reliabilities, and the deviation ratio, which is a ratio of test variances. A close study of this three-way interaction suggests that the blanket conclusion that difference scores are inherently unreliable is too pessimistic.
1.1. The basis of the criticism
The criticism that difference scores are unreliable comes directly from a theorem based on classical true score theory or classical test theory (CTT) that can be found in many places (e.g. Cronbach, Citation1990; Lord, Citation1963; see Gulliksen, Citation1987 for an excellent review of CTT theorems). CTT features the notion of reliability, which is usually defined as true score variance divided by observed score variance. Equation 1 provides the general formula that expresses the reliability of difference scores in terms of the reliabilities of the two tests
, the variances of the two tests
, and the correlation between observed scores on the two tests
(Designating a person’s score on one test as Xi and on the other as Yi implies that the difference score is di = Yi − Xi.).
(1)
(1)
If the variances of the two tests are equal, Equation 1 reduces to Equation 2 below.(2)
(2)
Under Equation 2, it is easy to see that as the correlation between the two tests increases, keeping the reliabilities of the two tests constant, the reliability of difference scores decreases. In the case of a pretest–posttest design, for example, one would expect the correlation between pretest and posttest to be substantial, and Equation 2 seems to indicate that this should result in unreliable difference scores. Historically, researchers with psychometric expertise have made similar arguments to militate against the reliability of difference scores.
However, despite widespread criticism, some have defended difference scores. For example, one problem with unreliable difference scores is that such unreliability might render it difficult to obtain statistically significant findings. After reviewing the literature on this criticism, Thomas and Zumbo (Citation2012) suggested that this sort of unreliability is not crucial for inferential statistical procedures, such as t-tests and ANOVAs (also see Gaito & Wiley, Citation1963). Possible limitations of this defense might be that this alleged lack of importance has not been demonstrated conclusively. Besides that, difference scores are used for many purposes other than significance tests. Suppose a researcher wishes to correlate difference scores with another variable. Or suppose a researcher wishes to base interventions on difference scores. In cases such as these, the unreliability of difference scores, if it holds up, would constitute an important problem, even if it were admitted that inferential statistics are not importantly affected.
Another defense involves a switch of focus from Equations 2 to 1. The idea here is that although difference scores are unreliable under the assumption of equal variances, if the variances are unequal, difference scores do not have to be unreliable. Chiou and Spreng (Citation1996) provided some examples of how the unreliability of difference scores is substantially mitigated by having sufficiently unequal variances of the two tests. Thus, their analysis suggested that a potential answer to the problem is to use tests with unequal variances. A limitation, however, is that it might be difficult to arrange matters in this way, particularly if the same test is given twice.
Another defense traces back to Bereiter (Citation1963), who pointed out that as the reliabilities of the individual tests increase, so does the reliability of the difference scores. This can be seen easily in Equation 2.
In his insightful analysis, Bereiter (Citation1963) pointed out that increasing the reliabilities of the individual tests has contradictory effects on the reliability of the difference scores. To understand these contradictory effects, it is necessary to recall that as the reliability of the tests increase, so does the observed correlation. Therefore, on the one hand, increasing the reliabilities of the individual tests decreases the reliability of the difference scores by increasing the correlation between observed scores over what it otherwise would be. But, on the other hand, as Equation 2 shows, increasing the reliabilities of the individual tests increases the reliability of the difference scores.
Unfortunately, Bereiter did not proceed further with his analysis, and researchers have not attended to these contradictory effects. Given that Equation 1, Equation 2, and the contradictory effects of varying the reliabilities of the individual tests, all depend on CTT, this lack of attention is surprising. My goal is to take the contradictory effects seriously to investigate their consequences for difference score reliability. In the analyses that follow, I intend to return to the roots of CTT to discern exactly the extent of the deleterious vs. beneficial effects of increasing the reliabilities of the individual tests on the reliability of the difference scores. In so doing, I am not committing to an actual belief or disbelief in CTT. Rather, because the criticisms stem from CTT, an investigation from the point of view of CTT directly addresses them. It also seems worthwhile to note that although more advanced theories have been proposed and favored by many researchers, such as generalizeability theory and item response theory, these reduce down to CTT in their simplest incarnations (see Cronbach, Gleser, Nanda, & Rajaratnam, Citation1972; Hulin, Drasgow, & Parsons, Citation1983, respectively).
1.2. The importance of CTT’s true correlations
Consider the attenuation formula from CTT, written below as Equation 3, where represents the true correlation between two tests (i.e. the correlation between true scores).
(3)
(3)
Equation 3 makes clear the importance of the reliabilities of the two tests. As the reliabilities of the tests increase, the observed correlation becomes increasingly similar to the true correlation. In the limiting case of perfectly reliable tests, the observed correlation equals the true correlation. When the tests are not perfectly reliable, the observed correlation is less than the true correlation. Thus, Equation 3 clarifies Bereiter’s (Citation1963) statement that increasing the reliabilities of the tests increases the correlation between observed scores, and hence decreases the reliability of the difference scores. To reiterate, however, Equation 2 shows a contradictory effect whereby increasing the reliability of the individual tests increases the reliability of the difference scores.
Let us again consider Equation 3, and the mathematical fact that the observed correlation depends on two things: the reliabilities of the individual tests and the true correlation coefficient. I have discussed the reliabilities of the individual tests above, but what about the true correlation coefficient? Equation 3 shows that, in general, as the true correlation increases, so does the observed correlation. An exception would be if the reliability of one or both of the individual tests equals zero, in which case, the observed correlation also would equal zero, regardless of the true correlation. Well, then, going back to Equation 2, we see that because increasing the true correlation tends to increase the observed correlation, and because increasing the observed correlation decreases the reliability of the difference scores, it follows that the reliability of the difference scores depends partly on the size of the true correlation. Specifically, the larger the true correlation, keeping the reliabilities of the individual tests constant, the less reliable the difference scores.
It is interesting that although the argument that difference scores have low reliability has its origin in CTT, and that CTT depends on the notion of a true correlation (which, in turn, depends on the notion of a true score), nobody to my knowledge has actually included true correlations in their investigation of the reliability of difference scores. In addition, my foregoing comments implicate the importance of the reliabilities of the individual tests as having complicated effects on the reliability of difference scores. Therefore, one of my main goals is to investigate how the true correlation interacts with the reliabilities of the two tests to determine the reliability of difference scores. It is not necessary to derive equations that are radically different from existing ones; rather, it is sufficient to express existing CTT equations in a form that includes true correlations and reliabilities of individual tests.
In addition, there is no law stating that the variances of the two tests have to be equal. As Chiou and Spreng (Citation1996) suggested, the sizes of variances between the two tests also matter. Possibly, the reliability of difference scores might be influenced by the interaction between the variances of the two tests, their reliabilities, and the true correlation. Therefore, my second goal is to investigate this potential triple interaction.
2. Analyses
I will present two types of analyses. First, I will assume equal variances and explore the reliability of difference scores as a function of the interaction between the reliabilities of the individual tests and the true correlation. Second, I will take into account the possibility of unequal variances and explore the reliability of difference scores as a function of three variables. These are the reliabilities of the individual tests, the true correlation, and the ratio of variances or standard deviations between the two tests.
2.1. Equal variances
To perform the analyses of interest, it is necessary to render the reliability of the difference scores in Equation 2 as a function of the reliabilities of the individual tests and the true correlation. At present, however, Equation 2 includes the observed correlation rather than the true correlation. Because the observed correlation is influenced, in part, by the reliabilities of the two tests, there is no way to vary these reliabilities and the observed correlation independently of each other. This inconvenience can be remedied simply by substituting Equation 3 into Equation 2. The result is Equation 4 below that features the true correlation, as opposed to the observed correlation.(4)
(4)
Based on Equation 4, Figure provides an illustration of how the reliabilities of the two tests interact with the true correlation to determine the reliability of the difference scores. In Figure , the reliabilities of the two tests were set equal to each other, and allowed to range from 0 to 1 along the horizontal axis. In addition, the curves represent the cases where the true correlation equals .1 (top curve), .3 (second curve), .5 (third curve), .7 (fourth curve), and .9 (bottom curve). Figure illustrates three effects. Most obviously, as the true correlation increases, the reliability of the difference scores (along the vertical axis) decreases. There also is an effect of the reliabilities of the tests on the reliability of the difference scores. Figure illustrates the totality of the contradictory effects I discussed earlier whereby increasing the reliabilities of the tests has both a deleterious effect on the reliability of difference scores (by increasing the true correlation) and a beneficial effect on the reliability of difference scores (by direct entry into Equation 2). The upward trend of all five curves in Figure , as the reliabilities of the individual tests increase, shows that the net effect of the contradictory forces is positive for the reliability of the difference scores. That is, as the reliabilities of the individual tests increase, so does the reliability of the difference scores.
Figure 1. The reliability of difference scores is presented as a function of the reliability of the individual tests and the true correlation.
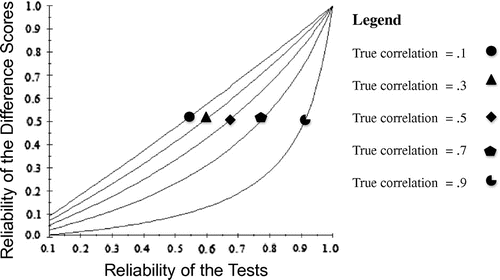
Finally, the interaction between the true correlation and the reliabilities of the individual tests deserves observation. As the true correlation increases, the effect of increasing the reliabilities of the individual tests on the reliability of the difference scores becomes increasingly nonlinear. For example, when the true correlation is .9, the reliabilities of the individual tests have to be quite impressive to result in a respectable reliability of the difference scores.
Well, then, are difference scores unreliable, as we so often have been told? Figure shows that the answer is, “It depends.” If the true correlation is large (e.g. .9), then it takes extremely impressive reliabilities of the individual tests to result in a reasonably sized reliability of difference scores; the shape of the bottom curve is a real problem. As an example, when the true correlation is .9, even if the reliabilities of both individual tests also equal .9, the reliability of the difference scores is an unimpressive .47. The reliabilities of both individual tests must be ratcheted up to .96 for the reliability of the difference scores to exceed an arbitrary cut-off of .7.
On the other hand, the problem is substantially mitigated if the true correlation is at lesser levels. For example, for the reliability of the difference scores to pass the cut-off of .7 when the true correlation is .7, it is only necessary for the reliabilities of the two tests to exceed .89—a difficult but far from impossible requirement. And as the true correlation decreases further, it is tolerable to have lesser reliabilities of the individual tests.
2.2. Unequal variances
Before conducting the analyses, it is again necessary to obtain an equation based on the true correlation as opposed to the observed correlation. In addition, it is necessary to include a ratio of standard deviations or variances (I will use standard deviations). The net effect of these considerations is to render a slightly more complicated derivation than in the previous section.
To commence, I define the deviation ratio (a) as the standard deviation of test X scores divided by the standard deviation of test Y scores, as is indicated in Equation 5 below. And Equation 6 is simply a rearrangement of Equation 5.(5)
(5)
(6)
(6)
Substituting Equation 6 into Equation 1 renders Equation 7.(7)
(7)
Cancelling renders Equation 8.
(8)
(8)
Although Equation 8 is nice to have, it is not sufficient in one respect. Specifically, it still retains the observed correlation, whereas we would like it to include the true correlation. The solution is to substitute Equation 3 into Equation 8 to render Equation 9.(9)
(9)
Figure illustrates how Equation 9 manifests where Panels A, B, and C represent when the true correlation is .1, .5, and .9, respectively; the reliabilities of the two tests are set equal to each other and vary from 0 to 1 (as in Figure ); and where the deviation ratio is 4 (top curve), 3, (second curve), 2 (third curve), or 1 (bottom curve). As in Figure , the reliability of the difference scores is indicated on the vertical axis.
Figure 2. The reliability of difference scores is presented as a function of the reliability of the individual tests, the true correlation, and the deviation ratio.
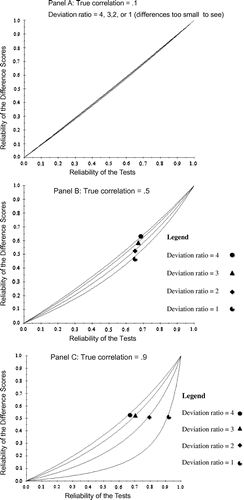
Figure shows that there are three trends that replicate what we saw in Figure . Comparing across panels in Figure shows that as the true correlation increases, the reliability of the difference scores decreases. Also, the upward curves in all three panels replicate that as the reliabilities of the individual tests increase, so does the reliability of the difference scores. Finally, these two effects qualify each other, as we saw earlier.
But some new observations also emerge. First, there is a general trend, which can be seen most easily in Panel C of Figure , that as the deviation ratio increases, so does the reliability of the difference scores. This effect supports the argument by Chiou and Spreng (Citation1996) in favor of high deviation ratios if one wishes to increase the reliability of difference scores. Second, there is an interesting interaction between the deviation ratio and the reliabilities of the individual tests that is particularly easy to see in Panel C of Figure . Hearkening back to Figure , recall the unfavorable shape of the curve when the true correlation was .9, which is illustrated again by the bottom curve in Panel C of Figure . But as the deviation ratio increases, note that the shape (and altitude) of the curve becomes increasingly less unfavorable. Thus, even in the worst-case scenario of a large true correlation, it is possible to obtain a respectable number for the reliability of the difference scores so long as the deviation ratio is high. Put another way, as the variances of the two tests become increasingly unequal, their reliabilities can be less impressive and still overcome the deleterious effects of a large true correlation. Finally, it is interesting to consider the three-way effect. In Panel A of Figure , where the true correlation is only .1, the altitude and shape of the curves is approximately the same regardless of the deviation ratio. In Panel B of Figure , where the true correlation is .5, the effect of the deviation ratio is perceptible, and shows that as it increases, so does the reliability of the difference scores. In Panel C, as we already have seen, the separation of the curves is impressive.
3. Discussion
Given Equations 4 and 9, as well as Figures and , what can we conclude about the reliability of difference scores? The analyses demonstrate that, if the reliabilities of the individual tests are good, the reliability of the difference scores will be at least reasonable so long as the true correlation is not too large. The difficulty comes in when the true correlation is extremely large (e.g. .9). In this case, the reliabilities of the individual tests have to be excellent—and better than can be expected from most tests—to enable the reliability of the difference scores to be reasonable. An exception would be if the two tests have very different variances (e.g. deviation ratio is 4), in which case, the reliabilities of the individual tests can be reduced and still result in a reasonable value for the reliability of the difference scores.
This seems like a good place to pause to make sure that a simple point does not get lost in the interactions illustrated by Figure . Specifically, in all of the analyses, the reliability of the difference scores is limited by the reliabilities of the individual tests. The researcher can tolerate somewhat greater or lesser reliabilities of the individual tests, depending on the true correlation and the deviation ratio. However, even when the true correlation is low (as in Panel A of Figure ), so the deviation ratio does not matter in a discernible way, the reliabilities of the individual tests still set a limit on how reliable the difference scores can be.
I commenced the present work by emphasizing the extent to which difference score research is deplored in much education and social science literature based on reliability grounds. However, the analyses show that a blanket condemnation is not justified. The analyses also provide the necessary information for sensible recommendations for answering the question, “With respect to the particular difference score research one wishes to conduct, how can one know whether or not to proceed?”
Let us recall Equation 4, which implies that with equal variances, the two important factors are the reliabilities of the individual tests and the true correlation. If one is using well-established tests, their reliabilities ought to already be known. And if not, the researcher can conduct a pilot study to find out. The true correlation is more of a problem because it is a hypothetical entity that cannot be obtained directly. However, it can be calculated indirectly. To see that this is so, consider a rearrangement of Equation 3 that is given below as Equation 10.(10)
(10)
If the correlation between the individual tests is known, and the reliabilities are known, or these can be determined by pilot research, Equation 10 provides an estimate of the true correlation. In turn, the true correlation and the reliabilities of the two tests can be instantiated into Equation 4 to predict the reliability of the difference scores. Using this method, anyone can determine, for the particular research under consideration, whether the difference scores will be sufficiently reliable for the purpose at hand. If so, the researcher can go ahead and perform the research without qualms about the reliability of the difference scores.
But suppose that the result of the foregoing process is not satisfactory. It still might not be necessary for the researcher to give up on his or her plans. Remember that Equation 4 assumes equal variances and this might not be so. If the variances of the two tests are known, or can be determined via pilot research, the researcher should use Equation 9 rather than Equation 4, which will result in a larger (and more accurate) predicted value for the reliability of the difference scores. If the result of using Equation 9 is satisfactory, the research can proceed without qualms about the reliability of the difference scores. However, if even the use of Equation 9 fails to result in a sufficient value for the reliability of the difference scores, then the researcher might contemplate returning to the drawing board.
Consider an example. Suppose that the reliabilities of the two tests are .85 for each, that the estimated true correlation is .5 (from Equation 10), and that the deviation ratio is 3. In that case, using Equation 4, the reliability of the difference scores would be predicted to be .74. Although this is not an outstanding value, it would take a hard-hearted reviewer to conclude that the proposed research should not be conducted. In addition, suppose we use Equation 9, rather than Equation 4, to take advantage of the deviation ratio (5). In that case, the reliability of the difference scores would be predicted to be .80, and even the hard-hearted reviewer should be satisfied.
The present analyses show that although much depends on the reliabilities of the individual tests, much also depends on the true correlation and on the deviation ratio. How likely are these to be favorable? It might depend on whether the difference score is based on the same test conducted at different times or on whether it is based on different tests. For example, if a school psychologist is interested in the difference between mathematical and verbal ability, tests of these are sufficiently different that the true correlation likely would not be particularly large. In addition, measurement in the area has advanced to the level where researchers routinely report reliabilities in excess of .9 for a variety of abilities. The combination of the low true correlation and high reliabilities of the individual tests implies that the reliability of the difference scores should be impressive, regardless of the deviation ratio. In contrast, if a researcher is interested in difference scores based on using the same test twice, the true correlation might be quite large. And this would be a problem unless the reliability of the test on both test-taking occasions is very impressive or the deviation ratio is large. Why might the deviation ratio be a large value? One reason is that the treatment might raise or lower scores so that they collect near a ceiling or floor, respectively. In that case, the variance would be much lower on the second test-taking occasion than on the first one, thereby causing a large deviation ratio. There is a risk that the reduction in variance on the second test-taking occasion might decrease the reliability of the test on that occasion, depending on the relative amounts by which true correlation variance vs. error variance decreases.
Of course, even if the same test is given before and after treatment, there are reasons why the true correlation might nevertheless not be a large value. To see why this might be so, contrast the case where the treatment has an approximately equal effect on each person against the case where the treatment has unequal effects on different persons—that is, there is a treatment × person interaction. In the former case, the true correlation will be a large number, the deviation ratio will be near unity, and so one would need extremely favorable reliabilities on both test-taking occasions to have reasonably reliable difference scores. However, if there is a treatment × person interaction, the true correlation likely will not be a large value, and so the difference scores will be reasonably reliable, provided that test is reasonably reliable on both test-taking occasions. As an additional possibility, in the absence of ceiling or floor effects, the treatment × person interaction could increase variance on the second test-taking occasion depending on the nature of the interaction. Although treatment × person interactions add error variance from the point of view of statistical tests, they can increase the reliability of difference scores by reducing the true correlation or by causing the deviation ratio to stray from unity.
Does it matter whether the difference scores are reliable? This depends on one’s purpose. If the goal is simply to demonstrate that the treatment works, lack of reliability of the difference scores likely will not be fatal for the statistical test, as Thomas and Zumbo (Citation2012) showed. But if the goal is to correlate the difference scores with another variable, then difference score reliability will matter a great deal.
In conclusion, based on Equations 1 and 2, much negativity has been directed towards difference scores. However, because these equations came out of CTT, and because CTT features the notion of true correlations, it is surprising that previous researchers who have been concerned with difference score reliability have not included the interaction between true correlations and reliabilities of individual tests in their analyses. But it is necessary to include this interaction because the reliabilities of individual tests interact with the true correlation to determine the observed correlation that plays so important a role in Equations 1 and 2. The reliabilities of the individual tests play an additional role through their direct entry in these equations. Finally, the deviation ratio also plays its part in an interaction with the reliabilities of the individual tests and the true correlation. It is only through Equations 4 and 9, derived above, that the interactions of these effects can be analyzed and understood. The analyses based on these equations suggest that difference scores may have received an undeserved bad reputation. Although there are some combinations of true correlations, reliabilities of individual tests, and deviation ratios that imply that difference scores are unreliable, there are other combinations that imply that difference scores are reasonably reliable or even extremely reliable. Rather than make a blanket statement, each person should evaluate, for herself or himself, the reliability of difference scores in the context in which they are to be used. Equation 4 (equal variances) or Equation 9 (unequal variances) provides the way for each researcher to come to his or her own conclusion with respect to the particular tests under consideration, as I demonstrated earlier. Surely, this is superior to basing research decisions on a blanket recommendation that often is wrong.
Additional information
Funding
Notes on contributors
David Trafimow
David Trafimow is a distinguished achievement professor of psychology at New Mexico State University, a fellow of the Association for Psychological Science, executive editor of the Journal of General Psychology, and also for Basic and Applied Social Psychology. He received his PhD in psychology from the University of Illinois at Urbana-Champaign in 1993. His current research interests include attribution, attitudes, cross-cultural research, ethics, morality, methodology and philosophy of science, potential performance theory, and descriptive and inferential statistical analyses.
References
- Bereiter, C. (1963). Some persisting dilemmas in the measurement of change. In C. W. Harris (Ed.), Problems in measuring change (pp. 3–20). Madison: University of Wisconsin Press.
- Chiou, J. S., & Spreng, R. A. (1996). The reliability of difference scores: A re-examination. Journal of Consumer Satisfaction, Dissatisfaction, and Complaining Behavior, 9, 158–167.
- Cronbach, L. J. (1990). Essentials of psychological testing (5th ed.). New York, NY: Harper Collins.
- Cronbach, L. J., Gleser, G. C., Nanda, H., & Rajaratnam, N. (1972). The dependability of behavioral measurements: Theory of generalizability for scores and profiles. New York, NY: Wiley.
- Gaito, J., & Wiley, D. E. (1963). Univariate analysis of variance procedures in the measurement of change. In C. W. Harris (Ed.), Problems in measuring change (pp. 60–84). Madison: University of Wisconsin Press.
- Gulliksen, H. (1987). Theory of mental tests. Hillsdale, NJ: Lawrence Erlbaum Associates.
- Hulin, C., Drasgow, F., & Parsons, C. (1983). Item response theory: Application to psychological measurement. Homewood, IL: Dow Jones-Irwin.
- Lord, F. M. (1963). Elementary models for measuring change. In C. W. Harris (Ed.), Problems in measuring change (pp. 21–38). Madison: University of Wisconsin Press.
- Thomas, D. R., & Zumbo, B. D. (2012). Difference scores from the point of view of reliability and repeated-measures ANOVA: In defense of difference scores for data analysis. Educational and Psychological Measurement, 72, 37–43.10.1177/0013164411409929