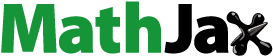
Abstract
The Wiener–Hopf factorization plays a crucial role in studying various mathematical problems. Unfortunately, in many situations, the Wiener–Hopf factorization cannot provide closed form solutions and one has to employ some approximation techniques to find its solutions. This article provides several weak, approximation for a given Wiener–Hopf factorization problem. Application of our finding in spectral factorization and Lévy processes have been given.
Public Interest Statement
This article provides several weak approximation for a given Wiener–Hopf factorization problem. Application of our finding in spectral factorization and Lévy processes have been given.
1. Introduction
Roughly speaking, the Wiener–Hopf factorization problem is a technique to find a single complex-valued function in which its radial limits, say
, are respectively analytic and bounded separately in the upper and lower complex half planes (i.e.
and
) and satisfy
, where
and
is a zero index function which satisfies the Hölder condition.
The Wiener–Hopf factorization has proved remarkably useful in solving an enormous variety of model problems in a wide range of branches of physics, mathematics, and engineering. Subjects for which the problem is applicable range from neutron transport (Noble, Citation1988), geophysical fluid dynamics (Davis, Citation1987; Kaoullas & Johnson, Citation2010), diffraction theory (Noble, Citation1988), fracture mechanics (Freund, Citation1998; Shakib, Akhgarian, & Ghaderi, Citation2015), non-destructive evaluation of materials (Achenbach, Citation2012), a wide class of integral equations (Payandeh Najafabadi & Kucerovsky, Citation2009,Citation2014b), acoustics (Abrahams & Wickham, Citation1990), elasticity (Norris & Achenbach, Citation1984; Ogilat, Citation2013), electromagnetics (Daniele, Citation2014; Sautbekov & Nilsson, Citation2009), water wave phenomena (Chakrabarti & George, Citation1994; Kim, Schiavone, & Ru, Citation2011), geophysics (Davis, Citation1987), financial mathematics (Beheshti, Payandeh Najafabadi, & Farnoosh, Citation2013; Fusai, Abrahams, & Sgarra, Citation2006), distribution of extrema in a wide class of Lévy processes (Payandeh Najafabadi & Kucerovsky, Citation2011,Citationin press), statistical decision problems (Kucerovsky, Marchand, Payandeh Najafabadi, & Strawderman, Citation2009), etc.
The key steps to solve a Wiener–Hopf factorization is decomposing of the kernel into a product of two terms,
and
, where
and
are analytic and bounded in the upper and the lower complex half planes, respectively. Such decomposition can be expressed in terms of a Sokhotski–Plemelj integral (see Equation, 1), but this form presents some difficulties in numerical work due to slow evaluation and numerical problems caused by singularities near the integral contour (see Kucerovsky & Payandeh Najafabadi, Citation2009, for more details). To overcome these problems, several approximation methods have been considered (see Abrahams, (Citation2000); Kudryavtsev & Levendorskiĭ, Citation2009; Kuznetsov, Citation2010; Rawlins, Citation2012 among others). But, as far as we known, ( i) none of them provides any estimation bound for their approximation methods; (ii) most of them need uniform convergence, which is usually hard to achieve.
This article studies the problem of solving a Wiener–Hopf factorization problem, approximately. Then, it provides (i) an ,
, approximation for a Wiener–Hopf factorization problem; (ii) estimation bounds for such approximation technique; (iii) application of our findings in spectral factorization and Lévy processes. This article has been developed as the following. Section 2 collects some useful elements which are used later. The main contribution of this article on approximating solutions of a given a Wiener–Hopf factorization problem has been given in Section 3. Application of our findings has been given in Section 4. Concluding remarks has been given in Section 5.
2. Preliminaries
Now, we collect some lemmas which are used later.
Definition 1
A function in
is said to be an exponential-type T function on the domain
if there are positive constants M and T such that
, for
.
The well-known Paley–Wiener theorem states that the Fourier transform of an function vanishes outside of an interval
, if and only if the function is of exponential-type T (see Dym & McKean, Citation1972, p. 158, for more details). The exponential-type functions are continuous functions which are infinitely differentiable everywhere and have a Taylor series expansion over every interval (see Champeney, Citation1987, p. 77; Walnut, Citation2002, p. 81). These functions are also called band-limited functions (see Bracewell, Citation2000, p. 119, for more details on bandlimited functions) (which are equivalent to exponential-type functions by the above stated Paley–Wiener theorem). The index of a complex-valued function
on a smooth oriented curve
, such that
is closed and compact, is defined to be the winding number of
about the origin (see Payandeh Najafabadi, Citation2007, §1, for more technical details). Computing the index of a function is usually a key step to determine the existence and number of solutions of a Wiener–Hopf factorization problem. The Sokhotski–Plemelj integral of a function s which satisfies the Hölder condition and it is defined by a principal value integral, as follows.
(1)
(1)
The following are some well-known properties of the Sokhotski-Plemelj integral, proofs can be found in Ablowitz and Fokas (Citation1990, §7), Gakhov (Citation1990, §2), and Pandey (Citation1996, §4), among others. The radial limit of the Sokhotski-Plemelj integral of s, given by can be represented as the jump formula. i.e.
, (or
) where
is the Hilbert transform of s and
.
The Hausdorff-Young theorem states that: If s is a function in . Then, its Fourier transform, say
, is an
function that satisfies
, where
and
, see Pandey (Citation1996) for more details. From the Hausdorff–Young Theorem, one can observe that if
is a sequence of functions converging in
,
, to s. Then, the Fourier transforms of
converge in
, to the Fourier transform of s, whenever
. Using the Hausdorff–Young theorem, Payandeh Najafabadi and Kucerovsky (Citation2014a) established that Hilbert transform of an
,
function s, say
, satisfies
(2)
(2)
Form this observation, one may conclude that “if ,
, is a sequence of functions which converge in
,
, to
. Then, the Hilbert transforms of
’s also converge in
to the Hilbert transform of
”.
The following, from Kucerovsky and Payandeh Najafabadi (Citation2009), recalls some further useful properties of functions in space.
Lemma 1
Suppose s and r are functions in , and suppose that
and
are bounded above by a. Then,
(i) | |||||
(ii) |
| ||||
(iii) |
| ||||
(iv) |
|
The followings recall definition and some useful properties on a mixture-gamma distribution, which plays an important role for the next sections (see Bracewell, Citation2000 for more details).
Definition 2
(mixture-gamma family of distributions) A non-negative random variable is said to be distributed according to a mixture-gamma distribution if its density function is given by
(3)
(3)
where and
are positive value which satisfy
.
Lemma 2
The characteristic function of a distribution (or equivalently the Fourier transform of its density function), say , has the following properties:
(i) |
| ||||
(ii) |
|
3. Main results
Definition 3
The Wiener–Hopf factorization is the problem of finding a sectionally analytic function whose upper and lower radial limits at the real line, say
, satisfy
(4)
(4)
where is a given continuous function satisfying a Hölder condition on
. Moreover,
is assumed to have zero index, to be non-vanishing on
, and bounded above by 1.
Payandeh Najafabadi and Kucerovsky (Citation2011) established that sectionally analytic functions satisfying a zero index Wiener–Hopf factorization (3) can be found by
where stands for the Sokhotski–Plemelj integration of
. Using the jump formula, the above
can be re-stated as
(5)
(5)
where stands for the Hilbert transform of
.
In many situations, the Wiener–Hopf factorization problems (3) cannot be solved explicitly and has to be solved approximately (see Kucerovsky & Payandeh Najafabadi, Citation2009, for more details). The following develops an approximate technique to solve Equation 3.
Theorem 1
Suppose in the the Wiener-Hopf factorization problem (3) is a given, bounded (above by a), zero index function, satisfies the Hölder condition and
. Moreover, suppose that there is a sequence of sectionally analytic functions
, where
-s converge (in
,
, sense) to
. Then, sectionally analytical solution of the Wiener–Hopf factorization problem (3), say
, can be approximated by
and the error estimate satisfies
Proof
Set and
. Now, from Equation 5, Equation 2, and Lemma 1, observe that
In our belief, the most favorable situation is to approximate by a sequence of rational functions, which are obtained from a Padé approximant or a continued fraction expansion.
Using the Shannon sampling theorem, the following develops an elegant scheme that allows to provide an explicit solution for a Wiener–Hopf factorization Equation 3.
Theorem 2
Suppose in the Wiener–Hopf factorization problem (Equation 3) is a given,
, bounded, zero index function, satisfies the Hölder condition. Moreover, suppose that there is a sequence of
is an exponential-type T function, then unique solutions of the Wiener–Hopf factorization (Equation 3) can be explicitly determined by
Proof
Using the fact that is an exponential-type T function, one can decompose
as
, where
Sectionally analytical properties of in
has been established by Kucerovsky and Payandeh Najafabadi (Citation2009).
The following theorem provides the error bound for approximate solution arrives from the Shannon sampling theorem.
Theorem 3
Suppose in the Wiener–Hopf factorization problem () is a given,
, bounded, zero index function, satisfies the Hölder condition and
is an exponential-type T function. Moreover, suppose that there is a sequence of
in
and
are exponential-type T functions. Then, approximate solutions of the Wiener–Hopf factorization problem () can be determined by
and the error bound satisfies
where the norm is defined by .
Proof
The proof is straightforward by a double application of Lemma (3.2, Payandeh Najafabadi & Kucerovsky, Citation2014a) and Theorem 2.
4. Applications
This section provides the application of the above results in two different contexts. The first subsection considers the problem of finding spectral factorization, whenever its corresponding spectral density function has been given. The second subsection derives density/probability functions of extrema random variables of a given Lévy processes.
4.1. Application to spectral factorization
Definition 4
Suppose is an autocorrelation of the stochastically stationary process
, i.e.
. Then, the spectral function
is defined by
, where
is a given measure.
The spectral density function has properties that: (1) for stochastically stationary processes, it can be understood as the Fourier transform of the autocorrelation
[The Wiener–Khintchine’s theorem: Reinsel (Citation1997, p. 219)]; (2) it is a Hermitian function (Koopmans, Citation1995, p. 122); (3) it defines almost everywhere (a.e.) on the interval
(Wilson, Citation1972); and (4)
is integrable and has a Fourier series expansion
, where
(Wilson, Citation1972).
The spectral factorization plays a crucial role in a wide range of scientific fields, such as communications (Magesacher & Cioffi, Citation2011), system theory (Janashia, Lagvilava, & Ephremidze, Citation2011), optimal control (Johannesson, Rantzer, & Bernhardsson, Citation2011), filtering theory (Anderson & Moore, Citation2005), network theory (Belevitch, Citation1968; Ivrlac & Nossek, Citation2014) prediction theory of stationary processes (Brockwell & Davis, Citation2002), deriving forward expression from a backward one in the ARIMA processes (Brockwell & Davis, Citation2009). In such applications, the spectral density factorization is the most difficult step. Since Wiener’s seminal efforts create a computational method for spectral factorization, several authors have developed different methods to do so, but none of them have provided a method that has an essential superiority over all others (Janashia et al., Citation2011). On the other hand, most of these methods impose some extra restriction on the spectral density (e.g. to be real or rational function, or to be non-singular on the boundary). The following represents an explicit solution for the problem of spectral factorization for spectral densities.
Proposition 1
Suppose is a spectral density function. Then, the right and left spectral factorizations
are given by
Proof
The exponential-type T condition of arrives from the fact that the spectral density can be understood as an extension version of the inverse Fourier transform on the autocorrelation function which is bounded function. The rest of proof arrives from an application of Theorem 2 along the fact that
is Hermitian function which its index is zero (Voronin, Citation2010,Citation2011).
4.2. Application to Lévy processes
Suppose is a one-dimensional real-valued Lévy process started from
and defined by a triple
the drift
, volatility
, and the jumps measure
which is given by a non-negative function defined on
satisfying
. Moreover, suppose that random stopping time
has either a geometric (with parameter
) or an exponential distribution (with parameter
) and independent of the Lévy process
which
. The Lévy–Khintchine formula states that the characteristic exponent
(i.e.
) can be represented by
(6)
(6)
The extrema of the Lévy process are given by
(7)
(7)
The Wiener–Hopf factorization is a well-known technique to study the characteristic functions of the extrema random variables (Bertoin, Citation1996). Namely, the Wiener–Hopf factorization states that: (i) random variables and
are independent; (ii) product of their characteristic functions equal to the characteristic function of Lévy process
(iii) random variable
(
) is infinitely divisible, positive (negative), and has zero drift.
In the cases that the characteristic function of Lévy process either a rational function or can be decomposed as a product of two sectionally analytic functions in the closed upper and lower half complex planes
and
. The characteristic functions of random variables
and
can be determined explicitly. Lewis and Mordecki (Citation2005) considered a Lévy process
which its negative jumps distributed according to a mixture-gamma family of distributions (given by Definition 2) and an arbitrary positive jumps measure. They established such process has the characteristic function which can decompose as a product of a rational function and an arbitrary function, which are analytic in
and
, respectively. Moreover, they provided an analog result for a Lévy process whose its corresponding positive jumps measure follows from a mixture-gamma family of distributions while its negative jumps measure is an arbitrary one, more details can be found in Lewis and Mordecki (Citation2008).
Unfortunately, in the most situations, the characteristic function of the process neither is a rational function nor can be decomposed as a product of two analytic functions in and
. Therefore, the characteristic functions of
and
should be expressed in terms of a Sokhotski-Plemelj integral (see Equation 1). But, this form, also, presents some difficulties in numerical work due to slow evaluation and numerical problems caused by singularities near the integral contour. To overcome these difficulties, approximation methods have to be considered.
It is well known that a Lévy process which its jumps distribution follows from the phase-type distribution has a rational characteristic function (Doney, Citation1987). Kuznetsov (Citation2010) utilized this fact and approximated a jumps measure
a ten-parameter family of Lévy processes (named
family of Lévy process) by a sequence of the phase-type measures. Then, he determined the characteristic functions of random variables
and
, approximately.
The following theorem represents an estimation bound for approximated extrema’s density/probability functions of a Lévy process.
Theorem 4
Suppose is a Lévy process defined by a triple
and its random stopping time
has been distributed according to either a geometric or an exponential distribution. Moreover suppose that there is a sequence of jumps measure
which satisfies the following two conditions:
A1) | They converge in | ||||
A2) | their corresponding characteristic exponents |
(i) | For exponentially distributed stopping time | ||||
(ii) | For geometric stopping time |
Proof
From Bertoin (Citation1996), one can observe that the Fourier transform of and
density functions, say respectively
and
, satisfy either the Wiener–Hopf factorization
, where
(for exponentially distributed stopping time) or the Wiener–Hopf factorization
, where
(for geometric stopping time). Now, from the fact that expressions
and
are the characteristic function of Lévy process
respectively for exponential and geometric stopping time, observe that both expressions are bounded by 1 (property of the characteristic function given by Lemma 2, part ii). For part (i), from Theorem 1 observe that
where . The second inequality arrives from part (iv) of Lemma 1, while the third inequality obtains from Equation 6 along with conditions
and an application of Hausdorff-Young theorem. The rest of proof arrives from an application of the Hausdorff-?Young theorem. Proof of part (ii) is quite similar.
It would worthwhile to mention that, in the case of . One may obtain sequence
which satisfy the desire condition.
The following utilizes result of the above theorem and provides a procedure to find the extrema’s density/probability of a wide class of Lévy processes, approximately.
Procedure 1
Suppose is a Lévy process with bounded characteristic exponents
. Moreover, suppose that random stopping time
has been distributed according to either a geometric or an exponential distribution. Then, by the following steps, one can approximate (in
, sense) density/probability functions of the extrema random variables
and
.
Step 1. | Approximate jumps measure | ||||
Step 2. | Decompose rational function | ||||
Step 3. | Obtain, approximate, density/probability functions of |
In many situations, it is more convenient to approximate the characteristic exponents , rather than the jumps measure
. The following extends results of Theorem 4 to such situations.
Corollary 1
Suppose is a Lévy process defined by a triple
and its random stopping time
has been distributed according to either a geometric or an exponential distribution. Moreover suppose that there is a sequence of bounded characteristic exponents
(i.e.
) which converge in
, to the characteristic exponent of the process
, and their corresponding jumps measure
satisfies
.
Then, density/probability function of the supremum and the infimum of Lévy process , say respectively
and
, can be approximated by sequence of density/probability functions
and
which has the following error bound.
(i) | For exponentially distributed stopping time | ||||
(ii) | For discrete stopping time |
Using the fact that, the characteristic exponent , is a real-valued function, Bertoin (Citation1996). One can suggest that following procedure to generate approximation density/probability functions for
and
.
Procedure 2
Suppose is a Lévy process with bounded characteristic exponents
. Moreover, suppose that random stopping time
has been distributed according to either a geometric or an exponential distribution. Then, by the following steps, one can approximate (in
, sense) density/probability functions of the extrema random variables
and
.
Step 1. | Approximate (in | ||||
Step 2. | Decompose rational function | ||||
Step 3. | Obtain, approximate, density/probability functions of |
Using Lemma 2, one can readily, conclude that the above two procedures approximate density/probability functions of and
by the mixture-gamma density functions.
Now, we provide several examples.
Example 1
Consider a 1-stable Lévy process with a jumps measure . One can, readily, show that the characteristic exponent of such process is
, where
is a normalized real-valued. The natural logarithm
has the continued fraction
(see Jones & Thron, Citation1931 or Cuyt, Petersen, Verdonk, Waadeland, & Jones, Citation2008, among others). In Practice, in the above-continued fraction, it has to cut off somewhere and obtain a rational function for
. Consequently, an expression
can be approximated by rational function
. Now, the characteristic functions for the extrema can be obtained after decomposing
into product of two rational, analytic, and bounded functions, in
and
.
Example 2
Suppose is a Lévy process with independent and continuous
and a jumps measure
. The characteristic exponent for such Lévy process is given by
where ,
,
, and
are given. The continued fraction for
is given by
(Cuyt et al., Citation2008). After cutting off the above-continued fraction at the
term, one may be obtained a rational function, say
, for
. Substituting the
in an expression
, we may obtain a rational function, say
. Therefore, the characteristic functions for the extrema can be found by decomposing a rational function
as a product of two rational, analytic, and bounded functions in
and
. This observation verifies Kuznetsov’s (Citation2010) result.
Example 3
Metron model is a Lévy process with a jumps measure and characteristic exponent
, where a,
,
, and
are given and
. Metron model has tail behaviors heavier than the Gaussian but all exponential moments are finite. The continued fraction for the exponential function
is given by
(Cuyt et al., Citation2008). After cutting off the above-continued fraction somewhere, and substituting the arrived rational function in an expression
, we may obtain a rational function, say
. The characteristic functions for the extrema can be found by decomposing
as a product of two rational, analytic, and bounded functions in
and
.
5. Conclusion and suggestion
This paper provides two techniques to solve a Wiener–Hopf factorization problem. Application of our findings, in two different contexts, has been given. It would be worthwhile mentioning that: (1) In the situation where given function is not of exponential-type function. We suggest to approximate such function with an exponential-type function which pointwise converges to such function (see Kucerovsky and Payandeh Najafabadi (Citation2009) for more details); (2) Other applications our findings can be developed to other situations where the Wiener–Hopf factorization is applicable, such as finding first/last passage time and the overshoot, the last time the extrema was archived, several kind of option pricing, etc.
Acknowledgements
Thanks to an anonymous reviewer for his/her constructive comments. The author Dan Z. Kucerovsky acknowledges Natural Sciences and Engineering Research Council (NSERC).
Additional information
Funding
Notes on contributors
Amir T. Payandeh Najafabadi
Amir T. Payandeh Najafabadi is an Associate Professor in Department of Mathematics sciences at Shahid Behashti University, Tehran, Evin (Email address: [email protected]). He was born on Sep 3, 1973. He received his PhD from University of New Brunswick, Canada in 2006. He has published 28 papers and was co-author of two books. His major research interests are: Statistical Decision Theory, Lévy processes, Risk theory, Riemann-Hilbert problem, & integral equations.
References
- Ablowitz, M. J., & Fokas, A. S. (1990). Complex variable, introduction and application. Berlin: Springer-Verlag.
- Abrahams, I. D. (2000). The application of Padéapproximants to Wiener–Hopf factorization. IMA Journal of Applied Mathematics, 65, 257–281.
- Abrahams, I. D., & Wickham, G. R. (1990). General Wiener–Hopf factorization of matrix kernels with exponential phase factors. SIAM Journal of Applied Mathematics, 50, 819–838.
- Achenbach, J. D. (2012). Wave propagation in elastic solids. Amsterdam: North-Holland.
- Anderson, B. D., & Moore, J. B. (2005). Optimal filtering. New York, NY: Dover.
- Beheshti, M. H., Payandeh Najafabadi, A. T., & Farnoosh, R. (2013). An analytical approach to pricing discrete barrier options under time-dependent models. European Journal of Scientific Research, 103, 304–312.
- Belevitch, V. (1968). Classical network theory. San Francisco, CA: Holden Day.
- Bertoin, J. (1996). Lévy processes. New York, NY: Cambridge University Press.
- Bracewell, R. N. (2000). The Fourier transform and its applications (3rd ed.). New York, NY: McGraw-Hill.
- Brockwell, P. J., & Davis, R. A. (2002). Introduction to time series and forecasting. New York, NY: Springer-Verlag.
- Brockwell, P. J., & Davis, R. A. (2009). Time series: Theory and methods. New York, NY: Springer-Verlag.
- Chakrabarti, A., & George, A. J. (1994). Solution of a singular integral equation involving two intervals arising in the theory of water waves. Applied Mathematics Letters, 7, 43–47.
- Champeney, D. C. (1987). A handbook of Fourier theorems. New York, NY: Cambridge University Press.
- Cuyt, A., Petersen, V. B., Verdonk, B., Waadeland, H., & Jones, W. B. (2008). Handbook of continued fractions for special functions. New York, NY: Springer.
- Daniele, V. G. (2014). The Wiener–Hopf method in electromagnetics (Technical report). New York, NY: Institution of Engineering and Technology.
- Davis, A. M. J. (1987). Continental shelf wave scattering by a semi-infinite coastline. Geophysical & Astrophysical Fluid Dynamics, 39, 25–55.
- Doney, R. A. (1987). On Wiener–Hopf factorisation and the distribution of extrema for certain stable processes. The Annals of Probability, 15, 1352–1362.
- Dym, H., & Mckean, H. P. (1972). Fourier series and integrals. Probability and mathematical statistics. New York, NY: Academic Press.
- Freund, L. B. (1998). Dynamic fracture mechanics. Cambridge: Cambridge University Press.
- Fusai, G., Abrahams, I. D., & Sgarra, C. (2006). An exact analytical solution for discrete barrier options. Finance and Stochastics, 10, 1–26.
- Gakhov, F. D. (1990). Boundary value problem (Translated from the Russian Reprint of the 1966 translation). New York, NY: Dover Publications.
- Ivrlac, M., & Nossek, J. (2014). The multiport communication theory. IEEE Circuits and Systems Magazine, 14, 27–44.
- Janashia, G., Lagvilava, E., & Ephremidze, L. (2011). A new method of matrix spectral factorization. IEEE Transactions on Information Theory, 57, 2318–2326.
- Johannesson, E., Rantzer, A., & Bernhardsson, B. (2011). Optimal linear control for channels with signal-to-noise ratio constraints. In American Control Conference. San Francisco, CA.
- Jones, W. B., & Thron, W. J. (1931). Continued fractions: Analytic theory and applications. New York, NY: Addison-Wesley.
- Kaoullas, G., & Johnson, E. R. (2010). Fast accurate computation of shelf waves for arbitrary depth profiles. Continental Shelf Research, 30, 833–836.
- Kim, C. I., Schiavone, P., & Ru, C. Q. (2011). The effect of surface elasticity on a Mode-III interface crack. Archives of Mechanics, 63, 267–286.
- Koopmans, L. H. (1995). The spectral analysis of time series. New York, NY: Academic Press.
- Kucerovsky, D., Marchand, É., Payandeh, A. T., & Strawderman, W. (2009). On the Bayesianity of maximum likelihood estimators of restricted location parameters under absolute value error loss. Statistics & Decisions, 27, 145–168.
- Kucerovsky, D., & Payandeh Najafabadi, A. T. (2009). An approximation for a subclass of the Riemann–Hilbert problems. IMA Journal of Applied Mathematics, 74, 533–547.
- Kudryavtsev, O., & Levendorskiĭ, S. (2009). Fast and accurate pricing of barrier options under Lévy processes. Finance and Stochastics, 13, 531–562.
- Kuznetsov, A. (2010). Wiener–Hopf factorization and distribution of extrema for a family of Lévy processes. The Annals of Applied Probability, 20, 1801–1830.
- Lewis, A., & Mordecki, E. (2005). Wiener–Hopf factorization for Lévy processes having negative jumps with rational transforms ( Technicall report). Retrieved from http://www.cmat.edu.uy/mordecki/articles/
- Lewis, A., & Mordecki, E. (2008). Wiener–Hopf factorization for Lévy processes having positive jumps with rational transforms. Journal of Applied Probability, 45, 118–134.
- Magesacher, T., & Cioffi, J. M. (2011). On minimum peak-to-average power ratio spectral factorization. In The 8th International Workshop on Multi-Carrier Systems & Solutions. Herrsching.
- Noble, B. (1988). Methods based on the Wiener–Hopf technique (2nd ed.). New York, NY: Chelsea Publishing.
- Norris, A. N., & Achenbach, J. D. (1984). Elastic wave diffraction by a semi infinite crack in a transversely isotropic material. Quarterly Journal of Mechanics and Applied Mathematics, 37, 565–580.
- Ogilat, O. N. (2013). Steady and unsteady free surface flow past a two-dimensional stern ( Doctoral dissertation). University of Southern Queensland, Queensland.
- Pandey, J. (1996). The Hilbert transform of Schwartz distributions and application. New York, NY: Wiley.
- Payandeh Najafabadi, A. T. (2007). Riemann–Hilbert and statistical inference problems in restricted parameter spaces ( Doctoral dissertation). Department of Mathematics and Statistics, University of New Brunswick, Feredricton.
- Payandeh Najafabadi, A. T., & Kucerovsky, D. (2009). A weak approximated solution for a subclass of Wiener–Hopf integral equation. IAENG International Journal of Applied Mathematics, 39, 247–252.
- Payandeh Najafabadi, A. T., & Kucerovsky, D. (2011). On distribution of extrema for a class of Lévy processes. Journal of Probability and Statistical Science, 9, 127–138.
- Payandeh Najafabadi, A. T., & Kucerovsky, D. (2014a). Exact solutions for a class of matrix Riemann–Hilbert problems. IMA Journal of Applied Mathematics, 79, 109–123.
- Payandeh Najafabadi, A. T., & Kucerovsky, D. (2014b). On solutions of a system of Wiener–Hopf integral equations. IAENG International Journal of Applied Mathematics, 44, 1–6.
- Payandeh Najafabadi, A. T., & Kucerovsky, D. (in press). Approximate Wiener–Hopf factorization for finance problems.
- Rawlins, A. D. (2012). The method of finite-product extraction and an application to Wiener–Hopf theory. IMA Journal of Applied Mathematics, 77, 590–602.
- Reinsel, G. C. (1997). Elements of multivariate time series analysis. New York, NY: Springer-Verlag.
- Sautbekov, S., & Nilsson, B. (2009). Electromagnetic scattering theory for gratings based on the Wiener–Hopf method. AIP Conference Proceedings, 1106, 110–117.
- Shakib, J. T., Akhgarian, E., & Ghaderi, A. (2015). The effect of hydraulic fracture characteristics on production rate in thermal EOR methods. Fuel, 141, 226–235.
- Voronin, A. F. (2010). Partial indices of unitary and Hermitian matrix functions. Siberian Mathematical Journal, 51, 805–809.
- Voronin, A. F. (2011). A method for determining the partial indices of symmetric matrix functions. Siberian Mathematical Journal, 52, 41–53.
- Walnut, D. F. (2002). An introduction to wavelet analysis (2nd ed.). New York, NY: Birkh\"{a}user.
- Wilson, G. T. (1972). The factorization of matricial spectral densities. SIAM, Journal of applied mathematics, 23, 420–426.