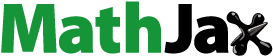
Abstract
Proper production planning can considerably improve a producer’s competitiveness in delivering low-cost products with high quality and short-cycle time. In a deterioration process, the quality may change during a production run, leading to early product failures. To mitigate the impact of deterioration such as process shifting, the optimal resetting cycle before the next setup must be determined, as well as the initial settings at the beginning of a production run. The aim of this study is to propose a mathematical model that consists of a new quality loss function and adds maintenance costs to the ordering and inventory holding costs commonly considered in the classical model. The model is applied to a real-world case study to demonstrate the effect of initial settings of the mean quality characteristic. The results demonstrate the superiority of the proposed approach in terms of total production cost, as compared with the economic production quantity model.
Public Interest Statement
Recently, the integration of production, maintenance, and quality control has attracted much attention. The deteriorating process and their maintenance have a great importance in reliability engineering. The paper proposed a new economic production quantity model to consider the production cycle time and quality characteristic to minimize total expected production cost that consists of quality loss and maintenance costs. We used the model to calculate total production cost in a spring production line in Iran. To investigate the initial effect of the mean quality characteristic, the computational analysis on a real world has been applied. The results illustrate the total production cost has been reduced from the proposed approach compared with the traditional economic production quantity model.
1. Introduction
Due to fast globalization, many manufacturers encounter tight competition in the market place. Consumers can find goods with cheaper prices, better quality, and faster delivery; consequently, production time and quality is increasingly important for competitiveness. Research has focused on production problems with constant processing time and non-deteriorated items; however, in many real industrial applications, processing times and values of products may change due to deterioration and wear phenomena such as decay, damage, spoilage, evaporation, obsolescence, pilferage, loss of utility, or loss of marginal value of commodities that result in decreasing usefulness (Wee, Citation1993). The process control and reset for deteriorating processes are challenging for production. Production planning should be adjusted according to the time control process to meet the production targets.
Hall and Eillon (Citation1963) discussed optimal production policies with two criteria: (a) maximum rate of production and (b) minimum cost. These optimal policies analyzed for processes show a linear trend in a variable dimension produced by the process with a known distribution. Gibra (Citation1967) combined the costs of resetting and nonconforming products to provide an economic model balancing resetting and not resetting. Padmanabhan and Vrat (Citation1995) proposed different economic order quantity (EOQ) models with a known and constant deterioration rate of the production process. Chen and Lin (Citation2002) developed a dynamic model to solve a deteriorating process problem with normally distributed shelf life products. Sana, Goyal, and Chaudhuri (Citation2004) determined the optimal number of production cycles of deteriorating products with a linear time-varying demand. Tadj, Bounkhel, and Benhadid (Citation2006) modeled the problem of controlling the production rate of deteriorating items for a production–inventory system. Lodree and Uzochukwu (Citation2008) derived an inventory model for a deteriorating product rate with known probability distribution.
A production process starts with an in-control policy and acceptable quality for producing conforming products. When the process starts to produce non-conforming items, preventive/regular maintenance activity should be applied to promptly mitigate and reset deterioration. Alfares, Khursheed, and Noman (Citation2005) proposed a model considering deterioration for both the produced items and the production equipment. This model integrates several realistic aspects, considering item and process deterioration, different demands and production rates, quality, maintenance and inspection. Radhoui, Rezg, and Chelbi (Citation2009) extended a joint quality control and preventive maintenance policy for a randomly failing production system producing conforming and non-conforming units, but not including a deteriorating process that transitions from conforming to nonconforming. Wee and Widyadana (Citation2013) developed a production model for deteriorating items with stochastic preventive maintenance time and rework using the first in first out (FIFO) rule.
Ollila and Malmipuro (Citation1999) present in their study that maintenance has a major impact on product quality.
Kazaz and Sloan (Citation2013) discuss how the critical ratios may be combined in order to determine the optimal policy, together with the trade-offs that involve production profits, maintenance costs, and the effect on the process condition; however, cost of poor quality is not considered. Hou and Lin (Citation2014) developed an economic production quantity (EPQ) model with finite production rate and derived an expression for the expected total annual cost function when maintenance actions and capital investment in set-up cost reduction are adopted; however, this is not considered in deterioration production process. Khan, Jaber, and Ahmad (Citation2014) represent a simple but integrated mathematical model for determining an optimal vendor–buyer inventory policy considering quality inspection errors at the buyer’s end and learning in production at the vendor’s end.
Taguchi, Elsayed, and Hsiang (Citation1989) proposed that customers become increasingly dissatisfied as performance departs farther from the target, which constitutes, a loss suffered by society. This loss can involve delay, waste scrap, etc. He suggested a quadratic curve to represent a customer’s dissatisfaction with a product’s performance (quality characteristic/metric/KPI). The Taguchi Loss Function (TLF) uses both the process average and the variation as critical measures of quality. A few studies are available for integrating preventive maintenance with combining quality loss functions. Al-Fawzan and Rahim (Citation2001) proposed a loss function, which included quadratic off-target costs, and time-based costs of maintenance and salvage value, but production policies were not considered. Chen and Jin (Citation2006) proposed a quality-oriented-maintenance model to minimize the total production cost including product quality loss function. Muchiri, Pintelon, Gelders, and Martin (Citation2011) developed a conceptual framework that provided guidelines for choosing maintenance performance indicators based on loss function, through alignment of manufacturing objectives. Chen and Huang (Citation2011) presented optimization models for determining the optimum process mean under different quality characteristic to minimize the manufacturing cost and the quality loss. Pandey, Kulkarni, and Vrat (Citation2012) developed an integrated model for joint optimization of preventive maintenance interval and control parameters incorporating the TLF. Chung, Lin, and Srivastava (Citation2014) proposed an inventory model for deteriorating items in which comprehensive analytic methods of mathematics explore the functional behaviors of APV(T); however, it is not considered loss quality function.
Ben-Daya and Makhdoum (Citation1998) studied the effect of various preventive maintenance policies on the joint optimization of the EPQ and the economic design of control chart for a deteriorating process. Subsequently, Ben-Daya (Citation2002) developed an integrated model for the joint optimization of the EPQ and the economic design of an -control chart. Chung and Hou (Citation2003) proposed a model to determine an optimal run time for a deteriorating production system under allowable shortage with EPQ. Liao, Chen, and Sheu (Citation2009) integrated maintenance and production programs with the EPQ model for an imperfect process involving a deteriorating production system with increasing hazard rate. Pan, Jin, and Wang (Citation2011) developed an extensive EPQ model considering an imperfect three-state production process. Pan, Jin, Wang, and Cang (Citation2012) then presented an EPQ model, which considers quality-related and maintenance-related costs in addition to the storage and ordering costs already considered in the classic model. The objective of their proposed model was to minimize the total production cost with the determination of EPQ and design parameters of control chart subjected to quality loss and other process costs; but in this model, a deteriorating process is not considered. Wen, Ershun, Ying, and Wenzhu (Citation2014) integrated predictive maintenance into EPQ model in which an autoregressive integrated moving average (ARIMA) time series model is adopted to predict a system’s health indicator. Shah, Patel, and Shah (Citation2015) analyzed an EPQ model with a time-varying trend in demand while the units are subject to a constant rate of deterioration.
A significant portion of the literature focused on the cost minimization using inventory models, while neglecting impact of the quality characteristic.
The rest of paper is organized as follows: Section 2 contains problem definition and represents mathematical modeling. Section 3 includes numerical example and sensitivity analysis. Finally, conclusion and future research are given in Section 4.
2. Problem definition
In this research, we propose a model to consider the production cycle time and quality characteristic so that the total expected manufacturing cost is minimized. It consists of a new quality loss function and maintenance costs other than ordering and inventory holding costs already considered in the classic model. Therefore, the purpose of this study develops a new EPQ model. The objective of the proposed model is to minimize total production cost per production cycle while simultaneously determining the interval between sampling, the sample size and maintenance decision theory. In this paper, we assumed that the process is subject to a random deterioration from an in-control state to an out-of-control state with an arbitrary distribution and, thus, produces some proportion of non-conforming items.
2.1. Mathematical modeling
The following notations and model formulation are explained throughout the paper.
μ: Initial qualitative characteristic mean (Spring constant) when the process is in control
μ*: Optimum qualitative characteristic mean (Spring constant) process (Decision variable)
T: Total production time (Decision variable)
K: Random part of loss function , A: quality loss index based on control chart, Δ: tolerance limit of control chart,
)
I: Setup cost
α: Type I error
θ: Failure rate
P: Yearly production rate
D: Yearly demand rate
h: Holding cost per unit item per time
τ: Interval time between two assignable causes
ɛ: Random part .
y(t, τ): Process quality characteristic function
μ(t, τ): Quality characteristic mean.
If t ≤ τ the process is in-control, otherwise the process is out of control
w(t, τ): Deviation function
ξ: Target value
M(t): Cost of maintenance at time t(2e0.03t)
S(t): Sinking value at time t(150e−0.9t)
ETC: Expected annual total cost function
E(L(t)): Expected value of quality loss function per unit time t
Assumptions
• | Quality Loss function is quadratic and nonlinear. | ||||
• | Lost sales cost per unit time is infinite. | ||||
• | Upside deviation in sales is considered. | ||||
• | At the end of a production period, part of a machine must be fixed or replaced. | ||||
• | Interval between two assignable causes (τ) follows an exponential distribution, i.e. | ||||
• | Maintenance cost increases based on deviation per unit time. | ||||
• | Machine value gradually decreases over time. | ||||
• | Deviation function follows a Gamma distribution. W(t, τ) = θ(t − τ), W(G(θ, λ)). |
A new expected annual total cost (ECT) function proposed, which consists of quality loss function cost, maintenance cost, sinking value, inventory holding cost, and setup cost. The production system is assumed to use the EPQ. Production processed during the T time. Expected quality loss function cost is:(1)
(1)
Since the loss function depends on τ, the expected value of quality loss function per unit time (t) is conditional and it reformulated as:(2)
(2)
Quality loss function reformulated as:(3)
(3)
Expected value of quality loss function reformulated as:(4)
(4)
Definition 1
Let X be a numerically valued random variable with expected value μ = E(X). Then the variance of X, denoted by V(X), is(5)
(5)
Using the above definition, (4) can be rewritten as:(6)
(6)
Using (1) and (6), E(L(t, τ)) reformulated as:(7)
(7)
The deviation function follows a Gamma distribution. W(t, τ) = θ(t − τ), W(G(θ, λ)), V(G(θ,λ)) = ,
and can be modeled as:
(8)
(8)
Substituting it formulated as:
(9)
(9)
The quality loss function from Equation (9) obtained for the total production time. Maintenance cost increases over time. The cumulative form is:(10)
(10)
Sinking value is decreasing over time and denoted as S(t).
Set up cost assumed to be:(11)
(11)
Inventory holding cost in EPQ formulated as:(12)
(12)
The ETC cost consists of quality loss function cost, maintenance cost, sinking value, inventory holding cost, and setup cost. The total cost per unit time modeled as follows:(13)
(13)
The optimal solution derived when:(14)
(14)
Total optimum production time (T*) can be calculated from (14). EPQ (Q*) obtained:(15)
(15)
3. Numerical example and sensitivity analysis
A real-world example and sensitivity analysis illustrates the proposed model, using a case study of a spring production line in Iran. One of the most common types of springs is the compressing spring.
The spring constant is the qualitative characteristic. Let σ = 1when the process is control, p = 70,000 annual production rate, D = 50,000 annual demand rate, I = $1,000 per unit time, h = $300 per unit per unit time, θ = 0.03, λ = 0.25, M(t) = 2e0.03t, S(t) = 150e−0.9t, A = 6.14 × 10−4, ξ = 140, α = 0.05. MATLAB 2013 software used for the calculations; the optimal total cost was calculated as $14,349, with the optimal qualitative characteristic mean process calculated as 138.43.
The results are compared with the classic EPQ model, in which the optimal total cost was $29,277. The comparison to the previous model is displayed in Table .
Table 1. Comparison of the results between the proposed and the previous model
The results demonstrate more optimal financial results from the proposed approach compared with the EPQ model. The sensitivity analysis is performed by changing parameters (I, h, θ). Figure shows that the effect of I on total production cost (ETC) and EPQ (Q). With the rising costs of setup time, production cycle and total production cost (ETC) are increasing.
Figure shows that the increase in inventory holding costs reduced total cost. This means that inverse relationships exist between inventory holding cost and total production cost.
Figure shows that increasing the θ (failure rate) leads to increase in production cycle time and total production cost. In fact, the failure rate representing the slope of the line is the deviation.
The effects of the parameters on the optimal values were determined. We observed impact of loss quality cost on the total production cost. This represents importance of a combination between production planning and control process.
4. Conclusion
In this study, an EPQ model is proposed considering the production cycle time and quality characteristic to minimize the total expected manufacturing cost that consists of quality loss and maintenance costs other than ordering and holding costs already considered in the classic model. We used this model to calculate total production cost in a spring production line in Iran. To investigate the initial effect of the mean quality characteristic, the computational analysis is applied on a real-world case study. The results demonstrate improved financial results from the proposed approach compared with the EPQ model. Proposed model decreased total production cost. The sensitivity analysis from our study shows that the total production cost is sensitive to loss quality cost. This represents the importance of a combination between production planning and control process. Future research may extend this model in an uncertain environment.
Additional information
Funding
Notes on contributors
Mohammad Hossein Zavvar Sabegh
Mohammad Hossein Zavvar Sabegh is currently an associate member at American Society for Quality (ASQ). He received his MSc degree in Industrial Engineering from Kharazmi University, Iran. He achieved the Superior student Star award between PhD and MSc students in June 2016 from Kharazmi University for his valuable researches with International Universities and professors. At Organizational Excellence Specialists (OES), he is working as a research partner on the “first global assessment on the current state of organizational excellence” that has been endorsed by the OETC. http://organizationalexcellencespecialists.ca/mohammad-hossein-zavvar-sabegh/. He is invited as a distinguished young researcher to the 8th International Conference (JIRICQRIT) 2017 by Prof. P.K. Kapur (Conference Chair). He serves at the mentioned Conference as Technical Committee http://amity.edu/jiricqrit2017/committee.html.His research interests include Quality Management, Performance and Productivity Measurement Models, Healthcare Management, Uncertain Decision Making, Supply and Operations Management. Also, he is member of IEEE. He has authored or co-authored many scientific papers and presentations. He reviewed more than 120 research papers for International peer-review journals.
References
- Alfares, H. K., Khursheed, S. N., & Noman, S. M. (2005). Integrating quality and maintenance decisions in a production-inventory model for deteriorating items. International Journal of Production Research, 43, 899–911.10.1080/0020754042000298511
- Al-Fawzan, M. A., & Rahim, M. A. (2001). Optimal control of a deteriorating process with a quadratic loss function. Quality and Reliability Engineering International, 17, 459–466.10.1002/(ISSN)1099-1638
- Ben-Daya, M. (2002). The economic production lot-sizing problem with imperfect production processes and imperfect maintenance. International Journal of Production Economics, 76, 257–264.10.1016/S0925-5273(01)00168-2
- Ben-Daya, M., & Makhdoum, M. (1998). Integrated production and quality model under various preventive maintenance policies. Journal of the Operational Research Society, 49, 840–853.10.1057/palgrave.jors.2600586
- Chen, J. M., & Lin, C. S. (2002). An optimal replenishment model for inventory items with normally distributed deterioration. Production Planning & Control, 13, 470–480.10.1080/09537280210144446
- Chen, C. H., & Huang, K. W. (2011). The determination of optimum process mean for a one-sided specification limit product with manufacturing cost and linear quality loss. Journal of Quality, 18, 19–33.
- Chen, Y., & Jin, J. (2006). Quality-oriented-maintenance for multiple interactive tooling components in discrete manufacturing processes. In Proceedings of the 2006 Annual Reliability Symposium IEEE Transactions, 55, 123–134.
- Chung, K. J., & Hou, K. L. (2003). An optimal production run time with imperfect production processes and allowable shortages. Computers & Operations Research, 30, 483–490.10.1016/S0305-0548(01)00091-0
- Chung, K. J., Lin, S. D., & Srivastava, H. M. (2014). The Inventory models for deteriorating items in the discounted cash-flows approach under conditional trade credit and cash discount in a supply chain system. Applied Mathematics & Information Sciences, 8, 2103–2111.10.12785/amis/080503
- Gibra, I. N. (1967). Optimal control of processes subject to linear trends. Journal of Industrial Engineering, 18, 35–41.
- Hall, R. I., & Eillon, S. (1963). Controlling production processes which are subject to linear trend. Operational Research Quarterly, 14, 179–189.
- Hou, K. L., & Lin, L. C. (2014). Optimal lot sizing and setup cost reduction for deteriorating production system. In Proceedings of the 2014 International Conference on Industrial Engineering and Operations Management Bali, Indonesia (pp. 827–833).
- Kazaz, B., & Sloan, T. W. (2013). The impact of process deterioration on production and maintenance policies. European Journal of Operational Research, 227, 88–100.10.1016/j.ejor.2012.11.052
- Khan, M., Jaber, M. Y., & Ahmad, A. R. (2014). An integrated supply chain model with errors in quality inspection and learning in production. Omega, 42, 16–24.10.1016/j.omega.2013.02.002
- Liao, G. L., Chen, Y. H., & Sheu, S. H. (2009). Optimal economic production quantity policy for imperfect process with imperfect repair and maintenance. European Journal of Operational Research, 195, 348–357.10.1016/j.ejor.2008.01.004
- Lodree, E. J., & Uzochukwu, B. M. (2008). Production planning for a deteriorating item with stochastic demand and consumer choice. International Journal of Production Economics, 116, 219–232.10.1016/j.ijpe.2008.09.010
- Muchiri, P., Pintelon, L., Gelders, L., & Martin, H. (2011). Development of maintenance function performance measurement framework and indicators. International Journal of Production Economics, 131, 295–302.10.1016/j.ijpe.2010.04.039
- Ollila, A., & Malmipuro, M. (1999). Maintenance has a role in quality. The TQM Magazine, 11, 17–21.10.1108/09544789910246589
- Padmanabhan, G., & Vrat, P. (1995). EOQ models for perishable items under stock dependent selling rate. European Journal of Operational Research, 86, 281–292.10.1016/0377-2217(94)00103-J
- Pan, E., Jin, Y., Wang, S., & Cang, T. (2012). An integrated EPQ model based on a control chart for an imperfect production process. International Journal of Production Research, 50, 6999–7011.10.1080/00207543.2011.642822
- Pan, E. S., Jin, Y., & Wang, Y. (2011). Integration of economic production quantity in optimization design of control chart based on loss function and random process shift. Journal of Manufacturing Technology Management, 22, 929–946.10.1108/17410381111160979
- Pandey, D., Kulkarni, M. S., & Vrat, P. (2012). A methodology for simultaneous optimisation of design parameters for the preventive maintenance and quality policy incorporating Taguchi loss function. International Journal of Production Research, 50, 2030–2045.10.1080/00207543.2011.561882
- Radhoui, M., Rezg, N., & Chelbi, A. (2009). Integrated model of preventive maintenance, quality control and buffer sizing for unreliable and imperfect production systems. International Journal of Production Research, 47, 389–402.10.1080/00207540802426201
- Sana, S., Goyal, S. K., & Chaudhuri, K. S. (2004). A production–inventory model for a deteriorating item with trended demand and shortages. European Journal of Operational Research, 157, 357–371.10.1016/S0377-2217(03)00222-4
- Shah, N. H., Patel, D. G., & Shah, D. B. (2015). EPQ model for imperfect production processes with rework and random preventive machine time for deteriorating items and trended demand. Yugoslav Journal of Operations Research, 25, 423–443.
- Tadj, L., Bounkhel, M., & Benhadid, Y. (2006). Optimal control of a production inventory system with deteriorating items. International Journal of Systems Science, 37, 1111–1121.10.1080/00207720601014123
- Taguchi, G., Elsayed, E. A., & Hsiang, T. C. (1989). Quality Engineering in Production Systems (Vol. 173). New York, NY: McGraw-Hill.
- Wee, H. M. (1993). Economic production lot size model for deteriorating items with partial back ordering. Computers & Industrial Engineering, 24, 449–458.10.1016/0360-8352(93)90040-5
- Wee, H. M., & Widyadana, G. A. (2013). A production model for deteriorating items with stochastic preventive maintenance time and rework process with FIFO rule. Omega, 41, 941–954.10.1016/j.omega.2012.12.001
- Wen, D., Ershun, P., Ying, W., & Wenzhu, L. (2014). An economic production quantity model for a deteriorating system integrated with predictive maintenance strategy. Journal of Intelligent Manufacturing, 1–11. doi:10.1007/s10845-014-0954-z