Abstract
The understanding of animal distribution in habitats located farther from water sources has not been dealt with adequately in the literature, yet this knowledge enables better prediction of species occurrence across an entire landscape. We tested whether elephant occurrence peaks away from water in addition to the known peak that is associated with water sources. We used the Maximum Entropy Modelling (MaxEnt) algorithm to predict the potential distribution of elephants in the Gonarezhou National Park, Zimbabwe. Elephant tracking data from Global Positioning System (GPS) collars were used as the response variable while NDVI (a proxy for forage quantity) and water sources data were the environmental variables. Results showed multiple peaks of elephant occurrence with increasing distance from water sources. Additionally, results illustrated that the peaks occur in high NDVI areas. Our findings emphasise the utility of GIS and remote sensing in enhancing our understanding of animal occurrence driven by water sources.
Public Interest Statement
In this paper, we sought to understand whether the African elephant utilises habitats located far from water in addition to their reported preference of places near water. Using species distribution modelling, we found out that in line with our guess, the elephants indeed extensively utilise areas away from water and these areas are characterised by green vegetation. The elephant is a large herbivore that transforms vegetation through pulling and pushing trees when browsing. Therefore, it potentially changes vegetation in areas where it spends most of its time. Elephant-driven vegetation change near water is known. However, elephant effect on vegetation far from water still needs investigation. Thus, our findings could therefore shift the attention of landscape ecologists to the highly preferred places away from water with a view to understanding whether these landscapes are also experiencing change similar to those close to water. Our study also underscores the importance of wildlife GPS tracking in enhancing knowledge on how animals utilise the landscape.
Competing interest
The authors declare no competing interest.
1. Introduction
Drinking water and forage are generally understood to be key drivers of animal distributions in tropical landscapes. This is particularly true in savannah ecosystems where vegetation cover is heterogeneous (Roever, van Aarde, & Leggett, Citation2013) and drinking water largely limiting (Chamaille James, Valeix, & Fritz, Citation2007). Animals forage in order to meet their energy and protein requirements, and drink water primarily for thermoregulatory purposes. Consequently, it is generally agreed that the movement of animals across landscapes is not random but rather follows the Optimal Foraging Theory which states that animals seek to get the most energy gain at the lowest cost as a strategy to maximise fitness (MacArthur & Pianka, Citation1966). Thus, the accurate prediction of the potential distribution of animals in most landscapes depends on the inclusion of water and forage as predictor variables.
Several studies reported that animals forage near water sources in order to minimise the distances they travel to access water (Epaphras et al., Citation2008; Follett & Delgado, Citation2002). This behaviour has largely been reported among water-dependent species that need to drink daily (Wilson, Citation1966) and is common during the hot and dry months when heat stress is enhanced by high temperatures (Coleman, Moore, & Wilson, Citation2004; Wakefield & Attum, Citation2006). For instance, African elephants (Loxodonta africana) have been observed to drink water daily (De Beer, Kilian, Versfeld, & Van Aarde, Citation2006) and also forage close to water throughout the year (Gaylard, Owen-Smith, & Redfern, Citation2003). When the probability of animal occurrence is relatively high in habitats close to water, we would expect density peaks to occur around water sources where distribution is linked to availability of water (Redfern, Grant, Biggs, & Getz, Citation2003; Shannon, Matthews, Page, Parker, & Smith, Citation2009). However, there is often a trade-off between access to water and forage as animals may have to weigh the benefits of foraging near water where the pressure on forage is high or travelling farther from water where competition on forage resources is reduced (Redfern et al., Citation2003). Despite widespread knowledge on animal occurrence near water, knowledge on how they are distributed in those habitats farther from water remains largely rudimentary. While the distribution of animals in habitats near water sources could be viewed as a strategy to maximise the intake of water, the habitat factors that drive the occurrence of animals in landscapes farther from water remain unclear. It is therefore important to also investigate the response of animals in habitats that are located farther from water sources in order to get a more complete view of animal distributions in the landscape.
In the past, studies that sought to understand the occurrence of animals in habitats near water sources relied to a great extent on point-based field measurements (e.g. Butler, Stehn, & Balogh, Citation1995; Lange, Citation1969). This approach virtually resulted in better understanding of more localised patterns that are limited to the sampled areas while ignoring those at the landscape scale. Consequently, understanding of animal occurrence dynamics near water sources has been well developed (Mukwashi, Gandiwa, & Kativu, Citation2012). Earlier studies widely reported the “piosphere effect” which predicts that the pressure of herbivory on vegetation is higher near water than farther from water (James, Landsberg, & Morton, Citation1999; Landman, Schoeman, Hall-Martin, & Kerley, Citation2012). As a result, animal-driven vegetation change near water points is well researched while the possible change farther from water has generally remained unclear. It is important to note that these earlier studies on the “piosphere effect” mostly depended on point measurements possibly because large-scale spatial data that allow for a landscape view were not readily available. In more recent years, the developments in Geographical Information Systems (GIS), remote sensing and Global Positioning Systems (GPS) have allowed the testing of hypotheses related with the potential distribution of animals at large spatial scales (Phillips, Anderson, & Schapire, Citation2006). For instance, it is now possible to obtain freely available remotely sensed data at the global scale and at relatively fine spatial resolutions which was impossible in the past (Hijmans, Cameron, Parra, Jones, & Jarvis, Citation2005). In addition, the developments in GPS collar tracking in recent years have made it possible to obtain fine grain movement data for target animal species. Thus, GIS and remote sensing can now be applied to enhance our understanding of animal occurrence near waterholes as well as farther from water.
In this study, we used elephant tracking data from GPS collars together with NDVI (proxy for forage quantity) and water sources data to test whether elephant occurrence peaks away from water in addition to the known peak that is associated with water sources. We also tested whether the peaks of elephant occurrence away from water are associated with high forage quantity (estimated using NDVI). We specifically hypothesised that elephant occurrence peaks near water in a hypothetical landscape with homogeneous vegetation (Figure (a)). This hypothetical pattern is mainly because the availability of forage will be uniform across the landscape and therefore water will be expected to have more impact on the distribution of elephants than the other predictor variables. However, in a more realistic landscape with heterogeneous vegetation cover such as the tropical savannahs, the response would be intricate and typified by multiple peaks of occurrence at increasing distance from water (Figure (b)). The other peaks farther from water would be driven by other factors like forage abundance. Our study provides important insights into the precise response of elephants to the distribution of water within landscapes characterised by heterogeneous vegetation cover.
2. Materials and methods
2.1. Ethics statement
Handling of African elephants for GPS collaring was monitored by the Zimbabwe Parks and Wildlife Management Authority as well as licensed drug handlers approved by the Division of Veterinary Services in Zimbabwe. The two departments provide and enforce the wildlife collaring guidelines for research in the country.
2.2. Study area
This study was carried out in northern Gonarezhou National Park, located between longitudes 31.75° and 32.41°E and latitudes 21.10° and 21.76°S in south-east Zimbabwe (Figure ). The study area has a typical seasonal savannah climate with long-term annual rainfall averaging 600 mm. Data collected from a weather station in the study area show that annual rainfall was 403 mm in 2009, 552 mm in 2010 and 580 mm in 2011. Rainfall is received from November to April while the rest of the year is dry (Gandiwa, Matsvayi, Ngwenya, & Gandiwa, Citation2011). Vegetation is dry deciduous savannah dominated by Colophospermum mopane and Combretum apiculatum.
Figure 2. Elephant location data (from Global Positioning System (GPS) collars) collected from the eight elephants during 13 selected months between July 2009 and November 2011 in northern Gonarezhou National Park, south-east Zimbabwe.
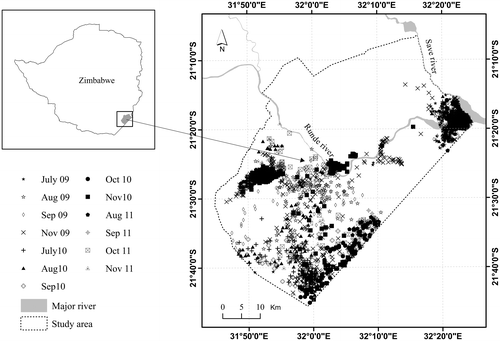
The study area lies within a protected area that has an estimated elephant population of about 10,000 (Dunham, van der Westhuizen, van der Westhuizen, & Ndaimani, Citation2013). We identified this area as ideal for answering our hypotheses because (1) data on the location of collared elephants are available for 3 years (2009, 2010 and 2011), allowing for analysis within three years of different rainfall amounts, and (2) perennial water is restricted to the major rivers especially during the dry season, thus making water an important driver of animal distribution. No artificial water sources exist in the study area, thus rivers and natural pans are the only source of drinking water for animals.
2.3. Elephant location data
Data on the location of elephants were collected during 13 selected months and the number of occurrence points used in the analyses is shown in Table . We selected the months used in the analyses based on the availability of data and those months with few occurrence points were left out.
Table 1. Elephant occurrence points used in the prediction of elephant habitat and the dates on which terra/MODIS NDVI image was acquired
Eight elephants were fitted with GPS collars in the study area in July 2009. The collars were programmed to take a minimum of three position fixes per day for a period of not less than 2 years depending on the battery life of the unit. The average fix success rate for the GPS collars during the 13 months selected for analyses was 81%. For our analyses, we used only the data collected during the 13 months presented in Table . We were particularly interested in analysing elephant distribution during the dry months when surface water is limiting in the study area. For this reason, the wetter months from December to June were not included in our analyses. Since our study area did not cover the entire range of the collared elephants, we masked out all position fixes that were located outside the study area boundary using the clip function implemented in a GIS.
2.4. Normalised difference vegetation index
NDVI data used to predict elephant habitat were extracted from the 16 days terra/MODIS data (MOD13Q1) freely available at the 250 m spatial resolution. These data are provided already corrected for water, clouds, heavy aerosols and cloud shadows (Olofsson, Eklundh, Lagergren, Jönsson, & Lindroth, Citation2007). We downloaded the data from the www.glovis.usgs.gov website where they are available for download free of charge. The dates on which these data were acquired are presented in Table . Since two composites of the 16 days data are available during each month, we selected the data acquired during the latter half of the month for consistency. Geometric corrections included conversion from the sinusoidal (SIN) to the Universal Transverse Mercator (UTM, WGS 84 Zone 36S) projection to ensure compatibility with the elephant location data. Two tiles (h20v11 and h21v11) were required to cover the entire study area. We later mosaicked and clipped the images to the size of the study area. In this study, we used NDVI as a proxy for forage quantity following Garroutte, Hansen, and Lawrence (Citation2016). Forage quantity is an important habitat variable for elephants during the drier months of the year because it is largely limiting at that time especially in savannah landscapes.
2.5. Distance from water sources
In order to determine the water sources available in the study area during the months included in the analyses, we used 16 days MODIS NDVI data described above. NDVI values range from −1 to+1, where negative values are generally associated with water bodies and positive values with vegetation. Thus, it is possible to use a threshold approach to extract water surfaces from NDVI data. We extracted water surfaces from the monthly generated NDVI following the method described by Huang, Li, and Xu (Citation2012), where a pixel is considered water when NDVI is less than zero. Although other water extracting indices have been proposed such as the Modified Normalised Difference Water Index (Xu, Citation2006), we could not use the index since it uses the green and mid-infrared bands which are not available on the MOD13Q1 product. While Landsat images have these bands, non-availability of cloud free images limited our analysis to MODIS images whose availability is enhanced by their high temporal resolution. We did not evaluate the accuracy of the resultant classification since we did not have an independent field-based data-set for validation. However, the method used in our study has been used elsewhere (e.g. Kameyama et al., Citation2004) and has yielded reasonably accurate results in those landscapes thus justifying its use.
After extracting the water surfaces for each of the study months, we then calculated the distance of individual pixels from the nearest water sources using the Euclidian distance calculation algorithm implemented in a GIS.
2.6. Modelling probability of elephant occurrence
We modelled the potential distribution of elephants in the study area using the Maximum Entropy Modelling (MaxEnt) algorithm. MaxEnt is a general-purpose machine learning method that has widely been used in species distribution modelling and has been observed to perform better than other methods (Elith & Graham, Citation2009). We used elephant occurrence data from the eight GPS collars described above as the response variable while NDVI and distance from water were used as the predictor variables. The two predictor variables were used in the models since they are widely accepted as key drivers of elephant distribution in most landscapes. Overall, we built 13 models, a model for each of the selected 13 months. The option for removing duplicate presence records was selected to ensure that MaxEnt does not sample the same pixel several times.
The Area Under Curve (AUC) of the Receiver Operating Curve (ROC) was used to validate the ability of individual models to predict elephant occurrence. A model was observed as adequate, very good and excellent when the AUC was 0.70–0.80, 0.80–0.90 and AUC = 0.90–1.00, respectively (Panczykowski et al., Citation2012).
Graphs showing the response of elephants to distance from water sources were used to determine peaks of elephant occurrence farther from water sources. The peaks were defined as the points where the predicted probability of occurrence was above the logistic threshold of equal training sensitivity and specificity. The distance from water at which elephant occurrence peaked was extracted from the response curves. The same procedure was followed for detecting peaks of elephant occurrence in relation to NDVI. Next we extracted NDVI and elephant probability of occurrence values at the elephant position fixes used for modelling. The extracted NDVI values were then plotted against extracted probabilities of elephant occurrence and polynomials fitted to the data. To establish whether the peaks of elephant occurrence coincided with high NDVI, we plotted elephant response to water on the same axis with the polynomials relating NDVI to distance from water.
3. Results
Results show that the two predictors, distance from water sources and NDVI, explained most of the variation in the probability of occurrence of elephants across the landscape (Table ). However, it can be observed that distance from water consistently predicts elephant distribution better than NDVI over the three-year period. We observe that the AUC of the ROC curves from the models can be rated adequate (August 2009, September 2009, November 2009, August 2010, September 2010 and October 2010), very good (July 2009, July 2010, November 2010, August 2011, October 2011 and November 2011) and excellent (September 2011).
Table 2. Performance and variable contribution of the MaxEnt models predicting the geographical distribution of elephants in the GNP during the dry season months of 2009, 2010 and 2011
Response curves illustrate that elephant occurrence consistently peaked twice with distance from water sources (Figure ). However, this pattern is not observed in October 2010 and September 2011 where elephant occurrence peaks three times and once, respectively. Overall, the first peak is located near water (not more than 9 km away) while the other is farther from water (more than 50 km away) (Table ).
Figure 3. Probability of elephant occurrence (P(E)) plotted against distance from water sources and NDVI from July 2009 to November 2011. Elephant occurrence generally peaked at least twice with distance from water sources.
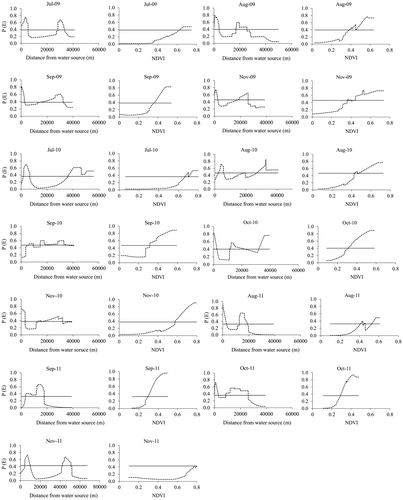
Table 3. Peaks of elephant occurrence away from water sources and minimum NDVI within elephant habitat (habitat = probability values > the logistic threshold of equal training sensitivity and specificity)
It can also be observed that high probability of elephant occurrence coincides with high NDVI values (Figure ). In addition to this observation, response curves illustrating elephant occurrence plotted against distance from water peaked consistently with those showing NDVI plotted against distance from water (Figure ). NDVI values in areas predicted as suitable for elephants range from a minimum of 0.28 in October 2011 to 0.80 in November 2011 (Table ). These NDVI values are observed to be generally higher in July and November than the rest of the months under study.
4. Discussion
Results in this study seem to suggest that elephant occurrence peaks farther from water in addition to the expected peak near water sources. This therefore confirms our main hypothesis that in a heterogeneous landscape characterised by patchy vegetation cover, elephant occurrence peaks more than once with distance from water sources in response to forage resource clustering. This is because evidence from our analyses suggests that the patches selected by elephants farther from water are characterised by high NDVI values, i.e. high forage (Figure ). In fact, elephant occurrence peaked in patches with minimum NDVI values of about 0.44 and these areas are generally classified as forests (Nemani & Running, Citation1997). This is not surprising as elephants are non-selective bulk feeders that often select highly productive patches (i.e. high NDVI) either for foraging (Ruggiero, Citation1992) or for thermoregulation under tree canopies (i.e. high NDVI) (Kinahan, Pimm, & van Aarde, Citation2007). Our results seem to contradict earlier studies that reported that elephants select landscapes of intermediate vegetation cover (Gara et al., Citation2017; Roever et al., Citation2013). Based on our findings, we thus deduce that elephant occurrence peaks in areas with high forage quantity farther from water and this could be for foraging purposes.
Our results also suggest that while both water and forage are key predictors of elephant occurrence, the availability of water explains the distribution of elephants better than vegetation cover (estimated by NDVI) (Table ). This finding is supported by earlier studies where elephants have been reported to drink water daily (De Beer et al., Citation2006; Viljoen, Citation1989) and stay within 20 km of water (Loarie, Van Aarde, & Pimm, Citation2009). This is particularly common during the hotter months of the year when demand for water is high. In this regard, data used in our analyses were also collected during hotter months of the year making the reported importance of water not surprising. Our findings therefore unsurprisingly reinforce the notion that elephants are water dependent since the first peak in elephant occurrence was generally observed at less than 9 km away from water, although during selected months they ventured further than 50 km away (Table ).
The finding that elephant occurrence peaks farther from water in addition to the peak close to water is of particular interest to landscape ecologists since elephant-induced vegetation change has historically been well explained in landscapes close to water than those farther away. Owing to local overabundance of elephants near water, most studies have reported elephant-induced vegetation change linked to water sources (Chamaille James et al., Citation2007; De Beer et al., Citation2006). However, following our findings, attention could now be directed to the hotspots of elephant occurrence farther from water to test whether these landscapes could also be experiencing elephant-induced vegetation change in a similar way to those landscapes closer to water. Our findings therefore form the basis for formulating hypotheses that test for elephant-induced vegetation change in landscapes located farther from water.
Results from our study represent observations largely made during the dry season. Application of the findings to the wet season should therefore be done with caution. Even though our observations pertain to the dry season, we however emphasise that an understanding of elephant distribution during that time of the year is critical since their ranging behaviour is highly influenced by the availability of surface water (De Beer & van Aarde, Citation2008). In addition, while our study has been successful in reporting multiple peaks of elephant occurrence farther from water using dry season data, future studies could extend this analysis to the wet season to establish whether similar findings can be made when surface water is abundant. Other work could involve tracking elephants in the field to establish whether they select high NDVI patches for forage, thermoregulation or any other purpose. Despite our effort to remove spatial dependence in elephant occurrence data using the “remove duplicate presence points” option in MaxEnt, future work could use more robust methods (e.g. spatial filtering) to deal with spatial bias.
5. Conclusion
In this study, we reported multiple peaks of elephant occurrence farther from water. We also reported that these areas of peak occurrence are associated with high NDVI. Our findings are among the first to provide empirical evidence for the existence of multiple peaks of elephant occurrence farther from water during the dry season. Results from our study provide the basis for formulating hypotheses that test for elephant-induced vegetation change in peak elephant occurrence patches located farther from water sources.
Supplementary material
Supplementary material for this article can be accessed here https://doi.org/10.1080/23311843.2017.1420364.
Funding
The authors received no direct funding for this research.
S1.tiff
Download TIFF Image (1.4 MB)Acknowledgements
We thank the Frankfurt Zoological Society as well as the Gonarezhou Conservation Trust for allowing us to use data from the collared elephants in our analyses. The Zimbabwe Parks and Wildlife Management Authority are also thanked for giving us permission to publish this work.
Additional information
Notes on contributors
Henry Ndaimani
Henry Ndaimani was born in Zaka, Zimbabwe, in 1979. He received his BSc degree in Geography at the University of Zimbabwe in 2003 and an M.Phil degree from the same university in 2012. Currently, he is studying for a PhD from the University of Zimbabwe.
From 2005 to 2014, he worked as a terrestrial ecologist for the Zimbabwe Parks and Wildlife Management Authority. From 2014 to date, he worked as a Staff development Fellow with the UZ, Department of Geography and Environmental Science (DGES). He is the author of 12 peer-reviewed articles. His research interests include Spatial Ecology as well as remote sensing applications to wildlife ecology.
References
- Butler, Jr., W. I., Stehn, R. A., & Balogh, G. R. (1995). GIS for mapping waterfowl density and distribution from aerial surveys. Wildlife Society Bulletin, 23, 140–147.
- Chamaille James, S., Valeix, M., & Fritz, H. (2007). Managing heterogeneity in elephant distribution: Interactions between elephant population density and surface water availability. Journal of Applied Ecology, 44, 625–633.10.1111/j.1365-2664.2007.01300.x
- Coleman, S. W., Moore, J. E., & Wilson, J. W. (2004). Quality and utilization. Warm-Season (C4) Grasses. Agronomy Series, 45, 267–308.
- De Beer, Y., Kilian, W., Versfeld, W., & Van Aarde, R. J. (2006). Elephants and low rainfall alter woody vegetation in Etosha National Park, Namibia. Journal of Arid Environments, 64, 412–421.10.1016/j.jaridenv.2005.06.015
- De Beer, Y., & van Aarde, R. J. (2008). Do landscape heterogeneity and water distribution explain aspects of elephant home range in southern Africa’s arid savannas? Journal of Arid Environments, 72, 2017–2025.10.1016/j.jaridenv.2008.07.002
- Dunham, K., van der Westhuizen, E., van der Westhuizen, H., & Ndaimani, H. (2013). Aerial survey of elephants and other large herbivores in Gonarezhou National Park (Zimbabwe), and surrounding areas: 2013. Zimbabwe Parks and wildlife Management Authority.
- Elith, J., & Graham, C. H. (2009). Do they? How do they? WHY do they differ? On finding reasons for differing performances of species distribution models. Ecography, 32, 66–77.10.1111/eco.2009.32.issue-1
- Epaphras, A., Gereta, E., Lejora, I., Meing’ataki, G. O., Ng’umbi, G., Kiwango, Y., … Balozi, J. (2008). Wildlife water utilization and importance of artificial waterholes during dry season at Ruaha National Park, Tanzania. Wetlands Ecology and Management, 16, 183–188.10.1007/s11273-007-9065-3
- Follett, R., & Delgado, J. (2002). Nitrogen fate and transport in agricultural systems. Journal of Soil and Water Conservation, 57, 402–408.
- Gandiwa, P., Matsvayi, M., Ngwenya, M. M., & Gandiwa, E. (2011). Assessment of livestock and human settlement encroachment into northern Gonarezhou National Park, Zimbabwe. Journal of Sustainable Development in Africa, 13, 19–33.
- Gara, T. W., Wang, T., Skidmore, A. K., Zengeya, F. M., Ngene, S. M., Murwira, A., & Ndaimani, H. (2017). Understanding the effect of landscape fragmentation and vegetation productivity on elephant habitat utilization in Amboseli ecosystem, Kenya. African Journal of Ecology, 55, 259–269.10.1111/aje.2017.55.issue-3
- Garroutte, E. L., Hansen, A. J., & Lawrence, R. L. (2016). Using NDVI and EVI to map spatiotemporal variation in the biomass and quality of forage for migratory Elk in the greater yellowstone ecosystem. Remote Sensing, 8, 404.10.3390/rs8050404
- Gaylard, A., Owen-Smith, N., & Redfern, J. (2003). Surface water availability: Implications for heterogeneity and ecosystem processes: The Kruger experience: Ecology and management of savanna heterogeneity. Washington: Island Press.
- Hijmans, R. J., Cameron, S. E., Parra, J. L., Jones, P. G., & Jarvis, A. (2005). Very high resolution interpolated climate surfaces for global land areas. International Journal of Climatology., 25, 1965–1978.10.1002/(ISSN)1097-0088
- Huang, S., Li, J., & Xu, M. (2012). Water surface variations monitoring and flood hazard analysis in Dongting Lake area using long-term Terra/MODIS data time series. Natural Hazards, 62, 93–100.10.1007/s11069-011-9921-6
- James, C. D., Landsberg, J., & Morton, S. R. (1999). Provision of watering points in the Australian arid zone: A review of effects on biota. Journal of Arid Environments, 41, 87–121.10.1006/jare.1998.0467
- Kameyama, S., Zhang, J., Wang, Q., Xu, K., Katoh, T., & Watanabe, M. (2004). An approach to estimate the water level and volume of Dongting Lake using Terra/MODIS data. Acta Geographica Sinica-Chinese Edition, 59, 88–94.
- Kinahan, A., Pimm, S. L., & van Aarde, R. J. (2007). Ambient temperature as a determinant of landscape use in the savanna elephant, Loxodonta africana. Journal of Thermal Biology, 32, 47–58.10.1016/j.jtherbio.2006.09.002
- Landman, M., Schoeman, D. S., Hall-Martin, A. J., & Kerley, G. I. (2012). Understanding long-term variations in an elephant piosphere effect to manage impacts. PLoS One, 7, e45334.10.1371/journal.pone.0045334
- Lange, R. T. (1969). The piosphere: Sheep track and dung patterns. Journal of Range Management, 22, 396–400.10.2307/3895849
- Loarie, S. R., Van Aarde, R. J., & Pimm, S. L. (2009). Fences and artificial water affect African savannah elephant movement patterns. Biological Conservation, 142, 3086–3098.10.1016/j.biocon.2009.08.008
- MacArthur, R. H., & Pianka, E. R. (1966). On optimal use of a patchy environment. American Naturalist, 100, 603–609.10.1086/282454
- Mukwashi, K., Gandiwa, E., & Kativu, S. (2012). Impact of African elephants on Baikiaea plurijuga woodland around natural and artificial watering points in northern Hwange National Park, Zimbabwe. International Journal of Environmental Sciences, 2, 1355–1368.
- Nemani, R., & Running, S. (1997). Land cover characterization using multitemporal red, near-IR, and thermal-IR data from NOAA/AVHRR. Ecological Applications, 7, 79–90.10.1890/1051-0761(1997)007[0079:LCCUMR]2.0.CO;2
- Olofsson, P., Eklundh, L., Lagergren, F., Jönsson, P., & Lindroth, A. (2007). Estimating net primary production for Scandinavian forests using data from Terra/MODIS. Advances in Space Research, 39, 125–130.10.1016/j.asr.2006.02.031
- Panczykowski, D. M., Puccio, A. M., Scruggs, B. J., Bauer, J. S., Hricik, A. J., Beers, S. R., & Okonkwo, D. O. (2012). Prospective independent validation of IMPACT modeling as a prognostic tool in severe traumatic brain injury. Journal of Neurotrauma, 29, 47–52.10.1089/neu.2010.1482
- Phillips, S. J., Anderson, R. P., & Schapire, R. E. (2006). Maximum entropy modeling of species geographic distributions. Ecological Modelling, 190, 231–259.10.1016/j.ecolmodel.2005.03.026
- Redfern, J. V., Grant, R., Biggs, H., & Getz, W. M. (2003). Surface-water constraints on herbivore foraging in the Kruger National Park, South Africa. Ecology, 84, 2092–2107.10.1890/01-0625
- Roever, C. L., van Aarde, R. J., & Leggett, K. (2013). Functional connectivity within conservation networks: Delineating corridors for African elephants. Biological Conservation, 157, 128–135.10.1016/j.biocon.2012.06.025
- Ruggiero, R. (1992). Seasonal forage utilization by elephants in Central Africa. African Journal of Ecology, 30, 137–148.10.1111/j.1365-2028.1992.tb00487.x
- Shannon, G., Matthews, W. S., Page, B. R., Parker, G. E., & Smith, R. J. (2009). The affects of artificial water availability on large herbivore ranging patterns in savanna habitats: A new approach based on modelling elephant path distributions. Diversity and Distributions, 15, 776–783.10.1111/ddi.2009.15.issue-5
- Viljoen, P. (1989). Spatial distribution and movements of elephants (Loxodonta africana) in the northern Namib Desert region of the Kaokoveld, South West Africa/Namibia. Journal of Zoology, 219, 1–19.
- Wakefield, S., & Attum, O. (2006). The effects of human visits on the use of a waterhole by endangered ungulates. Journal of Arid Environments, 65, 668–672.10.1016/j.jaridenv.2005.08.007
- Wilson, A. (1966). The intake and excretion of sodium by sheep fed on species of Atriplex (Saltbush) and Kochia (Bluebush). Australian Journal of Agricultural Research, 17, 155–163.10.1071/AR9660155
- Xu, H. (2006). Modification of normalised difference water index (NDWI) to enhance open water features in remotely sensed imagery. International Journal of Remote Sensing, 27, 3025–3033.10.1080/01431160600589179