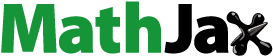
Abstract
The authors discuss the results of a study that was conducted on the factors that enhance effective interaction between students and learning contents in a technology-mediated learning environment in a higher education setting. The results revealed that effective student–content interaction is strongly enhanced by expansive learning through its five key elements; epistemic relation, contradictions, digital learning content, conglomeration of views and cognitive transformation. The intervening effect of network relations and mediating technology was also highlighted therefore, revealing the positive impact of both factors in enhancing effective student-content interaction. The empirical methods employed by the authors were focus group interview (n = 12) and questionnaire (n = 500) among students in a university. While Structural equation modelling was used to analyse the quantitative data, the focus group interviews were analysed using categories, themes and concepts. The authors present the findings and implications of the study by way of theory, practice and further research in the study.
Public Interest Statement
The introduction of digital learning systems in higher education all over the world has transformed the scope of teaching and learning significantly. While policy-makers, providers of education and developers of online learning management systems continue to find ways of improving teaching and learning in technology-mediated learning environments, it has become necessary to investigate the factors that promote student-content interaction. The outcome of this study shows that expansive learning provides a strong framing for effective interaction between students and the learning content deployed in a technology-mediated learning environment. Five elements of expansive learning; epistemic relation, contradictions, digital learning content, conglomeration of views and cognitive transformation all demonstrated stronger impact on student–content interaction. Additionally, mediating technology and network relations also served as intervening factors that support effective student–content interaction. The results suggest that, in developing the learning content of technology-mediated courses, providers of education should also focus on how students could obtain and apply new knowledge in their environment through the five elements of expansive learning.
1. Introduction
Teaching and learning in higher education institutions (HEIs) has evolved to include the role of technology-mediated learning systems in the development of the knowledge and skills of students. Although education providers focus on eventuating the aims of education through pedagogical innovation, it has become necessary to explore the critical factors that enhance knowledge and skills development in a technology-mediated learning environment. Previous research by some authors (Anderson, Citation2011; So & Brush, Citation2008) show that, interaction in a digital learning environment could take three different forms: learner–instructor interaction, learner–content interaction and learner–learner interaction. While the learner–learner interaction refer to communication between learners in a learning environment, learner–instructor interaction refer to the communication between learners and instructors (Zimmerman, Citation2012). However, Woo and Reeves (Citation2007) show that interaction alone does not necessarily lead to effective learning, rather, where interaction is directly connected to the intellectual growth of learners, then learning becomes impactful and meaningful. Donnelly (Citation2010, p. 351) also suggest that there is a qualitative dissimilarity between teaching courses online and merely putting a course online.
This study, therefore, focuses on exploring the relevant factors that promote interaction between students and contents in a digital learning environment. In order to explicate the importance of the phenomenon under study, we seek answers to two critical questions: (a) what factors promote effective student–content interaction in digital learning? and (b) how can student–content interaction stimulate the development of the knowledge and skills of students in a digital learning environment? Using an adapted third-generation activity theory as the theoretical underpinning of this study, the authors discuss the various factors that promote student–content interaction and how the knowledge and skills of students could be enhanced in a digital learning environment.
The first section of this study discusses third generation activity system as the theoretical underpinning of the study. Importantly, the subject, instrument, community, and object (Engeström, Citation2000, 2001; Isssroff & Scanlon, Citation2002; Scanlon & Issroff, Citation2005) are important in explicating the importance of student–content interaction in a digital learning environment. We further discuss three essential factors that promote student–content interaction in a digital learning environment: mediating technology, expansive learning and network relations while showing how a synthesis of the factors could support the knowledge and skill development of students. Through empirical methods of collecting and analysing data, we highlight the theoretical, practical, and knowledge gaps that exist in developing a robust interaction between students and the learning content in a digital learning environment. We confer and show our results in the analysis and discussion sections.
2. Theoretical review
Activity theory has a long tradition as a theoretical perspective that explains the rules governing the behaviour of individuals in society. Today, the rules that regulate the behaviour of actors in a technology-mediated learning environment draws on social and cultural activities (Engeström & Sannino, Citation2010). Invariably, the tools, subject, object, and community of an activity system defines the social and cultural activities. From a cultural perspective and in the context of digital learning, the tools represent the mediating artefacts and the knowledge that are pertinent to their continuous use (Engeström & Sannino, Citation2010). These tools also affect the way students interact with the system to acquire knowledge and use them practically. Donnelly (Citation2010) posits that the degree of technology-mediation, self-regulation, independence and value of facilitation by the facilitator are essential for the development of online content for students. The subject in an activity system defines the role of students and sub-groups whose agency is selected as the analytical point of view (Hasu & Engeström, Citation2000) in a community. A study by Hrastinski (Citation2009) revealed that online learner participation requires engagement in a learning environment that promotes the formulation and reformulation of knowledge. Through an activity system, students are actively involved in knowledge transfer that is also enhanced by appropriate learning resources (Akyol, Citation2014; Graham, Citation2013) and sustained effort to promote participation (Hrastinski, Citation2009). Interaction also enhance active participation by students in a digital learning environment (Allen & Seaman, Citation2014; Anderson, Citation2011; Bazelais, Doleck, & Lemay, Citation2018; So & Brush, Citation2008) thereby promoting knowledge sharing among students. The object-orientedness in an activity theory defines the goals of learners in a digital learning environment. Although the object in a technology mediated learning system is useful for the development of the knowledge and skills of learners, it does not explain how learners learn or participate in an online setup (Larbi-Siaw & Owusu-Agyeman, Citation2017). Providers of education need to move beyond the physical resources that support teaching and learning and also focus on how students obtain relevant knowledge and skills that are pertinent to the personal development and careers through technology-mediated processes (Alammary, Sheard, & Carbone, Citation2014; Porter & Graham, Citation2016). In juxtaposing the effect of student–content interaction on digital learning systems, the authors argue that the development of online learning content requires several factors such as: students’ knowledge of internet usage, learning objectives and students’ adaptation to the digital learning environment. Additionally, we argue that by sharing with others in a community, students acquire new knowledge which is essential to their epistemic development. The community, therefore, promotes all forms of network relations that essential to knowledge and skills acquisition in the learning environment.
3. Conceptual framework
Drawing from the theoretical discussions, the authors identify three key factors that influence student–content interaction in a digital learning environment; expansive learning, mediating technology and network relations. Additionally, by way of group interaction (Barkely, Citation2009; Price, Handley, & Millar, Citation2011), the content of an online learning course should promote the critical thinking abilities of students. The next paragraph discusses the factors that promote student–content interaction in a digital learning environment.
3.1. Expansive learning
Expansive learning explains how learners learn new things for their collective activity (Engeström & Sannino, Citation2010) that are not known to others and are able to practically demonstrate such new knowledge or skills in their environment. Contrastingly, Felstead et al. (Citation2005) argue that expansive learning only provides additional interpretation of the participation metaphor that is essential in learning. However, using an adapted activity system principles: digital learning content, conglomeration of views, epistemic relation, contradictions (Engeström, Citation2001), and cognitive transformation (Giordano, Cernkovich, & Rudolph, Citation2002), we discuss the theoretical importance of expansive learning in student–content interaction.
The first principle we identify is the digital learning content that is synonymous to what Engeström (Citation2001) refers to as element. The digital learning content explicate factors such as: discussion threads, timely participation, cooperative learning and innovative learning (see Hrastinski, Citation2008; So & Brush, Citation2008) that are necessary to the development of student–content interaction. The second principle is conglomeration of views that are found in the learning environment. Group discussions, chats and group activities carried out in an online learning environment serve to collate diverse opinion and views on different topics. Epistemic relation (Bernstein, Citation1999) provides explanation to the knowledge structures that are developed by learners in the learning process. In the context of technology-mediated learning, epistemic relation refers to the knowledge change that takes place through the use of mediating technology in the teaching and learning process (see Owusu-Agyeman & Larbi-Siaw, Citation2017). Importantly, epistemic relation provides a more advanced explanation of how the intrinsic make up of individuals, their mental processes and cultural characteristics are observed and assessed in a learning environment.
While epistemic relation takes account of the changes that take place in an activity system through continuous redesigning and mental reframing, the fourth principle identifies contradictions (see Engeström, Citation2001) that emerge from the activity system as sources of change and development. The contradictions that arise from the online learning platform serve as the basis for developing interventions that are intended to strengthen the teaching and learning processes. While students interact with their colleagues in groups and with the content, they begin to identify the contradictions that exist in the theories they learn and also question anomalies in the digital learning system. Importantly, emerging technologies are often the product of contradictions that arise from the continuous use of technology overtime. The fifth principle is cognitive transformation (Giordano et al., Citation2002) in a digital learning system. Cognitive transformation in a digital learning system occurs when students reorganise their thoughts through a process of knowledge formulation and reformulation to produce an outcome that is different from what was originally designed by education by the facilitators.
3.2. Mediating-technology
The mediating technology in an online learning environment serves as the processing domain of information among actors in a group (Eom, Wen, & Ashill, Citation2006; Hrastinski, Citation2008; So & Brush, Citation2008; Wu & Hiltz, Citation2004). Tools such as Prezi, Dipity, Thinglink, Animoto, Cacoo and Flashcard Exchange enable students to engage with online content. Additionally, learning management systems (software) and computers serve as important domains for promoting student–content interaction. When students use robust learning devices and find the learning management system user friendly, they are able to interact with the content easily within the community. Elements such as course structure, interface or page design, content engagement and usability define the structure of the mediating tool that strengthens teaching and learning processes in an online learning environment (Alammary et al., Citation2014; Porter & Graham, Citation2016; Tay, Citation2016). Therefore, interaction among students in a technology-mediated environment promotes; knowledge transfer among learners, discussion threads, participation, brainstorming, cooperative learning, group cohesion and dialogue.
3.3. Network relations
In the context of this study, network relation is synonymous with social relation which is defined as the transaction between the subject making the claim to knowledge and knowledge (Maton, Citation2004) which provides a sense of intimacy and immediacy (So & Brush, Citation2008). Learning is not solely an individual activity but a collectively shared process with significant cultural and historical dimensions (Barab, Evans, & Baek, Citation2004; Stetsenko, Citation1999), therefore, the need for network relations. Network relation in a digital learning environment is enhanced by: discussing content with colleagues and facilitators; reflecting on the content and providing answers to questions that are put on the learning platform (Akyol, Citation2014; Allen & Seaman, Citation2014; Hrastinski, Citation2009; Owusu-Agyeman & Larbi-Siaw, Citation2017; Remesal & Colomina, Citation2013). In amplifying the importance of network relation, the authors posit that facilitators provide students with relevant information and direction that are required in the teaching and learning processes. Wu and Hiltz (Citation2004), however, show that the role of the facilitator in stimulating learners’ interest in a technology-mediated environment could be considered as one of the primary roles in meeting the expectations of learners and promoting group discussion. Similarly, an effective learner–instructor interaction in a technology-mediated environment is driven by; strong management support, uninterrupted internet connectivity and a robust digital learning platform that is interactive and user support services (Owusu-Agyeman, Larbi-Siaw, Brenya, & Anyidoho, Citation2017, p. 53). Moore (Citation1993), however, encapsulates the three-way interaction: learner–instructor interaction, learner–content interaction, and learner–learner interaction by arguing that it allows learners to develop their knowledge through effective exchange of ideas in an educational setting.
The student–content interaction espouses the principle underlying human computer interaction in a learning environment. When students interact with the learning content online, it affords them the space to reflect on the content and also develop their own understanding of what they read (Anderson, Citation2011; Zimmerman, Citation2012). The learner–learner interaction on the other hand serves to provide students with a space to interact and share ideas through digital means. While interaction alone cannot provide students with the knowledge and skills they need (Woo & Reeves, Citation2007), it is important for facilitators to ensure that students are actively engaged and also develop their knowledge in an online learning environment (Donnelly, Citation2010).
4. Research questions and hypotheses
In order to explore the underlying features regarding student–content interaction in a digital learning environment, we developed research questions and a set of hypothesis to guide the study. The research questions (RQ) are: What factors promote student–content interaction in digital learning (RQ1)? And how can student–content interaction stimulate the development of the knowledge and skills of students in a digital learning environment RQ2)? Additionally, the following research hypothesis sought to provide further answers to RQ1;
Hypothesis 1
H1a: There is a statistically positive and significant relationship between mediating technology and student-content interaction.
H1b: Mediating technology enhances digital learning content.
Hypothesis 2
H2a: There is a statistically positive and significant relationship between network relations and student-content interaction.
H2b: Conglomeration of views is enhanced by network relations.
Hypothesis 3
H3a: There is a statistically positive and significant relationship between digital learning content and student-content interaction.
H3b: There is a statistically positive and significant relationship between conglomeration of view and student-content interaction.
H3c: There is a statistically positive and significant relationship between epistemic relation and student-content interaction.
H3d: There is a statistically positive and significant relationship between contradictions and student-content interaction.
H3e: There is a statistically positive and significant relationship between cognitive transformation and student-content interaction.
5. Materials and methods
This study relied on survey and interview schedules to provide a triangulation of the data-sets that were collected and analysed. The research methodology used in this study was consistent with the procedures used in conducting both quantitative and qualitative study (see Cohen, Manion, & Morrison, Citation2011; Lindlof, Citation1995) in a higher education setting.
5.1. Participants
The subjects in this study were second- and third-year students who were reading courses that were deployed by the Centre for Online Learning and Teaching (COLT) through face to face and online learning modes. The population in the study was five thousand and eight (5,008) undergraduate students in a university in Ghana who were pursuing different programmes at the faculties of engineering, computing and information system as well as information technology business. Using a probability sampling method (simple random sampling), the authors distributed 700 questionnaire to students out of which we received 521. However, the number of valid survey responses was 500 and this represented a valid response rate of 71.4%. Analysis of the data gathered was done using structural equation model and this is explained in detail in the results section. Non-probability sampling method (purposive sampling) was used in selecting interviewees for the focus group discussion (n = 12) and this consisted of three groups each from the three faculties. The interviewees (four from each faculty) were carefully selected by the researchers and this was mostly among students who were considered as very knowledgeable in the application of online learning technology and who contributed to discussion in class.
5.2. Instrument
The measures for all the constructs were culled validated instruments from different studies and modified to suit the digital learning environment. Seeing that the survey respondents were required to provide their responses on a self-report instrument, different prior relevant studies with similar variables and theoretical focus were reviewed to enable us capture all the measures that were required for this study. Primarily we derived the survey constructs from 9 key variables: expansive learning, digital learning content, conglomeration of views, contradictions, epistemic relation, cognitive transformation, student–content interaction, network relations and mediating-technology. Additionally, all items were measured on a five-point likert scale ranging from 1 (strongly agree) to 5 (strongly disagree). After developing the questionnaire, we proceeded with an iterative interview process with the instructional designer, the lecturers on the programme, some students who were taking courses in the online learning environment as well as some administrative staff who helped in facilitating the programme. The feedback we gathered from the interviewees was used to correct, refine, reify and alter some of the constructs. Reliability of the data-sets was also strengthened through a regimented procedure of gathering data from students (Lindlof, Citation1995; Patton, Citation2002; Teddlie & Tashakkori, Citation2009). Additional information on reliability of the constructs is provided in the analysis section of this study. We followed the rules of ethical consideration in conducting research first by seeking the consent of respondents to participate in the study and also avoided bias in interpreting the responses provided by respondents. Due to the low-response rate we recorded following a pilot study we conducted through the online mode, we resorted to using the paper mode of gathering data from respondents.
5.3. Procedure
The focus group interview consisted of four key discussion categories; expansive learning, network relations, mediating technology and student–content interaction. The interviewees from the various faculties are identified by the following abbreviations: Engineering—ENG; Informatics—INF and IT Business—ITB. The focus group discussion focused on providing relevant information on how student–content interaction could stimulate the development of the knowledge and skills of students in a digital learning environment.
Secondly, the authors employed a two-step approach to structural equation modelling (SEM) as suggested by Anderson and Gerbing (Citation1988) using analysis software; Statistica. The first process was the adoption of confirmatory factor analysis (CFA) to gauge the structure of the measurement models that also sought to explicate the importance of student–content interaction in a digital learning environment. Due to the significant sample size of large datasets, we divided the data into a considerably smaller number of factors while ensuring a minimum loss of information (Hair, Anderson, Tatham, & Black, Citation2010). We then proceeded to use SEM to evaluate the structural model that also tests for full and partial mediation of student–content interaction on the dependent variables. In order to demonstrate the model’s strength to reveal the relationship between the various variables, the authors undertook series of measurement tests beginning with the factor and correlational analysis. The mediator variables for the model consist of Mediating Technologies (MT) and Network Relations (NR). The other variables in the model are: Digital Learning Content (DLC), Conglomeration of views (COV), Epistemic Relation (ER), Contradictions (CTR), Cognitive Transformation (CTD) and Student–Content Interaction (SCI). The procedure below looks at the equations for the model’s path coefficients:
Equation (1) delineates the path between mediating technologies and student–content interaction.(1)
(1)
(2)
(2)
Equation (2) delineates the path between mediating technologies and digital learning content.(3)
(3)
Equation (3) delineates the path between network relations and student–content interaction.(4)
(4)
Equation (4) delineates the path between conglomeration of views and student–content interaction.
Equation (5) delineates the path between activity theory (DLC, COV, ER, CTD, CTR) and student–content interaction.(5)
(5)
SCI = Student Content Interaction, MT = Mediating Technologies, DLC = Digital Learning Content, NR = Network Relations, COV = Conglomeration of Views, CTD = Contradictions, CTR = Cognitive Transformation β, ϒ = Measurement Parameter, ζ = Indicates the Structural Error Term.
5.4. Measures
5.4.1. Factor analysis and correlation analysis
The study evaluated the internal consistency (reliability) of the measurement items using the Cronbach’s alpha criterion (see Cohen et al., Citation2011; O’Leary-Kelly & Vokurka, Citation1998) which postulates an acceptable figure of 0.7 or more. Table confirms Cronbach alpha averaging 0.9 across the measurement items hence confirming internal consistency of the latent variables. Secondly, in order to assess discriminant validity, the Average Variance Extracted (AVE) showed that a good amount of variance in indicator variables were explained by the construct. The AVE of each of the latent constructs is higher than the AVE criterion of 0.5. The average variance extracted was first proposed by Fornell and Larcker (Citation1981). Tentatively, a minimum number of the fitness indices are below the standard, but majority are good and excellent, the reliability (0.7 or more), and the explanatory power of the factor (0.5 or more) are exceeded. In other words, convergent validity is confirmed as a measure of the factors (Figure ).
Table 1. Goodness of fit statistics
Figure 1. Conceptual model and hypotheses
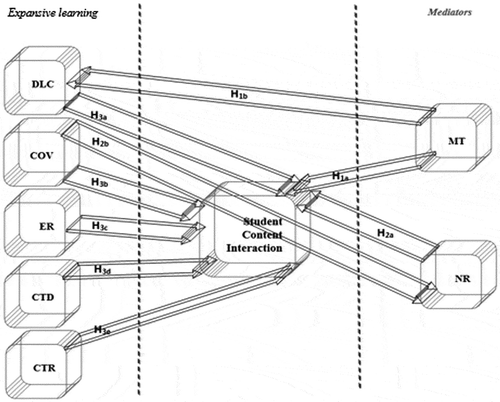
The CFA was piloted for each given construct, in order to conclude if the 40 items correctly computed the corresponding constructs. However, in estimating CFA models, maximum likelihood estimation was employed while expending criteria such as the Comparative Fit Index (CFI), Goodness-of-Fit Index (GFI), Adjusted Goodness-of-fit index (AGFI), Normal-fit index (NFI), Non-normal-fit index (NNFI) and χ2. Table provides a summary of CFI, NFI, NNFI, GFI and AGFI tests. We conclude that CFI values that are close to 1 show goodness-of-fit model indicators thus, values greater than 0.90, illustrate an acceptable fit to the data (Bentler, Citation1992; Raykov & Marcoulides, Citation2000). Conclusively, the ensuing results of the confirmatory factor analysis showed X2 = 345.000, df = 192, p = 0.000, RMR = 0.052, GFI = 0.976, AGFI = 0.055, NFI = 0.796, NNFI = 0.808 and CFI = 0.956 displays the conformance indices for specific confirmatory factor analysis results.
Table presents the results of confirmatory factor analysis which shows the various factors, path, indicators, estimates, error term, Cronbach’s Alpha and the AVEs.
Table 2. Results of confirmatory factor analysis
Following a single dimensionality of each factor using confirmatory factor analysis, we performed the correlation analysis to measure the prediction validity that determines the degree of relationship and direction of each factor. Discriminant validity (see Anderson & Gerbing, Citation1982) was assessed to determine whether each factor had differentiation. The result of the correlation analysis confirmed that the direction of the research model and the research hypothesis are in agreement with each other. In addition, it can be seen that the discriminant validity of the partial extraction index value (diagonal matrix value per factor) of each factor is greater than 0.7 (Table ).
Table 3. Correlation of factors
6. Results
This section expounds the results of the hypothesis testing, the goodness of fit for the four models tested as well as the focus group discussions of participants from three different faculties. Table shows the results of the hypotheses testing (see Section 5.1 for details of the hypotheses testing).
Table 4. Results of hypotheses testing
The results for communality and redundancy coefficients which in effect can be used as another representation of the R2 due to their similarities in explaining the amount of variance in the latent and manifest variables is presented in Table . Importantly, the model assessment is based on the analysis of fit indices, the main aim, to reveal the extent of predictive power for the appraised inner and outer model relationships. To conclusively devise a solution to this predicament, the Goodness of fit (GoF) model is introduced as a resolution for its ability to index and validate the PLS altogether. Generally, computing the GoF follows a procedure of calculating the geometric mean of the average communality and the average R2. The ensuing results in Table presents the GoF = √ [(0.725) × (0.703)] = 0.714, a satisfactory fit per Tenenhaus, Vinzi, Chatelin, and Lauro (Citation2005).
Table 5. Communality, redundancy and goodness-of-fit for models 1, 2, 3 and 4
Using Wold’s (1981) blind folding approach, we computed the CV-Communality Index (H2) for measurement of quality in the measurement models as well as CV-Redundancy Index (F2) to measure the quality of the structural model. The data presented in Table display a significant quality for both the measurement model and the structural model, i.e. H2 = 0.554 & F2 = 0.304, respectively. The best model for both qualities of measurement and quality of structural model is model 4 though all models synonymously depict communality and redundancy index.
Table 6. Blindfolding results for models 1, 2, 3 and 4
6.1. Assessing mediation effect of mediating technology and network relation characteristics with alternative models
In order to test the relationships in a causal order, a comparison between models 1, 2, 3 and 4 as displayed in Tables was done. Mediating Technology (MT) and Network Relations (NR) served as the mediator variables for the remaining six variable constructs: Digital Learning Content (DLC), Conglomeration of views (COV), Epistemic Relation (ER), Contradictions (CTR), Cognitive Transformation (CTD) and Student–Content Interaction (SCI). To test the mediation effect, the question raised is, how can the two mediator variables—Mediating Technology and Network Relation be justified as variables in the model if the latter does not assume to have any direct effect on the remaining six variable constructs.
Table 7. Parameters of the PLS model by the bootstrap method
Research model (Model 4) was adopted as the baseline for testing the direct and indirect path effect. In Model 1, Mediating Technology and Network Relation which are the two mediator variables are precluded while Digital Learning content, Conglomeration of Views, Epistemic Relation, Contradictions, Cognitive Transformation which are all Expansive Learning sub-factors are directly linked to Student–Content Interaction which is the dependent variable. Model (2) includes Digital Learning content, Conglomeration of Views, Epistemic Relation, Contradictions, Cognitive Transformation are directly linked to Student–Content Interaction via Mediating Technology but precludes Network Relation. Model 3 includes Digital Learning content, Conglomeration of Views, Epistemic Relation, Contradictions, Cognitive Transformation that are directly linked to Student–Content Interaction via Network Relations but precludes Mediating Technology. Model 4 includes all paths directly linked to Student–Content Interaction via Mediating Technology and Network Relation. This testing procedure follows Baron and Kenny’s (Citation1986) method.
Model 1 stipulates the relationships for the direct paths of Digital Learning Content, Epistemic Relation, Contradictions and Cognitive Transformation as significant due to the variables attaining t-values of 0.382, 2.981, 2.822 and 2.845, respectively, are >1.96. However, Conglomeration of views is insignificant in Model 1. In Model 2, the indirect path of Mediating Technologies exhibits an average t-value of 2.114 which is >1.96 hence statistically significant. Model 3 on the other hand, exhibits the indirect path of Network Relation with a t-value of 3.582 which is significant as it is >1.96. Conversely, Model 4 shows the most significant t-values for the full model including direct paths. The independent variable (Expansive Learning) via mediating technology and network relations to student–content interactions average a t-value of 4.855 > 1.96. The highest significant t-value attained in the path was 8.789 > 1.96 and the lowest 2.234 < 1.96. Two paths were rendered statistically insignificant in Model 4 specifically the two paths for Conglomeration of Views.
To buttress the significance of the path coefficients and t-values of the four models, further insight is deduced via the GoF in Table . The GoF’s in the Table are explained as the predictive power of each model based on the model’s R2. Model 1 illustrates an R2 of 0.517 and a GoF 0.519 for student–content interaction when the mediator variables are excluded from the model. Model 2 has an R2 of 0.572 and GoF of 0.581 for mediating technology and student–content interaction. In this case there is a mediating variable. Model three which also comprises a mediating variable in its pathing coefficient illustrates an R2 of 0.652 GoF of 0.645 for network relations and student–content interaction. Finally, Model 4 illustrates the best significant and highest PLS global quality with an R2 of 0.725 and GoF 0.714 GoF making the full stipulated research model the best fit model with full mediation effect. Respectively, there is an increment in both R2 and GoF consecutively from model 1–4 with Model four attaining the highest values in the full path model.
Table displays a medley of results for CV-Communality (H2) for all models: 1, 2, 3 and 4. The CV-Communality index (H2) explicates the measurements quality for the model whereas the CV-Redundancy index (F2) explains the structural models level of quality. This results accrued points to model 4 indicating the highest H2 at 0.554 and F2 at 0.304 between the four models. The result shows that Model 4 has both the best measurement of model quality, and a greater structural model quality when compared to the other three models. Between the two indices of model quality, F2 explains greater quality because it expounds the entire structural model, which in turn validates the effect of mediation in the model.
The result on the mediation effect shows that, overall, both mediating technology and network relations fully mediated the relationship between expansive learning (digital learning content, conglomeration of views, epistemic relation, contradictions, cognitive transformation) and student content interaction. Figure depicts the final model as well as the path coefficients, t-tests and R2.
Figure 2. Final structural model
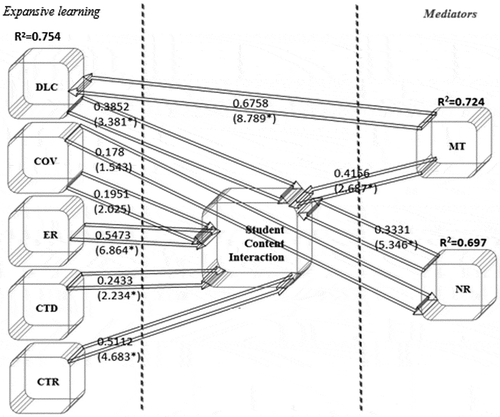
A summary of the proposed hypothesis (Figure ) shows that mediating technology have a direct and significant relationship with student–content interaction (H1a: β = 0.416, p = 0.001) and digital learning content (H1b: β = 0.676, p = 0.000). The study further revealed that network relation had a direct and significant relationship with student–content interaction (H2a: β = 0.333, p = 0.026) but an insignificant and indirect relationship with conglomeration of views (H2b: β = −0.178, p = 0.186). Student–content interaction demonstrated direct and significant relationship with digital learning content (H3a: β = 0.385, p = 0.011) and conglomeration of views (H3b: β = 0.195, p = 0.034). Additionally, student–content interaction revealed a direct and significant relationship with epistemic relation (H3c: β = 0.547, p = 0.001) and contradiction (H3d: β = 0.243, p = 0.022). Predictably, there was a significant and direct relation between cognitive transformation and student–content interaction (H3e: β = 0.511, p = 0.000).
The predictive power of the model displays an R2 = 0.725 meaning 72.5% of the variation in student–content interaction is explained by the variables of the model via the mediators of variation Mediating Technology (R2 = 0.724), 69.7% of variation in Network Relations (R2 = 0.697) and finally 75.4% of variation in Expansive learning (R2 = 0.754) for the models path coefficient.
6.2. Focus group interviews
The first discussion item required the interviewees to provide information regarding their ability to develop new ideas from the courses they study through online learning while applying them in a real-life setting; making meaning of their own through interaction and participation. This question also sought to amplify the importance of expansive learning (Engeström & Sannino, Citation2010) in digital learning environment. One of the groups noted that, the topics that require application of practical skills are mostly done at the laboratories; however, we are able to apply some of the courses that are delivered in real life environment [ENG]. Another group stated that, the courses that are delivered online are mostly theoretical and so do not require us to apply them directly on the field. They added that, courses such as C++ programming and Visual Basics require extensive face to face interactions because most of us need special assistance to understand these courses [INF]. The last group however stated that, although the online content requires us to understand and apply the theories in our context, they often do not provide detailed and practical information as we get from the face to face mode [ITB]. All the interviewees were of the opinion that the online learning mode was essential in the teaching and learning processes because it made room for students to learn on their own, at their own time and pace. The responses from the learners showed that reflective practice is enhanced in the digital learning environment through expansive learning (Engeström & Sannino, Citation2010), mediating technology (Hrastinski, Citation2008; So & Brush, Citation2008; Wu & Hiltz, Citation2004) and network relations (Fenwick & Edwards, Citation2013) that also allow students and facilitators share knowledge. Additionally, learners develop their knowledge and skills through a process of formulating and reformulating their ideas that also transforms their thinking and actions.
All the groups consented to the following as essential in promoting online learning: encouraging timely participation; encouraging clear, concise and relevant dialogue; collaborative task; group cohesion and individual reflection as well as adaptation to the e-learning environment by the students were essential factors that promote digital learning.
Regarding mediating technology, all the groups indicated that although a major challenge to the use of electronic learning platform was constant internet connectivity and adaptation to the e-learning environment by students, a well-structured training schedule could help build students’ proficiency in technology application. One of the groups indicated that students could be put in smaller groups so that students who required assistance could obtain them from colleagues.
The last item discussed was the importance of student–content interaction in the promotion of online learning. A group opined that, the role of lecturers were important in the development of course content that could support both face to face and online learning. They added that, the online content should be more detailed and have other materials such as live videos and chat sessions [ITB]. Another group opined that, the views of students should be considered in the development of the online learning content because we are the end users … it is important that the content we interact with are easy to understand and use [INF]. A group also indicated that, for the theoretical courses, student–content interaction is useful, however, since most of our courses require practical sessions, an interaction session through simulation is very important [ENG]. Pressed further, they indicated that they only took liberal courses online while most of their core courses were delivered via face to face sessions.
7. Discussions
Drawing upon the empirical evidence, the structural model provides answers to the factors that are necessary in promoting student–content interaction in a digital learning environment. The first hypothesis revealed a positive and significant relationship between mediating technology and student–content interaction. In expounding the first hypothesis, we posit that, mediating technology that describes the technological tools (Computers and Learning Management Systems) is important in promoting interaction between students and content in digital learning. Anderson (Citation2011) and Allen and Seaman (Citation2014) suggest that mediating technology promotes participation in digital learning environment. The second hypothesis demonstrated a direct and significant relationship between mediating technology and digital learning content. What the results mean is that, mediating technology is essential in developing the digital learning content and in meeting the knowledge and skills needs of students. This finding is amplified by some authors (Allen & Seaman, Citation2014; Anderson, Citation2011; Bazelais et al., Citation2018; Larbi-Siaw & Owusu-Agyeman, Citation2017; So & Brush, Citation2008) who identify elements such as; knowledge transfers among learners, discussion threads, participation, brainstorming and cooperative learning as activities that are driven by mediating technology.
In espousing H2a and H2b we show that although network relation influences student–content interaction, it has very little effect in controlling the conglomeration of views among learners in a digital learning environment. What the results mean is that network relations (Fenwick & Edwards, Citation2013) promote a community of interaction in an online learning environment through the discussion of content among students thereby, allowing students to reflect on the content and develop their knowledge and skills. Importantly, the result also suggests that while network relation promotes communication in a digital learning environment, it does not serve as a tool for gathering the views of learners. However, H3b revealed that student–content interaction is influenced by the conglomeration of views of learners in a digital learning environment.
The study revealed that student–content interaction has direct and significant relationship with digital learning content (H3b), epistemic relation (H3c), contradiction (H3d) and cognitive transformation (H3e). In explicating the effect of digital learning content on student-content interaction, we posit that the quality of digital learning content promote students’ active participation in a digital learning environment. Therefore, a well-prepared content that informs learners of the learning outcomes as well as providing them with the learning materials (Anderson, Citation2011; Davis, Citation2009; Svinicki & McKeachie, Citation2010) will help them acquire the needed knowledge and skills for their personal and professional development. The role of epistemic relation in the digital learning environment is very important primarily because it shows how the mental structures of learners’ supports the process of knowledge acquisition and application in the learning environment (Owusu-Agyeman & Larbi-Siaw, Citation2017). Importantly, through the development of mental structures, learners are able to identify changes that are required in the digital learning system, thereby proffering alternate designs that could improve the learning environment in a process referred to as contradictions. The contradictions that are primarily drawn from the views of the users of the digital learning systems (Barab, Citation2002; Isssroff & Scanlon, Citation2002) are essential in the development of a more robust learning system as well as better serving the needs of learners. Cognitive transformation (Giordano et al., Citation2002) is essential in student–content interaction because it explains how learners develop their own ideas through a process of knowledge formulation and reformulation thereby making meaning of the digital learning content.
In providing answers to RQ2, we show that student–content interaction stimulates the development of the knowledge and skills of students in a digital learning environment through an effective mix of mediating technology, expansive learning and network relations. The learner–instructor interaction and learner–learner interaction (Moore, Citation1993) provides additional interaction channels that support technology-mediated learning in HEIs. The views of the focus group participant revealed that students consider their interaction with digital learning content as very essential to their knowledge and skills development. Their opinion suggest that while other factors such as provision of mediating technology, internet connectivity and a discussion platform are important, the digital learning content enables them to obtain requisite knowledge and skills with minimal interference. The challenges identified by the participants, however, was that the digital learning system could not support learning that involved practical work thereby revealing the importance of face-to-face approach in the learning process. Face-to-face interaction with facilitators and other learners on the other hand serves to compliment the digital learning process through an effective synthesis of pedagogy, mediating technology (Donnelly, Citation2010), network relations and expansive learning.
The results also show that expansive learning is essential for developing the knowledge and skills of students in an online learning environment while supporting sturdy network relations that promote effective student–content interaction (Akyol, Citation2014; Remesal & Colomina, Citation2013). The focus group participants revealed that while the courses they took online did not require practical or laboratory activities, they needed some face-to-face interaction session to enable them to comprehend the lessons better. This opinion however, reveals the importance of some elements of expansive learning namely, conglomeration of views, contradictions, epistemic relation, and cognitive transformation (Giordano et al., Citation2002) with the latter serving as the pivot for achieving the learning goals. Additionally, as students interact with their colleagues, facilitators and content, they develop new ideas (Joo, Kim, & Kim, Citation2011; Wu & Hiltz, Citation2004), identify challenges with existing learning systems and recommend new methods of applying technology in the online learning environment.
The focus group participants also noted that while the digital learning content should provide all the learning materials needed, live videos and chat sessions (see Borup, West, & Graham, Citation2012) could further enhance their learning experience in the digital learning environment. Students identify incongruities in the content they receive and provide alternate pathways for explaining concepts and phenomenon in their environment. Through student-content interaction, collaborative tasks, effective communication channels and effective participation process (Barab, Citation2002; Eom et al., Citation2006; Isssroff & Scanlon, Citation2002; Scanlon & Issroff, Citation2005), are developed by learners who also build their own understanding of the theories they learn and apply them appropriately in their context.
8. Implications of the study
We present the implications of our study for theory, practice and further research in the next paragraphs. First, the process of teaching and learning in higher education has evolved over the years to include mediating technology that supports communication flow between students and lecturers; students and students and students and content. This study revealed that students are able to effectively interact with the content in online learning systems and further develop their own understanding of the theories and practical lessons they obtain through expansive learning. Additionally, as contradictions arise through expansive learning, students proffer alternative pathways for strengthening the content that are developed by facilitators through cognitive transformation.
Practically, while the mediating technology serve as the conduit for transferring information among students in a community, expansive learning through its five sub-factors; epistemic relation, contradictions, digital learning content, conglomeration of views and cognitive transformation also promote enhance effective learning. Network relations are also important because through active interaction and exchange of views new ideas and knowledge emerge thereby promoting cognitive transformation among students. Although students focus on engaging the content in a digital learning platform with the aim of obtaining further knowledge and skills, education providers should focus on ensuring that expansive learning, mediating technology and network relations are enhanced in an online learning to provide students with quality learning. Zimmerman (Citation2012) posits that an effectual student–content interaction process is also essential because it could lead to successful learning outcomes and course completion among learners. What this means is that if the right content and appropriate technology are developed, students will actively participate in online learning activities which will lead to the development of their knowledge and skills. We recommend further studies into alternative ways of stimulating students’ interest in engaging with the content of an online course.
Source of the image
Co-author - Mr. Otu Larbi-Siaw.
Funding
The authors received no direct funding for this research.
Data availability statement
The data that support the findings of this study shall be available on request from the corresponding author [Yaw Owusu-Agyeman – [email protected]]. The data are not publicly displayed due to restrictions based on ethical reasons.
Additional information
Notes on contributors
Yaw Owusu-Agyeman
Yaw Owusu-Agyeman is a fellow of the Higher Education Academy (FHEA), UK, the Head of Examinations and Records Unit, Ghana Technology University College. He holds a PhD in Curriculum Studies from Stellenbosch University and his dissertation focused on increasing resource and knowledge support for adult learners in the telecommunications and energy sectors of Ghana. Yaw is also a research associate at the Centre for Higher and Adult Education, Stellenbosch University.
Otu Larbi-Siaw
Otu Larbi-Siaw is an economist with a passion for designing systematic decision-making systems using models. He is the head of Department for Economics at Ghana Technology University College. His research interest is in the area of macroeconomics, innovation economics, game theory, artificial intelligence, digital analytics and finance. Otu is also a consultant for several companies in Ghana and he offers business solutions in diverse areas including the optimisation of business decision-making processes through the application of technology.
References
- Akyol, Z. (2014). The development of a community of inquiry over time in an online course: Understanding the progression and integration of social, cognitive, and teaching presence. Journal of Asynchronous Learning Networks, 12(3–4), 3–22.
- Alammary, A., Sheard, J., & Carbone, A. (2014). Blended learning in higher education: Three different design approaches. Australasian Journal of Educational Technology, 30(4).
- Allen, I. E., & Seaman, J. (2014). Grade change: Tracking online education in the United States. Babson Survey Research Group. Retrieved January 23, 2016, from http://www.onlinelearningsurvey.com/reports/gradechange.pdf
- Anderson, T. (2011). The theory and practice of online learning. Edmonton: AU Press.
- Anderson, J. C., & Gerbing, D. W. (1982). Some methods for respecifying measurement models to obtain unidimensional construct measurement. Journal of Marketing Research, 19(4), 453–460.10.2307/3151719
- Anderson, J. C., & Gerbing, D. W. (1988). Structural equation modeling in practice: A review and recommended two-step approach. Psychological Bulletin, 103(3), 411.
- Barab, S. A. (2002). Commentary: Human-field interaction as mediated by mobile computers. In T. Koschmann, R. Hall, & N. Miyake (Eds.), Computer supported collaborative learning (pp. 533–538). Mahwah, NJ: Erlbaum.
- Barab, S. A., Evans, M. A., & Baek, E. (2004). Activity theory as a lens for characterizing the participatory unit. In D. H. Jonassen (Ed.), Handbook of research for educational communications and technology (pp. 199–213). Mahwah, NJ: Lawrence Erlbaum.
- Barkely, E. (2009). Student engagement techniques: A handbook for college faculty. New York, NY: Jossey Bass.
- Baron, R. M., & Kenny, D. A. (1986). The moderator–mediator variable distinction in social psychological research: Conceptual, strategic, and statistical considerations. Journal of Personality and Social Psychology, 51(6), 1173.10.1037/0022-3514.51.6.1173
- Bazelais, P., Doleck, T., & Lemay, D. J. (2018). Investigating the predictive power of TAM: A case study of CEGEP students’ intentions to use online learning technologies. Education and Information Technologies, 23(1), 93–111.10.1007/s10639-017-9587-0
- Bentler, P. M. (1992). On the fit of models to covariance and methodology to the bulletin. Psychological Bulletin, 112(3), 400–404.10.1037/0033-2909.112.3.400
- Bernstein, B. (1999). Vertical and horizontal discourse: An essay. British Journal of Sociology of Education, 20(2), 157–173.10.1080/01425699995380
- Borup, J., West, R., & Graham, C. (2012). Improving online social presence through asynchronous video. Internet and Higher Education, 15(3), 195–203.10.1016/j.iheduc.2011.11.001
- Cohen, L., Manion, L., & Morrison, K. (2011). Research methods in education (7th ed.). New York, NY: Routledge.
- Davis, B. G. (2009). Tools for teaching (2nd ed.). San Francisco: Jossey-Bass.
- Donnelly, R. (2010). Harmonizing technology with interaction in blended problem-based learning. Computers & Education, 54, 350–359.10.1016/j.compedu.2009.08.012
- Engeström, Y. (2000). Activity theory as a framework for analyzing and redesigning work. Ergonomics, 43(7), 960–974.10.1080/001401300409143
- Engeström, Y. (2001). Expansive learning at work: Toward an activity theoretical reconceptualisation. Journal of Education and Work, 14(1), 133–156.10.1080/13639080020028747
- Engeström, Y., & Sannino, A. (2010). Studies of expansive learning: Foundations, findings and future challenges. Educational Research Review, 5(1), 1–24
- Eom, S. B., Wen, H. J., & Ashill, N. (2006). The determinants of students’ perceived learning outcomes and satisfaction in university online education: An empirical investigation. Decision Sciences Journal of Innovative Education, 4(2), 215–235.10.1111/dsji.2006.4.issue-2
- Felstead, A., Fuller, A., Unwin, L., Ashton, D., Butler, P., & Lee, T. (2005). Surveying the scene: Learning metaphors, survey design and the workplace context. Journal of Education and Work, 18(4), 359–383.10.1080/13639080500327857
- Fenwick, T., & Edwards, R. (2013). Performative ontologies: Sociomaterial approaches to researching adult education and lifelong learning. European Journal for Research on the Education and Learning of Adults, 4(1), 49–63.10.3384/rela.2000-7426
- Fornell, C., & Larcker, D. (1981). Structural equation models with unobservable variables and measurement error. Journal of Marketing Research, 18(1), 39–50.10.2307/3151312
- Giordano, P. C., Cernkovich, S. A., & Rudolph, J. L. (2002). Gender, crime, and desistance: Toward a theory of cognitive transformation. American Journal of Sociology, 107(4), 990–1064.10.1086/343191
- Graham, C. R. (2013). Emerging practice and research in blended learning. In M. J. Moore (Ed.), Handbook of distance education (3rd ed., pp. 333–350). New York, NY: Routledge.
- Hair, J. F., Anderson, R. E., Tatham, R. L., & Black, W. C. (2010). Multivariate data analysis: A global perspective (7th ed.). Upper Saddle River, NJ: Pearson Prentice-Hall.
- Hasu, M., & Engeström, Y. (2000). Measurement in action: An activity-theoretical perspective on producer–user interaction. International Journal of Human-Computer Studies, 53(1), 61–89.10.1006/ijhc.2000.0375
- Hrastinski, S. (2008). Asynchronous and synchronous e-learning. Educause quarterly, 31(4), 51–55.
- Hrastinski, S. (2009). A theory of online learning as online participation. Computers and Education, 52(1), 78–82.10.1016/j.compedu.2008.06.009
- Isssroff, K., & Scanlon, E. (2002). Using technology in higher education: An activity theory perspective. Journal of Computer Assisted Learning, 18(1), 77–83.10.1046/j.0266-4909.2001.00213.x
- Joo, Y. J., Kim, K. Y., & Kim, E. K. (2011). Online university students’ satisfaction and persistence: Examining perceived level of presences, usefulness, and ease of use as predictors in a structural model. Computers & Education, 57(2), 1654–1664.10.1016/j.compedu.2011.02.008
- Larbi-Siaw, O., & Owusu-Agyeman, Y. (2017). Miscellany of students’ satisfaction in an asynchronous learning environment. Journal of Educational Technology Systems, 45(4), 456–475. doi/10.1177/0047239516667499
- Lindlof, T. R. (1995). Qualitative communication research methods. Thousand Oaks: Sage.
- Maton, K. (2004). The wrong kind of knower: Education, expansion and the epistemic device. In J. Muller, B. Davies, & A. Morais (Eds.), Reading Bernstein, researching Bernstein (pp. 218–231). London/NewYork: Routlegde.
- Moore, M. G. (1993). Theory of transactional distance. In D. Keegan (Ed.), Theoretical principles of distance education (pp. 22–29). New York, NY: Routledge.
- O’Leary-Kelly, S. W., & Vokurka, R. J. (1998). The empirical assessment of construct validity. Journal of Operations Management, 16(4), 387–405.10.1016/S0272-6963(98)00020-5
- Owusu-Agyeman, Y., & Larbi-Siaw, O. (2017). Reframing the principle of specialization in legitimation code theory: A blended learning perspective. Education and Information Technologies, 22(5), 2583–2603. doi/10.1007/s10639-016-9563-0 .
- Owusu-Agyeman, Y., Larbi-Siaw, O., Brenya, B., & Anyidoho, A. (2017). An embedded fuzzy analytic hierarchy process for evaluating lecturers’ conceptions of teaching and learning. Studies in Educational Evaluation, 55, 46–57.10.1016/j.stueduc.2017.07.001
- Patton, M. Q. (2002). Qualitative research and evaluation methods. Thousand Oaks, CA: Sage.
- Porter, W. W., & Graham, C. R. (2016). Institutional drivers and barriers to faculty adoption of blended learning in higher education. British Journal of Educational Technology, 47(4), 748–762.10.1111/bjet.2016.47.issue-4
- Price, M., Handley, K., & Millar, J. (2011). Feedback: Focusing attention on engagement. Studies in Higher Education, 36(8), 879–896.10.1080/03075079.2010.483513
- Raykov, T., & Marcoulides, G. A. (2000). A first course in structural equation modelling. Mahwah, NJ: Lawrence Erlbaum Associates.
- Remesal, A., & Colomina, R. (2013). Social presence and online collaborative small group work: A socioconstructivist account. Computers & Education, 60(1), 357–367.10.1016/j.compedu.2012.07.009
- Scanlon, E., & Issroff, K. (2005). Activity theory and higher education: Evaluating learning technologies. Journal of Computer Assisted Learning, 21(6), 430–439.10.1111/j.1365-2729.2005.00153.x
- So, H. J., & Brush, T. A. (2008). Student perceptions of collaborative learning, social presence and satisfaction in a blended learning environment: Relationships and critical factors. Computers & Education, 51(1), 318–336.10.1016/j.compedu.2007.05.009
- Stetsenko, A. P. (1999). Social interaction, cultural tools and the zone of proximal development: In search of a synthesis. In S. Chaiklin, M. Hedegaard, & U. J. Jensen (Eds.), Activity theory and social practice: Cultural-historical approach (pp. 235–253). Aarhus, DK: Aarhus University Press.
- Svinicki, M., & McKeachie, W. J. (2010). McKeachie’s teaching tips: Strategies, research, and theory for college and university teachers. Belmont, CA: Wadsworth.
- Tay, H. Y. (2016). Investigating engagement in a blended learning course. Cogent Education, 3(1), 1135772.
- Teddlie, C., & Tashakkori, A. (2009). Foundations of mixed methods research: Integrating quantitative and qualitative in the social and behavioural sciences. Thousand Oaks: Sage.
- Tenenhaus, M., Vinzi, V. E., Chatelin, Y. M., & Lauro, C. (2005). PLS path modelling. Computational Statistics & Data Analysis, 48(1), 159–205.10.1016/j.csda.2004.03.005
- Woo, Y., & Reeves, T. C. (2007). Meaningful interaction in web-based learning: A social constructivist interpretation. The Internet and Higher Education, 10(1), 15–25.10.1016/j.iheduc.2006.10.005
- Wu, D., & Hiltz, S. R. (2004). Predicting learning from asynchronous online discussions. Journal of Asynchronous Learning Networks, 8(2), 139–152.
- Zimmerman, T. D. (2012). Exploring learner to content interaction as a success factor in online courses. The International Review of Research in Open and Distributed Learning, 13(4), 152–165.10.19173/irrodl.v13i4.1302