Abstract
The end of the COVID-19 pandemic that directly impacts students’ learning cannot be predicted with certainty. Previously dominated by face-to-face learning methods, student learning has fully transitioned into full e-learning, or online/distance learning provides a completely new experience for students. Students are important learning recipients and university stakeholders. Therefore, much attention should be paid to their learning satisfaction to ensure that higher education’s learning process is conducted well during a pandemic. The absence of quantitative empirical research on the drivers of e-learning satisfaction in the setting of private higher education is the theoretical impetus for this study. This study evaluated a learning satisfaction model during (early) the COVID-19 pandemic. An online questionnaire survey with a sample of 722 undergraduate students from a top-ranking private university was conducted in Indonesia, which reported the highest number of COVID-19 cases in Southeast Asia in 2020. Survey results identify the social presence, confirmation, and student-student interaction as the drivers of e-learning satisfaction during the pandemic. Moreover, robust learning system quality has a significant indirect influence on learning satisfaction that is mediated by student-student interaction. The findings of this study can provide implications for private university administrators in Indonesia to pay attention to and make improvements related to social presence, confirmation, learning system quality, and student-student interaction during a pandemic.
Public interest statement
During the COVID-19 pandemic, students learned entirely online, which was a very new experience for them. Given the importance of students as stakeholders in higher education institutions, their e-learning satisfaction, especially during the COVID-19 pandemic, warrants exploration. A survey with a sample of 722 undergraduate students from a top-ranking private university was conducted in Indonesia. Indonesia reported the highest number of COVID-19 cases in Southeast Asia in 2020. Private universities in Indonesia are recommended to prioritize confirmation to enhance the learning satisfaction of their students. Private universities in Indonesia should also focus on the constructs of social presence, confirmation, and student-student interaction. They should design an e-learning system that stimulates students and encourages their information and knowledge sharing. The quality of the learning system also comes out as an important factor that helps students interact with the e-learning system.
1. Introduction
The COVID-19 epidemic, regarded as one of the most devastating disasters in recent history, has touched many facets of society, particularly education (Irawan et al., Citation2020). In March 2020, all universities were required to deliver their classes online (Yarrow & Bhardwaj, Citation2020). However, the education system was largely unprepared for this sudden transition (Pangarso, Citation2016). The readiness of students to learn online affects their learning satisfaction (Pillay et al., Citation2007). Given the importance of students as stakeholders of higher education institutions, their e-learning satisfaction, especially during the COVID-19 pandemic, warrants exploration (Herdlein & Zurner, Citation2015).
Kucuk and Richardson (Citation2019) and Ooi et al. (Citation2018) argued that social presence, agency engagement and social presence with emotional engagement have insignificant effects on student satisfaction. Moreover, Bervell et al. (Citation2019) examined the direct effect of personal innovativeness on e-learning satisfaction and the mediating role of student–student interaction in the effect of personal innovativeness on e-learning satisfaction. Other scholars show that confirmation (Zareravasan & Ashrafi, Citation2019); (Venter & Swart, Citation2018); system quality (Chen, Citation2020); (Venter & Swart, Citation2018)), and LMS quality (Theresiawati et al., Citation2020) have significant influences on satisfaction. Moreover, Al-Rahmi et al. (Citation2018) pointed towards the need to examine the effect of engagement and interaction. According to Safsouf et al. (Citation2020), service quality has no significant effect on learner satisfaction, whereas Kuo et al. (Citation2013) found no significant relationship between student–student interaction and student satisfaction. However, Dwidienawati et al. (Citation2020); Arifiati et al. (Citation2020) and Murad et al. (Citation2020) pointed out two key gaps in the literature, namely, the unclear research methodology and the limited quantitative research that tests a research framework for the antecedents of e-learning satisfaction. To fill these gaps, we examine the e-learning satisfaction of students at the beginning of the COVID-19 pandemic. We specifically aim to answer the research question, what are the drivers of the e-learning satisfaction of university students at the beginning of the COVID-19 pandemic?
This research is significant since it is uncertain when the COVID-19 epidemic will cease, therefore e-learning is still a significant learning medium for the time being. The COVID-19 pandemic necessitated knowledge, testing, and analysis of factors influencing e-learning satisfaction in the context of private higher education in Indonesia. Research needs to be done quickly so that continuous improvement can be carried out related to matters that significantly affect, both directly and indirectly.
2. Literature review
By using the keywords “e-learning” OR “online learning” OR “distance learning” AND “satisfaction” AND “SEM” OR “PLS” on the Scopus database, we retrieved 124 related studies dating back to early 2021. As shown in Figure , these studies followed the Prisma (Moher et al., Citation2009). All these studies had no duplicates and were filtered using English-language published from 2016 to 2021. After screening these documents, we retained 68 articles in our sample. We further screened these articles and retained only those that focused on the higher education/university context, hence leaving us with 36 articles for the analysis.
We summarise below the seven previous studies that provide the basis for our development of a conceptual model/research framework in Figure .
The information system success model (ISM) provides the main theoretical framework for exploring the antecedents of learning satisfaction (DeLone & McLean, Citation1992). On the basis of previous studies, we establish a link between those constructs that make up the ISM and those other constructs from six other theories (Table ). On the basis of this relationship, we create a highly comprehensive research framework for our empirical testing. This research framework combines the nine antecedents of e-learning satisfaction with seven underlying theories. To the best of our knowledge, this framework has never been published and tested quantitatively and empirically in the past.
Table 1. Research comparison
The Community of Inquiry (CoI) framework (. R. Garrison et al., Citation2010; Randy, Citation2010; Garrison & Arbaugh, Citation2007; Garrison & Akyol, Citation2016) posits that teaching presence, social presence and cognitive presence create an effective and high-quality learning process (which involves satisfaction and engagement). We only examine the social presence construct following the arguments of Kucuk and Richardson (Citation2019) and Ooi et al. (Citation2018) who stated that social presence does not have a significant effect on emotional engagement and satisfaction, hence leaving us an interesting research gap worth exploring.
Besides affecting learning satisfaction, social presence also affects engagement. We define the concept of engagement in learning following Fredricks et al. (Citation2004). Specifically, engagement refers to the active involvement of learners in their learning process. Emotional engagement is an enthusiastic attitude manifested in the positive emotional expressions of learners and their willingness to follow the rules of their lecturers. E-learning during the COVID-19 pandemic requires agency and emotional engagement to maintain learning enthusiasm. In e-learning, emotional expression, openness in communication and closeness/cohesiveness in a group affect learning enthusiasm. Lee et al. (Citation2020), Lim and Richardson (Citation2021), Park and Kim (Citation2020) demonstrated that social presence significantly affects learning satisfaction. From these arguments, we propose.
H1: Social presence significantly affects agency engagement.
H2: Social presence significantly affects learning satisfaction.
H3: Social presence significantly affects emotional engagement.
In ISM (DeLone & McLean, Citation1992), six factors determine the success of an information system (IS): success quality, system quality, information utilization, user happiness, individual impact, and organizational effect. In this study, we define the antecedents of such success as system quality, service, lecturer or instructor quality and user (student) satisfaction. We do not discuss the impact/success of an IS on an individual or organisation but rather focus on the satisfaction of users with this system.
Lecturer/service quality affects emotional engagement. Emotional engagement is a positive attitude that is manifested in one’s enthusiasm in online learning (Kucuk & Richardson, Citation2019). At the start of the COVID-19 epidemic, learning management system (LMS) users had to demonstrate excitement (positive attitude). Meanwhile, the quality service of lecturers affects the enthusiasm of students or the recipients of learning materials.
H4: Lecturer quality significantly affects emotional engagement.
The updated ISM (DeLone & McLean, Citation2003) has an additional element, namely, the service/lecturer quality construct. This model is similar to Servqual theory of e-learning (Parasuraman et al., Citation1988; Uppal et al., Citation2018), which states that quality online learning is influenced by assurance, empathy, responsiveness, reliability, tangibility, learning content and learning quality. In the context of online learning, especially in higher education, the primary interaction between the student and lecturer (teacher) enables the transmission of knowledge. Given that the learning materials are delivered by lecturers, the quality of learning services corresponds to the quality of these lecturers. Quality lecturers are qualified servants and hence affect the satisfaction of students in online learning (Al-Azawei, Citation2019; Al-Maroof & Salloum, Citation2021; Daghan & Akkoyunlu, Citation2016; Efiloğlu Kurt, Citation2018; Hadoussa, Citation2020; Isaac et al., Citation2019; Pérez-Pérez et al., Citation2020; Safsouf et al., Citation2020; Zhao et al., Citation2020). Teaching quality, which includes the quality of lecturers (which is a course-related factor), can therefore predict the satisfaction of students in online learning (Denson et al., Citation2010).
H5: Lecturer quality significantly affects learning satisfaction.
A high-quality learning system provides students and instructors with a learning tool or location. This system’s student interactions are explained by the SCT/social constructivism paradigm for online learning (Anderson, Citation2004) and MOI//Models of online interaction theory both of which identify student, content and lecturer/instructor as the main elements in online learning. According to Bervell et al. (Citation2019), Najmul Islam (Citation2011), Daghan and Akkoyunlu (Citation2016), Isaac et al. (Citation2019), Zhao et al. (Citation2020), Hadoussa (Citation2020) and Theresiawati et al. (Citation2020), learning system quality also has a significant effect on the satisfaction of students in online learning. The following hypotheses are then proposed based on these arguments.
H6: Learning system quality significantly affects student–student interaction.
H7: Learning system quality significantly affects learning satisfaction.
H8: Learning system quality significantly affects lecturer-student interaction.
Agarwal and Prasad (Citation1998) stated that personal innovativeness refers to the willingness of individuals to learn new things related to online learning. Al-Busaidi and Al-Shihi (Citation2012) found that personal innovativeness has a significant effect on instructor satisfaction. Furthermore, Bervell et al. (Citation2019) stated that personal innovativeness has a significant effect on LMS satisfaction as mediated by student–material, student–student and teacher–student interactions. Personal innovation also affects emotional engagement. The encouragement to learn new things during the COVID-19 pandemic through online learning has resulted in enthusiasm for learning online. On the basis of these arguments, we propose:
H9: Personal innovation significantly affects learning satisfaction.
H10: Personal innovation significantly affects emotional engagement.
Expectation–confirmation theory (ECT) (Bhattacherjee, Citation2001; Oliver, Citation1980) states that in the online learning context, a positive confirmation (belief) from learners about their learning expectations leads to their satisfaction in learning online. Moreover, Najmul Islam (Citation2011), Daghan and Akkoyunlu (Citation2016), Zareravasan and Ashrafi (Citation2019), Cheng (Citation2019), Daneji et al. (Citation2019), Cheng (Citation2020), Dai et al. (Citation2020) and Al-Maroof & Salloum, Citation2021) found that confirmation has a significant effect on learning satisfaction. Therefore, we propose:
H11: Confirmation significantly affects learning satisfaction.
Hwee et al. (Citation2020) found that intense online learning through LMS promotes interaction and engagement. Poort et al. (Citation2022) argued that student engagement is an important factor related to collaborative learning that affects learning satisfaction. Kucuk and Richardson (Citation2019) explored the significant effect of engagement on learning satisfaction. Meanwhile, Al-Rahmi et al. (Citation2018) stated that future research should further explore the effect of engagement related to student satisfaction. The CoI framework also confirms the influence of engagement on learning satisfaction. If learners can have good agency engagement in adherence to regulations in the form of positive emotional expressions, then this engagement affects their learning satisfaction. We therefore propose:
H12: Agency engagement significantly affects learning satisfaction.
H13: Emotional engagement significantly affects learning satisfaction.
Interactions and perceptions about the learning context (related to the quality of lectures) are important aspects related to online learning at universities (Ellis et al., Citation2009) (Cheng , Citation2020). Previous research conducted by Sher (Citation2009), Parahoo et al. (Citation2016), Kuo et al. (Citation2013), Bervell et al. (Citation2019), Cheng (Citation2020) and Tadesse et al. (Citation2020) confirmed the significant effect of interaction on learning satisfaction. Meanwhile, Al-Rahmi et al. (Citation2018) stated that future research should further explore the effect of interactivity related to student satisfaction. We therefore propose:
H14: Student–student interaction significantly affects learning satisfaction.
H15: Lecturer–student interaction significantly affects learning satisfaction.
3. Methods
We adopted a quantitative survey for the data collection (Hulland et al., Citation2018; Johnson & Joanne, Citation2000). We chose the 26,182 students of a top-ranking private university in Indonesia (Indonesia Ministry of Research, 2020). As our research population for several reasons. Firstly, higher education in Indonesia is still dominated by private universities, which play critical roles in achieving the “Golden Indonesia” goal of the country by 2045 (Pangarso, Citation2019; Pangarso et al., Citation2020; Park & Kim, Citation2020). (2) Secondly, the top private university in Indonesia has excellent institutional governance. Thirdly, the research sample included students coming from all major islands of Indonesia.
We only recruited those students who underwent e-learning at the beginning of the COVID-19 pandemic. Sampling using G power requires a minimum of 109 respondents (Memon et al., Citation2020). Non-probability sampling was performed using convenience sampling (Bornstein et al., Citation2013) by prospective respondents voluntarily. Convenience sampling is appropriate for this study because the initial momentum of the early COVID-19 pandemic requires a rapid response rate in collecting data. The research timeframe was cross sectional (during May 2020; even semester of the 2019/2020 school year specifically for new students (Masyarakat & Kebudayaan, Citation2020)).
We distributed the questionnaires as Google Forms with the help of an LMS administrator as an enumerator on May 2020 (2019/2020 academic year). These questionnaires were distributed in two waves (with a one-week interval). To ensure that each respondent would only fill one form, they were asked to indicate the email address of their institution. All respondents gave their written consent to participate. All items in the questionnaire were proven to be statistically valid and reliable and were rated on a Likert-type scale ranging from 1 (very disagree) to 5 (very agree). There were pilot tests on 40 student representatives of each faculty to check the questionnaire statement items’ validity (all questionnaire items show Pearson correlation/r value = 0,320 > r table value) and reliability (Cronbach’s Alpha > 0,70) using SPSS. Research variable operationalization for research variables consists of:
Social presence, adopted and modified from Arbaugh (Citation2008).
Lecturer quality, adopted and modified from Cheng (Citation2014).
Learning system quality, adopted and modified from Cheng (Citation2014).
Personal innovation, adopted and modified from van Raaij and Schepers (Citation2008).
Confirmation consists, adopted and modified from Bhattacherjee, Citation2001).
Agency engagement, adopted and modified from Fredricks et al. (Citation2004).
Emotional engagement, adopted and modified from Reeve (Citation2013).
Student-student interaction was adopted and modified from Sher (Citation2009).
Lecturer-student interaction, adopted and modified from Sher (Citation2009).
Learning satisfaction, adopted and modified from Kuo et al. (Citation2013).
A total of 722 questionnaires were received, yielding a response rate that exceeded 100%. All these questionnaires were filled correctly and were free from outliers, hence making them usable for further data processing. We effectively minimised non-response bias in the survey by following the recommendations of Fuchs et al. (Citation2013) to recruit an LMS administrator as an enumerator, who was in charge of directly contacting the respondents and reminding them to fill out the questionnaire. The use of internal research enumerators simplifies and speed up data collecting. LMS is officially a campus infrastructure that provides trust. The questionnaire also clearly explained to the respondents the importance of the research, which encouraged them to contribute their responses.
Following Kock (Citation2015); Podsakoff et al. (Citation2012); Tehseen et al, (Citation2017), we applied procedural and statistical remedies to minimise common method variance (CMV). Firstly, we mentioned in the questionnaire that the items were not related to one another. Secondly, we mentioned that all data shared by the respondents would be kept confidential. Thirdly, we used simple and easy to understand sentences in our questions. Fourthly, specific statistics for data processing through an AFVIF (full collinearity) value smaller than 3.3 (Kock, Citation2015; Podsakoff et al., Citation2012). The AFVIF values presented on the diagonal of Table show that all research variables are free of CMV.
Table 2. AFVIF
The first part of the questionnaire asked for the demographic data of the respondents showed that: (1) the sex was balanced between men and women; (2) age was dominated by respondents aged 17–22 years; (3) study from home (SFM) students based on their area of origin are dominated by those who live on the island of Java and Bali; (4) student science is dominated by social science; (5) students are predominantly studying in semester 2; (6) Zoom and Google Meet dominate four of the platforms used for online learning; (7) laptops and smartphones are the most commonly used tools for online learning; (8) the average length of time for online compulsory learning in one day is less than 2 hours and more than 8 hours; and (9) the average length of time for independent online learning in one day is less than 2 hours to less than 5 hours.
4. Results
For the theoretical framework hypothesis testing, we used the SEM variant type, namely, partial least square (PLS) (Chin, Citation1998; Wold, Citation1995). We performed structural equation modelling via Smart PLS 3.2.9 to test our hypotheses (Ringle et al., Citation2015). We selected this software due to its functions for processing complex structural models with a large number of indicators (Chin & Newsted, Citation1999; Fornell & Bookstein, Citation1982). PLS-SEM (using Smart PLS software) can also process data that are not normally distributed (Fornell & Bookstein, Citation1982; Gefen et al., Citation2000) and has been widely used in studies that are published in top journals (Benitez et al., Citation2020; Hair et al., Citation2012; Richter et al., Citation2016; Rigdon et al., Citation2017).
Data processing via Smart PLS involves two inspection elements, namely, measurement (outer) model check and structural (inner) model check, along with a robustness check (Hair et al., Citation2019). Before examining the measurement and structural model, we tested the overall fit of our model. We obtained an SRMR value of 0.055 (< 0.080) and RMS theta value of 0.112 (< 0.12), both of which validate the good fit of our model (.Hair et al., Citation2017).
All our indicators have a unidimensional reflective form. To evaluate our measurement model, we used reflective indicators, including indicator reliability (loading factor, cut-off > 0.708), construct reliability (rho-A, cut-off > 0.800), convergent validity (AVE, cut-off > 0.500) and discriminant validity (HTMT, cut-off < 0.90) (Gudergan et al., Citation2008; Kwong & Wong, Citation2019; Zulherman et al., Citation2021). All loading factors in Table met the cut-off with a value of > 0.708 (or > 0.70).
Table 3. Descriptive statistics, measurement model, R2 and Q2 value
All our variables for construct reliability, convergent validity and discriminant validity (Table ) met the cut-off with values of rho-A > 0.80, AVE > 0.50 and HTMT < 0.90, respectively.
Table 4. Discriminant validity
We then performed a robustness check using CTA PLS, and the results show that our measurement model is reflective in shape.
We assessed the structural model assessment by testing the collinearity (inner VIF, cut-off < 5), explanatory power (R2, cut-off > 0 and Q2, cut-off > 0) and relevance of the path coefficient (p-value < 0.05) in addition to conducting a robustness check (Sarstedt et al., Citation2019). Table shows that the inner VIF values for all variables are less than 5, thereby suggesting the absence of collinearity.
Table 5. Inner VIF value
Table shows that LS has the highest R2 value of 0.739, which means that 73.9% of LS variables have a strong explanatory power. LS also has the highest Q2 value of 0.568, which means that 56.8% of the structural model with endogenous LS variables have high predictive relevance (accuracy).
Table shows that H1–H4, H6–H9, H11, H14 and H15 are all accepted with p-values of 0.000 and 0.033 for H14 (all other p-values are less than 0.05). The f square value is above 0.35, which means that the independent variable has a relatively large impact on the dependent variable. Meanwhile, H5, H10, H12 and H13 are all rejected with a p-value of > 0.05 and an f square value of < 0.02.
Table 6. Hypotheses testing
We tested for the non-linear effects using Smart PLS and R (R: The R Project for Statistical Computing, n.d.). We employed the Gaussian Copulas approach in R to examine endogeneity following the procedures prescribed in Huit et al. (Citation2018). Meanwhile, we adopted the FIMIX method to test for unobserved heterogeneity (Hair, Jr. et al., Citation2016; Matthews et al., Citation2016).
Table shows a significant pathway from LSQ to LS through SSI and LSI, which have p-values of 0.035 and 0.001, respectively (< 0.05) but the path that shows significant and robust is only on the LSQ to LS through SSI path.. Meanwhile, we observe the indirect effect that insignificant: (1) all channels of SP on LS through AA and EE, which have p-values of 0.991 and 0.611 (> 0.05), (2) the LQ path on LS through EE with a p-value of 0.606 (> 0.05) and (3) the PI path to LS through EE with a p-value of 0.600 (> 0.05).
5. Discussion
Table shows the results of the influence between constructs/variables, both directly (hypothesis testing) and indirectly (mediation) for the analysis of significance and robustness (non-linearity and endogeneity). Table shows that the rightmost column shows significance and robustness, where the word “yes” means a significant and robust influence between variables. This large and robust relationship across factors is studied further in regard to the determinants of student learning satisfaction at the onset of the COVID-19 epidemic in the context of private universities in Indonesia. There are eight hypotheses of the influence between significant variables and robust in Table , namely: (1) H1, the influence of social presence on agency engagement; (2) H2, the influence of social presence on learning satisfaction; (3) H3, the influence of social presence on emotional engagement; (5) H7, the influence of learning system quality on student interaction; (6) H9, personal innovation on emotional engagement; (7) H11, confirmation on learning satisfaction; and (8) H14, student-student interaction on learning satisfaction. Of these hypotheses, H2, H11, and H14 are the most significant and robust hypotheses related to learning satisfaction. Therefore, social presence, confirmation, and student-to-student connection at the onset of the COVID-19 epidemic in the setting of private colleges in Indonesia are the drivers of learning satisfaction. Next, Table demonstrates the robust and significant indirect influence of drivers on learning satisfaction at the onset of the COVID-19 epidemic in the setting of private universities in Indonesia. Learning system quality, as mediated by student-student interaction, was a significant and robust indirect path of influencing learning satisfaction at the start of the COVID-19 pandemic in private universities in Indonesia (Table ).
The positive significant influence of SP on LS supports the CoI framework, thereby filling the research gap of and supporting the findings of Kucuk and Richardson (Citation2019); Ooi et al. (Citation2018) and Lee et al. (Citation2020); Lim and Richardson (Citation2021); Park and Kim (Citation2020). At the onset of the COVID-19 pandemic, our data show demonstrate that a superior SP can boost the e-learning satisfaction of private university students. Social presence is the student’s ability to show personal characteristics in the online learning community, so social presence is important in interaction and communication (Garrison, Citation203). At the start of the COVID-19 pandemic, this study found that having a better social presence results in higher satisfaction among private university students in Indonesia when learning online. Specifically, these students feel accepted, comfortable and valued and even form a solidarity bond with their lecturers through e-learning. Lecturers have been perceived by students to have formed solidarity in online learning.
The significant positive effect of C on LS supports the contentions of ECT, thereby filling the research gap mentioned in Venter and Swart (Citation2018) and supporting the findings of Al-Maroof & Salloum, Citation2021), Cheng (Citation2019, Citation2020), Daghan and Akkoyunlu (Citation2016), Daneji et al. (Citation2019), Najmul Islam (Citation2011), Zareravasan and Ashrafi (Citation2019). This study confirms that the better the confirmation, the more satisfied private university students in Indonesia are with studying online at the start of the COVID-19 pandemic. Specifically, a higher grade equates to greater e-learning satisfaction among private university students at the onset of the COVID-19 epidemic. The confirmation is related to the student’s perception of fulfillment, even exceeding expectations, and the need for online learning. This student confirmation proves that online learning during the pandemic of private universities in Indonesia succeeded in satisfying students. Confirmation has the greatest effect (indicated by the value of f2) compared to social presence and student–student interaction, so confirmation is the most important variable that affects learning satisfaction.
The significant positive effect of SSI on LS supports the contentions of SCT and MOI, hence filling the research gaps mentioned in Bervell et al. (Citation2019) and support the findings of Al-Rahmi et al. (Citation2018), Bervell et al. (Citation2019), Kuo et al. (Citation2013), Parahoo et al. (Citation2016), Sher (Citation2009). Specifically, a better interaction amongst students increases their satisfaction in e-learning at the start of the COVID-19 pandemic. Interactions between students are related to their perceptions of cohesiveness (the feeling of the community), learning in groups, and sharing experiences (information and knowledge). The interaction between students shows that private universities in Indonesia have been able to help students learn online during a pandemic.
Meanwhile, the mediating role of SSI in the effect of LSQ on LS supports the contentions of ISM, SCT and MOI. Specifically, a high-quality LSQ improves the interaction among students; filling the research gap Kuo et al. (Citation2013). The finding of this indirect effect is novel. To the author’s knowledge, based on the Scopus document database up to January 2021, no quantitative empirical research has been found that confirms the significant effect of learning system quality on learning satisfaction with student-student interaction as a mediator. A good online learning system states about the independent learning that students can do: it facilitates and stimulates the formation of interactions with lecturers and fellow students, and it has multimedia learning materials. This study found that a quality learning system led to better interaction between students. Students were satisfied studying online at the start of the COVID-19 pandemic.
This research supports the theories of CoI and ISM. At the early stages of the COVID-19 pandemic, private universities have focused on the constructs of SP, C, SSI and LSQ to increase their LS. The findings of this work can serve as inputs for examining e-learning satisfaction in other crises. The confirmation is a construct that deserves first place regarding the increase in private university students’ learning satisfaction at the beginning of the COVID-19 pandemic. It is recommended that private universities in Indonesia prioritize confirmation to improve their students’ LS. LS is important because students are one of the main stakeholders in higher education. Especially in private universities, there are quite a lot of undergraduate students (Pangarso, Citation2019).
These universities should also focus on the constructs of SP and SSI. Private campuses make sure that their online learning systems make it easy for students to be themselves and feel accepted by their online learning environment. They should design an e-learning system that stimulates the e-learning of students and encourages their information and knowledge sharing. LSQ also appears as an important construct that facilitates student e-learning system interactions.
The practical implication or contribution of this research is in the context of full online learning during the COVID-19 pandemic, which can also be used as alternative for other similar potential crisis conditions. To achieve students’ learning satisfaction, Indonesian private university (or even other private universities in Indonesia) must prioritize confirmation. Students are especially important stakeholders, and many undergraduate students come from private universities. This enables future research agendas to delve deeper into what causes confirmation in college students, particularly during the COVID-19 pandemic. In addition, based on the effect size value, the second construct that must receive further attention is social presence. During the COVID-19 pandemic, the best Indonesian private university, as well as other private universities in Indonesia, should prioritize social presence in order to increase student learning satisfaction. How can the best Indonesian private university or private campus design an online learning system that makes students feel comfortable expressing themselves through the online learning environment? This is also a future research agenda to determine the antecedents of social presence. After social presence, the smallest effect size is student–student interaction. The research institution or a private campus should design an online learning system that stimulates students’ online learning actively in groups and shares information and knowledge. The last point is that learning system quality is also an important construct to increase interaction between students and learning satisfaction. The learning system’s quality also needs to be studied further for future research in detail about what influences it. So, if the four constructs that affect learning satisfaction either directly or indirectly can be known for their respective antecedents and it is also possible to obtain inter-antecedent influence, then this can later become a more specific novelty that can explain the practical contribution to learning satisfaction, especially in the early COVID-19 pandemic.
6. Conclusion
This study was successful in constructing a research framework based on a combination of seven theories and 10 constructs linked to e-learning satisfaction, which were examined at the onset of the COVID-19 pandemic in Indonesian private universities. In sum, social presence, confirmation and student–student interaction are the drivers of the learning satisfaction of students at the beginning of the COVID-19 pandemic. We also find that learning system quality affects learning satisfaction under the mediating influence of student–student interaction. This research contributes theoretically by supporting five theories, including the Community of Inquiry framework related to social presence; the expectation-confirmation theory or model related to confirmation; the social constructivism theory for online learning; models of online interaction theory related to student-student interaction; and the information system success model related to learning system quality. In addition, confirmation is not only the most important construct but also the construct with the largest effect size that affects the best Indonesian private university students’ learning satisfaction. Nevertheless, this construct should be ranked first in terms of increasing the best Indonesian private university students’ learning satisfaction at the beginning of the COVID-19 pandemic.
Despite the size of the sample, one of the study’s drawbacks is the selection of samples from a single university. This restricts the generalizability of the results. Future research may focus on other members of the student population, including diploma, undergraduate and graduate students, from vocational, non-vocational, public and private higher education institutions. It will even be more interesting if future research involves lecturers as research respondents because learning is initiated by lecturers, so lecturer satisfaction with online learning is also an important thing to research. Even, if possible, research can be conducted for all levels of education, including primary and secondary education, to obtain a full picture of e-learning satisfaction for higher education in Indonesia. As one of its limitations, this study was limited to the beginning of the COVID-19 pandemic. Future research could concentrate on different phases of the epidemic to complement the results of this study.
We also highlight the limitations of some extant theories. For instance, the IS success model fails to explain the effect of lecturer quality on learning satisfaction. Personal innovativeness theory fails to explain the effect of personal innovativeness on learning satisfaction and the CoI framework fails to explain the influence of engagement (both agency and emotional engagement) on learning satisfaction. These results open up opportunities for future research to re-test these three theories in different contexts. This study is also limited to only using the constructs of learning system quality and user satisfaction. Future research should use all constructs in SCT and MOI, including the interaction between lecturers and students and their learning materials.
Acknowledgements
LPPM Telkom University
Disclosure statement
No potential conflict of interest was reported by the author(s).
Additional information
Notes on contributors
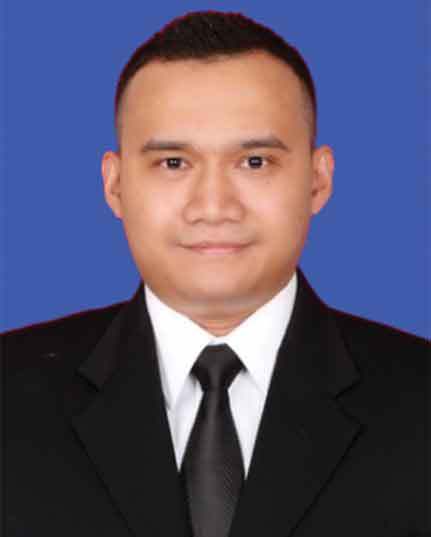
Astadi Pangarso
Our research was conducted at the beginning of the pandemic, which of course has the potential to be different from similar studies with different timescales. In the future, it is hoped that there will be research on e-learning satisfaction in the context of higher education for details of different settings so that it can complete a big picture of e-learning satisfaction before, at the beginning, in the middle, at the end, and after the pandemic is officially declared over.
Astadi Pangarso is an assistant professor at Telkom University, Bandung, Indonesia. His duties in this study are: Conceptualization; Methodology; Investigation; Writing – Original Draft; Writing – Review & Editing.
Retno Setyorini
Retno Setyorini is an assistant professor at Telkom University, Bandung, Indonesia. Her duties in this study are: Investigation; Writing – Original Draft.
References
- Agarwal, R., & Prasad, J. (1998). A Conceptual and Operational Definition of Personal Innovativeness in the Domain of Information Technology. Information Systems Research, 9(2), 204–27. https://doi.org/10.1287/isre.9.2.204
- Al-Azawei, A. (2019). What drives successful social media in education and e-learning? A comparative study on Facebook and moodle. Journal of Information Technology Education: Research, 18, 253–274. https://doi.org/10.28945/4360
- Al-Busaidi, K. A., & Al-Shihi, H. (2012). Key factors to instructors’ satisfaction of learning management systems in blended learning. Journal of Computing in Higher Education, 24(1), 18–39. https://doi.org/10.1007/s12528-011-9051-x
- Al-Maroof, R. S., & Salloum, S. A. (2021). An Integrated Model of Continuous Intention to Use of Google Classroom. In Recent Advances in Intelligent Systems and Smart Applications. Studies in Systems, Decision and Control 295 (pp. 311–335). Springer, Cham. https://doi.org/10.1007/978-3-030-47411-9_18
- Al-Rahmi, W. M., Alias, N., Othman, M. S., Alzahrani, A. I., Alfarraj, O., Saged, A. A., & Rahman, N. S. A. (2018). Use of E-Learning by University Students in Malaysian Higher Educational Institutions: A Case in Universiti Teknologi Malaysia. IEEE Access, 6, 14268–14276. https://doi.org/10.1109/ACCESS.2018.2802325
- Anderson, T. (2004). Towards a theory of online schedulers. Theory and Practice of Online Learning, 323. https://doi.org/10.1145/588053.588057
- Arbaugh, J. B. (2008). Does the Community of Inquiry Framework Predict Outcomes in Online MBA Courses? International Review of Research in Open and Distance Learning, 9(2). https://doi.org/10.19173/irrodl.v9i2.490
- Benitez, J., Henseler, J., Castillo, A., & Schuberth, F. (2020). How to perform and report an impactful analysis using partial least squares: Guidelines for confirmatory and explanatory IS research. Information and Management, 57(2), 103168. https://doi.org/10.1016/j.im.2019.05.003
- Bervell, B., Umar, I. N., & Kamilin, M. H. (2019). Towards a model for online learning satisfaction (MOLS): re-considering non-linear relationships among personal innovativeness and modes of online interaction. Open Learning, 109–119. https://doi.org/10.1080/02680513.2019.1662776
- Bhattacherjee, A. (2001). Understanding Information Systems Continuance: An Expectation-Confirmation Model. MIS Quarterly, 25(3), 351–370. https://doi.org/10.2307/3250921
- Bornsteinet al , 2013” to the referenceSampling in developmental science: Situations, shortcomings, solutions, and standards: In M. H. Bornstein, J. Jager, & D. L. Putnick (2013). 2013” to the referenceSampling in developmental science: Situations, shortcomings, solutions, and standards. Developmental Review, Vol. 33(4), pp. 357–370 https://doi.org/10.1016/j.dr.2013.08.003
- Chen, H. J. (2020). Clarifying the impact of surprise in e-learning system design based on university students with multiple learning goals orientation. Education and Information Technologies, 25(6), 5873–5892. https://doi.org/10.1007/s10639-020-10249-6
- Cheng, Y. M. (2014). Extending the expectation-confirmation model with quality and flow to explore nurses’ continued blended e-learning intention. Information Technology and People, 27(3), 230–258. https://doi.org/10.1108/ITP-01-2013-0024
- Cheng, Y. M. (2019). How does task-technology fit influence cloud-based e-learning continuance and impact? Education and Training, 61(4), 480–499. https://doi.org/10.1108/ET-09-2018-0203
- Cheng, Y. M. (2020). Students’ satisfaction and continuance intention of the cloud-based e-learning system: roles of interactivity and course quality factors. Education and Training, 62(9), 1037–1059. https://doi.org/10.1108/ET-10-2019-0245
- Cheng, Y. M. (2020). Students' satisfaction and continuance intention of the cloud-based e-learning system: Roles of interactivity and course quality factors. Education and Training, 62(9), 1037–1059. https://doi.org/10.1108/ET-10-2019-0245
- Chin, W. W. (1998). The partial least squares approach for structural equation modeling. In G. A. Marcoulides (Eds.), Modern methods for business research. Lawrence Erlbaum Associates Publishers. https://psycnet.apa.org/record/1998-07269-010
- Chin, W. W., & Newsted, P. R. (1999). Structural equation modeling analysis with small samples using partial least squares. In Statistical Strategies for Small Sample Research (pp. 307–341). Thousand Oaks: CA: Sage Publications.
- Daghan, G., & Akkoyunlu, B. (2016). Modeling the continuance usage intention of online learning environments. Computers in Human Behavior, 60, 198–211. https://doi.org/10.1016/j.chb.2016.02.066
- Dai, H. M., Teo, T., Rappa, N. A., & Huang, F. (2020). Explaining Chinese university students’ continuance learning intention in the MOOC setting: A modified expectation confirmation model perspective. Computers and Education, 150(February), 103850. https://doi.org/10.1016/j.compedu.2020.103850
- Daneji, A. A., Ayub, A. F. M., & Khambari, M. N. M. (2019). The effects of perceived usefulness, confirmation and satisfaction on continuance intention in using massive open online course (MOOC). Knowledge Management and E-Learning, 11(2), 201–214. https://doi.org/10.34105/j.kmel.2019.11.010
- DeLone, W. H., & McLean, E. R. (1992). Information systems success: The quest for the dependent variable. Information Systems Research, 3(1), 60–95. https://doi.org/10.1287/isre.3.1.60
- DeLone, W. H., & McLean, E. R. (2003). The DeLone and McLean model of information systems success: A ten-year update. Journal of Management Information Systems, 19(4), 9–30. https://doi.org/10.1080/07421222.2003.11045748
- Denson, N., Loveday, T., & Dalton, H. (2010). Student evaluation of courses : what predicts satisfaction? Higher Education Research & Development, 29(4), 339–356. https://doi.org/10.1080/07294360903394466
- Dilas, D. B., Mackie, C., Huang, Y., & Trines, S. (2019). Education in Indonesia. https://wenr.wes.org/2019/03/education-in-indonesia-2
- Dwidienawati, D., Abdinagoro, S. B., Tjahjana, D., Gandasari, D., & Munawaroh. (2020). Forced shifting to e-learning during the covid-19 outbreak: Information quality, system quality, service quality, and goal orientation influence to e-learning satisfaction and perceived performance. International Journal of Advanced Trends in Computer Science and Engineering, 9(2), 1518–1525. https://doi.org/10.30534/ijatcse/2020/93922020
- Efiloğlu Kurt, Ö. (2018). Examining an e-learning system through the lens of the information systems success model: Empirical evidence from Italy. Education and Information Technologies, 24(2), 1173–1184. https://doi.org/10.1007/s10639-018-9821-4
- Ellis, R. A., Ginns, P., & Piggott, L. (2009). E ‐ learning in higher education : some key aspects and their relationship to approaches to study. Higher Education Research & Development, 28(3), 303–318. https://doi.org/10.1080/07294360902839909
- Fatoni, Arifiati, Nurce, Nurkhayati, Etty, Nurdiawati, Ella, Fidziah, Pamungkas, Giantoro, Adha, Suhroji, Irawan, Purwanto, Agus, Julyanto, Octoberry, Azizi, Enji (2020). University Students Online Learning System During Covid-19 Pandemic: Advantages, Constraints and Solutions. Systematic Reviews in Pharmacy, 11(7), 570–576. http://dx.doi.org/10.31838/srp.2020.7.81.
- Fornell, C., & Bookstein, F. (1982). Two Structural Equation Models: LISREL and PLS Applied to Consumer Exit-Voice Theory. Journal of Marketing Research, 19(4), 440–452. https://doi.org/10.2307/3151718
- Fredricks, J. A., Blumenfeld, P. C., & Paris, A. H. (2004). School engagement: Potential of the concept, state of the evidence. Review of Educational Research, 74(1), 59–109. https://doi.org/10.3102/00346543074001059
- Fuchs, M., Bossert, D., & Stukowski, S. (2013). Response Rate and Nonresponse Bias - Impact of the Number of Contact Attempts on Data Quality in the European Social Survey. Bulletin of Sociological Methodology/Bulletin de Méthodologie Sociologiqu, 117(1), 26–45 https://doi.org/10.1177/0759106312465547.
- Garrison, D. Randy (2016). The Community of Inquiry Theoretical Framework: In the Context of Online and Blended Learning. In E-Learning in the 21st Century. Routledge 220 9781315667263 https://www.taylorfrancis.com/books/mono/10.4324/9781315667263/learning-21st-century-randy-garrison https://doi.org/10.4324/9781315667263 .
- Garrison, D. Randy (203 Social Presence 1st). . E-Learning in the 21st Century A Community of Inquiry Framework for Research and Practice (Routledge), (), 184 9780203166093 . https://doi.org/10.4324/9780203166093
- Garrison, D. R., Anderson, T., & Archer, W. (2010). The first decade of the community of inquiry framework: A retrospective. Internet and Higher Education, 13(1–2), 5–9. https://doi.org/10.1016/j.iheduc.2009.10.003
- Garrison, D. R., & Arbaugh, J. B. (2007). Researching the community of inquiry framework: Review, issues, and future directions. Internet and Higher Education, 10(3), 157–172. https://doi.org/10.1016/j.iheduc.2007.04.001
- Garrison, D. R., Cleveland-Innes, M., & Fung, T. S. (2010). Exploring causal relationships among teaching, cognitive and social presence: Student perceptions of the community of inquiry framework. Internet and Higher Education, 13(1–2), 31–36. https://doi.org/10.1016/j.iheduc.2009.10.002
- Gefen, D., Straub, D., & Boudreau, M.-C. (2000). Structural Equation Modeling and Regression: Guidelines for Research Practice. Communications of the Association for Information Systems, 4(October). https://doi.org/10.17705/1cais.00407
- Gudergan, S. P., Ringle, C. M., Wende, S., & Will, A. (2008). Confirmatory tetrad analysis in PLS path modeling. Journal of Business Research, 61(12), 1238–1249. https://doi.org/10.1016/j.jbusres.2008.01.012
- Hadoussa, S. (2020). Evaluation of e-learning system on higher education institutions in KSA: A survey at Saudi Electronic University. International Journal of Technology Enhanced Learning, 12(2), 180–199. https://doi.org/10.1504/IJTEL.2020.106285
- Hair, J. F. J., Hult, G. T. M., Ringle, C. M., & Sarstedt, M. (2017). A Primer on Partial Least Squares Structural Equation Modeling (PLS-SEM). In, Second edition. https://doi.org/10.1016/j.lrp.2013.01.002
- Hair, Jr., J. F., Sarstedt, M., Matthews, L. M., & Ringle, C. M. (2016). Identifying and treating unobserved heterogeneity with FIMIX-PLS: part I – method. European Business Review, 28(1), 63–76. https://doi.org/10.1108/EBR-09-2015-0094
- Hair, J. F., Risher, J. J., Sarstedt, M., & Ringle, C. M. (2019). When to use and how to report the results of PLS-SEM. European Business Review, 31(1), 2–24. https://doi.org/10.1108/EBR-11-2018-0203
- Hair, J. F., Sarstedt, M., Pieper, T. M., & Ringle, C. M. (2012). The Use of Partial Least Squares Structural Equation Modeling in Strategic Management Research: A Review of Past Practices and Recommendations for Future Applications. Long Range Planning, 45(5–6), 320–340. https://doi.org/10.1016/j.lrp.2012.09.008
- Herdlein, R., & Zurner, E. (2015). Student Satisfaction, Needs, and Learning Outcomes: A Case Study Approach at a European University. SAGE Open, 5(2), 215824401558037. https://doi.org/10.1177/2158244015580373
- Hulland, J., Baumgartner, H., & Smith, K. M. (2018). Marketing survey research best practices: evidence and recommendations from a review of JAMS articles. Journal of the Academy of Marketing Science, 46(1), 92–108. https://doi.org/10.1007/s11747-017-0532-y
- Hult, G. T. M., Hair, J. F., Proksch, D., Sarstedt, M., Pinkwart, A., & Ringle, C. M. (2018). Addressing endogeneity in international marketing applications of partial least squares structural equation modeling. Journal of International Marketing, 26(3), 1–21. https://doi.org/10.1509/jim.17.0151
- Hwee, J., Koh, L., Yen, R., Kan, P., Hwee, J., & Koh, L. (2020). Students ’ use of learning management systems and desired e-learning experiences : are they ready for next generation digital learning environments ? digital learning environments ? Higher Education Research & Development, 1–16. https://doi.org/10.1080/07294360.2020.1799949
- Irawan, D. E., Pangarso, A., Ridlo, I. A., & Fuad, A. (2020). Telaah bibliometrik pola penyebaran pengetahuan tentang COVID-19 di dunia. April, 1–15. https://doi.org/10.6084/m9.figshare.12084339.v3
- Isaac, O., Aldholay, A., Abdullah, Z., & Ramayah, T. (2019). Online learning usage within Yemeni higher education: The role of compatibility and task-technology fit as mediating variables in the IS success model. Computers and Education, 136(November 2017), 113–129. https://doi.org/10.1016/j.compedu.2019.02.012
- Islam, A. K. M. Najmul (2011). Extending information system continuance theory with system quality in e-learning context. 17th Americas Conference on Information Systems 2011, AMCIS 2011, 1(AIS), 50–59. https://aisel.aisnet.org/amcis2011_submissions/8
- Johnson, P., & Joanne, D. (2000). Understanding Management Research An Introduction to Epistemology.
- Kebudayaan, Biro Kerja Sama dan Hubungan Masyarakat Kementerian Pendidikan dan (2020). Panduan Penyelenggaraan Pembelajaran pada Tahun Ajaran dan Tahun Akademik Baru di Masa Covid-19. https://www.kemdikbud.go.id/main/blog/2020/06/panduan-penyelenggaraan-pembelajaran-pada-tahun-ajaran-dan-tahun-akademik-baru-di-masa-covid19
- Kock, N. (2015). Common method bias in PLS-SEM: A full collinearity assessment approach. International Journal of E-Collaboration, 11(4), 1–10. https://doi.org/10.4018/ijec.2015100101
- Kucuk, S., & Richardson, J. C. (2019). A structural equation model of predictors of online learners’ engagement and satisfaction. Online Learning Journal, 23(2), 196–216. https://doi.org/10.24059/olj.v23i2.1455
- Kuo, Y., Walker, A. E., Belland, B. R., & Schroder, K. E. E. (2013). A predictive study of student satisfaction in online education programs | Kuo | The International Review of Research in Open and Distance Learning. The International Review of Research in Open and Distance Learning, 14(1), 16. doi:10.19173/irrodl.v14i1.1338
- Kwong, K., & Wong, K. (2019). Confirmatory Tetrad Analysis, Quadratic Effect Modeling, and Heterogeneity Modeling in Partial Least Squares Structural Equation Modeling (PLS-SEM) using SmartPLS 3. Mastering Partial Least Squares Structural Equation Modeling (PLS-SEM) in 38 Hours. February, 35. https://www.researchgate.net/publication/331025056
- Lee, R., Hoe Looi, K., Faulkner, M., & Neale, L. (2020). The moderating influence of environment factors in an extended community of inquiry model of e-learning. Asia Pacific Journal of Education, 1–15. https://doi.org/10.1080/02188791.2020.1758032
- Lim, J., & Richardson, J. C. (2021). Predictive effects of undergraduate students’ perceptions of social, cognitive, and teaching presence on affective learning outcomes according to disciplines. Computers and Education, 161, 104063. https://doi.org/10.1016/j.compedu.2020.104063
- Matthews, L. M., Sarstedt, M., Hair, J. F., & Ringle, C. M. (2016). Identifying and treating unobserved heterogeneity with FIMIX-PLS: Part II – A case study. European Business Review, 28(2), 208–224. https://doi.org/10.1108/EBR-09-2015-0095
- Memon, M. A., Ting, H., Cheah, J.-H., Thurasamy, R., Chuah, F., & Cham, T. H. (2020). SAMPLE SIZE FOR SURVEY RESEARCH : REVIEW AND RECOMMENDATIONS. Journal of Applied Structural Equation Modeling, 4(2), i–xx. doi:10.47263/JASEM.4(2)01
- Moher, D., Liberati, A., Tetzlaff, J., Altman, D. G., Altman, D., Antes, G., Atkins, D., Barbour, V., Barrowman, N., Berlin, J. A., Clark, J., Clarke, M., Cook, D., D’Amico, R., Deeks, J. J., Devereaux, P. J., Dickersin, K., Egger, M., Ernst, E., & Tugwell, P. (2009). Preferred reporting items for systematic reviews and meta-analyses: The PRISMA statement. PLoS Medicine, 6(7), e1000097. https://doi.org/10.1371/journal.pmed.1000097
- Murad, D. F., Hassan, R., Heryadi, Y., Wijanarko, B. D., & Titan. (2020). The Impact of the COVID-19 Pandemic in Indonesia (Face to face versus Online Learning). Proceeding - 2020 3rd International Conference on Vocational Education and Electrical Engineering: Strengthening the Framework of Society 5.0 through Innovations in Education, Electrical, Engineering and Informatics Engineering, ICVEE 2020. IEEE. 4–7. https://doi.org/10.1109/ICVEE50212.2020.9243202
- Oliver, R. L. (1980). A cognitive model of the Antecedents and Consequences of Satisfaction Decisions. Journal of Marketing Research, 17(4), 460–469. doi:10.1177/002224378001700405
- Ooi, Keng-Boon, Hew, Jun-Jie, & Lee, Voon-Hsien (2018). Could the mobile and social perspectives of mobile social learning platforms motivate learners to learn continuously? Computers and Education, 120, 127–145. https://doi.org/10.1016/j.compedu.2018.01.017
- Pangarso, A. (2016). Lecturer sociometric badge as digital platform in Indonesia higher education institutions from organizational effectiveness perspective. Advanced Science Letters, 22(12), 4308–4311. https://doi.org/10.1166/asl.2016.8134
- Pangarso, A. (2019). Bagaimana meningkatkan kualitas universitas swasta, tempat mayoritas mahasiswa Indonesia kuliah. . https://theconversation.com/bagaimana-meningkatkan-kualitas-universitas-swasta-tempat-mayoritas-mahasiswa-indonesia-kuliah-111520?fbclid=IwAR0l4qfb-wg1miLTBu3WOkDUz_6Rrtuzof_uK2invQrO0xCv0GTzyURWD8Q
- Pangarso, Astadi (2020). Indonesian private higher education agility, the big challenge. https://medium.com/@astadipangarso/indonesian-private-higher-education-agility-the-big-challenge-4477b9a32cbe
- Pangarso, A., Astuti, E. S., Raharjo, K., & Afrianty, T. W. (2020). The impact of absorptive capacity and innovation ambidexterity on sustainable competitive advantage: the case of Indonesian higher education. Entrepreneurship and Sustainability Issues, 7(3), 2436–2455. doi:10.9770/jesi.2020.7.3(65)
- Parahoo, S. K., Santally, M. I., Rajabalee, Y., & Harvey, H. L. (2016). Designing a predictive model of student satisfaction in online learning. Journal of Marketing for Higher Education, 26(1), 1–19. doi:10.1080/08841241.2015.1083511
- Parasuraman, A., Zeithaml, V. A., & Berry, L. L. (1988). SERVQUAL: A multiple-item scale for measuring consumer perceptions of service quality. Journal of Retailing, 64(1), 12–40. https://psycnet.apa.org/record/1989-10632-001
- Park, C. W., & Kim, D. G. (2020). Exploring the Roles of Social Presence and Gender Difference in Online Learning. Decision Sciences Journal of Innovative Education, 18(2), 291–312. https://doi.org/10.1111/dsji.12207
- Pérez-Pérez, M., Serrano-Bedia, A. M., & García-Piqueres, G. (2020). An analysis of factors affecting students´ perceptions of learning outcomes with Moodle. Journal of Further and Higher Education, 44(8), 1114–1129. doi:10.1080/0309877X.2019.1664730
- Pillay, H., Irving, K., Tones, M., Pillay, H., Irving, K., & Tones, M. (2007). Validation of the diagnostic tool for assessing Tertiary students’ readiness for online learning Validation of the diagnostic tool for assessing Tertiary students’ readiness for online learning. Higher Education Research & Development, 26(2), 217–234. https://doi.org/10.1080/07294360701310821
- Podsakoff, P. M., MacKenzie, S. B., & Podsakoff, N. P. (2012). Sources of Method Bias in Social Science Research and Recommendations on How to Control It. Annual Review of Psychology, 63(1), 539–569. doi:10.1146/annurev-psych-120710-100452
- Poort, I., Jansen, E., Hofman, A., & Jansen, E. (2022). Does the group matter ? Effects of trust, cultural diversity, and group formation on engagement in group work in higher education education. Higher Education Research & Development, 41(2), 511–526. https://doi.org/10.1080/07294360.2020.1839024
- Reeve, J. (2013). How students create motivationally supportive learning environments for themselves: The concept of agentic engagement. Journal of Educational Psychology, 105(3), 579–595. https://doi.org/10.1037/a0032690
- Richter, N. F., Sinkovics, R. R., Ringle, C. M., & Schlägel, C. (2016). A critical look at the use of SEM in international business research. International Marketing Review, 33(3), 376–404. doi:10.1108/IMR-04-2014-0148
- Rigdon, E. E., Sarstedt, M., & Ringle, C. M. (2017). On Comparing Results from CB-SEM and PLS-SEM: Five Perspectives and Five Recommendations. Marketing ZFP, 39(3), 4–16. doi:10.15358/0344-1369-2017-3-4
- Ringle , 2015 : Ringle, ., Wende, S., Becker, J. M., et al (2015). SmartPLS 3. SmartPLS GmbH, Boenningstedt. http://www.smartpls.com
- Safsouf, Y., Mansouri, K., & Poirier, F. (2020). An Analysis to Understand the Online Learners’ Success in Public Higher Education in Morocco. Journal of Information Technology Education: Research, 19(March), 087–112. https://doi.org/10.28945/4518
- Sarstedt , 2019 : Sarstedt, Ringle, M., M., C., Cheah, J. -H., Ting, H., Moisescu, O. I., Radomir, L. et al (). Structural model robustness checks in PLS-SE Tourism Economics, Vol. 26(4), (pp. 531–554). https://doi.org/10.1177/1354816618823921
- Sher, A. (2009). Assessing the relationship of student-instructor and student-student interaction to student learning and satisfaction in Web-based Online Learning Environment. Journal of Interactive Online Learning, 8(2), 102–120. https://eric.ed.gov/?id=EJ938824
- Tadesse, T., Gillies, R. M., & Manathunga, C. (2020). The effects of informal cooperative learning pedagogy on teaching effectiveness, task orientation, and learning satisfaction in undergraduate classrooms in Ethiopia. Higher Education Research & Development, 1–19. https://doi.org/10.1080/07294360.2020.1765320
- Tehseen, S., Ramayah, T., & Sajilan, S. (2017). Testing and Controlling for Common Method Variance: A Review of Available Methods. Journal of Management Sciences, 4(2), 142–168. https://doi.org/10.20547/jms.2014.1704202
- Theresiawati, Seta, HenkiBayu, Hidayanto, AchmadNizar, & Abidin, Zaenal (2020). Variables affecting e-learning services quality in Indonesian higher education: Students’ perspectives. Journal of Information Technology Education: Research, 19, 259–286.: https://dx.doi.org/10.28945/4489
- Uppal, M. A., Ali, S., & Gulliver, S. R. (2018). Factors determining e-learning service quality. British Journal of Educational Technology, 49(3), 412–426. https://doi.org/10.1111/bjet.12552
- van Raaij, E. M., & Schepers, J. J. L. (2008). The acceptance and use of a virtual learning environment in China. Computers and Education, 50(3), 838–852. https://doi.org/10.1016/j.compedu.2006.09.001
- Venter, Marisa, & Swart, Arthur James (2018). An integrated model for the continuous use intention of Microsoft Office simulation software. 2018 IEEE Global Engineering Education Conference (EDUCON) 17-20 April 2018 Santa Cruz de Tenerife, Spain, (IEEE), (pp. 320–329). https://doi.org/10.1109/EDUCON.2018.8363246
- Wold, H. (1985 Partial Least Squares). . Encyclopedia of statistical sciences, 6 (John Wiley)https://onlinelibrary.wiley.com/doi/10.1002/0471667196.ess1914 https://doi.org/10.1002/0471667196.ess1914 .
- Yarrow, N., & Bhardwaj, R. (2020). Indonesia’s education technology during COVID-19 and beyond. East Asia & Pacific on the Rise. https://blogs.worldbank.org/eastasiapacific/indonesias-education-technology-during-covid-19-and-beyond
- Zareravasan, A., & Ashrafi, A. (2019, March). Influencing factors on students’ continuance intention to use Learning Management System (LMS). ACM International Conference Proceeding Series, 2020, 165–169. https://doi.org/10.1145/3357419.3357429
- Zhao, Y., Bandyopadhyay, K., & Bandyopadhyay, S. (2020). Evaluating complex online technology-enabled course delivery: A contextualized view of a decomposed IS success model. Communications of the Association for Information Systems, 46, 209–229. https://doi.org/10.17705/1CAIS.04609
- Zulherman, N., Pangarso, Z., & Zain, F. M. (2021). Factor of zoom cloud meetings: Technology adoption in the pandemic of COVID-19. International Journal of Evaluation and Research in Education, 10(3), 816–825. https://doi.org/10.11591/ijere.v10i3.21726