Abstract
Data-driven decision-making and data-intensive research are becoming prevalent in many sectors of modern society, i.e. healthcare, politics, business, and entertainment. During the COVID-19 pandemic, huge amounts of educational data and new types of evidence were generated through various online platforms, digital tools, and communication applications. Meanwhile, it is acknowledged that education lacks computational infrastructure and human capacity to fully exploit the potential of big data. This paper explores the use of Learning Analytics (LA) in higher education for measurement purposes. Four main LA functions in the assessment are outlined: (a) monitoring and analysis, (b) automated feedback, (c) prediction, prevention, and intervention, and (d) new forms of assessment. The paper concludes by discussing the challenges of adopting and upscaling LA as well as the implications for instructors in higher education.
1. Introduction
We live in a data-driven age, data is at the heart of any analysis and decision-making. International Data Corporation (IDC, Citation2019), predicts by 2022, 46% plus of global GDP will be digitized and estimates that by 2025, the global data sphere will grow to 175 zettabytes, compared to 33 zettabytes (about one trillion gigabytes) in 2018. More than 80% of this data is “unstructured”. What remains unclear is who will own this data? how will it be processed, analyzed, and modeled? What will it be used for? (Liebowitz, Citation2021). Educational institutions are also experiencing exponential growth in data generation due to developments in online learning, big data, and national accountability for evidence-based assessment and evaluation (Ferguson, Citation2012). Furthermore, advances in computing power_ according to Moor’s Law, computing power doubles every two years (Moor, 1965), cost reduction in data storage and processing due to, cloud computing, GPU (graphical processing unit), Internet of Things (IoT), digitization of existing analog data, and improvement of algorithms and machine learning open up new opportunities for higher education to enhance and transform its process and outcomes (Prodromou, Citation2021).
Although higher education has always relied on evidence and evaluation, the huge amount of data with different formats and granularity, coming from different sources and environments make it almost impossible to collect and analyze them either manually or with conventional data management systems such as SPSS. Moreover, it’s no longer enough to collect data to measure what happened; rather methods are needed to inform what is happening now and what will happen in the future in order to be well-prepared. One automated approach to achieve these goals is Learning Analytics (LA). It applies analytics techniques to discover hidden patterns inside educational datasets and uses the analysis outcome to take actions, including prediction, intervention, recommendation, personalization, and reflection (Khalil & Ebner, Citation2015).
This paper aims to examine three questions:
•Research Question 1. How does higher education exploit the potential of LA for assessmentpurposes?
•Research Question 2. What are some challenges and barriers to adapting LA in highereducation?
•Research Question 3. What are the implications of LA applications for instructors?
To answer these questions, a review of relevant literature was conducted. We examined 97 theoretical, conceptual, and empirical studies (including a few book chapters and conference proceedings) conducted on LA between 2011 (the year that LA came to emergence) and 2022. Only peer-reviewed studies which appeared in indexed, relevant journals were examined (e.g., Journal of Learning Analytics, Computers in Education, International Journal of Educational Technology in Higher Education, etc.). About 44 articles were excluded due to not being directly relevant to teaching and learning assessment. Overall, 53 works that explicitly made reference to education, assessment, and LA were put under scrutiny. It should be mentioned that for a particular section: examining four uses of LA, namely monitoring, feedback, prediction, and new forms, we predominantly used empirical studies which reported on the effectiveness of a particular tool in classrooms (i.e. Course Signals, Inq-Blotter, LOCO-Analyst, etc.).
The paper is organized in the following way: First, big data, its definition, characteristics, and uses in online learning are presented. Next, different types and functions of analytics in higher education will be explored. Specifically, four uses of LA for assessment will be analyzed, namely (a) monitoring and analysis, (b) automated feedback, (c) prediction, prevention, and intervention, and (d) new assessment forms. In the end, we reflect upon the challenges and implications of adopting LA for higher education and its instructors.
2. Online learning and big data
Online learning and its accompanying developments, such as mobile learning (e.g., learning apps), e-learning, blended learning, e-book portals, learning management systems (i.e. Moodle, Blackboard), Massive Open Online Courses (MOOCs), Open Educational Resources (OER), social networks (i.e. YouTube, Facebook), chat forums, immersive environments (i.e. online games, simulation, virtual lab) have led to an increasingly large amount of structured and unstructured data called big data (Daniel, Citation2017).
Wu et al. (Citation2014, p. 98) defined big data as “large-volume, complex, heterogeneous, growing data sets with multiple, autonomous sources”. Generally, big data is defined according to 5-V characteristics (Géczy, Citation2014) that include: volume (amount of data), variety (diversity of types and sources), velocity (speed of data generation and transmission), veracity (trustworthiness and accuracy), and value (monetary value).
In education, big data can be collected from two major sources, namely digital environments and administrative sources (Krumm et al., Citation2018). Digital environments provide both process data (i.e. log or interaction data, real-time learning behavior, habits, preferences, and instructional quality) and outcome data (test scores or drop-out rates), Administrative sources collect input data (e.g., demographics such as socio-economic, gender, race and funding) as well as survey data (e.g., students’ satisfaction, experience or attitudes), which are mostly stored in Students Information Systems (SIS). Furthermore, affective data on students’ moods or emotions such as boredom, confusion, frustration, and excitement, can also be collected from video recordings, eye tracking, sensors, handheld devices, or wearables.
Traditional data management techniques do not have the capacity to store or process such a large, complex dataset due to big data’s 5-V characteristics. Therefore, acquisition, storage, distribution, analysis, and management of big data are conducted through innovative computational technologies called Big Data Analytics (Lazer et al., Citation2014).
3. Analytics in higher education
In education, there are three areas of overlapping analytics (Lawson et al., Citation2016) including Academic Analytics (AA), Educational Data Mining (EDM), and Learning Analytics (LA). All three have a common goal: to use educational data to understand and improve processes, outcomes, and decisions. AA (Simanca et al., Citation2020) is mainly concerned with data-driven, strategic decision-making at institutional, regional, and national levels (i.e. administrative efficiency, resource allocation, financing, academic productivity, and institutional progression). EDM refers to analytic techniques application of data mining techniques, i.e. cluster mining, social network analysis, and text mining, to extract or discover patterns of certain variables in big data sets to solve educational issues, e.g., to test a learning theory or to model learners (Liñán & Pérez, Citation2015; Siemens & Baker, Citation2012). While AA involves business intelligence to enhance institutional decisions, EDM involves actionable intelligence to improve data selection and management.
LA is defined as “the measurement, collection, analysis and reporting of data about learners and their contexts for purposes of understanding and optimizing learning and the environments in which it occurs” (Long & Siemens, Citation2011, p. 34). It has its roots in assessment and evaluation, personal and social learning, data mining, and business intelligence (Dawson et al., Citation2014). Relying on disciplines such as psychology, artificial intelligence, and learning science, LA uses a variety of technologies, including data mining, social network analysis, statistics, visualization, text analytics, and machine learning (Chen & Zhang, Citation2014).
The purpose of LA is to use learner-produced data to gain actionable knowledge about learner’s behavior in order to optimize contexts and opportunities for online learning (e.g., correlating the online activity with academic performance). Since its introduction in 2011, LA has enjoyed increased attentions in academic areas (see, Figure ).
Figure 1. Studies on LA in the SCOPUS-indexed publications (L-K. Lee et al., Citation2020)
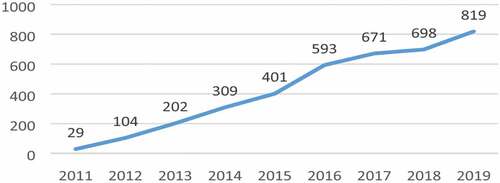
Daniel (Citation2014) identified three types of LA, namely descriptive, predictive, and prescriptive. Descriptive analytics answers the question of “what happened” by summarizing, interpreting, visualizing, and highlighting trends in data (e.g., student enrollment, graduation rates, and progression into higher degrees). Predictive analytics predicts “what will happen” based on projections made using the current conditions or historical trends (likelihood of dropping out or failing a course). Prescriptive analytics combines descriptive and predictive results to determine “what we should do next and why” to achieve desirable outcomes.
The levels of LA application is not unanimous and can take different degrees. Drawing on Picciano’s (Citation2014), Renz and Hilbig (Citation2020) indicated that with increasing data collection and analysis, the functional levels of LA in the optimization of teaching and learning will also increase. Three levels of LA applications in education, from simple to more sophisticated levels, are summarized as follows:
1. Basic LA: analytics are used to generate basic statistics of online learning and teaching behavior e.g., time spend in a course, assessment, content (video, simulation), types and number of classroom interactions, and collaborative activities (blog, wikis).
2. Recommendation LA: at the second level, analytics use several sources of data (e.g., Student Information System and online performance) to customize and tailor learning recommendations which can be done automatically, e.g., algorithm, or by human-intervention, e.g., teachers.
3. AI-Powered LA: these are the most advanced analytics which use different artificial intelligence techniques (i.e. natural language processing, machine learning, or machine vision) to provide adaptive, personalized instruction based on the constant use of predictive, diagnostic, and prescriptive analytics.
4. LA for assessment purposes
Assessment is believed to be a powerful leverage that can shape what and how teachers teach and learners learn (Black & Wiliam, Citation1998). Assessment is defined as “a process of reasoning from the necessarily limited evidence of what students do in a testing situation to claims about what they know and can do in the real world” (Zieky, Citation2014, p. 79). Unaided monitoring of individual learning has always posed a practical challenge to instructors. The digital learning environments provide multimodal, trace data_cognitive, affective, motivational, and collaborative_that allow the application of LA to infer individual competence and ability through non-intrusive, ongoing stealth assessment (DiCerbo et al., Citation2017).
Chatti et al. (Citation2012) identified different practical applications of LA, i.e. to promote reflection and awareness, estimate learners’ knowledge, monitor and feedback, adapt, recommend, etc. Although the use of analytics in assessment is distinct from the use of assessment and evaluation data for analytics, in this section both are examined to provide a comprehensive answer to the following question:
4.1. RQ1. How does higher education exploit the potential of LA for assessment purposes?
Among several functions that LA fulfills, we focus here on four specific applications of LA in assessment, namely (a) monitoring, (b) feedback, (c) intervention, and (d) new assessment forms.
4.2. Monitoring and analysis
LA is used to evaluate online performance of students. Measures such as attendance, time in the LMS, performance on quizzes, types and intensity of activities can be summarized. It may also show an aggregated summary of class/group performance. For instance, Google Analytics generates basic statistics such as frequency and mean values of the learning behaviors such as click, media choice, and chat activities. The results can be visualized as bar charts, tables, and graphs on a dashboard to instructors. For example, LOCO-Analyst aims at supporting teachers by reporting on the learning activities of students in a web-based learning environment (Romero & Ventura, Citation2020). Inq-Blotter is a dashboard that informs instructors about students’ performance and activities (Mislevy et al., Citation2020). Student dashboards can be used to track progress and give real-time reports to students about their online performance and engagement (i.e. visually display the proportion of a specific unit being completed and what is remaining). Such visual tools can promote learning autonomy by giving students more control over their own learning paths (Roberts et al., Citation2016). However, such tools do not reveal what decisions or actions should be taken to improve learning and teaching (Conde-González et al., Citation2015).
4.3. Automated feedback
Like formative assessment, LA infers learner knowledge from responses to objective questions and provides immediate, direct feedback in a form of a quantitative score. It may also give qualitative comments on why an answer is wrong or how to improve future work through explanations or tips (feedforward). Robo-graders provide automatic correction for free texts. OpenEssayist provides automated feedback on draft essays that facilitates learner reflection and development (Van Labeke et al., Citation2013).
4.4. Prediction, prevention, and intervention
Prediction. At the institutional and administrative level, one of the key functions of LA in higher education is to predict drop-out rates. Considering the negative consequences associated with drop-out, including monetary losses, poor university public image, feelings of inadequacy, and social stigmatization (Larsen et al., Citation2013), reducing attrition and improving retention have always been critical missions of educational institutions. These systems use history, log, interaction data as well as administrative data (e.g., data related to admission, attendance, completed courses, demographics, etc.) to predict which students are at the risk of leaving the program.
Prevention. At the course level, LA can act as a “warning system” to identify at-risk students and send an alert to the instructor. These students can then be warned and provided opportunities to improve their performance, i.e. face-to-face tutoring, studying in a group, and using academic advisors. Şahin and Yurdugül (Citation2020) characterized at-risk students as those learners who demonstrate the following behaviors in online environments:
• May not interact with the system after logging into the system
• May interact with the system at first, but quit interacting with it later on
• May interact with the system at a low level with very long intervals
In Germany, early-warning systems developed at Karlsruhe Institute of Technology showed an accuracy of 95% after three semesters of usage (Kemper et al., Citation2020).
Intervention. An important application of LA in assessment is to predict academic performance. The Course Signals developed at Purdue University use detailed data on engagement/mood and match them to task-level performance (Papamitsiou & Economides, Citation2014). The tool allows instructors to identify a student who might have academic challenges and contact them to offer assistance or remediation before it is too late (Arnold & Pistilli, Citation2012). AI-based software solutions, such as “Bettermark” and “Knewton”, collect data on learning behavior of individuals and classify their learning types to predict their learning success (Dräger & Müller-Eiselt, Citation2017). Continuous, formative assessment of learner performance allows profiling and modeling of learners to provide personalized, adaptive instruction. LA uses data such as performance on a quiz, time spent with the content, requested hints, patterns in errors, engagement, etc to model and predict future learning behavior. Intelligent Tutoring Systems (ITS), such as “Cognitive Tutor” in Mathematics, use LA and AI techniques to give adaptive one-to-one tutoring. Based on an early estimation of learners’ ability, it provides curated content, customized activities, appropriate testing items, immediate feedback, recommendation for the next activity and learning pathways. Information on progress and mastery of each achievable skill can be delivered immediately to instructors and learners. “ASSISTments” is an ITS in mathematics that sends teachers a report of previous homework. Teachers use such data to optimize learning materials and redesign activities, i.e. focus on questions that students found difficult (Lee et al., Citation2021).
4.5. New assessment forms
Before the advent of online environments, educational data were in different formats, dispersed in different locations, and kept by different people (teachers, administrators). Conventional assessments in higher education is conducted in contrived formats (e.g., multiple-choice, essay, or survey) with infrequent, delayed, and subjective feedback (Dede et al., Citation2016). Other evaluation methods such as self-report, observation, or interview are impractical and costly for large-scale classes and suffer from reliability issues. Technology-rich environments, with their authentic situations and high-fidelity tasks, open up opportunities for the so-called “stealth assessment”, which is an ongoing, embedded, unobtrusive, and ubiquitous form of assessment. Such assessments can occur in serious games, simulations, virtual laboratories, or social interaction in forums, in which evidence can be collected while students are performing tasks. Such process data, while learners are learning in real-time, provide more representative samples of the actual skills and evolving competencies of the individuals. They can be captured in fine granularity (minute-by-minute analysis of performance), with a strong temporal dimension and in a longitudinal manner.
5. Challenges and barriers
Higher education is at a critical juncture: on the one hand, it needs to adapt top-notch technologies to keep its relevance and competitiveness in rapidly changing socio-economic situations. On the other hand, it cannot yield the control of educational agendas, ethos and sources of information to the profit-seeking Tech lords, e.g., Microsoft, Google and Amazon, which are continuously looking for new and bigger markets (Popenici & Kerr, Citation2017). This section aims to explore the following question:
5.1. RQ2. What are some challenges and barriers to adapting LA in higher education?
Although the arguments are not exhaustive, some challenges are discussed below:
Legal: Data are institutional strategic assets. Using LA to collect and process students’ data is perceived as a legal barrier by the educational institutions (Sclater, Citation2014). Institutes need to have clear policies and procedures regarding data protection and privacy to ensure compliance with local and national legislation, such as European Union (EU) General Data Protection Regulation (GDPR; Mondschein & Monda, Citation2019).
Transparency: This is related to fairness in assessment. Through transparent criteria, we try to assure equity and equality. Many LA lack transparency due to their “black-box” nature: data are entered and results are generated, but it is not possible to identify or track which criteria are used to arrive at such results, this is especially true for machine learning (Slade et al., Citation2019).
Ethics and privacy: These issues are not only legal but also moral obligations. Analytics-based assessments require frequent access to different sources of data about learners. Learners should have the right to be informed about which data are gathered about them, where and how long they are stored, under what conditions (data security and privacy), who can access them, and for what purposes. Furthermore, risks associated with privacy (e.g., student data breach, especially data related to financial aids), misuse of data (intentional and unintentional), inappropriate interpretation and decision due to flawed, outdated data or statistical techniques should be addressed before adopting learning analytics in an institute (Millet et al., Citation2021).
Algorithmic bias: In educational measurement, several approaches are adapted to prevent bias and subjective impressions while judging quality. Big data may contain imbalanced or disproportional information. For example, if a specific ethnic background or gender performed poorly in the past course, algorithms are likely to use those demographic details to predict the failure of similar future students. This could produce systematic errors or automated discrimination that blames learners for failure, rather than a poor inclusive instructional design (Obermeyer et al., Citation2019).
Impact on robust learning: Currently, there is no overwhelming evidence that the application of LA can directly foster or improve robust learning, i.e. Learning that is retained over a long time, transfers to new situations, and prepares students for future learning (Koedinger et al., Citation2012). Although there are some empirical studies on LA (Ifenthaler & Yau, Citation2020; Sonderlund et al., Citation2018), there is a need for carefully-designed experimentation, based on sound models or frameworks in learning sciences, that allow for the selection of appropriate data for a given issue. Validation of LA tools is still an issue.
Validity issues: trustworthiness and accuracy of LA findings, especially when big data is used, are questioned in terms of problems such as overfitting (using a large number of independent variables to predict an outcome with limited scope), spurious correlation (e.g., P-hacking), generalizability, etc. A suggested solution is to supplement LA with conventional data collection methods, e.g., experiment, observation, questionnaire, interview, etc. to validate results from LA tools (Raubenheimer, Citation2021).
Human factors: New technologies require innovative methods of instruction, engagement, and assessment. Luan et al. (Citation2020) and Wheeler (Citation2019) identified that a large number of teachers are not ready to accept and adopt new technologies for different reasons such as the lack of willingness to take risks or change, lack of competence to integrate tools in their instruction, and lack of incentive or funding for anything different from traditional methods of instruction.
6. Implications for instructors
There are several stakeholders affected by LA deployment, e.g., teachers, students, administrators, leaders, future employers, etc. In this section, we focus exclusively on instructors. There is a gap between LA potential as identified in the literature and its practical applications as experienced by instructors in their daily teaching. Bates et al., (Citation2020) argued that educators themselves are not paying enough attention to the potential of LA or AI, instead they mostly focus on the negative aspects such as ethical issues and the potential to replace teachers with machines. Furthermore, educational researchers are not very active in researching LA and most scientific studies on LA come from computer sciences or STEM (Zawacki-Richter et al., Citation2019). This section will deal with the following question:
6.1. RQ 3. What are the implications of LA for Instructors?
First, there should be a constructive collaboration between technology developers and educators. Standing on the sidelines, criticizing, or avoiding participating can no longer be considered a sustainable approach. LA developers should also consider engaging instructors as one of the main stakeholders throughout the process to create learning analytics more aligned with pedagogical principles. For instance, Jivet et al. (Citation2018) indicated that dashboards’ evaluations rarely use validated instruments or consider concepts from learning sciences.
Second, higher education should invest substantially in faculty professional development by providing continuous training and supporting instructors in developing:
(a) data and visual literacy skills to interpret data meaningfully and to understand dashboards,
(b) competence in using LA to support students’ learning (pedagogical data literacy),
(c) ability to aggregate versatile data from different sources to make an informed decision,
(d) identifying discrimination and biases such as selection bias, over-reliance on outliers, and confirmation bias,
(e) building “communities of practice” with opportunities for networking, where the faculty can share their experiences, learn from “best practice examples”, and conduct participative classroom research on the use of LA tools. (Webber & Zheng, Citation2020).
However, a review of several studies of educators’ data literacy in higher education showed that these programs focus mainly on management and technical skills, instead of addressing critical, personal, and ethical approaches to datafication in education (Raffaghelli & Stewart, Citation2020).
7. Conclusion
In the last decade, universities have encountered massification, internationalization, and democratization of education, along with increasing numbers of students, cuts in financial resources, and emerging technologies (i.e. Intelligent Tutoring Systems). LA is a fast-developing field that has the potential to trigger a paradigm shift in measurement in higher education. LA can simultaneously facilitate sustainable “assessment for learning”, “assessment of learning”, and “assessment as learning” (Boud & Soler, Citation2016; Brown, Citation2005; Dann, Citation2014). This paper explored the technological and methodological applications of LA for monitoring and analysis, automated feedback, predicting drop-out, and identifying at-risk students. It also described adaptive technology that can be used to provide personalized, intelligent tutoring. It’s argued that despite the high potential and interest on the side of government, institutions, and teachers, the adoption of learning analytics mandates capabilities, i.e. substantial investment in advanced analytics and infrastructure, faculty development, policy, and regularities regarding ethics and privacy, which might not allow its full application in many educational institutes.
It should be noted that the recent advances in sensor technologies, including Electroencephalogram (EEG), eye-tracking, hand-held devices (e.g., iPad or smart glasses) and machine learning (e.g., Deep Learning algorithms) are facilitating the development of Multimodal Learning Analytics (MMLA) that can utilize rich sources of data from online, hybrid and face-to-face context (Caspari-Sadeghi, Citation2022).
Blikstein and Worsley (Citation2016, p. 233) defined MMLA as ”a set of techniques employing multiple sources of data (video, logs, text, artifacts, audio, gestures, biosensors) to examine learning in realistic, ecologically valid, social, mixed-media learning environments”. Such approaches also allow in-depth analysis of learning from cognitive, social, and behavioral perspectives at more micro-level dimensions, which in turn support teachers to understand, reflect and scaffold collaborative learning more skillfully in online environments (Ouyang et al., Citation2022).
As a concluding remark, we suggest that higher education can benefit from adopting approaches such as “learning analytics process model“ (Verbert et al., Citation2013) to integrate assessment into data-driven instruction. This model acknowledges that data in themselves are not very useful; rather the users, i.e. teachers, and students, should be involved in the “awareness-and-sensemaking” cyclical process: (1) the data is presented to users, (2) users formulate questions and assess the relevance and usefulness of data for addressing those questions, (3) users answer those questions by drawing actionable knowledge or inferring new insights, and (4) users inform and improve actions accordingly. Such data-driven decision-making, based on LA dashboards and big data, facilitates continuous modification of learning design, adapting instruction to the needs of the learners and raising the awareness and reflection of both instructors and learners about the progress towards learning outcomes and goals.
During the COVID-19 pandemic, the rapid shift to online learning led to the adoption of technological solutions mostly based on their accessibility, cost-effectiveness, and ease of use. Since LA systems are still in their infancy, more evidence-based research and evaluation should be conducted to examine issues such as privacy protection, the effectiveness of such platforms and products, short-term and long-term effects of LA tools (i.e. social isolation and engagement) by involving a wide range of stakeholders such as teachers, students, institutional leaders, and administrative staff.
Authors information
Dr. Sima Caspari-Sadeghi is currently doing her Habilitation on “Technology-Enhanced Assessment” and is also responsible for Faculty Professional Development (head of Evidence-based Evaluation) at Passau University, Germany. Her area of interest includes AI-based technologies in educational assessment.
Acknowledgement
I acknowledge support by Deutsche Forschungsgemeinschaft and University Library Passau (Open Access Publication Funding).
Disclosure statement
No potential conflict of interest was reported by the author(s).
Additional information
Funding
References
- Arnold, K. E., & Pistilli, M. D. (2012). Course signals at Purdue: Using learning analytics to increase student success. Proceedings of the 2nd international conference on learning analytics and knowledge (LAK ’12), 267–11.
- Bates, Y., Cobo, C., Mariño, O., & Wheeler, S. (2020). Can artificial intelligence transform higher education. International Journal of Educational Technology in Higher Education, 14(42). https://doi.org/10.1186/s41239-020-00218-x
- Black, P., & Wiliam, D. (1998). Inside the black box: Raising standards through classroom assessment. Phi Delta Kappan, 80(2), 139–148. https://kappanonline.org/inside-the-black-box-raising-standards-through-classroom-assessment/
- Blikstein, P., & Worsley, M. (2016). Multimodal learning analytics and education data mining: Using computational technologies to measure complex learning tasks. Journal of Learning Analytics, 3(2), 220–238. https://doi.org/10.18608/jla.2016.32.11
- Boud, D., & Soler, R. (2016). Sustainable assessment revisited. Assessment & Evaluation in Higher Education, 41(3), 400–413. https://doi.org/10.1080/02602938.2015.1018133
- Brown, S. (2005). Assessment for Learning. Learning & Teaching in Higher Education, 1, 81–89. http://eprints.glos.ac.uk/id/eprint/3607
- Caspari-Sadeghi, S. (2022). Applying learning analytics in online environments: Measuring learners’ engagement unobtrusively. Frontiers in Education, 7, 840947. https://doi.org/10.3389/feduc.2022.840947
- Chatti, M. A., Dyckhoff, A. L., Schroeder, U., & Thüs, H. (2012). A reference model for learning analytics. International Journal of Technology Enhanced Learning, 4(5/6), 318–331. https://doi.org/10.1504/IJTEL.2012.051815
- Chen, C., & Zhang, C. (2014). Data-intensive applications, challenges, techniques and technologies: A survey on Big Data. Information Sciences, 275, 314–347. https://doi.org/10.1016/j.ins.2014.01.015
- Conde-González, M. Á., Hernández-García, Á., García-Peñalvo, F. J., & Sein-Echaluce, M. L. (2015). Exploring student interactions: Learning analytics tools for student tracking. In P. Zaphiris & I. Ioannou (Eds.), Learning and Collaboration Technologies. Second International Conference, LCT 2015 (pp. 50–61). Switzerland: Springer International Publishing.
- Daniel, B. K. (2014). Big data and analytics in higher education: Opportunities and challenges. British Journal of Educational Technology, 46(5), 904–920. https://doi.org/10.1111/bjet.12230
- Daniel, B. K. (2017). Overview of big data and analytics in higher education. In B. K. Daniel (Ed.), Big data and learning analytics in higher education: Current theory and practice (pp. 1–4). Springer, Cham. https://doi.org/10.1007/978-3-319-06520-5_1
- Dann, R. (2014). Assessment as learning: Blurring the boundaries of assessment and learning for theory, policy and practice. Assessment in Education: Principles, Policy & Practice, 21(2), 149–166. https://doi.org/10.1080/0969594X.2014.898128
- Dawson, S., Gašević, D., Siemens, G., & Joksimovic, S. (2014). Current State and Future Trends: A Citation Network Analysis of the Learning Analytics Field. In Proceedings of the Fourth International Conference on Learning Analytics And Knowledge (pp. 231–240). New York, NY, USA: ACM.
- Dede, C., Ho, A., & Mitros, P. (2016). Big data analysis in higher education: Promises and pitfalls. EDUCAUSE Review, 51(5), 23–34. https://er.educause.edu/articles/2016/8/big-data-analysis-in-higher-education-promises-and-pitfalls
- DiCerbo, K. E., Shute, V., & Kim, Y. J. (2017). The future of assessment in technology rich environments: Psychometric considerations of ongoing assessment. In J. M. Spector, B. Lockee, & M. Childress (Eds.), Learning, design, and technology: An international compendium of theory, research, practice, and policy (pp. 1–21). Springer.
- Dräger, J., & Müller-Eiselt, R. (2017). Die digitale Bildungsrevolution. Der radikale Wandel des Lernens und wie wir ihn gestalten können. Deutsche Verlags-Anstalt.
- Ferguson, R. (2012). Learning analytics: Drivers, developments and challenges. International Journal of Technology Enhanced Learning, 4(5–6), 304–317. https://doi.org/10.1504/IJTEL.2012.051816
- Géczy, P. (2014). Big Data Characteristics. The Macrotheme Review: A Multidisciplinary Journal of Global Macro Trends, 3(6), 94–104. https://macrotheme.com/yahoo_site_admin/assets/docs/8MR36Pe.97110828.pdf
- IDC FutureScape.: Worldwide Artificial Intelligence 2020 Predictions. (2019). ( IDC Doc #US45576319, Oct 2019).https://www.seagate.com/files/www-content/our-story/trends/files/idc-seagate-dataage-whitepaper.pdf.
- Ifenthaler, D., & Yau, J. Y.-K. (2020). Utilising learning analytics for study success: A systematic review. Educational Technology Research and Development, 68(4), 1961–1990. https://doi.org/10.1007/s11423-020-09788-z
- Jivet, I., Scheffel, M., Specht, M., & Drachsler, H. (2018). License to evaluate: Preparing learning analytics dashboards for educational practice. In Proceedings of the 8th International Conference on Learning Analytics and Knowledge: (LAK ‘18) (pp. 32–40). Association for Computing Machinery (ACM).
- Kemper, L., Vorhoff, G., & Wigger, B. U. (2020). Predicting student dropout: A machine learning. European Journal of Higher Education, 10(1), 28–47. https://doi.org/10.1080/21568235.2020.1718520
- Khalil, M., & Ebner, M. (2015). Learning analytics: Principles and constraints. In Proceedings of ED-Media 2015 conference.
- Koedinger, K. R., Corbett, A. T., & Perfetti, C. (2012). The knowledge–learning–instruction (KLI) framework bridging the science–practice chasm to enhance robust student learning. Cognitive Science, 36(5), 757–798. https://doi.org/10.1111/j.1551-6709.2012.01245.x
- Krumm, A., Means, B., & Bienkowski, M. (2018). Learning analytics goes to school: A collaborative approach to improving education. Routledge.
- Larsen, M. S., Kornbeck, K. P., Kristensen, R. M., Larsen, M. R. &., & Sommersel, H. B. (2013). Dropout Phenomena at Universities: what is dropout? why does dropout occur? what can be done at universities to prevent or reduce it? A systematic review. Danish Clearinghouse for Educational Research, Department of Education, Aarhus University.
- Lawson, C., Beer, C., Dolene, R., Moore, T., & Fleming, J. (2016). Identification of “At Risk” students using learning analytics: The ethical dilemmas of intervention strategies in higher education institution. Educational Technology Research & Development, 64(5), 957–968. https://doi.org/10.1007/s11423-016-9459-0
- Lazer, D., Kennedy, R., King, G., & Vespignani, A. (2014). Big data. The parable of Google Flu: Traps in big data analysis. Science, 343(6176), 1203–1205. https://doi.org/10.1126/science.1248506
- Lee, L.-K., Cheung, S. K. S., & Kwok, L.-F. (2020). Learning analytics: Current trends and innovative practices. Journal of Computers in Education, 7(1), 1–6. https://doi.org/10.1007/s40692-020-00155-8
- Lee, C.-A., Tzeng, J.-W., Huang, N.-F., & Su, Y.-S. (2021). Prediction of student performance in massive open online courses using deep learning system based on learning behaviors. Educational Technology & Society, 24(3), 130–146. https://www.jstor.org/stable/27032861
- Liebowitz, J. (Ed.). (2021). Online learning analytics. Auerbach Publications.
- Liñán, L. C., & Pérez, A. A. J. (2015). Educational data mining and learning analytics: Differences, similarities, and time evolution. International Journal of Educational Technology in Higher Education, 12(3), 98–112. http://dx.doi.org/10.7238/rusc.v12i3.2515
- Long, P., & Siemens, G. (2011). Penetrating the Fog: Analytics in learning and education. EDUCAUSE Review, 31–40. https://er.educause.edu/articles/2011/9/penetrating-the-fog-analytics-in-learning-and-education
- Luan, H., Geczy, P., Lai, H., Gobert, J., Yang, S. J. H., Ogata, H., Baltes, J., Guerra, R., Li, P., & Tsai, C. (2020). Challenges and future directions of big data and artificial intelligence in education. Frontiers in Psychology, 11, 580820. https://doi.org/10.3389/fpsyg.2020.580820
- Millet, C., Resig, J., & Pursel, B. (2021). Democratizing data at a large R1 institution supporting data-informed decision-making for advisers, faculty, and instructional designers. In J. Liebowitz (Ed.), Online learning analytics (pp. 115–143). Auerbach Publications.
- Mislevy, R. J., Yan, D., Gobert, J., & Sao Pedro, M. (2020). Automated scoring in intelligent tutoring systems. In D. Yan, A. A. Rupp, & P. W. Foltz (Eds.), Handbook of Automated Scoring (pp. 403–422). Chapman and Hall/CRC.
- Mondschein, C. F., & Monda, C. (2019). The EU’s general data protection regulation (GDPR) in a Research Context. In P. Kubben, M. Dumontier, & A. Dekker (Eds.), Fundamentals of Clinical Data Science (pp. 55–71). Springer. https://doi.org/10.1007/978-3-319-99713-1_5
- Obermeyer, Z., Powers, B., Vogeli, C., & Mullainathan, S. (2019). Dissecting racial bias in an algorithm used to manage the health of populations. Science, 366(6464), 447–453. https://doi.org/10.1126/science.aax2342
- Ouyang, F., Dai, X., & Chen, S. (2022). Applying multimodal learning analytics to examine the immediate and delayed effects of instructor scaffoldings on small groups’ collaborative programming. International Journal of STEM Education, 9(45), 1–21. https://doi.org/10.1186/s40594-022-00361-z
- Papamitsiou, Z., & Economides, A. A. (2014). Learning analytics and educational data mining in practice: A systematic literature review of empirical evidence. Educational Technology & Society, 17(4), 49–64. http://www.jstor.org/stable/jeductechsoci.17.4.49
- Picciano, A. G. (2014). Big data and learning analytics in blended learning environments: Benefits and concerns. International Journal of Artificial Intelligence and Interactive Multimedia, 2(7), 35–43. https://doi.org/10.9781/ijimai.2014.275
- Popenici, S. A., & Kerr, S. (2017). Exploring the impact of artificial intelligence on teaching and learning in higher education. Research and Practice in Technology Enhanced Learning, 12(1), 22–29. https://doi.org/10.1186/s41039-017-0062-8
- Prodromou, T. (Ed.). (2021). Big Data in Education: Pedagogy & Research. Springer Nature.
- Raffaghelli, J. E., & Stewart, B. (2020). Centering complexity in ‘educators’ data literacy’ to support future practices in faculty development: A systematic review of the literature. Teaching in Higher Education, 25(4), 435–455. https://doi.org/10.1080/13562517.2019.1696301
- Raubenheimer, J. (2021). Big data in academic research: Challenges, pitfalls, and opportunities. In Prodromou (Ed.), Big Data in Education: Pedagogy and Research (pp. 3–37). Springer, Cham.
- Renz, A., & Hilbig, R. (2020). Prerequisites for artificial intelligence in further education: Identification of drivers, barriers, and business models of educational technology companies. International Journal of Educational Technology in Higher Education, 17(14). https://doi.org/10.1186/s41239-020-00193-3
- Roberts, L. D., Howell, J. A., Seaman, K., & Gibson, D. C. (2016). Student attitudes toward learning analytics in higher education: “The Fitbit version of the learning world”. Frontiers in Psychology, 7, 1–11. https://doi.org/10.3389/fpsyg.2016.01959
- Romero, C., & Ventura, S. (2020). Educational data mining and learning analytics: An updated survey. WIREs: Data Mining and Knowledge Discovery.10, 1355. https://doi.org/10.1002/widm.1355
- Şahin, M., & Yurdugül, H. (2020). The Framework of Learning Analytics for Prevention, Intervention, and Postvention in E-Learning Environments. In D. Ifenthaler & D. Gibson (Eds.), Adoption of Data Analytics in Higher Education Learning and Teaching (pp. 53–69). Springer.
- Sclater, N. (2014). Code of practice for learning analytics: A literature review of the ethical and legal issues. Joint Information System Committee (JISC). http://analytics.jiscinvolve.org/wp/2015/06/04/code-of-practice-for-learning-analytics-launched/
- Siemens, G., & Baker, R. (2012). Learning analytics and educational data mining: Towards communication and collaboration. Proceedings of the second international conference on learning analytics & knowledge (pp. 252–254). ACM.
- Simanca, H.F. A., Hernández Arteaga, I., Unriza Puin, M. E., Blanco Garrido, F., Paez Paez, J., Cortes Méndez, J., & Alvarez, A. (2020). Model for the collection and analysis of data from teachers and students supported by Academic Analytics. Procedia Computer Science, 177, 284–291. https://doi.org/10.1016/j.procs.2020.10.039
- Slade, S., Prinsloo, P., & Khalil, M. (2019). Learning analytics at the intersections of student trust, disclosure and benefit. In J. Cunningham, N. Hoover, S. Hsiao, G. Lynch, K. McCarthy, C. Brooks, R. Ferguson, & U. Hoppe (Eds.), ICPS proceedings of the 9th international conference on learning analytics and knowledge – LAK 2019 (pp. 235–244). New York: ACM.
- Sonderlund, A., Hughes, E., & Smith, J. R. (2018). The efficacy of learning analytics interventions in higher education: A systematic review. British Journal of Educational Technology, 50(5), 2594–2618. https://doi.org/10.1111/bjet.12720
- Van Labeke, N., Whitelock, D., Field, D., Pulman, S., & Richardson, J. T. E. (2013). OpenEssayist: Extractive summarisation and formative assessment of free-text essays. In: Proceedings of the 1st International Workshop on Discourse-Centric Learning Analytics. April 2013, Leuven, Belgium.
- Verbert, K., Duval, E., Klerkx, J., Govaerts, S., & Santos, J. L. (2013). Learning analytics dashboard applications. American Behavioral Scientist, 57(10), 1500–1509. https://doi.org/10.1177/0002764213479363
- Webber, K. L., & Zheng, H. (2020). Big Data on Campus. Data Analytics and Decision Making in Higher Education. Johns Hopkins University Press.
- Wheeler, S. (2019). Digital learning in organizations. Kogan Page.
- Wu, X., Zhu, X., Wu, G. Q., & Ding, W. (2014). Data mining with big data. IEEE Transactions on Knowledge and Data Engineering, 26(1), 97–107. https://doi.org/10.1109/TKDE.2013.109
- Zawacki-Richter, O., Marin, V., Bond, M., & Gouverneur, F. (2019). A systematic review of research on artificial intelligence applications in higher education – Where are the educators? International Journal of Educational Technology in Higher Education, 16(39), 2–27. https://doi.org/10.1186/s41239-019-0171-0
- Zieky, M. J. (2014). An introduction to the use of evidence-centered design in test development. Psicología Educativa, 20(2), 79–87. https://doi.org/10.1016/j.pse.2014.11.003