Abstract
Introduction: Knowledge of results (KR) is a method of augmented feedback where frequency or timing varies depending on the individual’s performance. In previous studies, bandwidth (BW) KR was not established according to the individual’s performance; thus, it is unclear how BW should be set based on changes in individual performance. Therefore, this study investigated the effectiveness of adjusted BW KR. Method: Forty-seven participants were divided into four groups (control, narrow BW, wide BW, and adjusted BW). The adjustability of the grasping force task was measured by having participants perform the task for 60 seconds, with each group receiving a different KR. We used the absolute constant error (ACE) and variable error (VE) as respective measures of the accuracy and consistency of the movements, and the sum of ACEs outside of the BW as a measure of the amount of error information. Measurements (pretest, practice sessions, and immediate- and delayed-retention tests) were performed over the course of two days. Results: The adjusted BW group showed a significant decrease in VE compared with the wide BW group in the immediate-retention test. Conclusion: This study partially indicates the effectiveness of adjusted BW KR compared to traditional BW KR.
1. Introduction
Augmented feedback (FB) refers to information that enhances motor learning (Sigrist et al., Citation2013). Augmented FB implies externally informed FB (quantitatively and/or qualitatively) as opposed to intrinsic FB (Lauber & Keller, Citation2014). Knowledge of results (KR) is a type of augmented FB, featuring verbalizable information about the results of a movement task (APA Dictionary of Psychology, Citationn.d.). KR also includes non-verbal information about the results of a movement, such as visual or auditory information. The effects of KR guide a learner to identify and correct errors to achieve goal movement. Previous research has shown that KR affects an individual’s performance by manipulating the frequency (e.g., 100% KR, 50% KR) and timing (e.g., concurrent, terminal) of providing KR (Schmidt, Citation1988; Winstein, Citation1991). Guadagnoli and Lee (Citation2004) noted that practice conditions, including the method of giving KR, influenced the amount of information available to learners to improve and enhance learning in the challenge point framework (CPF). For example, even if a learner’s skill level and nominal task difficulty were the same, the functional task difficulty would change depending on the method of giving KR. Therefore, to enhance performance and facilitate motor learning, it is necessary to practice at the optimal challenge point that is commensurate with the learner’s information processing ability and the amount of information obtained from the results of performing a task. Furthermore, Wulf & Lewthwaite, Citation2016) reported that KR enhances learner’s performance by increasing their intrinsic motivation in the optimizing performance through intrinsic motivation and attention for learning theory (OPTIMAL theory).
Bandwidth KR (BW KR) is an effective way to enhance motor learning (Sherwood, Citation1988). BW implies the error tolerance for the success of the task. When the movement exceeds the BW, learners receive KR to improve their movement. Conversely, when the movement falls within the BW, learners do not receive information about the movement (e.g., “going well”), but show no KR. Therefore, the BW KR is assumed to be an effective method based on the guidance hypothesis (Lee et al., Citation1990). Salmoni et al. (Citation1984) reported that learners hinder their motor learning through dependency on excessive KR present in the guidance hypothesis. However, the effects of BW KR differ based on the type of task or manipulation of the BW.
Studies have examined the effects of BW KR in various timing tasks (Goodwin & Meeuwsen, Citation1995; Badets & Blandin, Citation2005, Citation2010; Ishikura, Citation2008; Lai et al., Citation2000; Lee & Maraj, Citation1994; Sherwood, Citation1988; Smith et al., Citation1997). For example, Ishikura (Citation2011) examined the effects of BW based on the standard deviation of performance time on motor learning, using a timing task. The author compared the performance of three groups: 100% BW group, 10% BW group, and fluctuating BW group. The 100% BW group received KR for every practice trial, whereas the 10% BW and fluctuating BW group received KR when their performance time deviated from the BW tolerance. The BW tolerance of 10% BW group was set to 10% of the target time, and the BW tolerance of the fluctuating BW group varied with the standard deviation of the performance time of the five previous trials by individual participants. Therefore, the BW tolerance under the 10% BW group was constant, but the BW tolerance under the fluctuating group was variable. The results indicated that the mean standard deviation of error time and variable error (VE) for the fluctuating BW group were lower than the 100% BW group. However, there was no difference in these errors between the fluctuating BW group and the 10% BW group. This study could not reveal the effectiveness of BW based on the individual’s standard deviation of performance.
Previous studies have reported several motor tasks and methods for establishing BW (Butler et al., Citation1996; Cauraugh et al., Citation1993; Ugrinowitsch et al., Citation2010). However, the method to determine the optimal BW has not been established. Therefore, this study aims to establish a way to determine optimal BW according to the individual’s performance on the force control task. Previous studies did not determine the BW based on the individual’s performance accuracy; rather, this was left to the discretion of the researcher (e.g., 5% BW KR, 10% BW KR, or 15% BW KR; Goodwin & Meeuwsen, Citation1995; Badets & Blandin, Citation2005, Citation2010; Lai et al., Citation2000; Lee & Maraj, Citation1994; Salmoni et al., Citation1984; Sherwood, Citation1988; Smith et al., Citation1997). This study developed the adjusted BW KR method that shows the BW changes based on the individual’s performance accuracy. Our hypothesis is that the adjusted BW KR condition shows better performance and motor learning than the other conditions.
2. Materials and methods
2.1. Participants
A power analysis was conducted to estimate sample sizes using G*Power 3.1.9.4 (Heinrich Heine University Düsseldorf, Düsseldorf, Germany). We used repeated measured analysis of variance (ANOVA), within-between interaction with α error level probability of .05 and power (1‐β error probability) of 80%. An effect size of Cohen’s f = .25. was expected based on the effect size reported in a previous study (Yamamoto et al., Citation2022). As a result of the sample size calculation, a minimum of 7 participants per condition were required.
47 healthy young adults (21 men and 26 women; mean age of years 22.0 ± 1.3 years) participated in this study. All participants were right-handed and had never performed the experimental task. They were informed about the study and provided written informed consent. This study was approved by the Ethics Committee of Ibaraki Prefectural University of Health Sciences (approval number: 744).
2.2. Apparatus
All participants used a grasping device (iWakka, Aimu, Co., Ltd) in this study (Figure ). This device consisted of a cylindrical PVC pipe with a height of 80 mm and diameter of 65 mm with an attached plate spring. The change of grasping force was detected by using a strain gauge to measure the strain of the plate spring caused by opening and closing the grasping device. Participants were shown the difference between the target grasping force and the measured grasping force on the monitor. This grasping device measured the target grasping force to a maximum of 0.4 kg, changeable to 0.1-second intervals. The adjustability of grasping force (AGF) is defined as the error between the target and the measured grasping force. In other words, the AGF indicates the superiority of a small discrepancy between target and measured grasping forces. Kaneno et al. (Citation2017) reported that assessing the AGF using this grasping device in healthy young adults is valid.
2.3. Experimental conditions and tasks
Participants sat in a chair with their hands on the desk in front of them. We placed the monitor in the center of the desk and set an aluminum plate underneath the grasping device to reduce any friction with the floor. Participants wore an eye mask to prevent visual information during the trial. We used a metronome (60 bpm) with a sound that changed every five seconds to guide them when to grasp the device. Furthermore, an input device, “Nandemo switch USB” (Technotools, Japan), was used to synchronize the start of the measurement with the metronome. Participants were instructed to adjust their grasping force to make the error as small as possible. The experimental task consisted of three phases (total 60 seconds). Participants were required to gradually increase the grasping force from 0 to 0.4 kg in the first half of the first phase (5–10 seconds), then maintain it at 0.4 kg in the second half of the first phase (10–15 seconds). Next, participants were required to gradually increase the grasping force from 0 to 0.3 kg in the first half of the second phase (25–30 seconds), then maintain it at 0.3 kg in the second half of that phase (30–35 seconds). Finally, participants were required to gradually increase the grasping force from 0 to 0.2 kg in the first half of the third phase (45–50 seconds), then maintain it at 0.2 kg in the second half of the third phase (50–55 seconds). Other phases (0–5 seconds, 15–25 seconds, 35–45 seconds, and 55–60 seconds) were interval phases (Figure ).
2.4. Procedure
We randomly assigned participants to one of the four conditions by lottery method. All participants drew one of four lots with a group’s name it. We stopped recruiting when the number of participants reached 10 or more in all groups. Hence, the number of participants in each condition differed. The four conditions included the following: a control condition with no BW (n = 11), a narrow BW condition with ± 0.05 kg BW of the target grasping force (n = 14), a wide BW condition with ± 0.10 kg BW of the target grasping force (n = 10), and an adjusted BW condition that set the average of the absolute constant error (ACE) of the previous block to the BW (n = 12; Figure ). The control condition presented the difference between the target and measured results with no BW. The narrow BW condition presented error information when the measurement result exceeded the target grasping force by ± 0.05 kg. The wide condition presented error information when the measurement result exceeded ± 0.10 kg of the target grasping force. This means that the differences between narrow and wide conditions lie in the different range of errors that are allowed. The adjusted BW condition is the only condition that changes the tolerance of BW based on performance. The BW of block 2 was the tolerance of the average ACE of block 1 of the participants, and the BW of block 3 was the tolerance of the average ACE of block 2 of the participants.
Figure 3. KR displayed for each practice condition. target grasping force shown as dashed lines and measured grasping force shown as solid lines. The black-filled area indicates the BW. (A) The control condition has no BW. (B) The narrow BW condition has a tolerance of ± 0.05 kg BW. (C) The wide BW condition has a tolerance of ± 0.10 kg BW. (D) The adjusted BW condition changes the tolerance of the BW based on the previous block’s performance. The narrow BW condition and the wide BW condition did not change BW, and the adjusted BW condition only changed the tolerance of the BW (block arrows indicate changes in the tolerance of the BW).
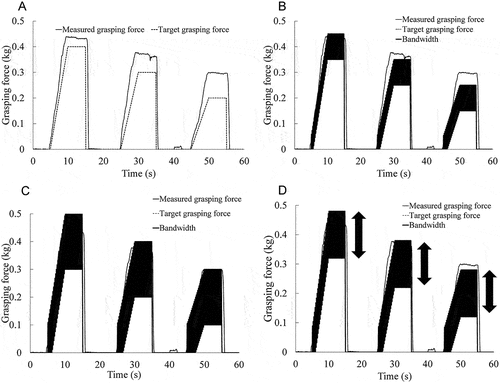
The measurement was conducted over two days: the pretest, practice sessions, and immediate-retention test on the first day, and the delayed-retention test on the second day. The pre- and retention tests included five trials each under no-KR condition. The pretest was started after a 90 second trial of a grasping force task that differed from the measurement task, to familiarize participants with the grasping device. Five minutes after completing the pretest, participants performed the first day’s practice sessions that included 15 trials. Five trials were considered as one block for practice sessions, with a one-minute rest period between blocks. KR was provided after completing the trial and presented on the monitor for 10 seconds.
2.5. Outcomes
ACE. ACE was a measure of overall performance accuracy, and represents the absolute value of the constant error from the target and measured values. ACE was calculated as the sum of the three phases (5–15 seconds, 25–35 seconds, and 45–55 seconds).
VE. VE was a measure of overall performance consistency, and represents the standard deviation of the constant error from the target and measured values. VE was calculated as the sum of the three phases (5–15 seconds, 25–35 seconds, and 45–55 seconds).
The sum of the ACEs outside of the BW. The sum of the ACEs outside of the BW represents the amount of error information contained in BW KR. This score was calculated as the sum of ACE from the measured value and the upper or lower limit of BW, not the target value. As participants could only recognize errors in parts that deviated from BW when they were provided the KR, this score accurately reflects the amount of error information. If this score is small, the participants perceived that the task was going well.
2.6. Data analysis
The difference in measured variables (ACE and VE) between the four conditions (group) and six blocks (test and practice sessions) were evaluated using two-way repeated ANOVAs. The post hoc tests were conducted using the Holm method for groups and blocks. Based on previous studies (Yamamoto et al., Citation2019; Yamamoto & Ohashi, Citation2014), we analyzed all trial blocks to include performance transitions from pretest to practice sessions and practice sessions to retention-test. The difference in the sum of ACEs outside of the BW between the three BW conditions (BW group only) and three blocks (practice only) were evaluated using a two-way ANOVA. The post hoc tests were conducted using the Holm method for conditions and blocks.
All statistical analysis tests were computed using SPSS (Version 25) and R (Version 4.2.1) with “ANOVA-kun.” The significance level was set at p < .05.
3. Results
Figure shows the trends of BW in practice sessions. The adjusted BW condition was gradually narrowed as the practice block progressed (± 0.13 kg, ± 0.08 kg, and ± 0.06 kg).
Figure 4. The trends of BW trend in practice sessions (Blocks 1–3). The narrow BW and wide BW conditions did not change bandwidth during the practice sessions, while the adjusted BW condition gradually narrowed bandwidth through the practice sessions.
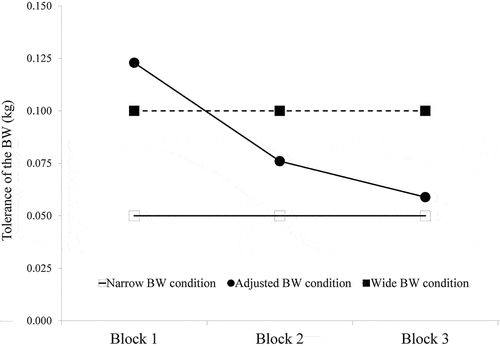
3.1. ACE
Figure shows the trends of the ACE. For repeated measures analyses, the Greenhouse-Geisser correction was applied to adjust for sphericity. The two-way ANOVA revealed a significant main effect of the block, F (2.112, 90.809) = 33.847, p < .001, ηG2 = .047. However, the group × blocks interaction, F (6.335, 90.809) = 1.243, p = .291, ηG2 = .053, was not significant. The post hoc analysis using the blocks indicated a significant decrease in all practice blocks and immediate-retention tests compared with the pretest (all p < .001).
Figure 5. The trends of ACE (pretest–delay retention-test). Five trials were conducted for each block, and the test block was conducted without KR. Error bars show standard deviation. the control condition is shown as white circles and dashed lines, the narrow BW condition as white squares and solid lines, the wide BW condition as black squares and dashed lines, and the adjusted BW condition as black circles and solid lines.
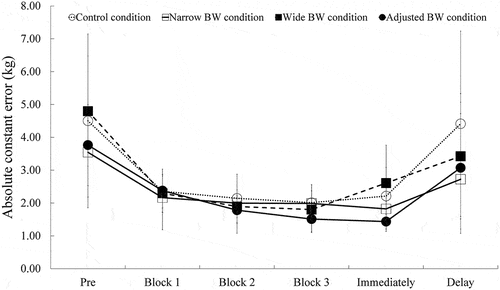
VE
Figure shows the results of the VE. For repeated measures analyses, the Greenhouse-Geisser correction was applied to adjust for sphericity. The two-way ANOVA revealed a significant main effect of the blocks, F (2.49, 107.27) = 22.991, p < .001, ηG2 = .147, and the group × blocks interaction, F (7.48, 107.27) = 2.231 p = .034, ηG2 = .048. The post hoc test for the group indicated that the adjusted BW group was better than the wide BW group in the immediate-retention test (p = .023). The post hoc test for the blocks of each practice condition indicated a significant decrease from the pretest to the delayed-retention test in three BW conditions (all p < 0.01). However, there was no significant difference between the pretest and delayed-retention test in the control condition (p = .376).
Figure 6. The trends of VE (pretest–delay retention-test). Five trials were conducted for each block, and the test block was conducted without KR. Error bars show standard deviation. The control condition is shown as white circles and dashed lines, the narrow BW condition as white squares and solid lines, the wide BW condition as black squares and dashed lines, and the adjusted BW condition as black circles and solid lines.
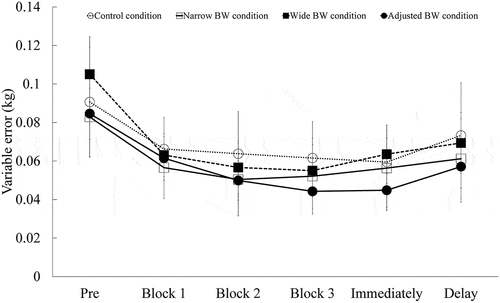
3.2. The sum of ACEs outside of the BW
Figure shows the trends of the sum of ACEs outside of the BW. Maucly’s sphericity was applied in this analysis. The two-way ANOVA revealed a significant main effect of the group, F (2, 33) = 7.892, p = .002, ηG2 = .265. However, the main effect of blocks F, (2, 66) = .801, p = .453, ηG2 = .006, and the group × blocks interaction, F (4, 66) = .336, p = .853, ηG2 = .050, were not significant. The post hoc analysis for the main effects of the group indicated that the narrow BW condition was larger than other two BW conditions (narrow BW condition vs adjusted BW condition p= .003, narrow BW condition vs wide BW condition p = .009). However, there was no significant difference between the adjusted BW condition and the wide BW condition (p = 0.490).
4. Discussion
Previous BW studies examined the effects of the difference between quantitative KR (error information) and no-quantitative KR (absence of quantitative KR represents information; Cauraugh et al., Citation1993), and the influence of the difference in the BW tolerance (0%, 5%, 10%, and 15%) on skill acquisition (Ugrinowitsch et al., Citation2010). Although BW KR was effective for skill acquisition, the method of setting the tolerance of BW was determined by the individual researcher and was not based on the learner’s performance accuracy. Therefore, this study aimed to determine the effectiveness of adjusted BW, where tolerance of BW varies based on individual performance accuracy.
We found no significant differences in the performance accuracy between the BW conditions. Previous studies have reported several different results regarding the effect of BW KR on performance accuracy. Lee and Maraj (Citation1994) showed that BW KR improved performance accuracy in a simple timing task. However, Agethen and Krause (Citation2016) could not clarify the performance accuracy in the BW KR group better than the no-BW KR group in the extension-flexion sequence with three movement reversals tasks (70°, 20°, and 70°). In addition, Coca-Ugrinowitsch et al. (Citation2014) showed that performance accuracy in the two BW KR groups (narrow BW group and wide BW group) were not better than the no- BW KR group (control group) in a task involving throwing a saloon dart. Thus, the effect of BW KR could depend on task complexity. Our results failed to show that the three BW KR groups (narrow BW KR group, wide BW KR group, and adjusted BW KR group) had better performance accuracy than the control group. The grasping force task used in this study was considered a simple task for healthy young participants. However, the task may have required participants to process a considerable amount of information because it was a continuous task with relatively long trial times. According to the CPF, functional task difficulty depends on the information obtained from the task performance and the learner’s ability to process information, and excessive amounts of information inhibit learning (Guadagnoli & Lee, Citation2004). Thus, in this study, the functional task difficulty might have been increased. In addition, at the appropriate time, participants were required to adjust the AGF (0–0.4 kg) to a smaller grip strength through a few practices. In daily life, about 20% of one’s maximum muscle strength was exerted (Kern et al., Citation2001), thus suggesting the AGF to be a small grasping force not experienced by the participants in this task.
No statistically significant differences were found, but the practice conditions reached a plateau in performance after block 1, except for the adjusted BW group, which showed improved performance after block 1. In previous studies on motor learning using the grasping force control task, the total number of practice trials was between 30 and 60 trials (Liu et al., Citation2021; Tarnas et al., Citation2020). Therefore, the small amount of practice trials suggests that differences in practice conditions did not affect performance or motor learning.
In contrast, the three BW groups showed significant improvements of the VE from the pretest to the delayed-retention test. Furthermore, the adjusted BW group displayed a greater decrease in VE than the wide BW group in the immediate-retention test. Our study applied BW KR to manipulate the amount of visual information. The tolerance of the BW on the adjusted BW group was larger than the wide BW group in block 1, while it was comparable to the narrow BW group in block 3 (see, Figure ). The adjusted BW groups received moderate error information, as the tolerance of the BW changed with improving performance consistency. However, the wide BW group displayed increased VE over the adjusted BW group because the tolerance of BW did not vary with the change in individual’s performance, and required error information converged within the BW. We adjusted the tolerance of BW based on the learner’s task performance, which may have resulted in a closer approach to the learner’s optimal challenge point. Consequently, the adjusted BW group was better than the wide BW group in terms of performance consistency. However, the results of the sum of ACEs outside of the BW indicated that the adjusted BW group and the wide BW group received the same amount of error information. It is suggested that adjusting the amount of error information in response to changes in the individual’s skill, rather than the total amount of error information given to the learners during practice trial, is important for reducing VE. The control group did not show improvement in performance consistency from the pretest to the delayed-retention test. As described in the guidance hypothesis (Lee et al., Citation1990), the control group received a large amount of error information during all practice trials. Therefore, the control group was dependent on KR and had insufficient information about intrinsic criteria regarding AGF.
Our study has several limitations. First, the sample size was small. We performed a priori power analysis to determine the sample size. However, Lohse et al. (Citation2016) reported that 11 participants per group were inadequate for a motor learning study. Our study recruited approximately 12 participants per group, which was probably insufficient compared with previous studies. Second, in this study, the practice time provided was small. The practice sessions consisted of 15 trials of 60 seconds each. Shea et al. (Citation1990) investigated the relationship between skill level and the amount of practice in the force control task and reported that a performance improvement would require about 150 to 200 trials. Thus, with sufficient practice, adjusted BW may be more effective than other practice conditions in enhancing performance and facilitating learning. Third, we did not measure psychological, neurological, or physiological outcomes to evaluate performance or learning. In their OPTIMAL theory, Wulf and Lewthwaite (Citation2016) found that psychological factors such as intrinsic motivation influence the improvement of performance and the enhancement of learning. In addition, Krakauer et al. (Citation2019) found that learning involves motivation and many brain regions (e.g., basal ganglia, cerebellum). In future studies, we will adjust the amount of practice in the grasping force control task, employ a large sample size, and measure psychological, neurological, and/or physiological outcomes of the learners.
4.1. Conclusion
This study aimed to clarify the effectiveness of adjusted BW KR, which sets BW based on an individual’s performance accuracy in grasping force tasks. The adjusted BW KR provides KR according to the change in an individual’s skill. Our study results partially suggest the effectiveness of the adjusted BW KR compared to the traditional BW used in previous studies.
Disclosure statement
No potential conflict of interest was reported by the author(s).
Data availability statement
The raw data included in the manuscript can be accessed from the corresponding author Jun Yabuki upon request through email: [email protected]
Additional information
Funding
Notes on contributors
Jun Yabuki
Jun Yabuki (Ph.D. Ibaraki Prefectural University of Health Sciences) is a physical therapist in Ibaraki Prefectural University of Health Sciences Hospital. His research interests include motor control, motor learning and rehabilitation.
Kazunori Akizuki
Kazunori Akizuki (Ph.D. Ibaraki Prefectural University of Health Sciences) is a Senior Assistant Professor at Mejiro University. His research interests include motor learning, motor control and rehabilitation.
Ryohei Yamamoto
Ryohei Yamamoto (Ph.D. Ibaraki Prefectural University of Health Sciences) is an Associate Professor at Kumamoto Health Science University. His research interests include motor learning, motor control and rehabilitation.
Kazuto Yamaguchi
Kazuto Yamaguchi (Ph.D. Ibaraki Prefectural University of Health Sciences) is an Assistant Professor at Nihon Institute of Medical Science. His research interests include motor learning, motor control, and rehabilitation.
Yukari Ohashi
Yukari Ohashi (Ph.D. Tokyo Medical University) is an Emeritus Professor at Ibaraki Prefectural University of Health Sciences. Her research interests include experimental psychology, motor control, motor learning and rehabilitation.
References
- Agethen, M., & Krause, D. (2016). Effects of bandwidth feedback on the automatization of an arm movement sequence. Human Movement Science, 45, 71–11. https://doi.org/10.1016/j.humov.2015.11.005
- APA Dictionary of Psychology. (n.d.). Retrieved March 12, 2022, from https://dictionary.apa.org/
- Badets, A., & Blandin, Y. (2005). Observational learning: effects of bandwidth knowledge of results. Journal of Motor Behavior, 37(3), 211–216. https://doi.org/10.3200/JMBR.37.3.211-216
- Badets, A., & Blandin, Y. (2010). Feedback schedules for motor-skill learning: the similarities and differences between physical and observational practice. Journal of Motor Behavior, 42(4), 257–268. https://doi.org/10.1080/00222895.2010.497512
- Butler, M. S., Reeve, T. G., & Fischman, M. G. (1996). Effects of the instructional set in the bandwidth feedback paradigm on motor skill acquisition. Research Quarterly for Exercise and Sport, 67(3), 355–359. https://doi.org/10.1080/02701367.1996.10607964
- Cauraugh, J. H., Chen, D., & Radio, S. J. (1993). Effects of traditional and reversed bandwidth knowledge of results on motor learning. Research Quarterly for Exercise and Sport, 64(4), 413–417. https://doi.org/10.1080/02701367.1993.10607594
- Coca-Ugrinowitsch, A. A., Benda, R. N., Aburachid, L. M., De Andrade, A. G. P., Greco, P. J., Menzel, H.-J. K., & Ugrinowitsch, H. (2014). Bandwidth knowledge of results on the learning of the saloon dart throwing task. Perceptual and Motor Skills, 118(2), 462–474. https://doi.org/10.2466/25.23.PMS.118k17w9.
- Goodwin, J. E., & Meeuwsen, H. J. (1995). Using bandwidth knowledge of results to alter relative frequencies during motor skill acquisition. Research Quarterly for Exercise and Sport, 66(2), 99–104. https://doi.org/10.1080/02701367.1995.10762217
- Guadagnoli, M. A., & Lee, T. D. (2004). Challenge point: a framework for conceptualizing the effects of various practice conditions in motor learning. Journal of Motor Behavior, 36(2), 212–224. https://doi.org/10.3200/JMBR.36.2.212-224
- Ishikura, T. (2008). Reduced relative frequency of knowledge of results without visual feedback in learning a golf-putting task. Perceptual and Motor Skills, 106(1), 225–233. https://doi.org/10.2466/pms.106.1.225-233
- Ishikura, T. (2011). The use of knowledge of results based on the stability of performance during acquisition of timing skill: a validity study. Perceptual and Motor Skills, 112(1), 122–132. https://doi.org/10.2466/22.25.PMS.112.1.122-132
- Kaneno, T., Sato, A., Akizuki, K., Yamaguchi, A., Yasaki, K., & Morita, Y. (2017). Assessing the adjustability of grasping force using the iwakka in elderly individuals. Journal of Physical Therapy Science, 29(12), 2215–2219. https://doi.org/10.1589/jpts.29.2215
- Kern, D. S., Semmler, J. G., & Enoka, R. M. (2001). Long-term activity in upper- and lower-limb muscles of humans. Journal of Applied Physiology, 91(5), 2224–2232. https://doi.org/10.1152/jappl.2001.91.5.2224.
- Krakauer, J. W., Hadjiosif, A. M., Xu, J., Wong, A. L., & Haith, A. M. (2019). Motor Learning. In R. Terjung (Ed.), Comprehensive Physiology (1st ed ed., pp. 613–663). Wiley. https://doi.org/10.1002/cphy.c170043
- Lai, Q., Shea, C. H., Wulf, G., & Wright, D. L. (2000). Optimizing generalized motor program and parameter learning. Research Quarterly for Exercise and Sport, 71(1), 10–24. https://doi.org/10.1080/02701367.2000.10608876
- Lauber, B., & Keller, M. (2014). Improving motor performance: selected aspects of augmented feedback in exercise and health. European Journal of Sport Science, 14(1), 36–43. https://doi.org/10.1080/17461391.2012.725104
- Lee, T. D., & Maraj, B. K. V. (1994). Effects of bandwidth goals and bandwidth knowledge of results on motor learning. Research Quarterly for Exercise and Sport, 65(3), 244–249. https://doi.org/10.1080/02701367.1994.10607625
- Lee, T. D., White, M. A., & Carnahan, H. (1990). On the role of knowledge of results in motor learning: exploring the guidance hypothesis. Journal of Motor Behavior, 22(2), 191–208. https://doi.org/10.1080/00222895.1990.10735510
- Liu, M., Wilder, S., Sanford, S., Saleh, S., Harel, N. Y., & Nataraj, R. (2021). Training with agency-inspired feedback from an instrumented glove to improve functional grasp performance. Sensors, 21(4), 1173. https://doi.org/10.3390/s21041173
- Lohse, K., Buchanan, T., & Miller, M. (2016). Underpowered and overworked: problems with data analysis in motor learning studies. Journal of Motor Learning and Development, 4(1), 37–58. https://doi.org/10.1123/jmld.2015-0010
- Salmoni, A. W., Schmidt, R. A., & Walter, C. B. (1984). Knowledge of results and motor learning: a review and critical reappraisal. Psychological Bulletin, 95(3), 355–386. https://doi.org/10.1037/0033-2909.95.3.355.
- Schmidt, R. A. (1988). Motor control and learning: A behavioral emphasis (2nd ed.). Human Kinetics Publishers.
- Shea, C. H., Kohl, R., & Indermill, C. (1990). Contextual interference: contributions of practice. Acta Psychologica, 73(2), 145–157. https://doi.org/10.1016/0001-6918(90)
- Sherwood, D. E. (1988). Effect of bandwidth knowledge of results on movement consistency. Perceptual and Motor Skills, 66(2), 535–542. https://doi.org/10.2466/pms.1988.66.2.535
- Sigrist, R., Rauter, G., Riener, R., & Wolf, P. (2013). Augmented visual, auditory, haptic, and multimodal feedback in motor learning: A review. Psychonomic Bulletin and Review, 20(1), 21–53. https://doi.org/10.3758/s13423-012-0333-8.
- Smith, P. J. K., Taylor, S. J., & Withers, K. (1997). Applying bandwidth feedback scheduling to a golf shot. Research Quarterly for Exercise and Sport, 68(3), 215–221. https://doi.org/10.1080/02701367.1997.10608000
- Tarnas, J., Stemplewski, R., & Krutki, P. (2020). Sex differences in maintaining the requested handgrip force enhanced by auditory or visual feedback. International Journal of Environmental Research and Public Health, 17(14), 5158. https://doi.org/10.3390/ijerph17145158
- Ugrinowitsch, H., Ugrinowitsch, A. A. C., Benda, R. N., & Tertuliano, I. W. (2010). Effect of bandwidth knowledge of results on the learning of a grip force control task. Perceptual and Motor Skills, 111(3), 643–652. https://doi.org/10.2466/23.25.PMS.111.6.643-652
- Winstein, C. J. (1991). Knowledge of results and motor learning—implications for physical therapy. Physical Therapy, 71(2), 140–149. https://doi.org/10.1093/ptj/71.2.140
- Wulf, G., & Lewthwaite, R. (2016). Optimizing performance through intrinsic motivation and attention for learning: the optimal theory of motor learning. Psychonomic Bulletin & Review, 23(5), 1382–1414. https://doi.org/10.3758/s13423-015-0999-9
- Yamamoto, R., Akizuki, K., Kanai, Y., Nakano, W., Kobayashi, Y., & Ohashi, Y. (2019). Differences in skill level influence the effects of visual feedback on motor learning. Journal of Physical Therapy Science, 31(11), 939–945. https://doi.org/10.1589/jpts.31.939
- Yamamoto, R., Akizuki, K., Yamaguchi, K., Yabuki, J., & Kaneno, T. (2022). A study on how concurrent visual feedback affects motor learning of adjustability of grasping force in younger and older adults. Scientific Reports, 12(1), 10755. https://doi.org/10.1038/s41598-022-14975-4
- Yamamoto, R., & Ohashi, Y. (2014). The effects of inaccessible visual feedback used concurrently or terminally. Journal of Physical Therapy Science, 26(5), 731–735. https://doi.org/10.1589/jpts.26.731.