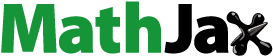
Abstract
The objective of this paper is to compare the overall performance of two turbulence models used for the simulation of fire scenarios in ventilated tunnels. Two Reynolds Averaged Navier–Stokes turbulence models were used; the low-Re k–ω SST and the standard k–ε model with wall functions treatment. Comparison was conducted on two different fire scenarios. The varied parameters were the heat release rate and the ventilation rate. Results predicted by the two turbulence models were also compared to the results produced from the commercial package Ansys Fluent. Quite faster simulations were performed using the k–ε turbulence model with wall functions and our findings, as to the basic characteristics of smoke movement, were in good agreement with Ansys Fluent ones.
Public Interest Statement
Much attention has been paid on tunnel fires because of their negative consequences in human lives, repair cost, and cost due to the stop of tunnels operation. The methods used for the simulation of a tunnel fire are experimental, theoretical, and computational. Computational simulations cost less than experimental ones and they are more accurate compared to theoretical approaches.
Modeling of turbulence is a serious aspect of a CFD solver. The objective of this paper is the comparison of the overall performance of two turbulence models in two widely studied fire scenarios in a tunnel. Results predicted are also compared to the results of the general purpose commercial package Ansys Fluent. Both turbulence models produced accurate results and in good agreement to those of Ansys Fluent. Greater temperatures downstream from the heat source were calculated compared to experimental results, showing the importance of radiation and wall heat conduction modeling.
1. Introduction
Much attention has been paid on tunnel fires because of their negative consequences. A case of a fire accident in a tunnel constitutes an extremely dangerous situation for people who are inside it, as hot toxic gases are produced. In addition to this, the repair cost and the cost due to the closing of tunnels operation are often huge.
The methods used for the simulation of a tunnel fire are experimental, theoretical, and computational. Full-scale experimental investigation is often prohibitive due to the necessary high cost, but it provides large amounts of reliable data. Many full-scale experiments for the Memorial tunnel are reported in the Memorial tunnel fire ventilation test program-test report (Massachusetts Highway Department, Citation1995). Small-scale experiments cost less and require careful choice of the scaling factors between the prototype and small scale (Lee & Ryou, Citation2005, Citation2006). Analytical methods and Computational Fluid Dynamics (CFD) simulations are the most affordable and permit the investigation of various alternative cases with proper modifications on the model. CFD is the most accurate between them, but a realistic CFD simulation of a fire scenario requires fluid dynamics, turbulence, radiation, wall conduction, and combustion numerical modeling.
Plenty CFD simulations of tunnel fires could be found in the literature, using commercial, open source and fewer of them research codes. When CFD simulation is applied, the selection of the appropriate turbulence model constitutes a serious aspect. Abanto, Reggio, Barrero, and Petro (Citation2006) used Fluent and a research code to study smoke movement in a case of fire in an underwater tunnel. Turbulence was modeled with the standard k–ε model. Hui, Li, and Lixin (Citation2009) have modeled the longitudinal ventilation of the 4th Beijing subway line using CFX and have compared their results for the critical velocity to previous formulations proposed. They preferred the standard k–ε model rather than LES because of computer load reasons. Lee and Ryou (Citation2006) have studied the aspect ratio effect on smoke movement using Fire Dynamics Simulator (FDS) of the National Institute of Standards and Technology and compared their results to reduced-scale experimental ones. Turbulence was modeled using LES model. Hu, Peng, and Huo (Citation2008) have studied the effect of the place of the fire in the critical velocity using FDS and LES turbulence model. Hu, Huo, Wang, and Yang (Citation2007) have compared FDS with LES turbulence model results to full-scale experimental data with promising conclusions for the validity of FDS solver. Wu and Bakar (Citation2000) applied an experimental and CFD investigation on the critical velocity formulations. For the CFD simulation, Fluent was used with the standard k–ε turbulence model.
The most dangerous factor for human lives in tunnel fires is the excess of the concentration limits of the combustion products and not the extreme temperatures. Therefore, the appropriate ventilation system is required for the smoke control. One of the most important aims of a numerical model is the accurate prediction of the back-layering length and critical velocity produced by the current ventilation system. The distance of the smoke front from the heat source is the back-layering length. The critical velocity refers to longitudinal ventilation systems and is the lowest ventilation velocity that could prevent smoke back-layering. Longitudinal ventilation systems drive smoke to tunnel exit, ensuring the safe escape of passengers through the tunnel entrance and/or emergency exits. The ventilation system should prevent back-layering, but high ventilation velocities feed the fire with more oxygen, augmenting the heat release rate (Chow, Citation1998) and increase the resistance to the passengers, reducing the escaping rate (Hui et al., Citation2009). For the calculation of the critical velocity, mostly, Froude number-based analytical formulae have been used. Despite their simplicity, they do not account for some specific characteristics of each tunnel such as the existence of lateral evacuation hallways (Banjac & Nikolic, Citation2008), or the conditions of each fire scenario such as the place where accident happened or the obstructions that may exist. It was found that obstructions affect significantly the critical velocity (Kang, Citation2006; Oka & Atkinson, Citation1995). In case of not taking into account all these facts, huge investment costs for the ventilation system may be produced, or inadequate safety measures may be adopted. This fact makes CFD simulation the ideal method for the design of ventilation systems.
Our purpose is the comparison of the overall performance of the low-Re k–ω SST and the standard k–ε with wall functions treatment turbulence models. The developed numerical solver is based on the incompressible Navier–Stokes and energy equations. Buoyancy force, due to temperature differences, was approximated by means of Boussinesq approximation. For both turbulence models, the necessary buoyancy source terms are included. Our results are also compared to those produced by the commercial package Ansys Fluent, using the standard k–ε turbulence model with wall functions under the same simplifications.
2. Mathematical model and numerical procedure
2.1. Test case and governing equations
The test case presented below is a widely studied one. Apte, Green, and Kent (Citation1991) have carried out the experimental investigation. Fletcher, Kent, Apte, and Green (Citation1994) have also presented experimental results and a numerical investigation using a steady-state approach and k–ε model with wall functions. Gao, Liu, Chow, and Fong (Citation2004) have presented a numerical investigation using an unsteady approach and an LES turbulence model. Miloua, Azzi, and Wang (Citation2011) also numerically studied this test case using FDS for the comparison of combustion models and wall boundary conditions, using LES turbulence model.
The tunnel geometry is described in Figure . A pool fire exists at 59.5 m from the entrance of the tunnel. The pool fire was assumed to be a cubic volumetric heat source, with the heat release rate being constant and having its maximum value from the beginning till the end of the simulation. The flow field in all cases tested was regarded as incompressible, because Mach number remained below 0.3. For the buoyancy forces, as a result of the temperature differences, Boussinesq approximation was adopted. Moreover, the viscous dissipation term in the energy equation was neglected, because the thermal energy due to viscous shear at low velocities is small (Gao et al., Citation2004). For the closure of the Reynolds Averaged Navier–Stokes (RANS) mean flow equations, two alternative turbulence models were used and compared; the low-Re k–ω SST and the less computationally demanding standard k–ε with wall functions treatment.
The general form of the conservation equations is:(1)
(1)
The conserved quantity “ϕ”, the diffusion coefficient “Γϕ” and the source term “Sϕ” for each one of the conservation equations are given in Table in tensorial notations.
Table 1. Coefficients of Equation 1 and constants values
The flow variables are the pressure “p,” the Cartesian velocity components “u,” “v,” and “w” in the “x,” “y,” and “z” directions, respectively, and the temperature “T.” Turbulence modeling inserts two additional dependent variables. The kinetic energy of turbulence “k” and turbulence dissipation “ε” when standard k–ε model is used and the turbulence kinetic energy “k” and specific dissipation rate “ω” when k–ω SST is used. The term “gβΤΔΤ” in the “z” direction momentum equation is the buoyancy force term, where “g” is the gravitational acceleration, “βΤ” is the thermal expansion coefficient, and “ΔΤ” is the subtraction of the ambient temperature Το (which is equal to 26°C) from the local temperature. “ρ” is the fluid density at the ambient temperature and “Cp” is the specific heat at constant pressure. “” is the heat release rate per unit volume. Effective kinematic viscosity “νeff” is the sum of the molecular kinematic viscosity “ν” and the turbulence kinematic viscosity “νt”. Turbulence kinematic viscosity is calculated by νt = cμk2/ɛ when standard k–ε is used and
when k–ω SST is used. For more information on the k–ω SST turbulence model readers are referred to (Menter, Citation1993). “Pr” is the Prandtl number and “Prt” is the turbulence Prandtl number for temperature. “σk”, “σε”, and “σω” are turbulence model constants (Barakos, Mitsoulis, & Assimacopoulos, Citation1994; Menter, Citation1993). Terms “Pk” and “Gk” correspond to shear and buoyancy production rates of the turbulence kinetic energy respectively. The inclusion of the “Gk” term in the turbulence conservative equations is of vital importance, since without this extremely false back-layering lengths may be calculated (Fletcher et al., Citation1994). “CDω” is the cross-diffusion term (Menter, Citation1993).
2.2. Boundary and initial conditions
Two different test cases were simulated, varying in the total heat release rate of the heat source and the ventilation rate. The total heat release rate and the ventilation velocity for these two cases are given in Table .
Table 2. Cases data and calculated results
At the inlet of the tunnel uniform velocity profile was prescribed, with the “u” velocity being equal to the ventilation velocity while “v” and “w” velocities were equal to zero (v = w = 0). At the outlet of the tunnel, pressure was prescribed and set equal to the ambient pressure. At the tunnel walls no-slip boundary conditions were applied and they were assumed to be adiabatic, which corresponds to the worst situation. When low-Re k–ω SST turbulence model was applied, turbulence variables at the walls were(2)
(2)
where “Δy” is the distance to the next point away from the wall. When high-Re k–ε turbulence model was applied, appropriate wall functions were used leading to coarser numerical meshes and less time demanding calculations. The values for “u”, “v”, “w”, “T”, “k”, and “ε” were calculated using the following wall functions.(3)
(3)
(4a)
(4a)
(4b)
(4b)
(5)
(5)
(6)
(6)
(7)
(7)
(8)
(8)
(9)
(9)
(10)
(10)
(11)
(11)
For y+ ≤ 11.6 the first grid point is in the viscous sub-layer and for y+ > 11.6 in the logarithmic region. It is desirable that the first grid point belongs to the logarithmic region of the boundary layer, where the viscous effects are weaker compared to the turbulent ones (Launder & Spalding, Citation1974). For the temperature near the wall, the formulation proposed by Kader was used (Kader, Citation1981), which is valid in all zones of the boundary layer and for a large range of Prandtl numbers.
As initial condition, the converged to steady-state flow field of the isothermal case (without the heat source) was used. The temperature was equal to the ambient temperature.
2.3. Numerical methodology
The solution procedure of the mean flow equations has been analyzed in details by Stokos, Vrahliotis, Pappou, and Tsangaris (Citation2012, Citation2013) and Vrahliotis, Pappou, and Tsangaris (Citation2012). A node centered finite volume discretization technique has been developed for hybrid numerical meshes. The solution of the incompressible flow equations was based on the artificial compressibility method. A Roe’s approximate Riemann solver was used for the evaluation of the convective fluxes. For the discretization of the viscous fluxes a central scheme was used. For the evaluation of the convective fluxes of the turbulence equations a first-order upwind scheme was applied, as proposed by Menter (Citation1993). For the calculation of turbulence equations viscous fluxes, the same method used for the mean flow equations was applied. For the temporal discretization, a dual-time stepping approach was adopted. Specifically, an implicit second-order backward difference scheme was implemented for the physical-time marching and an implicit first-order scheme for the pseudo-time marching. To pass from one physical time step to another convergence in pseudo-time needed to be achieved. The mean flow and turbulence equations were loosely coupled. The mean flow equations were first solved with the turbulence kinematic viscosity fixed. Then, the turbulence equations were solved with the velocity and temperature fields fixed. The resulting non-linear algebraic system of equations was linearized using Newton’s method. The arising linear system was solved using the Jacobi iterative method with suitable under-relaxation. Parallel processing was used for the acceleration of the computations.
2.4. Numerical mesh
The computing domain was composed of 481,950 nodes and 449,000 hexahedrons when standard k–ε turbulence model with wall functions was used (Figure ). The first layer thickness was approximately equal to 0.01. When low-Re k–ω SST was used the computing mesh was composed of 1,094,252 nodes and 1,075,400 hexahedrons (Figure ). The first layer thickness was of the order of 10−5 so that the y+ < 2 condition is fulfilled and the growing factor was equal to 1.2. Both numerical meshes were denser near the heat source. The physical time step was set equal to 0.01 s.
3. Results and discussion
3.1. Transient results
When no concentrations of smoke are calculated and radiative heat transfer is not considered, it could be assumed that smoke movement is analogous to the temperature field. In Figures and , the evolution of the flame and temperature field for cases 1 and 2, respectively, for the first 10 s are presented. It is obvious that the predicted smoke movement through time by the two turbulence models is similar.
Figure 4. Temperature isolines through time for case 1.
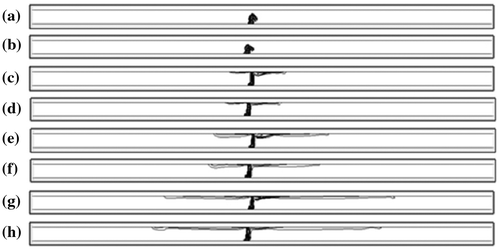
Figure 5. Temperature isolines through time for case 2.
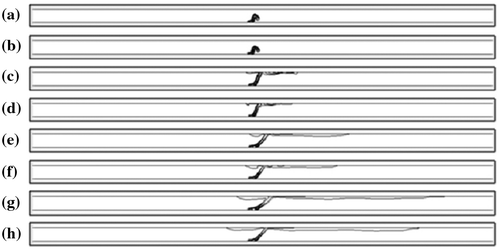
In a tunnel fire case surrounding medium (air and smoke) in the vicinity of the heat source is heated and raises up till the ceiling of the tunnel. Then having reached the ceiling it moves to the side walls and along the ceiling to the tunnel exit and the tunnel inlet forming the back-layering length. Reaching the side walls, smoke moves downward to the ground. In Figures and velocity vectors predicted by both turbulence models are given at characteristic sections and moments. Velocity vectors reveal smoke movement. It is obvious that smoke requires less than 1 s to reach the ceiling and less than 5 s to reach the side walls for both cases. Similar flow patterns were predicted by the turbulence models.
Figure 6. Velocity vectors at characteristic sections and moments for case 1 predicted by the standard k–ε model (left column) and the low-Re k–ω SST model (right column).
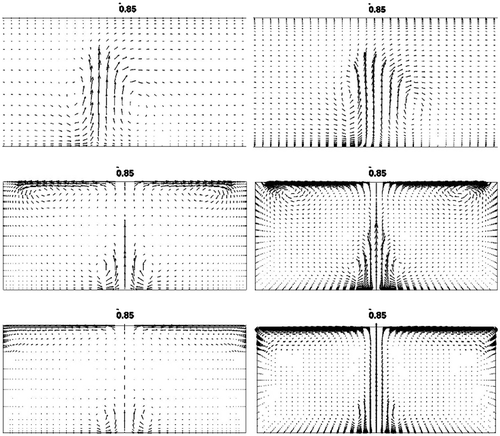
Figure 7. Velocity vectors at characteristic sections and moments for case 2 predicted by the standard k–ε model (left column) and the low-Re k–ω SST model (right column).
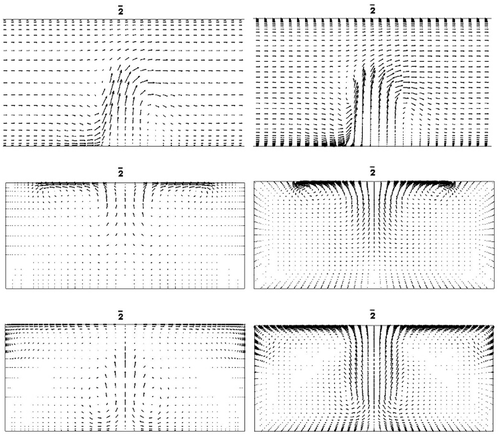
In Figure , we present the predicted using both turbulence models temperature vertical profiles at 18 and 30 m downstream from the heat source for case 2, 20 s after fire breaking. Small differences (less than 6%) are observed for the vertical temperature profiles.
Figure 8. Case 2, 20 s after fire breaking.
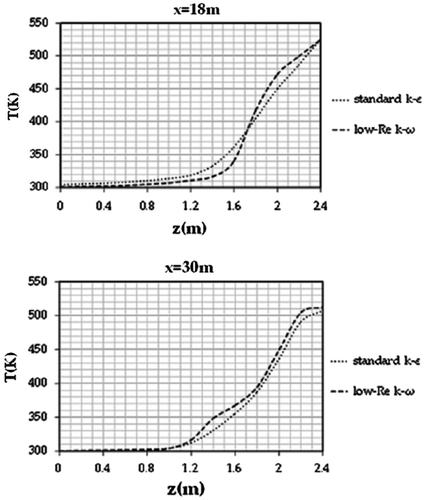
In Figure , we present for case 2 the convergence history for the temperature corrections in pseudo-time for three physical time steps, using the standard k–ε turbulence model. The starting point is t = 2 s. It is obvious that less than 250 pseudo-time steps are required for each physical time step. However, it should be noted that the first 2 s are the most numerically crucial and more pseudo-time steps are required for pseudo-time convergence compared to the rest of the simulation. Same smooth convergence occurred and for the other dependent variables.
3.2. Steady state results
Gao et al. (Citation2004) claim that flame shape is defined by the maximum temperature gradients. In the literature, many definitions for the flame angle have been found (Anderson et al., Citation2006). In Figures and , flame shapes and the definition used for the calculation of the flame angle are given. Flame angle βt is defined by the vertical line passing through the core of the heat source and the line connecting the core of the heat source with the upper point of the flame. In the same figures, temperature fields in the vicinity of the heat source are compared to those predicted by Ansys Fluent. The greatest temperature values, flame shapes, flame angles and back-layering lengths seem to agree satisfactorily. Flame angles and back-layering lengths predicted by our solver and Ansys Fluent are given in Table . It is evident that the backflow is less and flame tilt greater for higher ventilation velocities. The time needed for the smoke front to reach the steady-state back-layering length was about 50 and 15 s for case 1 and case 2, respectively.
Figure 10. Flame shape comparison for case 1.
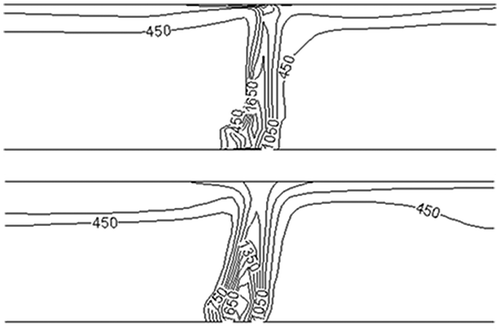
Figure 11. Flame shape comparison for case 2.
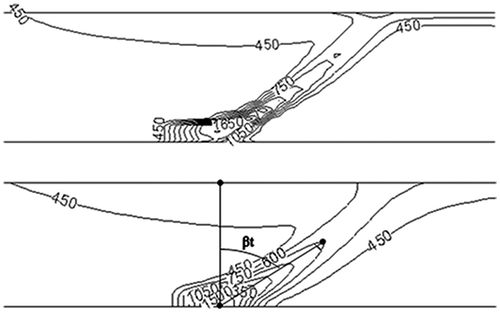
Radiation plays a significant role in a case of fire in a tunnel. The fraction of the heat release from a heat source in a tunnel fire in form of radiation is in the order of 20–50% (Bettelini, Citation2001; Hostikka, Citation2008; Grant, Jagger, & Lea, Citation1998). However, the solution of the radiative heat transfer equation is time consuming because a great number of radiation intensities have to be calculated at each computational node. More than 100 solid angles are utilized for each node at a finite volume-based method. Consequently, heat transfer due to radiation is often not taken into account, or the assumed amount of radiation loss is subtracted from the heat release rate (Kang, Citation2006). In Figure , temperature profiles are given 18 and 40 m downstream of the heat source. Greater values for the temperature are computed compared to the experimental values of Fletcher et al. (Citation1994). This discrepancy is attributed to the omission of radiation modeling, according to the aforementioned role of radiation, and the omission of heat conduction inside tunnel wall. Similar discrepancies were calculated by Gao et al. (Citation2004) who applied the same simplifications. However, the curves calculated are of the same “s” form. Temperature increases with increasing height and reaches its maximum values inside the smoke plume. Both turbulence models calculated approximate temperature profiles 18 and 40 m downstream from the heat source. Experimental investigation also showed approximate temperature profiles 18 and 40 m from the heat source.
Figure 12. Comparison of temperature profiles along height when steady state was reached.
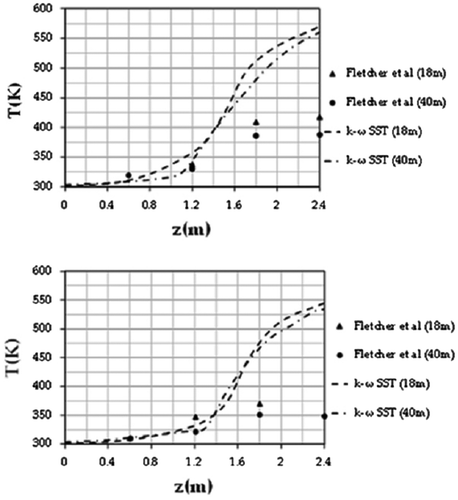
Velocity vectors for case 2 with the standard k–ε model when steady state was achieved are presented in Figure . The stagnation point which defines the back-layering length is marked with a red circle.
Finally, as far as computation time is concerned, a speedup approximately equal to 2.5, at each pseudo-time step, was gained when k–ε turbulence model with wall functions was used compared to the low-Re k–ω SST turbulence model. This fact is mostly a result of the less dense grid required from the k–ε turbulence model due to the use of wall functions.
4. Conclusions
The performance of the standard k–ε with wall functions and the low-Re k–ω SST turbulence models was compared in terms of accuracy, stability, and calculation time. Flow pattern and temperature fields were in good agreement. Our results were also compared to the results produced by the commercial general purpose code Ansys Fluent. Back-layering lengths and flame angles for both cases agreed satisfactorily with Ansys Fluent ones. However, the omission of radiation and wall conduction modeling led to higher temperatures compared to experimental results. Simulations including both of these models will be presented in a future article with improved calculated temperature fields.
Acknowledgments
Simulations were performed on the high-performance platform “VELOS” of the Laboratory of Thermal Turbomachines, Parallel CFD and Optimization Unit, School of Mechanical Engineering of the National Technical University of Athens.
Additional information
Funding
Notes on contributors
Konstantinos G. Stokos
Our group’s research and working areas relate to the development and application of software for computational mechanics. Specifically, the development and application for heat transfer problems (conduction, convection, and radiation), structural problems, aerodynamics, fluid–structure interaction, biofluid mechanics, numerical mesh generation, and optimization constitutes our field. Our team is involved in several transnational research projects in collaboration with organizations and companies. Many papers of our work have been published in scientific journals and international conferences.
References
- Abanto, J., Reggio, M., Barrero, D., & Petro, E. (2006). Prediction of fire and smoke propagation in an underwater tunnel. Tunnelling and Underground Space Technology, 22, 90–95.
- Anderson, W., Pastor, E., Butler, B., Catchpole, E., Dupuy, J. L., Fernandes, P., … Ventura, J. (2006, November 27–30). Evaluating models to estimate flame characteristics for free-burning fires using laboratory and field data. In 5th International Conference on Forest Fire Research. Coimbra, Portugal.
- Apte, V. B., Green, A. R., & Kent, J. H. (1991). Pool fire plume flow in a large-scale wind tunnel. Fire Safety Science, 3, 425–434.10.3801/IAFSS.FSS.3-425
- Banjac, M., & Nikolic, B. (2008). Numerical study of smoke flow control in tunnel fires using ventilation systems. FME Transactions, 36, 145–150.
- Barakos, G., Mitsoulis, E., & Assimacopoulos, D. (1994). Natural convection flow in a square cavity revisited: Laminar and turbulent models with wall functions. International Journal for Numerical Methods in Fluids, 18, 695–719.10.1002/(ISSN)1097-0363
- Bettelini, M. (2001, September 17–18). CFD for tunnel safety. In Fluent users’ meeting. Bingen.
- Chow, W. K. (1998). On smoke control for tunnels by longitudinal ventilation. Tunnelling and Underground Space Technology, 13, 271–275.10.1016/S0886-7798(98)00061-3
- Fletcher, D. F., Kent, J. H., Apte, V. B., & Green, A. R. (1994). Numerical simulations of smoke movement from a pool fire in a ventilated tunnel. Fire Safety Journal, 23, 305–325.10.1016/0379-7112(94)90033-7
- Gao, P. Z., Liu, S. L., Chow, W. K., & Fong, N. K. (2004). Large eddy simulations for studying tunnel smoke ventilation. Tunnelling and Underground Space Technology, 19, 577–586.10.1016/j.tust.2004.01.005
- Grant, G. B., Jagger, S. F., & Lea, C. J. (1998). Fires in tunnels. Philosophical Transactions: Mathematical, Physical and Engineering Sciences, 356, 2873–2906.
- Hostikka, S. (2008). Development of fire simulation models for radiative heat transfer and probabilistic risk assessment ( PhD Thesis). VTT Technical Research Centre of Finland, Espoo. ISBN 978-951-38-7099-7.
- Hu, L. H., Huo, R., Wang, H. B., & Yang, R. X. (2007). Experimental and numerical studies on longitudinal smoke temperature distribution upstream and downstream from the fire in a road tunnel. Journal of Fire Sciences, 25, 23–43.10.1177/0734904107062357
- Hu, L. H., Peng, W., & Huo, R. (2008). Critical wind velocity for arresting upwind gas and smoke dispersion induced by near-wall fire in a road tunnel. Journal of Hazardous Materials, 150, 68–75.10.1016/j.jhazmat.2007.04.094
- Hui, Y., Li, J., & Lixin, Y. (2009). Numerical analysis of tunnel thermal plume control using longitudinal ventilation. Fire Safety Journal, 44, 1067–1077.10.1016/j.firesaf.2009.07.006
- Kader, B. A. (1981). Temperature and concentration profiles in fully turbulent boundary layers. International Journal of Heat and Mass Transfer, 24, 1541–1544.10.1016/0017-9310(81)90220-9
- Kang, K. (2006). Computational study of longitudinal ventilation control during an enclosure fire within a tunnel. Journal of Fire Protection Engineering, 16, 159–181.10.1177/1042391506056737
- Launder, B. E., & Spalding, D. B. (1974). The numerical computation of turbulent flows. Computer Methods in Applied Mechanics and Engineering, 3, 269–289.10.1016/0045-7825(74)90029-2
- Lee, S. R., & Ryou, H. S. (2005). An experimental study of the effect of the aspect ratio on the critical velocity in longitudinal ventilation tunnel fires. Journal of Fire Sciences, 23, 119–138.10.1177/0734904105044630
- Lee, S. R., & Ryou, H. S. (2006). A numerical study on smoke movement in longitudinal ventilation tunnel fires for different aspect ratio. Building and Environment, 41, 719–725.10.1016/j.buildenv.2005.03.010
- Massachusetts Highway Department. (1995). Memorial tunnel fire ventilation test program comprehensive test report.
- Menter, F. R. (1993, July 6–9). Zonal two equation k-ω turbulence models for aerodynamic flows. In AIAA 24th Fluid Dynamics Conference. Orlando, FL, AIAA 93-2906.
- Miloua, H., Azzi, A., & Wang, H. Y. (2011). Evaluation of different numerical approaches for a ventilated tunnel fire. Journal of Fire Sciences, 29, 403–429.10.1177/0734904111400976
- Oka, Y., & Atkinson, G. T. (1995). Control of smoke flow in tunnel fires. Fire Safety Journal, 25, 305–322.10.1016/0379-7112(96)00007-0
- Stokos, K. G., Vrahliotis, S. I., Pappou, Th. I., & Tsangaris, S. (2012, July 4–7). Development and validation of a 3-D Navier–Stokes solver including heat transfer and natural convection. In Proceedings of 5th International Conference from Scientific Computing to Computational Engineering, 5th IC-SCCE. Athens Greece.
- Stokos, K. G., Vrahliotis, S. I., Pappou, Th. I., & Tsangaris, S. (2013, May 25–27). Development and validation of a Navier–Stokes solver including heat transfer and mixed convection. In Proceedings of 10th International Congress on Mechanics, 10th HSTAM. Chania, Crete, Greece.
- Vrahliotis, S., Pappou, Th, & Tsangaris, S. (2012). Artificial compressibility 3-D Navier–Stokes solver for unsteady incompressible flows with hybrid grids. Engineering Applications of Computational Fluid Mechanics, 6, 248–270.10.1080/19942060.2012.11015419
- Wu, Y., & Bakar, M. Z. A. (2000). Control of smoke flow in tunnel fires using longitudinal ventilation systems—A study of the critical velocity. Fire Safety Journal, 35, 363–390.10.1016/S0379-7112(00)00031-X