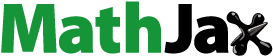
Abstract
Road traffic accidents (RTA) have been a very big problem in many developing countries including Nigeria, causing many deaths and disabilities. The aim of this research is to model the effects of intervention measures adopted by the Nigerian government in curbing RTA. In the research road traffic accident data from 1960 to 2014 were analyzed using Box-Jenkins intervention methodology. The result of the modeling and analysis showed that the establishment of Federal Road Safety Commission in 1987, a Nigerian Government intervention measure, had an abrupt temporary impact on RTA in Nigeria (ω0 = −2,423). The findings also showed that the total number of accident cases in Nigeria from 1961 to 1987 (26 years), the period before the intervention, was 657,280 while the total number of accident cases from 1988 to 2014 (26 years), the period after the intervention, was 430,721. This represents a 34.5% reduction in total accident cases after the intervention. In terms of accident density there was a 67.4% reduction in accident density during the post intervention period under consideration. It can be concluded that the establishment of the road safety agency has a positive impact on total cases of RTA in Nigeria by reducing it significantly, although RTA still continues to be a big problem in Nigeria. This model and analysis will assist road safety agencies to re-strategize in their policy implementation in order to further reduce RTA occurrence, the number of persons killed and injured in Nigeria.
Public Interest Statement
Usually governments and regulatory agencies initiate certain policy interventions in order to achieve certain outcomes geared towards improving the living standards and for general societal wellbeing. Assessment of the outcomes of such policy interventions are usually done to evaluate the effectiveness of such policies in order to determine the need for modifications or improvement. Quantitative assessment of the effectiveness of such policies is invaluable to policy makers and regulators in improving the quality of social interventions.
In this work a quantitative assessment of the effectiveness of the establishment in Nigeria of a road safety agency on yearly total accident cases was successfully carried out using Box-Jenkins intervention analysis model.
The model effectively modeled the effect of the policy intervention on total road traffic accidents in Nigeria. The model would provide an invaluable quantitative tool to the road safety agency in improving the quality of its road safety interventions.
1. Introduction
Road Traffic Accidents (RTA), which has caused the deaths of millions of people, many injuries and permanent disabilities as well as numerous properties losses, has been a major problem in many parts of the world. According to World Health Organization WHO (Citation2008), about 1.2 million persons died annually which approximate about 125 persons per hour. RTA is one of the leading causes of morbidity and mortality worldwide, especially in developing countries. The total annual costs of road crashes to developing countries are estimated to be about US$ 65 billion, which is more than the total annual revenue received in development assistance (Jacobs & Aeron-Thomas, Citation2000). According to Shbeeb and Awad (Citation2016), road traffic injuries ranked as the ninth leading cause of death in 2004 and expected to become the fifth leading cause of death in 2030. Recent studies have shown an increase in the rate of RTA in many developing nations (Peden, Citation2004). This is attributed primarily to human, technical/Vehicular and environmental factors as well as urbanization, industrialization, population growth and increase in the number of motor vehicles on the roads. Nigeria, as a developing nation is equally bedeviled with this canker worm called RTA, hence the need for the Federal Government to introduce counter-measures in reducing Road Traffic Accident in Nigeria. These measures are what we shall refer to as interventions.
The literature is replete with various interventions measures taken to control RTA in various countries and regions of the world. Yang and Kim (Citation2003) studied the effects of policy interventions prior to the 2002 world cup on RTA in Korea. They analyzed road accident data from 1970 to 2000, before the preparations for 2002 World Cup began, and after the preparation began in 2001. From their findings, through multiple policy interventions, partly in response to the 2002 FIFA World Cup preparation, about two thousand road traffic deaths and nine thousand traffic-related disabilities were averted in 2001 alone. They equally found that through multiple policy interventions, road traffic crashes in Korea were reduced in a relatively short period of time, along with their associated injuries and fatalities. They however opined that road traffic crashes still pose a major public health problem, threatening the quality of life of the Korean people. Khayesi (Citation2012) studied why despite the intervention measures the risk of RTA in Kenya appear to be increasing when road safety measures exist. His findings showed that though the basic policy guidelines, institutional framework and measures to tackle RTA in Kenya exist, they are faced with a major problem of lack of commitment by key stakeholders. Other problems he found out include financial constraints, poor coordination, ineffective enforcement and limited community involvement and limited community involvement. The overall recommendation emanating from the analysis of his research was that there is need for a sustainable road safety strategy in Kenya that should go beyond the existing state of procrastination and symbolic actions. Sebego et al. (Citation2014), examined the impact of the implementation of alcohol and road safety-related policies on crash rates, including overall crash rates, fatal crash rates, and single-vehicle nighttime fatal (SVNF) crash rates, in Botswana from 2004 to 2011. Their findings showed that the overall crash rate declined significantly in June 2009 and June 2010, such that the overall crash rate from June 2010 to December 2011 was 22% lower than the overall crash rate from January 2004 to May 2009. They equally found out that there were significant declines in average fatal crash and SVNF crash rates in early 2010. Their findings also showed that Botswana’s then recent crash rate reductions occurred during a time when aggressive policies and other activities (e.g. education, enforcement) were implemented to reduce alcohol consumption and improve road safety. They opined that while it is unclear which of the policies or activities contributed to these declines and to what extent, these reductions are likely the result of several, combined efforts. Pridemore, Chamlin, Kaylen, and Andreev (Citation2013) studied the impact of a suite of 2006 Russian alcohol control policies on deaths due to traffic accidents in the country. They used autoregressive integrated moving average (ARIMA) interrupted time–series techniques to model the impact of the intervention on the outcome series from 2000 to 2010. Their findings show that the 2006 set of alcohol policies had no impact on female deaths due to traffic accidents. However, the intervention model revealed an immediate and sustained monthly decrease of 203 deaths due to transport accidents for males, which represents an 11% reduction relative to pre-intervention levels. They therefore concluded that the implementation of the suite of 2006 Russian alcohol control policies is partially responsible for saving more than 2400 male lives annually that would otherwise have been lost to traffic accidents. In Nigeria, the agency saddled with the responsibility for the implementations of road traffic accident reduction intervention policies is the Federal Road Safety Corps (FRSC). The impact of this agency had been studied in Edo and Oyo states of Nigeria by Igboanugo and Ekhuemelo (Citation2007) and Charles-Owaba and Adebiyi (Citation2001) respectively. From their analysis it was seen that there is a significant impact of intervention measures in RTA reductions in those areas since the inception of FRSC in 1988.
Concerted efforts had been made by several researchers over the years, as enumerated above, to identify the root cause of RTAs and their possible solutions. Also attempt had made to developed various methods of analyzing RTA through the use of univariate regression methods, descriptive statistics and charts in organizing and analyzing accidents data in selected roads and State in Nigeria and their possible solutions. These methods to an extent have helped in assessing RTAs situations in Nigeria. However these methods do not give holistic information and estimates of road traffic accident trend, and its related outcomes. Again, it does not predict accurate estimates of RTA cases, number of injuries and death in the near future. Also these methods do not give detailed evaluation of intervention measures implemented by road safety agencies in reducing RTA cases in Nigeria. Hence, the needful for a robust approach in identifying and predicting the extent of intervention policy on RTA in Nigeria.
The aim of this study therefore is to assess the impacts of the establishment of the road safety agency (FRSC) in Nigeria and to develop an intervention model for quantifying the impact of the agency on total accident cases in Nigeria.
2. Theoretical background
2.1. Intervention model
Generally intervention model function constitutes two main components namely; an intervention function and an ARIMA noise model (DeLurgio, Citation1998; Pridemore et al., Citation2013; Sebego et al., Citation2014).
Mathematically, the intervention model according to Box, Jenkins, and Reinsel (Citation2008) is expressed as(1)
(1)
where = the intervention function; Nt = ARIMA (p,d,q)
.
The general intervention model can be re-expressed in the this form(2)
(2)
where ω = intervention effect; δ = gradual increases or decreases term; B = backshift operator; Yt and Xt represent either deviations from their means for stationary series; r = level of autoregression or power of B in the denominator; S + 1 = number of ω terms in the numerator; b = delay in the intervention impact as shown by Xt−b and the notation is ARIMA noise model which can be expressed in the form (Box et al., Citation2008):
(2)
(2)
where = autoregressive coefficient; et = White noise residuals/errors; θ = moving average coefficient; q = Order of moving average operator; p = order of autoregressive operator.
2.2. Common intervention types
Majorly intervention model can be classified into two types; Zero-Order Intervention Functions and First-Order Intervention Functions.
(1) | Zero-order intervention functions-consist of the following components
| ||||||||||||||||
(2) | First-Order Intervention Functions-consist of the following components
|
where I = 1 or 0 otherwise; θ0 = Nt = normal central value of the total accident cases obtained from ARIMA analysis; ω0 = initial intervention effect; −ω1 = delay intervention effect; δ1 = gradual increases or decreases term of the first order intervention model.
3. Intervention modelling procedures
In order to model the impact of an intervention, DeLurgio (Citation1998), stated that we need to model the time series without that impact. It implies that we model the pre-intervention series. Hence the following are steps for intervention modelling (DeLurgio, Citation1998; Pridemore et al., Citation2013; Sebego et al., Citation2014):
(1) | The date of intervention should be known | ||||
(2) | Theory suggesting the types of impact on time series should be determined | ||||
(3) | Sufficient observation to identify the ARIMA noise model before the intervention | ||||
(4) | Sufficient intervention and post-intervention observation. |
3.1. ARIMA (Noise) modelling procedure
In time series analysis, an autoregressive model is represented in the following form (Box et al., Citation2008; Cryer & Chan, Citation2008; Pridemore et al., Citation2013; Sebego et al., Citation2014).
(8)
(8)
where yt = time series variable; ∅ = autoregressive coefficient; et = white noise residuals/errors.
Similarly, a moving average model is represented in the following form (Box et al., Citation2008; Cryer & Chan, Citation2008).(9)
(9)
where θ = moving average coefficient.
ARIMA Model was pioneered by the land mark work of Box and Jenkins in 1970 (Nwobi-Okoye & Igboanugo, Citation2012, Citation2015); hence it is often referred to as Box–Jenkins method. This model integrates the autoregressive and moving average models with appropriate differencing to achieve stationarity. In order words, ARIMA extends the combination of AR and MA process to non stationary processes.
From Equations (8) and (9), Equation (10) is obtained:(10)
(10)
Equation (9) could be represented in shortened form as:(11)
(11)
If the output xt is differenced d times to achieve stationarity, Equation (5) is obtained.(12)
(12)
where d = number of differencing.
In general by combining Equations (5) and (6), the model could be written as:(13)
(13)
In order to realize the ARIMA model based on Equation (7), a plot of the 27-year pre-intervention accident data was done using SPSS software. After the plot, the data was investigated for stationarity, using the plots of the autocorrelation functions (ACF) and Partial autocorrelation functions (PACF). The accident cases series derived from the plots were found not to be stationary, hence differencing was used to achieve stationarity. Stochastic regularity was achieved after the first differencing. Following the achievement of stationarity of the data, a univariate model was fitted to yt.
4. Results and discussion
4.1. Intervention modelling of road traffic accident cases in Nigeria
Generally as earlier noted, intervention model constitute two main components namely: an intervention function and an ARIMA noise model. The models shown in Sections 2 and 3 were used in this section to develop the intervention models for analyzing the impact of FRSC on total RTA in Nigeria. The details of the analysis are presented in here in Section 4.
4.2. Pre-intervention analysis and results
To model the impact of government policies (intervention), it is imperative to model the times series data of the total accident cases when there no intervention as described in Section 3.1. This required that we model the pre-intervention series which exist as noise Nt in the equation above (i.e. ARIMA model). Let the pre-intervention period for road traffic accident cases in Nigeria be 1960–1987. That is, before the establishment of FRSC. To obtain a parsimonious model various component of ARIMA has been analyzed below.
The plot in Figure shows the actual versus predicted value of RTA in Nigeria from 1960 to 2019, while the plot in Figure below shows the actual figures of RTA in Nigeria from 1960 to 1987 (pre-intervention period).
A close examination of ACFs and PACFs of the pre-intervention series shown in Figures and suggest an ARIMA (1,1,1)1 model. From Figure , impact of the intervention was noticeable in 1988 with an exponential declining profile. And it was observed that the trends were of Gradual, temporal impact of the first order intervention functions. To determine the best ARIMA noise model, Tables –, indicated ARIMA (1,1,1)1 which gave the highest R2, lowest BIC, and relatively low Ljung Box. From Box et al. (Citation2008) and DeLurgio (Citation1998), ARIMA (1,1,1)1 can be expressed, as shown in Equation (9), as:(14)
(14)
Table 1. Parameters estimation of ARIMA model for total accident cases (1960–1987)
Table 2. Summary of diagnostics test evaluation of ARIMA models for pre-intervention period of total accident cases in Nigeria from 1960 to 1987
Table 3. Summary of Ljung-Box diagnostics test of ARIMA models for pre-intervention period of total accident cases from 1960 to 1987
where Yt = total accident cases; θ0, ∅1, θ1. are coefficients as defined previously above.
In terms of the observed series the model can be expressed as:
(15)
(15)
(16)
(16)
Rearranging Equation (16), Equation (17) is obtained:(17)
(17)
Therefore applying equation for ARIMA (1,1,1)1, (Box et al., Citation2008; DeLurgio,Citation1998) and fitting total accident cases model by applying Equations (14)–(17) and Table , we have :(18)
(18)
Table 4. ARIMA (1,1,1)1 from 1960 to 1987
In forecasting form Equation (19) becomes:(19)
(19)
Figure shows the actual vs. fitted value of ARIMA (1,1,1)1 for total accident cases (1960–1987) using Equation (20).
4.3. Intervention analysis and results
We hereby present the intervention analysis modeling results used to access the impact of FRSC on total accident cases in Nigeria. Examining the graph of the yearly total accident cases in Nigeria from 1960 to 2014 shown in Figure , shows that the impact of the intervention in 1987 could be modeled as a second order intervention model with abrupt temporary impact.
Recall that:
From Equation (7) which represents a second order intervention model with abrupt temporary impact, we have:
δ is a parameter of Gradual, temporal impact of intervention model employed, constrained in an interval of −1 ≤ δ ≥ 1. For model stability a central value of 0.5 was chosen.
Therefore, let δ = 0.5
Expanding(20)
(20)
(21)
(21)
Table shows the result of the intervention model for road traffic accident in Nigeria from 1960 to 2014. Table shows the summary of reported road traffic crashes trends in Nigeria from 1960 to 2014. It could be seen from Table that the first gradual reduction in road traffic accident cases was period 27 and 28 which corresponds to 1987 and 1988.
Table 5. Results of intervention model for road traffic accident cases in Nigeria
Table 6. Road traffic accidents data in Nigeria (from 1960 to 2014)
Therefore, the intervention effect ω0 is the difference in year 1987 and 1988, which can be expressed as:
(22)
(22)
Figure shows the plot of total accident cases and predicted accident cases without intervention. As shown in Figure , without intervention in 1988, the total accident cases recorded would have sky rocketed causing pains and hardship to the populace. Figure shows the plot of total accident cases versus the predicted total accident cases with the intervention model. As Figure shows, there is a gradual reduction in total accident cases from 1988 downwards, clearly showing the effects of the intervention.
Generally, the impact of intervention measure on RTA occurrence was modelled as shown in Equation (23), and Table shows a summary of the expected intervention impacts. From the analysis, the impact of intervention measures on road traffic accident occurrence in Nigeria yields considerable results with gradual reduction of total accident cases (ω0 = −2,423). For example the total number of accident cases from 1961 to 1987 (26 years), the period before the intervention, was 657,280 while the total number of accident cases from 1988 to 2014 (26 years), the period after the intervention, was 430,721. This represents a 34.5% reduction in total accident cases after the intervention. Considering the fact that the average yearly population of Nigeria from 1961 to 1987 was 64,159,442 while the average from 1988 to 2014 was 128,973,480, if accident density is defined as the number of accidents per person, the average accident density in percentage from 1961 to 1987 was 1.0244 and from 1988 to 2014 it was 0.3340. Hence, there was a 67.4% reduction in accident density during the post intervention period under consideration. If road traffic agencies in Nigeria would continue to build on the existing structures, there would be a dramatic reduction of RTA occurrence in Nigeria.
However from Figures and and Table , it could be observed that the number of persons killed seems to be on the increase while the number injured in RTA in Nigeria seems to decrease. Hence, it appears that the declining rate of RTA cases did not translate to reduction in the number of persons killed when compared to the pre-intervention period. But there is a reduction in the number of persons injured during the post-intervention period in Nigeria.
For instance the total number of fatal accident cases from 1961 to 1987 (26 years), the period before the intervention, was 66,490 while the total number of accident cases from 1988 to 2014 (26 years), the period after the intervention, was 114,207. This represents a 71.77% increase in serious accident cases after the intervention. Considering the fact that the average yearly population of Nigeria from 1961 to 1987 was 64,159,442 while the average from 1988 to 2014 was 128,973,480, if fatality density is defined as the number of accidents per person, the average fatality density in percentage from 1961 to 1987 was 0.1036 and from 1988 to 2014 it was 0.0885. Hence, there was a 14.55% reduction in fatality density during the post intervention period under consideration.
Furthermore, the total number of serious accident cases from 1961 to 1987 (26 years), the period before the intervention, was 274,973 while the total number of accident cases from 1988 to 2014 (26 years), the period after the intervention, was 201,001. This represents a 26.54% decrease in serious accident cases after the intervention. Considering the fact that the average yearly population of Nigeria from 1961 to 1987 was 64,159,442 while the average from 1988 to 2014 was 128,973,480, if seriousness density is defined as the number of serious accidents per person, the average seriousness density in percentage from 1961 to 1987 was 0.4286 and from 1988 to 2014 it was 0.1566. Hence, there was a 63.45% reduction in seriousness density during the post intervention period under consideration.
From the analysis above, it could be concluded that the increase in fatalities during the post intervention period could be due to population and economic growth which has led to increase in the number of roads and road users. Concerted effort should be made by road traffic agencies and stakeholders to explore fully the root-cause of high number of deaths and injuries still occurring on our roads despite the government interventions. As noted by Nwobi-Okoye (Citation2011), most road traffic problems in Nigeria are due to the tyranny of small decisions by motorists, citizens and law enforcement agents. Nwobi-Okoye identified inadequate law enforcement by government regulatory agencies as being responsible for the road traffic problems in Nigeria. It appears that after the temporary impact on account of the initial government intervention, the government regulatory agencies local, state and federal government had not done enough to sustain the tempo and curb to the barest minimum defective behaviours that Nwobi-Okoye (Citation2011) identified as the main causes of road traffic problems in Nigeria. These defective behaviours include but not limited to:
(1) | Driving against the traffic. | ||||
(2) | Following illegal routes. | ||||
(3) | Dropping/picking passengers at unauthorized points along the road. | ||||
(4) | Abandoning broken down vehicles on the road. | ||||
(5) | Buying goods from hawkers without clearing away from the road. | ||||
(6) | Illegal parking of vehicles along the main road. | ||||
(7) | Construction and use of illegal structures for businesses along the main road. | ||||
8) | Unrestricted use of private cars. | ||||
(9) | Illegal check points mounted by the police and other law enforcement agents etc. |
ChikkaKrishna, Parida, and Jain (Citation2015) noted that there is a significant need to improve the highway safety during roadway planning, design and operations in developing countries and Nigeria is not an exception. It is imperative that the authorities and policy makers in Nigeria adopt this approach in the development and management of its roads.
5. Conclusions
The intervention model developed in this work has successfully been used to assess the impact of Government policies on reducing RTA in Nigeria through the road safety agency known as FRSC. The conclusions from this study are as follows:
(1) | The establishment of the road safety agency has had an abrupt temporary impact on the total accident cases in Nigeria. Hence there was a significant reduction in the total accident cases during the post intervention period. | ||||
(2) | The number of serious accident cases declined significantly in the post intervention period. | ||||
(3) | Although there seemed to be an increase in fatalities during the post-intervention period, the fact that the fatality density decreased significantly during the post-intervention period means that the increase could be attributed to population growth (the populations has doubled in the post-intervention period) and economic growth (economy has more than doubled during the post-intervention period, in comparison with the pre-intervention period, to become the largest in Africa). | ||||
(4) | This quantitative model would be invaluable to the Nigerian government and the road safety agency in re-strategizing their policy implementation in order to reduce RTA occurrence, the number of persons killed and injured in Nigeria. To do this requires the increase in the absolute value of ω0 from the current value of 2,423 to a much higher value such as 5,000 (ω0 = −5,000) or higher. |
Additional information
Funding
Notes on contributors
Benjamin Ufuoma Oreko
Benjamin Ufuoma Oreko is a lecturer in the Department of Mechanical Engineering, Federal University of Petroleum Resources, Effurun, Nigeria. His academic research interest include: Engineering materials, Nanotechnology, Operation Research, Mechanical Design and Renewable Energy.
Chukwuemeka Chidozie Nwobi-Okoye
Chukwuemeka Chidozie Nwobi-Okoye is lecturer in the Faculty of Engineering of Chukwuemeka Odumegwu Ojukwu University, Nigeria. His research interest include: Operations Research, CAD/CAM, Game Theory, Simulation and Artificial Intelligence.
Stanley Okiy
Stanley Okiy is a training officer in the department of welding engineering and offshore technology, Petroleum Training Institute, Effurun, Nigeria. He is involved in active academic research and engineering consultancy. His research interest include: Operations research and project management.
Anthony Clement Igboanugo
Anthony Clement Igboanugo is a Professor in the department of Production Engineering, University of Benin, Nigeria. He has 21 years industrial and university teaching experience and has to his credit over 80 scholarly publications. He is involved in active academic research and engineering consultancy for government and industries.
References
- Box, G. E. P., Jenkins, G. M., & Reinsel, G. C. (2008). Time series analysis forecasting and control. New York, NY: McGraw-Hill.
- Charles-Owaba, O. E., & Adebiyi, K. A. (2001). On the performance of the FRSC: The case with the Oyo State sector command. Nigerian Journal of Engineering Management, 2, 50–56.
- ChikkaKrishna, N. K., Parida, M., & Jain, S. S. (2015). Calibration of safety performance function for crashes on inter-city four lane highways in India. Cogent Engineering, 2(1), 1031929.
- Cryer, J. D., & Chan, K. (2008). Time series analysis with applications in R (2nd ed.). New York, NY: Springer.
- DeLurgio, S. A. (1998). Forecasting principles and applications (3rd ed.). New York, NY: McGraw-Hill.
- Jacobs, G., & Aeron-Thomas, A. (2000). Africa road safety review final report. Washington, DC: US Department of Transportation, Federal Highway Administration.
- Igboanugo, A. C., & Ekhuemelo, E. F. (2007). Intervention analysis of road traffic accident. Advanced Materials Research, 18–19, 375–382.10.4028/www.scientific.net/AMR.18-19
- Khayesi, M. (2012). An analysis of the pattern of road traffic accidents in relation to selected socio-economic dynamics and intervention measures in Kenya (Doctoral dissertation). Nairobi: Kenyatta University.
- Nwobi-Okoye, C. C. (2011). Game theoretic analysis of road traffic problems in Nigeria. African Research Review, 5, 184–199.
- Nwobi-Okoye, C. C., & Igboanugo, A. C. (2012). Performance evaluation of hydropower generation system using transfer function modelling. International Journal of Electrical Power and Energy Systems, 43, 245–254.
- Nwobi-Okoye, C. C., & Igboanugo, A. C. (2015). Performance appraisal of gas based electricity power generation system using transfer function modelling. Ain Shams Engineering Journal, 6, 541–551.
- Peden, M. (2004). World report on road traffic injury prevention. Geneva: World Health Organisation.
- Pridemore, W. A., Chamlin, M. B., Kaylen, M. T., & Andreev, E. (2013). The impact of a national alcohol policy on deaths due to transport accidents in Russia. Addiction, 108, 2112–2118.10.1111/add.12311
- Sebego, M., Naumann, R. B., Rudd, R. A., Voetsch, K., Dellinger, A. M., & Ndlovu, C. (2014). The impact of alcohol and road traffic policies on crash rates in Botswana, 2004–2011: A time-series analysis. Accident Analysis & Prevention, 70, 33–39.10.1016/j.aap.2014.02.017
- Shbeeb, L. I., & Awad, W. E. H. (2016). Road traffic safety perception in Jordan. Cogent Engineering, 3(1), 1127748.
- WHO. (2008). World Report on road traffic injury prevention: Summary. Geneva: Author.
- Yang, B. M., & Kim, J. (2003). Road traffic accidents and policy interventions in Korea. Injury Control and Safety Promotion, 10, 89–94.10.1076/icsp.10.1.89.14120