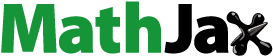
Abstract
Dissolved Oxygen (DO) is an important parameter to be monitored as far as water quality of rivers and streams are concerned. On the other hand, in rivers and streams, varying roughness occurs naturally but their contributions to DO availability is yet unknown. This paper examines the effects of varying roughness of different sizes and arrangement patterns on DO and also reveals how Error Correction Methodology as a modelling technique can be applied in river studies rather than using the traditional ordinary least square method with velocity (V), Froude number (Fr), roughness coefficient (K) and dispersion coefficient (d) captured as explanatory variables. The findings of this study revealed that roughness coefficient (K) had no effect on DO i.e. negative relationship with coefficient value of −0.796, with corresponding t-statistics (t = 0.615) suggesting its non-significance. In addition, Froude number (Fr) and dispersion coefficient (d) also showed negative relationships respectively (−77.71 and −2.039) with DO but with sharp significance as revealed by the corresponding t-ratio (t-ratio = −2.75 and −4.08). Thus, the study suggests that dispersion coefficient or its dimensionless number as a variable is important and should be included in the modelling, otherwise, the spread of pollutants (BOD) in the transverse and vertical directions rather than their single centre point values are essential to improve the outcome of DO and reaeration coefficient (k 2) modelling.
Public Interest Statement
Dissolved Oxygen (DO) is very important in assessing the health status of a river. This study shows how the modelling of DO in rivers can be improved through the use of the Error Correction Methodologies (ECM) rather than the usual linear regression method, a different technique relatively new to river modelling. It also reveals how the technique can be applied thereby assisting researcher to compute this important parameter properly. Furthermore, it identifies a new variable to be added or a procedure to be adopted with respect to sampling technique that will improve DO and reaeration coefficient modelling.
1. Introduction
Surface water abounds, yet having to access it void of pollution for our daily needs is far from reality (Owusu, Sarkodie, & Ameyo, Citation2016). This is because most people assume it to be a waste stream by which waste materials are transported to other locations. Unknowingly, these surface water have a meeting point with ground water sources (Olukanni, Adebayo and Tenebe, Citation2014; Tenebe, Ogbiye, Omole, & Emenike, Citation2016); and can be contaminated, thus showing a need for its protection. Conversely, the contaminants in surface water could be either organic or inorganic in form. The organic form is identified by measuring the concentration levels of certain wastewater parameters i.e. Biochemical oxygen demand (BOD), Chemical Oxygen demand (COD), Total suspended solids (TSS) among others while the inorganic pollution levels are measured by the presence of metals and non-metals. The presence of both organic and inorganic substances in rivers can drop the DO levels below the required values as more DO will be required for degradation as pollution increases. For instance, when rivers or streams are faced with pollution, the BOD—which is a measure of the level of oxygen used up by micro-organisms for degradation is increased. This reduces the light penetration level into the river (Martin, McEachern, Yu, & Zhu, Citation2013), thereby negatively impacting on reaeration capacities of the receiving bodies, slows down degradation process and results in the emergence of water-related diseases when consumed unconsciously (Tenebe et al., Citation2016). However, the BOD levels can be reduced in the presence of sufficient dissolved oxygen (DO) as it would improve the health status and water quality of the receiving water bodies, thereby cheaply meeting the set guidelines recommended for unpolluted surface water by various health organizations (Ugbebor, Agunwamba, & Amah, Citation2012). Therefore, it is necessary that constant monitoring of water bodies is carried out as this would assist in the monitoring of DO levels and take measures to improve the same in surface waters when required thereby regulating the rate of anthropogenic pollution. This makes it an important factor to be considered when the ecosystem is brought into perspective (Chu, Hua, & Ji, Citation2014), with its value varying randomly due to variation in the physical, chemical, biological compositions of rivers or streams (Facchini, Mocenni, Marwan, Vicino, & Tiezzi, Citation2007) as well as variation in aspect ratio of the river or stream along with the degree of dispersion occurring. Nevertheless, recent studies have shown that introducing artificial aerator devices could increase the oxygen level into a river or stream. The introduction of clean oxygen into receiving streams have been very effective in time past (Dong, Zhu, & Miller, Citation2009; Kumar, Moulick, & Mal, Citation2010; Moulick & Mal, Citation2009) but are not sustainable in practice. Recently, Chu et al. (Citation2014) showed that these artificial methods of DO improvement might include the placement of hydraulic structures but this approach may be expensive to adopt. Without doubt therefore, there is need to identify sustainable methods for DO inclusion, as urbanization is increasing in every part of the world, making pollution of receiving bodies inevitable. Therefore, a major breakthrough in river management practice is to see how oxygen is included sufficiently and naturally. Roughness occurs naturally in streams and in rivers most times, although it can be introduced at the beds or walls of artificially constructed channels as masonry works to increase stability of soils and reduce possible erosion. However, it may also serve as a source of DO inclusion as water coming in contact with rough surfaces may result in changes in the elevation and velocity of watercourse. On the other hand, modelling of DO has been making the rounds since the era of Streeter and Phelps in Citation1925 (Omole, Longe, et al., Citation2012). Although, modelling of such an important variable requires large data inputs which may put us at a disadvantage when cost, labour and time are to be considered simultaneously, but this has not reduced the application of several modelling techniques as it seems to be a better alternative when compared to constant monitoring or field data collection. But to rely on the latter, adequate parameters and the proper modelling technique need to be employed. Most experimental results obtained in the literature made use of linear multiple regression methodologies for prediction which are simple and straightforward but applying this technique may lead to bogus results due to non-stationarity of time series variables and datasets as well as presence of serial or autocorrelation, both of which can reduce the predictive strength of the endogenous variables. Autocorrelation is a kind of correlation coefficient that reveals the relationship between errors of two datasets from a particular variable, measured at different times (Box & Jenkins, Citation1994). Also, it is used to identify variables that are not skewed as well as to increase the confidence placed on the suitability of a time series data at normalized state. This is the scenario adopted in developing reaeration coefficients of rivers around the globe (Jha et al., Citation2001; Langbein & Dururn, Citation1967; CitationOmole et al., 2013; Owens, Edwards, & Gibbs, Citation1964) where the data-set were analysed using OLS methods. Consequently, developing a robust reaeration coefficient (k 2) is achieved by modelling the DO profile of a given river (Lin & Lee, Citation2007; Omole, Longe, et al., Citation2012) with the BOD (Omole, Citation2012). Thereafter, the mathematical combination involved the outright use of Ordinary Least Square estimates which is very like to yield inconsistent coefficients and relationships between the explanatory variables because the assumptions governing OLS method is that the variables are linearly related and are error free (Hutcheson, Citation2011). Generally, time series data and variables need to be tested for cointegration and stationarity to determine short-run and long-run stability relationship of the coefficients of the variables (Parajuli, Chang, & Hill, Citation2015). The stability of the variables when achieved will give more confidence to the model generated. The stationarity of the variables can be achieved using Augmented dickey fuller (ADF) or the Philip-Perron (PP) test while Cointegration is achieved using the CUSUM test (Tenebe, Ogbiye, Omole, & Emenike, Citation2017). These statistical methods are used to test the new form of a data-set to remove errors associated with time series measurements after being transformed to standard form that will not be biased since they have varying units and if ignored, could result in a low or high significance (t-statistics) of the corresponding coefficients. This implies that neglecting these procedures may result in some variables been significant whereas they should not be and vice-visa. Furthermore, the Error Correction Method (ECM) can be used in place of Ordinary multiple regression method to adjust such variability including those obtained during the experimental process. Also, It has the advantage of using limited data-set for modelling yet pointing out the significance of each of the variable considered. The use of this technique in modelling water quality parameters and their relationships are rare in the literature but has recently been used to show BOD variability due to disinfectant application in sewage (Tenebe, Emenike et al., Citation2017). Therefore, the objectives of this study are to investigate and model the effects of including roughness of different sizes and arrangement patterns on DO, to assist water resources managers and policy makers know their contributions in rivers and streams and to expose a new method of regression in river studies that can be applied when field data are collected using the Error Correction Methodology for improved result. This method involves a step-wise process of data clean up for error reduction as shown in this paper.
2. Materials and methods
2.1. Assembling of the aggregates
Aggregates (Granite) of different sizes were collected from a granite outlet in Ota, Ogun State, Nigeria. The granite aggregates were packed in black polythene bags and transported to the Geotechnical laboratory, in the department of Civil Engineering, Covenant University, where they were washed thrice with distilled water to remove any form of impurities. After which, the stones were exposed and dried up for three days as well. Sieve analysis was carried out to separate the stones into different particle sizes. The various particle sizes were glued on a thick hard material and attached firmly to the channel walls of the flow channel to avoid removal due to water pressure. Consequently, the roughness coefficient of the different particle size were determined using Equations (3.1) and (3.2) respectively used in a study previously (Agunwamba, Anyanwu, Owhondah, & Raji, Citation2008), then the values obtained through this process were used for the statistical modelling.
(3.1)
(3.1)
(3.2)
(3.2)
2.2. Tracer studies experiment and dissolved oxygen measurements
Common salt was used as salt tracer for the measurement of dispersion coefficient. This tracer served as pollutants released into channels, streams or rivers. 30 gram of common salt was premixed in a 250 ml volumetric flask filled with 100 ml of distilled water and mixed properly. Serial dilution was carried out to develop the relationship between Electrical Conductivity (measured with Hanna Instrument Edge Portable Multi-meter-HI98194) and its corresponding concentration were obtained. With the concentration obtained, the dispersion coefficient values were calculated using the variable distance and time method which has been reported elsewhere (Agunwamba, Citation1997; Tenebe et al., Citation2016; Tenebe, Ogbiye et al., Citation2017). Furthermore, DO measurements were collected with Hanna Instrument Edge multi-meter (HI 2020) using a high sensitive probe connected to it. Dissolved Oxygen measurements were measured at the inlet and outlet of the laboratory channel respectively simultaneously and the difference between the two points used for statistical modelling. Calibration of the Hanna Instrument was done regularly by using the 1413 µS/cm and 12.33 mS/cm calibration standard solution manufactured by Hanna instruments to improve the accuracy of the data obtained during experimental process.
2.3. Experimental set-up
This experiment was carried out in the Hydraulics laboratory of the Department of Civil Engineering, Covenant University, Ota, Ogun State. It involved the use of a flow channel with dimensions 4.0 m × 0.15 m × 0.175 m; water from a source was pumped into it, and regulated manually to achieve the desired flow conditions. The velocity of flow were obtained using a velocity flow meter and the results were recorded three times. Finally, the sidewalls of the channel were coated with roughness of different sizes and arrangement which has been estimated (see Table ) and clipped to prevent distortion of the material during the experimental process. The explanatory variables measured, namely DO, Velocity, dispersion, depths and roughness coefficient were obtained in duplicates for precision of the data.
Table 1. Aggregate sizes, combinations and their respective roughness coefficients.
2.4. Data analysis
Assembling of data for analysis was carried out on Microsoft Excel 2013. eViews version 8.0 was used to conduct the descriptive statistics and modelling process as well as the various statistical considerations mentioned in this study such as: Jarque-bera test for normality, Augumented Dicky fuller test for stationarity, Cusum test for coefficient stability, Johansen Cointegration Test to ascertain the long run relationships for all the explanatory variables, while HAC (Newey-West) and Durbin Watson statistic were also used to control heteroscedasticity and auto-correlation respectively in the model. All these and their applications will be explained in details in the next sections.
3. Results and discussion
Table shows the descriptive statistics carried out on the datasets. It revealed that Dissolved Oxygen (DO), velocity (V), Froude number (Fr), roughness (K) and Dispersion (d) have mean values of 0.466 ± 0.065, 0.241 ± 0.210, 0.025 ± 0.005, 0.148 ± 0.119 and 0.107 ± 0.05 respectively. Also, the normality of the variables—which depicts the symmetry of the samples, was conducted using Jarque-Bera test. The test showed that all the samples or observation with the exception to Froude number were normally distributed.
Table 2. Descriptive statistics of the explanatory variables
In addition, the inter-variable relationship and multi-collinearity that exist among the variables were investigated to identify variables with exact relationships. These were determined using the correlation matrix. Table shows that there is a strong positive relationship between velocity—roughness, (r = 0.875) while a strong relationship exist between Froude number—velocity and Froude number—roughness (k) having values of r = 0.69 and −0.94 respectively. For velocity-roughness relationship, it is observed that as the roughness of the particle increased, the velocity increased by 0.875. This value also revealed the presence of multi-collinearity in the statistical model to be generated with its value greater than 0.8. Conversely, the presence of multi-collinearity implies that there is an exact relationship between parameters in the model thereby reducing the trust placed on the R 2 values obtained from an ordinary regression model (low or high R 2). Likewise, the same can be inferred from the Froude number-roughness relationship, although in this case, an inverse relationship exists with a value of 0.94.
Table 3. Correlation statistics of all variables used for ECM modelling
According to the findings of Granger and Newbold (Citation1974), it is very uncommon for time dependent data-set to be stationary, and when used in that form, the result obtained may not yield good findings i.e. low R 2 and with insignificant coefficient values resulting from low t-statistics, insignificant coefficient resulting from low t-statistics etc. Therefore, stationarity test at first or second difference should be considered. Furthermore, Ramanathan (Citation1992) revealed that most time series data are usually stationary at either first or second difference. Thus, the Augmented Dickey Fuller test for unit root was conducted and employed to determine the levels of stationarity of the variables. Table shows the summarised ADF statistics and from the stationarity test result, the variables were found to be stationary at first difference and hence, exhibit first order integration I (1).
Table 4. Augmented Dickey Fuller test for stationarity of the variables after first difference
Also, co-integration test was performed on the variables. This is required to ascertain the long-run stability relationship among the variables present in the model. This was achieved using the co-integration test (Johansen, Citation1988; Johansen and Juselius, Citation1990). In this method, Max-eigenvalue and trace test were employed to determine the degree of cointegration among the variables at first difference. Tables and shows the result from Johansen co-integration test.
Table 5a. Cointegration test for all the variables considered for ECM showing no deterministic trend (Trace)
Table 5b. Cointegration test for all the variables considered for ECM showing no deterministic trend (Max-Eigen)
From the outcome, the statistics from both test indicated that the hypothesis of no co-integration among the variables is rejected. Specifically, in the Trace test, five (5) cointegrating equation(s) at p = 0.05 (Table ), while the Max-eigenvalue (Table ) test revealed three (3) co-integrating equation(s) at p = 0.05. To conclude, the results indicated that there is a long-run relationship as well as stability of the variables to be used for the statistical model.
In furtherance, since co-integration of the variables has been achieved, the ECM is developed to capture the relationships of all the variables. The ECM helps to balance the speed of adjustment among the parameters in the equation developed unlike an ordinary regression model, thereby making the coefficients to be obtained in the long run more reliable. Equation (3.3) shows the representation of the correction model:(3.3)
(3.3)
where i = 1, 2, 3, … n. Also; β o = constant or intercept; β 1i = coefficient of Velocity with velocity measured in (m/s); β 2i = coefficient of Froude number with Froude number dimensionless; β 3i = coefficient of Dispersion with Dispersion measured in (m2/s); β 44i = coefficient of Roughness (K) with dimensionless units
Table reveals the Error corrected regressed equation of the explanatory variables considered during the experimental period. From the table, the constant value is −0.023601. This implies that if the explanatory variables in the equation were fixed, obviously, the level of DO within the system would be fixed at −0.024 units. In practice, the reduction maybe due to micro-organism using up the available oxygen without it being replaced. However, this is insignificant as revealed by the corresponding t-ratio (t = −0.434436) as this is likely not to occur. In addition, the co-efficient of velocity is −0.653344. This also implied that a 1unit increase in velocity will lead to a decrease in DO by 0.653. The reduction in the DO values may arise due to turbulent flow in the system that limits the exchange of oxygen. This is supported by the t-ratio which further revealed that although this relationship aforementioned occurred, there is no contribution of roughness to the improvement of the current characteristics of this parameter (t = −0.531523). However, the velocity relationship finding is countered with the relationship between DO and Froude number which has a coefficient value of −77.71. This revealed that a 1 unit decrease in DO will emanate from a 77.71 increase in velocity, and decrease in depth. This is in line with our a priori expectations that increase velocity cannot bring about DO increase without experiencing a variation in depth. This is one of the advantages of including hydraulic jump in some hydraulic structures as it contribution to oxygen enrichment into the system. Furthermore, a decrease in depth rapidly favours the transfer and distribution of DO within the river, stream or channel system as more oxygen will be present. This findings corroborates that of Ezeilo and Dune (Citation2012) which revealed a significant large amount of DO in the dry season as compared to the wet season in Amadi creek in Port-Harcourt, Nigeria due to low depth variation observed in the dry season. In addition, this findings further supports the reaeration coefficient equations in the literature (Agunwamba, Maduka, & Ofosaren, Citation2007; Omole, Citation2011; Omole & Longe, Citation2012; Owens et al., Citation1964). Consequently, a reduction in velocity allows oxygen to interact with stream/river system more adequately leading to high degradation. This technique is employed in the treatment of waste stabilization points (WSPs) and wetlands. This is emphasized by the t-statistic, which has a value of −2.755 and significant at 5% (p < 0.05). In addition, the coefficient value for dispersion coefficient is −2.03882. This also connotes that a 1-unit increase in DO will exist when dispersion coefficient is reduced by 2.038882. This is important for increased degradation of pollutants in stream/river thereby resulting in improved water quality. In a river system, if pollutants are spread quickly, there is little or no degradation-taking place. Therefore, most likely, the concentration of pollutants obtained from the inlet & outlet may be same especially at low depths coupled with high velocities. In addition, the findings of this study regarding inculcating dispersion coefficient can also be likened to obtaining pollutant concentration (BOD) from different points within a given width of a river channel rather than from a single point. This is essential because these pollutants are constantly degrading and spreading and at such, oxygen is used up in that distributive pattern. Likewise, the t- ratio shows that the variable (dispersion coefficient), having t = −4.083 (p < 0.05) is important when DO is to be modelled, but these scenario has not been extensively captured in DO or reaeration coefficient model as observed from the literature.
On the order hand, the coefficient of Roughness coefficient (k), and how it can affect DO was considered and the value obtained was −0.7963. This implied that a 1unit increase in DO will be achieved if the roughness is reduced by 0.796. But this effect was not statistically significant (p > 0.05) as revealed by the t-ratio (t = −0.6146). This may be because of low velocities usually experienced at the boundaries of the channels, as well as the small sizes of roughness materials used during the study which produce little or no turbulence to accommodate any significant DO increase.
Additionally, the ECM of this equation is −0.8177. This implies that a 1 period lag of the Error correction term, indicates that 82% of the short run deviations or disequilibrium that would have affected the coefficients with their corresponding significance adversely is now been corrected in the long run by this technique thereby improving the accuracy of the result obtained. The corresponding t-values and probability reveals that its contribution to the model is highly significant at 5% (t = −5.308806; p = 0.001).
According to Field (Citation2009), the Durbin Watson (DW) values obtained for a model should lie between 1.5 and 2.5 but values less than 1 and greater than 3 should be out-rightly rejected This dimensionless parameter is used to measures the degree at which the residual error value of a regressed model (linear or multiple) are dependent on each other (Harvey, Citation1990). When they are serially correlated, the value of DW is expected to fall within the unacceptable range. Finally, with the statistical value set at approximately 2.06, with R 2 value of 0.84, the model findings are acceptable. This showed that about 84% of the total variation in DO is explained by the joint influence of the explanatory variables used to develop this model. Furthermore, the F-statistics in the equation is 11.175 with probability of 0.000059 (p < 0.05), indicating that the variables considered in the model are simultaneously significant. The ECM result for DO statistical modelling is reported in Table .
Table 6. Error correction estimates for dissolved oxygen modelling
However, these findings could still be in doubt when Heteroscedasticity, auto-correlation and short run instability still exist in the model as their presence will affect the estimated parameters. By heteroscedasticity, we imply that the continuous data of a variable used for predicting another variable is uneven in distribution, while auto-correlation suggest the presence of similarity in error terms obtained from a time series study. According to Costa and Castagliola (Citation2011), the presence of auto-correlated errors with error due to calibration and experimentation can make a model perform very poorly. Whereas, a simple ordinary least square estimates only assumes homoscedasticity and no serial correlation which in most times never exist in a time series analysis. To circumvent the former, we have transformed the data obtained by taking a first difference to ensure that the continuous datasets are stationary before using the ECM for regression. Thereafter, we need to test for Heteroscedasticity, auto-correction and parameter stability test using Breusch-Pagan-Godfrey test, Breusch-Godfrey Serial Correlation LM Test (Breusch & Pagan, Citation1979) and Cumulative Sum of Recursive Residual (CUSUM) before the model result is accepted.
Tables and shows the Heteroscedasticity and auto-correlation status of the model. From the F-statistics, the values are 0.729329 and 0.497489 with p-values of 0.6111 and 0.4922 respectively. The null hypothesis of both tests is to reject when p < 0.05 and conclude that there is presence of Heteroscedasticity and auto-correlation in the statistical model. But as seen in Tables and , the p-values are greater than 0.05. This implies that the null hypothesis will be accepted and thus concluded that both heteroscedasticity and auto-correlation do not exist. Similarly, the CUSUM test reveals positive conclusions. This is based on the fact that, the blue lines within the region do not extend or exceed from the boundary pink line (Figure ).
Table 7. Heteroscedasticity test using the Breusch-Pagan-Godfrey test
Table 8. Breusch-Godfrey serial correlation LM test
4. Conclusion
The effect of varying roughness on Dissolved Oxygen (DO) has been considered in this study using Error Correction Methodology (ECM). The ECM was selected over the OLS method because the mathematical assumptions governing its use may not be met in a time series experimental study like this and has shown to be effective as findings of the study corroborates with earlier versions in the literature in terms of relationships of variables. Moreover, this study is important for an effective river management practise as the insight can assist researchers and policy makers reduce the pollution levels of surface water by increasing the DO concentration in rivers or streams with the artificial inclusion of roughness (wall or bed). On the other hand, even though roughness co-exists at the bed or channel walls, their synergistic contributions also are unknown in this context. Therefore, from this study, it revealed that Froude number and dispersion coefficient are important variables to be considered when modelling DO or considering reaeration coefficient in streams, rivers, WSP or wetlands; but on the contrary, dispersion coefficient has been avoided in previous models. This parameter can be included by proper capturing BOD by taking spatial measurements not only in breadth but also in depth to improve DO modelling rather than using centre point measurements. Likewise, the reaeration coefficient (k 2) will equally give better estimates bearing in mind that both DO and k 2 have synergetic relationships. Consequently, river wall (of which roughness occurs naturally) roughness had an insignificant contribution in DO levels as revealed by the t-ratio and p-value (t = 0.615 and p = 0.5480). Therefore, future work should include bottom or both wall and bottom roughness on DO, as well as roughness effects on dispersion coefficient and more channels of different aspect ratios should be used in order to reveal any significant changes as highlighted in this study. To wrap up, aside the considerations already implemented by taking temporal measurements of DO and BOD in k 2 modelling, we further emphasize that spatial variability of DO, BOD and k 2 should be considered in future field modelling studies due to the findings of this study and the strong significant relationships that exist between them.
Funding
The authors received no direct funding for this research.
Acknowledgement
The authors thank Covenant University for their support in carrying out this study. Also, we are so grateful to the reviewers of this work for their valuable contributions towards the final draft.
Additional information
Notes on contributors
I.T. Tenebe
I.T. Tenebe is a Lecturer in the Department of Civil Engineering, Covenant University Ota, Ogun State. His research interest is in the public health aspect of Environmental Engineering, Health Statistics, Risk analysis, Solid Waste Management, Water and Waste Water Treatment, Adsorption, Constructed Wetland and WASH-related research among others. He has authored and Co-authored over twenty-five (25) peer reviewed scholarly articles within his research interest and has attended four (4) conferences.
References
- Agunwamba, J. C. (1997). Reduction of sampling time in tracer studies. Water Environment Research , 69 , 343–349. doi:10.2175/106143097x125542.
- Agunwamba, J. C. , Anyanwu, S. C. , Owhondah, C. O. , & Raji, A. M. (2008). The effect of roughness on dispersion. International Journal of Science and Technology Research , 5 (1–2), 202–210.
- Agunwamba, J. C. , Maduka, C. N. , & Ofosaren, A. M. (2007). Analysis of pollution status of Amadi Creek and its management. Journal of Water Supply: Research and Technology-AQUA , 55 (6), 427–435.
- Breusch, T. S. , & Pagan, A. R. (1979). A simple test for heteroscedasticity and random coefficient variation. Econometrica , 47 , 1287–1294.10.2307/1911963
- Box, G. E. P. , Jenkins, G. M. , & Reinsel, G. C. (1994). Time series analysis: Forecasting and control (3rd ed.). Englewood Cliffs, NJ: Prentice Hall.
- Chu, K. , Hua, Z. , & Ji, L. (2014). Aeration at overflow dams with curved surfaces by different flashboard spillway. Journal of Environmental Engineering and Landscape Management , 22 (3), 226–236.10.3846/16486897.2014.901226
- Costa, A. F. B. , & Castagliola, P. (2011). Effect of measurement error and autocorrelation on the X¯ chart. Journal of Applied Statistics , 38 (4), 661–673.10.1080/02664760903563627
- Dong, C. , Zhu, J. , & Miller, C. F. (2009). Evaluation of six aerator modules built on venturi air injectors using clean water test. Water Science & Technology , 60 (5), 1353–1359.https://doi.org/10.2166/wst.2009.420 10.2166/wst.2009.420
- Ezeilo, F. E. , & Dune, K. K. (2012). Effect of environmental pollution on a receiving water bodies: A cade study of Amadi Creek, Port-Harcourt, Nigeria. Transnational Journal of Science and Technology , 2 (8), 30–42.
- Facchini, A. , Mocenni, C. , Marwan, N. , Vicino, A. , & Tiezzi, E. (2007). Nonlinear time series analysis of dissolved oxygen in the Orbetello Lagoon (Italy). Ecological Modelling , 203 (3–4), 339–348. doi:10.1016/j.ecolmodel.2006.12.001
- Field, A. P. (2009). Discovering statistics using SPSS: And sex and drugs and rock ‘n’ roll (3rd ed.). London: Sage.
- Granger, C. , & Newbold, P. (1974). Spurious regressions in econometrics. Journal of Econometrics , 2 (2), 111–120. doi:10.1016/0304-4076(74)90034-7
- Harvey, A. C. (1990). The econometric analysis of time series (2nd ed.). Cambridge, MA: MIT Press.
- Hutcheson, G. D. (2011). Ordinary least-square regression. The Sage dictionary of Quantitative Management Research , 224–228.
- Johansen, S. (1988). Statistical analysis of co-integrating vectors. Journal of Economic Dynamics and Control , 12 , 231–254.10.1016/0165-1889(88)90041-3
- Jha, R. , Ojha, C. S. P. , & Bhatia, K. K. S. (2001). Refinement of predictive reaeration equations for a typical India river. Hydrological Processes , 15 (6), 1047–1060.
- Kumar, A. , Moulick, S. , & Mal, B. C. (2010). Performance evaluation of propeller-aspirator-pump aerator. Aquacultural Engineering , 42 (2), 70–74. doi:10.1016/j.aquaeng.2009.12.001
- Langbein, W. B. , & Dururn, W. H. (1967). The aeration capacity of streams . Circular S42. Reston: U.S. Geological Survey.
- Lin, S. D. , & Lee, C. C. (2007). Water and wastewater calculations manual . New York, NY : McGraw Hill Companies.
- MacKinnon, J. G. , Haug, A. A. , & Michelis, L. (1999). Numerical distribution functions of likelihood ratio tests for cointegration. Journal of Applied Econometrics , 14 , 563–577.10.1002/(ISSN)1099-1255
- Martin, N. , McEachern, P. , Yu, T. , & Zhu, D. Z. (2013). Model development for prediction and mitigation of dissolved oxygen sags in the Athabasca River, Canada. Science of the Total Environment , 443 , 403–412. doi:10.1016/j.scitotenv.2012.10.030
- Moulick, S. , & Mal, B. C. (2009). Performance evaluation of doublehub paddle wheel aerator. Journal of Environmental Engineering , 135 (7), 562–566.10.1061/(ASCE)0733-9372(2009)135:7(562)
- Olukanni, D. O. , Adebayo, R. A. , & Tenebe, I. T. (2014). Assessment of urban drainage and sanitation challenges in Nigeria. International Journal of Emerging Technology and Advanced Engineering , 4 , 100–105.
- Omole, D. O. (2011). Reaeration coefficient modeling: Case study of River Atuwara, Ota, Nigeria . Saarbrücken: LAP Lambert Academic.
- Omole, D. O. (2012). Composite goodness of fit in reaeration coeffcient modeling. Environment and Natural Resources Research , 2 (3), 71–83.
- Omole, D. O. , & Longe, E. O. (2012). Reaeration coefficient modeling: A case study of River Atuwara in Nigeria. Research Journal of Applied Sciences Engineering and Technology , 4 (10), 1237–1243.
- Omole, D. O. , Longe, E. O. , & Musa, A. G. (2012). An approach to reaeration coefficient modeling in local surface water quality monitoring. Environmental Modeling & Assessment , 18 (1), 85–94. doi:10.1007/s10666-012-9328-0
- Owens, M. , Edwards, R. W. , & Gibbs, J. W. (1964). Some reaeration studies in Streams. International Journal of Air and Water Pollution , 8 , 469–486.
- Owusu, P. A , Sarkodie, S. A. , Ameyo, P. (2016). A review of Ghana’s water resource management and the future prospect. Cogent Engineering , 3 , 1164275. https://doi.org/10.1080/23311916.2016.1164275 10.1080/23311916.2016.1164275
- Parajuli, R. , Chang, S. J. , & Hill, R. C. (2015). How effective is the United States-Canada softwood Lumber agreement 2006? An econometric study Forest Science , 61 (6), 1041–1049. doi:10.5849/forsci.15-014
- Ramanathan, R. (1992). Introductory econometrics with applications (2nd ed.). Harcourt New York: Brace Jovanovich.
- Streeter, H. W. , & Phelps, E. B. (1925). A study of the pollution and natural purification of the Ohio River . Public Health Bulletin No 146. Washington, DC: Public Health Service.
- Tenebe, I. T. , Emenike, P. C , Omole, D. O , Nkpa M. O. , Aikuola, A. O. , & Omeje U. A. (2017). Predicting degradation with biochemical oxygen demand in disinfectant-polluted sewage. Wessex Institute Conference on Water & Society 2017, 5th–7th June 2017, Seville, Spain.
- Tenebe, I. T. , Ogbiye, A. S. , Omole, D. O. , & Emenike, P. C. (2016). Estimation of longitudinal dispersion co-efficient: A review. Cogent Engineering , 3 (1), 1216244. doi:10.1080/23311916.2016.1216244
- Tenebe, I. T. , Ogbiye, A. S. , Omole, D. O. , & Emenike, P. C. (2017). Modelling and sensitivity analysis of varying roughness effect on dispersion coefficient: A laboratory study. Desalination and Water Treatment , 87 , 1–7. doi:10.5004/dwt.2017.21298
- Ugbebor, J. N. , Agunwamba, J. C. , & Amah, V. E. (2012). Determination of reaeration coefficient K2 for polluted stream as a function of depth, hydraulic radius, temperature and velocity. Nigerian Journal of Technology (NIJOTECH) , 31 (2), 174-180.