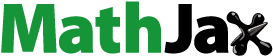
Abstract
This paper was aimed at using an adaptive control to develop a backstepping method for a special class of nonlinear systems, which requires no information on the upper bound of parametric uncertainty or disturbance. In this research, a novel control approach, including a developed backstepping method and an adaptive controlling method, is introduced as an adaptive generalized backstepping method (AGBM). Compared to the standard backstepping method, AGBM is far more efficient because the standard method is applicable in strictly feedback systems, but AGBM expands this class of systems. Another advantage of AGBM is that it can be applied to a special class of nonlinear systems with unmatched uncertainty as well as unknown upper bound disturbance. In this method, an adaptive controlling method is used to compensate uncertainty and parametric uncertainty besides stabilizing the controller against disturbances applied to the system. Finally, numerical simulation results demonstrate the advantages and feasibility of the presented algorithm, showing that the AGBM controller guarantees robustness and system stability in the presence of disturbance and uncertainty.
PUBLIC INTEREST STATEMENT
This paper focuses on using an adaptive control to develop a backstepping method for a special class of nonlinear systems, which does not require any information about the upper bound of parametric uncertainty or disturbance. In this research, a novel control approach (including the developed backstepping method and the adaptive controlling method) is introduced as the adaptive generalized backstepping method (AGBM). Compared to the standard backstepping method, AGBM is far more efficient because the standard method is applicable in strictly feedback systems, but AGBM expands this class of systems. Another advantage of AGBM is that it can be applied to a special class of nonlinear systems with unmatched uncertainty as well as with unknown upper bound disturbance. This control method tends to stabilize the compressor system under surge instability.
1. Introduction
The nonlinear control community has paid much attention to the adaptive control of nonlinear systems with parametric uncertainty (Isidori, Citation1995; Khalil, Citation2002; Krstic, Kanellakopoulos, & Kokotovic, Citation1995; Niu, Ahn, Li, & Liu, Citation2017; Niu & Li, Citation2017). Nonlinear systems with unknown parameters were exposed to the limitations of growth rate for uncertainties and matching conditions (Kanellakopoulos, Kokotovic, & Marino, Citation1991; Sastry & Isidori, Citation1989), which can be linearized by adaptive controlled algorithms. The introduction of integrator backstepping design (Kanellakopoulos, Kokotovic, & Morse, Citation1991; Krstic et al., Citation1995; Seto, Annaswamy, & Baillieul, Citation1994) has removed these limitations. Advances in adaptive nonlinear control have shifted the focus toward the robust adaptive control problem for nonlinear systems with time-varying disturbances and un-modeled dynamics, which can be applied to many problems. Among these, adaptive backstepping is a widely used method (Ghanavati & Chakravarthy, Citation2015; Kanellakopoulos et al., Citation1991; Krstic et al., Citation1995). Adaptive backstepping control is a successful method to gain global stability and asymptotic tracking in a special class of nonlinear systems with parametric strict feedback (Kanellakopoulos et al., Citation1991). Some studies (Krstic et al., Citation1995; Qian & Lin, Citation2002) have discussed the adaptive controlling method in parametric strict feedback systems to generalize the backstepping control to a greater set of nonlinear systems. Local stability was achieved in these two research, and they proposed a number of adaptive methods for nonlinear systems with a triangular structure. The backstepping method has a major disadvantage – certain functions should be linear in unknown parameters (Zhang, Fidan, & Ioannou, Citation2003), and thus long and tiresome calculations are required to determine the regression matrices.
Recently, some studies have concentrated on robust adaptive control for a special class of nonlinear systems with uncertainties, including nonlinearly appearing parametric uncertainty, uncertain nonlinearity, and unmeasured input-to-state stable dynamics, to develop the scope of adaptive control design for nonlinear uncertain systems (Jiang & Hill, Citation1999; Jiang & Praly, Citation1998; Plycarpou & Ioannou, Citation1995). A robust adaptive nonlinear control algorithm for nonlinear systems has been proposed in Plycarpou & Ioannou (Citation1995), including both parametric uncertainty and unknown nonlinearity. It has been assumed in this study that the unknown functions address the conditions of the triangular structure. This study can be extended to the scope of universal adaptive stabilization methods for a greater group of uncertain systems. Jiang & Hill (Citation1999) and Jiang & Praly (Citation1998) have presented a robust control algorithm for disturbed strict feedback nonlinear systems exposed to nonlinear parametric uncertainty, uncertain nonlinearity, and un-modeled dynamics. Some robust adaptive controlling methods have been discussed in Jiang & Hill (Citation1999), Jiang & Praly (Citation1998), and Plycarpou & Ioannou (Citation1995) that can guarantee the uniform ultimate boundedness of closed-loop system signals. Ghanavati & Chakravarthy (Citation2017) and Yao & Tomizukai (Citation1997) combined the backstepping adaptive technique and formal deterministic robust control to produce an adaptive robust control method. The nonlinear systems discussed in Jiang & Hill (Citation1999), Jiang & Praly (Citation1998), and Plycarpou & Ioannou (Citation1995) share some characteristics. For example, system uncertainties have a linearly parameterized form and system virtual control coefficients are considered equal to one. An adaptive robust controller using the backstepping method was discussed in Sheng, Huang, Zhang, & Huang (Citation2014), in which a fuzzy system is used to model unknown system characteristics. However, the main disadvantage of all the above-mentioned controllers is that the designer needs information on system characteristics and/or the disturbance bounds. In practical systems, information on system characteristics, uncertainty, and unknown upper bound is sometimes incomplete and there is no detailed information about them to design a controller. Thus, robustness of the controlled system can cope with the above-mentioned problems.
Inspired by the generalized backstepping method, Sahab & Zarif (Citation2009) aimed at stabilizing and tracking a special class of autonomous nonlinear control systems. However, there is no constraint on the input and state variables so the present paper tries to address this issue. Therefore, this study improves the findings of Sahab & Zarif (Citation2009) in order to design a special class of nonlinear systems where no information on the upper bound of uncertainties and disturbances is required. The method (AGBM) proposed in this paper has two major advantages over the adaptive robust backstepping method with regulation functions (Yao, Citation2003; Zhang, Lu, & Xu, Citation2012). On the one hand, theoretically, this method guarantees the asymptotic stability of the state variables and, on the other hand, AGBM has a higher efficiency over other methods (it can be employed in a special group of nonlinear systems with unmatched uncertainty and unknown upper bound). A numerical simulation was conducted to demonstrate the advantages and feasibility of the presented algorithm. Results of the simulation show that the AGBM controller guarantees robustness and, at the same time, can control surge in serial compressors with disturbance and uncertainty. In the conclusion section, it can be seen that the proposed method has a higher performance in comparison with the improved backstepping method employed in Sahab & Zarif (Citation2009) and Sheng et al. (Citation2014).
Compared to the above-mentioned results, content obtained from this paper indicates that AGBM has several advantages, such as guaranteeing asymptotic stability of the state variables and capability of a special class of nonlinear systems with unmatched uncertainty and unknown upper bound. A numerical simulation was conducted to demonstrate the advantages and achievability of the presented algorithm. Simulation results show that the AGBM controller guarantees robustness and, at the same time, it is able to control surge in serial compressors with disturbance and uncertainty.
The rest of the paper is organized as follows. Section 2 presents the process of generalizing the robust backstepping method for a special group of nonlinear systems with unmatched uncertainty, along with the related assumptions. In Section 3, AGBM is employed in designing a surge controller for serial compressors with flow and pressure disturbance. Section 4 presents the simulation results and further discussions. Finally, Section 5 presents our conclusions. A summary of the notations is presented in Table .
Table 1. Dimensional and nondimensional variables (Uddin & Gravdahl, Citation2012 )
1.1. Nomenclature
2. Problem formulation and proving AGBM
Sahab & Zarif (Citation2009) used the extended backstepping method for a special class of systems, which is bigger than the normal backstepping method. This class of nonlinear systems is
where f, g, F, and G denote known functions and is the control input. Moreover,
.
To develop a new method using the approaches employed in Sahab & Zarif (Citation2009) and adaptive control, which can be applied to a special class of nonlinear systems with unmatched uncertainty and unknown upper bound, this paper has considered the nonlinear systems as follows:
where are known functions,
are unknown functions, and
are upper unknown bounded.
indicates the control inputs. Moreover, we have:
and
. In addition, it is considered that
for all
, in relation to
they are piecewise continuous and in relation to
they are locally Lipschitz. Furthermore,
is uniformly bounded for all
, and
is uniformly bonded for all
.
Assuming the positive definite function with a derivative negative definite as follows:
where and
are the estimation parameters of
and
is a regulating parameter, we have
By selecting
we have
To negate the derivative of Lyapunov function for stability, the adaptive law is defined as follows:
According to Sheng et al. (Citation2014)
We have
where denotes a positive definite function and
are states feedback control law. Equation (2) can be rewritten as follows:
Then, if we use the following inverse variation, we obtain:
Having rewritten (10), we obtain
Next, the Lyapunov function mentioned in (2) is chosen as follows:
This function is a positive definite function. Therefore, the only thing to do is to specify whether its derivative is negative definite. We differentiated Equation (14) in relation to time to reach the following function:
Having (9) in mind, we can rewrite (15) in a simpler way:
Then, the control inputs, , can be regarded as
where denotes the virtual control input and
. Having applied the control inputs in (17), we are able to rewrite (16) as
To negate the derivative of the Lyapunov function for stability, the parameter of the adaptive law is presented as follows:
Considering (8), we have
At this moment, assuming (21), one can search for the virtual control variables,
By choosing
Then we have
where denotes the upper unknown bound on
. In this equation,
Equation (23) reaches the following maximum:
Thus, it is obtained that
Because belongs to class
, it is always true that
is negative outside some ball, and according to Lemma 14.1 from Khalil (Citation2002), which addresses the principle of designing a nominal controller at first and then the corrective term controller (using the same nominal Lyapunov function) to a matched uncertainty term with desirable stability properties, the solution of the closed-loop system is uniformly bounded for any initial state
.
3. An illustrated example
In this section, a developed backstepping controller is designed, which requires no information on disturbance upper bound or parametric uncertainty and throttle gain and throttle valve feature. Thus, we showed the adaptive of the controlled system to cope with the time-varying flow and pressure disturbances. Moor and Greitzer (Gravdahl & Egeland, Citation1997; Moor & Greitzer, Citation1986) provided a dynamic model for a centrifugal compressor. As it can be seen in Figure , this dynamic model (MG-Model) consists of a gas compressor, a pressure tank, a close coupled valve (CCV), a throttle valve, and the inlet and exit ducts.
Figure 1. The generalized compressor system of the MG-model (Gravdahl & Egeland, Citation1997).
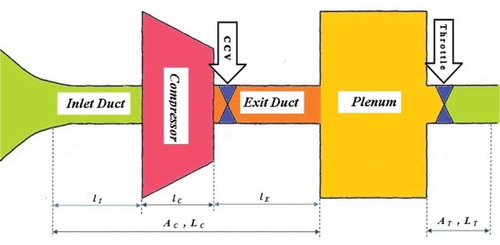
The equations of the modes of three serial compressors description, shown in Figure , are presented as follows:
By choosing
Given Gravdahl & Egeland (Citation1999), the following is obtained:
Equation (27) can be rewritten as
Then, by choosing
Given Equation (12), the overall Lyapunov function is chosen as
Thus, it can be observed that this equation is a positive definite function. To stabilize the overall system, the only thing to do is to have a negative Lyapunov equation derivative.
The virtual control vectors and i = 1, 2, 3 are chosen as
We obtain:
By choosing
We have
By selecting
Finally, we have
Given by Eq. (2.45) from Gravdahl & Egeland (Citation1999), it is supposed that the throttle is passive, i.e. . We can obtain
And given in Lemma 2.26 from Krstic et al. (Citation1995), which expresses the closed-loop system ISS stability with respect to disturbance input and global uniform boundedness condition, it can be concluded that is negative whenever
.
4. Simulation results and discussions
In this paper, we applied the above-mentioned AGBM controller in three serial compressors, which are shown in Figure . It was conducted using MATLAB Software. The MG mfodel was employed to model the compressors and they all were equipped with a CCV (Gravdahl & Egeland, Citation1997).
Figure 2. Arrangement of three serial compressors (Menon, Citation2005).
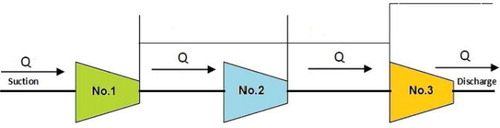
Compressors have similar parameters, and based on Greitzer (Citation1976), they are assumed to be
Based on Gravdahl & Egeland (Citation1999), flow disturbance can be a result of the compressor upstream processes or their serial pattern. Therefore, in the simulations presented in this section, the impact resulting from the serial pattern of compressors is denoted as flow disturbance in the equations. A comparison was made to make our investigation more accurate on the above-mentioned controller and to reach a deeper understanding of the controller performance in different states. This comparison is between the output pressure diagram and the compressor output flow diagram before and after the use of controlling force. Despite the fact that there is no information on the parametric uncertainty upper bound in this research, it can be seen that the designed controller performance with AGBM is robust and more effective in all investigated states compared with the extended backstepping method presented in Sahab & Zarif (Citation2009). The approach employed by them, unlike our model, needs information about nonlinear uncertain systems under matching conditions and it can be easily seen that this model is not robust to bounded uncertainties.
There are several applications (Ghanavati, Salahshoor, Jahed Motlagh, Ramezani, & Moarefianpour, Citation2017; Sheng et al., Citation2014; Yao, Deng, & Jiao, Citation2015; Yao, Jiao, & Ma, Citation2015) that can be used for the controller to demonstrate the effectiveness of our method. In this section, we consider the system in Sheng et al. (Citation2014), and our goal is to compare the method presented in this paper with the existing backstepping controllers. In the first step, the system is simulated with no control input. Then, in compressor 1, at t = 100 s, in compressor 2, at t = 120 s, and in compressor 3, at t = 150 s, the throttle valve gain coefficient, , was reduced from 0.65 to 0.6. The flow and pressure outputs for compressors 1–3 are shown in Figure .
The results of simulation on the uncontrolled system are presented in Figure . It can be observed in the figures that when the throttle gain is reduced from 0.65 to 0.6, the compression system becomes unstable. The simulation results clearly show the transformation of the compressor’s operating range and its entrance into the surge instability area, which creates a limit cycle. Figures – show the control signals. As it can be seen, this signal is positive and follows the actuator restriction (valve capacity requires the constraint 0 ≤ u(t) ≤ 1 to hold), whereas the control signal derived from Sheng et al. (Citation2014) is negative. Figures – show the status of output flow studied by applying the control signal through the AGBM controller. The pressure outputs for compressors 1–3 using the AGBM controller are shown in Figures –, respectively.
As it can be seen in Figures –, the flow and pressure for compressors 1, 2, and 3 were stabilized by using the designed AGBM controller and the system was controlled in the presence of disturbance while the equilibrium point is located in the unstable area. The AGBM controller, as derived in Section 2, can damp the unstable modes as the operation of the compression system is pushed into the surge area by restricting the flow through the throttle valve. Given that the control signal is applied to the CCV actuator, it cannot take a negative value and this is an advantage of this research and the designed controller, whereas the control signal from Sheng et al. (Citation2014) is negative and physically impractical. In addition, the controller presented in Sahab & Zarif (Citation2009) cannot control any of these compressors by applying the control signal using the method of Sahab & Zarif (Citation2009) and all three compressors studied in this research enter the surge area and become unstable. Because Sahab’s work is for systems without disturbance, disturbed nonlinear systems are considered in this article. It is clear that Sahab’s method does not respond to this class of systems.
5. Conclusion
The simulation results indicate that AGBM can present the outputs with a high precision. It can be easily seen in the simulation figures of this paper that the AGBM controller can stabilize three serial compressors (with flow and pressure disturbances) with a high precision. The AGBM controller can retain its high performance in parametric uncertainty and unmatched disturbance conditions. Because of the minimum level of error and better tracking in the presented method, when there is unmatched uncertainty and unknown upper bound disturbance, AGBM is more appropriate for a special class of nonlinear systems than the extended backstepping method. Additionally, unlike the standard backstepping method, there was no instability in the results obtained by simulation. Thus, it can be said that the AGBM proposed in this study is more robust to bounded uncertainties, which can be considered as another advantage of AGBM. Finally, it should be noted that AGBM provides accurate outputs for nonlinear systems with a strictly feedback form, no matter if there is a bounded uncertainty or disturbance.
Competing interests
The authors declare that there is no conflict of interest.
Acknowledgments
The authors would like to thank the referees for their constructive comments.
Additional information
Funding
Notes on contributors
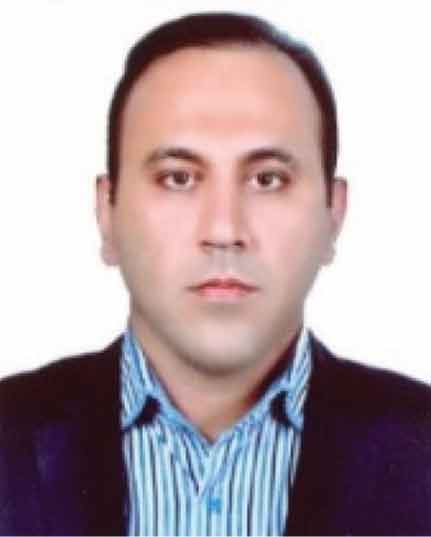
Malek Ghanavati
Malek Ghanavati received his BSc in Electrical Power, 2001, and his MSc degree in 2006, Iran. In 2017, he received his PhD in Control and System Engineering, Science and Research branch, Islamic Azad University, Iran. His research interests include nonlinear control and process control.
Karim Salahshoor
Karim Salahshoor is a professor at the Department of Automation and Instrumentation, Petroleum University of Technology, Iran. His research interests include intelligent control, advanced process control, and intelligent monitoring.
Mohammad Reza Jahed-Motlagh
Mohammad Reza Jahed Motlagh is a professor at the Department of Electrical Engineering, Iran University of Science and Technology, Iran. His research interests include control engineering, nonlinear system, and artificial intelligence.
Amin Ramezani
Amin Ramezani is an assistant professor at the Department of Electrical Engineering, Tarbiat Modares University, Iran. His research interests include process control and automation, discrete event systems, and stochastic process control.
Ali Moarefianpur
Ali Moarefianpour is an assistant professor of Electrical Engineering Department, Science and Research branch, Islamic Azad University, Iran. His research interests are convex optimization, industrial control, and artificial intelligence.
References
- Ghanavati, M. , & Chakravarthy, A. (2015). Demand-side energy management using an adaptive control strategy for aggregate thermostatic loads. In AIAA conference, January 5-9, (pp. 1–7). Kissimmee: Scitech.
- Ghanavati, M. , & Chakravarthy, A. (2017). Demand-side energy management by use of a design-then-approximate controller for aggregated thermostatic loads. IEEE Transactions on Control Systems Technology , 1833–1838. doi:10.1109/TCST.2017.2705157
- Ghanavati, M. , Salahshoor, K. , Jahed Motlagh, M. R. , Ramezani, A. , & Moarefianpour, A. (2017). A novel robust generalized backstepping controlling method for a class of nonlinear systems. Cogent Engineering , 4, 1342309, 1–17. doi:10.1080/23311916.2017.1342309
- Gravdahl, J. T. , & Egeland, O. (1997). Compressor surge control using a close-coupled valve and backstepping. In Proceedings of the American control conference, June 4-6, (pp. 2398–2406). Albuquerque.
- Gravdahl, J. T. , & Egeland, O. (1999). Compressor surge and rotating stall: Modeling and control . London: Springer Verlag. ISBN-13: 978-1-4471-1211-2.
- Greitzer, E. M. (1976). Surge and rotating stall in axial flow compressors. Part I: Theoretical compression system model. ASME Journal of Engineering for Power , 98(2), 191–198.
- Isidori, A. . (1995). Nonlinear control systems . Berlin: Springer-Verlag.
- Jiang, Z. , & Hill, D. J. (1999). A robust adaptive backstepping scheme for nonlinear systems with unmodeled dynamics. IEEE Transactions on Automatic Control , 44, 1705–1711. doi:10.1109/9.788536
- Jiang, Z. , & Praly, L. (1998). Design of robust adaptive controllers for nonlinear systems with dynamic uncertainties. Automatica , 34, 825–840. doi:10.1016/S0005-1098(98)00018-1
- Kanellakopoulos, I. , Kokotovic, P. , & Morse, A. (1991). Systematic design of adaptive controllers for feedback linearizable systems. IEEE Transactions on Automatic Control , 36, 1241–1253. doi:10.1109/9.100933
- Kanellakopoulos, I. , Kokotovic, P. V. , & Marino, R. (1991). An extended direct scheme for robust adaptive nonlinear control. Automatica , 27, 247–255. doi:10.1016/0005-1098(91)90075-D
- Khalil, H. K. (2002). Nonlinear systems (3rd ed.). Upper Saddle River, NJ: Prentice Hall.
- Krstic, M. , Kanellakopoulos, I. , & Kokotovic, P. (1995). Nonlinear and adaptive control design . New York, NY: Wiley.
- Menon, E. (2005). Gas pipeline hydraulics .
- Moor, F. K. , & Greitzer, E. M. (1986). A theory of post-stall transients in axial compression systems: Part I—Development of equations. Journal of Engineering for Gas Turbines and Power , 108, 68–76. doi:10.1115/1.3239887
- Niu, B. , Ahn, C. , Li, H. , & Liu, M. (2017). Adaptive control for stochastic switched non-lower triangular nonlinear systems and its application to one-link manipulator. IEEE Transactions on Systems, Man, and Cybernetics: Systems , 1–15.
- Niu, B. , & Li, L. (2017). Adaptive backstepping-based neural tracking control for MIMO nonlinear switched systems subject to input delays. IEEE Transactions on Neural Networks and Learning Systems , 29(6), 1–7.
- Plycarpou, M. M. , & Ioannou, P. (1995). A robust adaptive nonlinear control design. Automatica , 31, 423–427.
- Qian, C. , & Lin, W. (2002). Output feedback control of a class of nonlinear systems: A non-separation principle paradigm. IEEE Transactions on Automatic Control , 47(10), 1710–1715. doi:10.1109/TAC.2002.803542
- Sahab, A. , & Zarif, M. (2009). Improve backstepping method to GBM. World Applied Sciences Journal , 6(10), 1399–1403.
- Sastry, S. , & Isidori, A. (1989). Adaptive control of linearizable systems. IEEE Transactions on Automatic Control , 34, 1123–1131. doi:10.1109/9.40741
- Seto, D. , Annaswamy, A. , & Baillieul, J. (1994). Adaptive control of nonlinear systems with a triangular structure. IEEE Transactions on Automatic Control , 39, 1411–1428. doi:10.1109/9.299624
- Sheng, H. , Huang, W. , Zhang, T. , & Huang, X. (2014). Robust adaptive fuzzy control of compressor surge using backstepping. Arabian Journal for Science and Engineering , 39, 9301–9308. doi:10.1007/s13369-014-1448-1
- Uddin, N. , & Gravdahl, J. T. (2012). A compressor surge control system: Combination active surge control system and surge avoidance system . ISUAAAT Scientific Committee with JSASS Publication. Chuo-ku, Tokyo.
- Yao, B. (2003). Integrated direct/indirect adaptive robust control of SISO nonlinear systems in semi-strict feedback form. American Control Conference , 4, 1320–1325.
- Yao, B. , & Tomizukai, M. (1997). Adaptive robust control of SISO nonlinear systems in a semi-strict feedback form. Automatica , 33(5), 893–900. doi:10.1016/S0005-1098(96)00222-1
- Yao, J. , Deng, W. , & Jiao, Z. (2015). Adaptive control of hydraulic actuators with LuGre model based friction compensation. IEEE Transactions on Industrial Electronics , 62, 6469–6477. doi:10.1109/TIE.2015.2423660
- Yao, J. , Jiao, Z. , & Ma, D. (2015). A practical nonlinear adaptive control of hydraulic servomechanisms with periodic-like disturbances. IEEE/ASME Transactions on Mechatronics , 20, 2752–2760.
- Zhang, Y. , Fidan, B. , & Ioannou, P. A. (2003). Backstepping control of linear time-varying systems with known and unknown parameters. IEEE Transactions on Automatic Control , 48(11), 1908–1925. doi:10.1109/TAC.2003.819074
- Zhang, Z. , Lu, J. , & Xu, S. (2012). Tuning functions-based robust adaptive tracking control of a class of nonlinear systems with time delays. International Journal of Robust and Nonlinear Control , 22(14), 1631–1646. doi:10.1002/rnc.v22.14