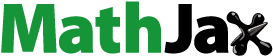
Abstract
Production from thin oil rim reservoirs can be very challenging due to the thin spread of the oil resources, complicated mechanism of production and fluid contact movement. Typically, the recovery from these types of reservoirs is usually low thereby making them economically unattractive. The objective of this present study is to evaluate the optimal well design for improved recovery from thin oil rim reservoirs. A surrogate modelling approach was deployed for evaluating three different development strategies. Numerical reservoir simulations were conducted to define the basis for the surrogate modelling. In all these strategies, the rim height, reservoir anisotropy, oil viscosity, horizontal permeability, bottom-hole pressure (BHP) and horizontal well length were considered as uncertainty. The selection of the best strategy was based on cumulative hydrocarbon recovery after 30 years of simulation. Uncertainty quantification was achieved using regular Monte Carlo Simulation. Management of a wide range of subsurface uncertainties was considered. The results showed that placing the well just above the oil-water contact (OWC) allowed more oil recovery compared to other strategies considered in this study. The results derived from surrogate model predictions compared favourably with those observed from the YADD thin oil rim reservoirs located in the Niger Delta. The methodology adopted saves time and is reproducible where oil rim development is desirable.
PUBLIC INTEREST STATEMENT
Oil Rim Reservoir production is generally considered to be uneconomical because of the low oil production rates and high water/gas production. This low recovery occurs as a result of the fluid contact movement. In a bid to improve production from these types of reservoirs and the complicated nature of the production mechanism a study of the parameters affecting fluid flow is carried out. An evaluation of three development strategies is done using a surrogate modelling approach. The use of modern experimental design methods such as uniform design as demonstrated in this study offers reliable proxy modelling for uncertainty quantification Well placement just above the oil-water contact gave the highest recovery. The results from the surrogate model predictions were validated with results obtained from a Niger Delta Oil Rim Reservoir.
1. Introduction
The goal of an oil field development project is the acceleration of the hydrocarbon production and maximization of oil recovery at a minimal cost. Thin oil reservoirs with a huge gas cap are common in the Niger Delta sedimentary basin. Despite having thin pay thickness, these reservoirs still contain substantial volumes of oil-in-place. There are many technical and non-technical issues/challenges associated with optimal oil rim development. With increasing knowledge of reservoir management and improving production technologies, more complex reservoirs (with respect to location, formation heterogeneities and dimension) can be explored and produced, one of such is the thin oil rim reservoir. Oil recovery in these thin oil rim reservoirs is often difficult because coning or cresting of unwanted fluids is inevitable, leading to low rates of recovery.
There are several sub-surface factors affecting the recovery process of oil rim reservoirs. The subsurface factors quoted in different studies and field applications are; oil column size, gas cap volumes/size, permeability, aquifer-strength and oil viscosity. These factors play important roles in determining the fluid flow dynamics and recovery factor for a given oil rim reservoir configuration. In addition, the impact of other subsurface and operational factors such as reservoir geometry (the presence of high permeability streaks, extensive shale breaks), degree of heterogeneity and magnitude of bed dip, well type and location and operating philosophy can also be significant.
Several strategies have been used for the development of oil rim overlain by a large gas cap. The conventional approach involves the use of horizontal wells, vertical wells, a combination of horizontal and vertical wells and use of water injectors and oil production wells. However, the optimal well design with respect to orientation, vertical location trajectory, production rate and horizontal well length is critical for the attainment of significant economic benefit especially for offshore projects (Yang & Wattenbarger, Citation1991). Kabir, Mckenzie, Cornell, and O’Sullivan (Citation1998) suggested the use of the gas injection technique for producing a narrow oil rim. This technique is not a new concept in the targeted oil field or elsewhere in the world. However, the incremental recovery of oil recorded in a narrow oil rim had not been recorded in the literature as at that time. The Kabir et al. (Citation1998) method provides direct displacement as well as pressure maintenance; helps target multiple layers in a single wellbore and reduces the sensitivity of the rim to distance from the fluid contact, unlike primary recovery. Onyeukwu, Peacock, and Matemilola (Citation2012) investigated the technical feasibility of gas and water injection to produce an oil rim reservoir under various subsurface uncertainties. The development involves the completion of horizontal gas injector well in the gas cap while the horizontal oil producer was completed in the oil rim. The results of the work show that simultaneous water and gas injection could increase oil recovery, an observation similar to the result of Kabir et al. (Citation1998) except that more recovery is achieved using water injection in cases where weak aquifer support is predominant. A study on two innovative concepts of smart completions for the control of water cresting in horizontal wells was done by Inikori and Wojtanowicz (Citation2002); that is, the use of tailpipe sink and bi-lateral water sink. This method involved production segregation of oil and water in a dual completion with zonal isolation. Their research work indicates that dual completions can reduce the amount of by-passed oil at the toe of the horizontal wells and recovery of about 7% was achieved using the bilateral water sink (BWS) while the tail-pipe water sink (TWS) was limited to drain holes and short radius horizontal wells. Chugbo, Roux, and Bosio (Citation1989) observed in their study that horizontal well technology for the development of thin oil rims in an unconsolidated sand deposit located in a deltaic environment may be erroneous, but no reports of further studies are available to validate their claim. Ehlig-Economides, Chan, and Spath (Citation1996) proposed that a dual horizontal well completion, one in the oil zone and the other in the water zone could reduce the water cresting problem in horizontal wells. No results were presented in that research to validate the proposition. Mohamed, Ezuka, and Ghasen (Citation2010) proposed a smart development strategy in a field case study of the IKU6 reservoir in the Ekeh field of the Niger Delta Basin. Their study proposed the use of an intelligent multilateral well to simultaneously produce oil and gas from the same well bore drilled through the thin oil rim with the large gas cap. Apart from being cost-effective relative to the conventional technique of drilling two wells, a reduction of excess production of unwanted fluids (water) was also recorded during oil and gas production using intelligent well technology. However, as beneficial as this technology has proven, its applicability is limited only to reservoirs with production and injection using horizontal wells that are completed and equipped with permanent downhole sensors and valves. This paper is aimed at evaluating the optimal well design to produce thin oil rim reservoirs. A surrogate modelling approach is used in this work to permit an understanding of the complexity of the fluid system and efficient analysis of the uncertainties associated with oil recovery from thin oil rim reservoirs.
2. Methodology
2.1. Modelling approach
Many oil rim reservoirs have been identified in the Niger Delta. These reservoirs are characterized by thin oil column (30–77 ft) with the thick gas cap. The development of these thin oil rim reservoirs is very challenging due to the complexity of the fluid system (volatile oil, retrograde condensate gas cap gas), complexity of the geology of the field (compartmentalization, fracturing, and rapid lithological variation), high heterogeneity in reservoir properties (permeability ranges from 100mD to 15,000 mD), and strong water drive which is responsible for the observed threat of high water cut.
To evaluate and select best development strategy for optimal production from these fields, this study proposes Surrogate Modeling Methodology (SMM) as an alternative to time-consuming and costly Full Field Simulation (FFS) of a horizontal well. The motivation is to build a fit-for-purpose surrogate model for efficient and rapid analysis of the uncertainties associated with the development of thin oil rim reservoirs.
2.2. Model description and grid size selection
The first step in developing any Surrogate Reservoir Model is to identify the full-field model characteristics that will be captured during the simulations. A synthetic three-dimensional numerical reservoir model capable of accurately mimicking the behaviour of full-field model with all its details and complexity was selected based on the historical field characteristics. Local grid refinement was done around the fluid contact out of the need to have a finer grid which helps in the tracking of the water and gas-front accurately and study its impact on oil recovery. Figure shows a schematic of the model used in this study.
The total daily oil production from this oil rim reservoir is reported as 1500 barrels per day. It is known that the field can produce more oil than what is reported. Furthermore, each well is capped at 800 barrels of liquid per day. Since water cut has been a problem observed in some wells, the production cap is imposed to avoid bypassing oil and creating hard-to-produce oil banks that are left behind. It was suspected that several wells in the field can produce more oil without the threat of high water cut and a carefully planned well placement program was desired; and this became the main objective of this project.
A sensitivity analysis was carried out on various grid systems by simulating production from the oil rim using four different grid sizes. Figure shows the evolution of water cut profile for different grid sizes. The grid size (Nx = 74;Ny = 35; Nz = 24) gives a longer breakthrough time as illustrated in Figure , but takes a longer simulation run time. Therefore, an optimum grid size of (Nx = 58; Ny = 28; Nz = 24) was selected based upon water breakthrough time (BTT), simulation run time and observed field production profiles.
Table lists the range of parameters obtained from different oil rim reservoirs in the Niger Delta. These parameters were used for the SMM development from the full-field dynamic model. The PVT analyses of the reservoir fluid used in this study showed light oil (API 40). The reservoir contained a big gas cap with an initial volume of gas-in-place of about 40 BSCF, and the estimated liquid reserve is about 45 MMSTB of oil which corresponds to the current recovery factor of 28%. It is desired to increase the recovery factor by evaluating the recovery performance considering horizontal well placement within the reservoir.
Table 1. Model uncertainty parameters and their range of values
2.3. Proposed development strategy
Generally, the configuration of thin oil reservoirs is usually described as doughnut or pancake. There have been numerous field examples of the dynamics and characteristics of these two reservoir configurations. The “doughnut” and “pancake” type schematic diagrams are shown in
Figure . Note that figures labelled a(i) and b(i) show the top-view of the doughnut and pancake type reservoirs, respectively; both indicating the positions of the fluid contacts while the figures labelled a(ii) and b(ii) are the frontal views of the doughnut and pancake type reservoirs, respectively. For the purpose of this work, the doughnut type reservoir was used for performance evaluation. A horizontal well was placed between the gas-oil-contact (GOC) and oil-water-contact (OWC) and allowed to produce for 30 years. This study proposes three placement options for the horizontal well in the oil column in order to exploit the residual oil column. These options include:
Figure 3. Idealized models of oil rim reservoirs (Lawal, Inewari, & Adenuga, Citation2010)
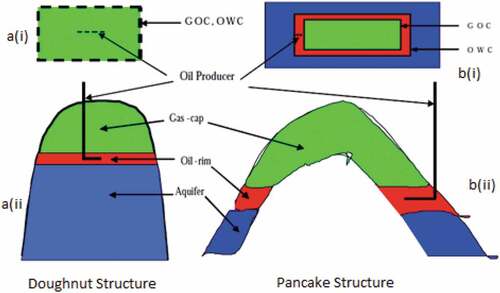
(i) Well placement near the gas-oil-contact (GOC).
(ii) Well placement near the oil-water-contact (OWC).
(iii) Well placement mid-way between the GOC and OWC (conventional)
2.3.1. Uncertainty screening
The regular two-level experimental design was deployed to identify “heavy hitters” associated with different reservoir development strategies. Using the parameters listed in Table , a total of 16 experiments were performed for each option implemented. The contribution of each uncertainty factor was estimated following the significant test for the regression used in the analysis of variance (ANOVA) for all the development strategies. The two hypotheses used for the test are as follows.
a. The null hypothesis: all treatments are of equal effect:
b. The alternative hypothesis: some treatment is of unequal effects:
To reject the null hypothesis H= 0 at least one of the variables explains significantly the variability observed on the response so that the model is valid. Figure shows the results obtained for all the options evaluated expressed as a Pareto chart. It was observed that the horizontal permeability, well lateral length, size of the oil rim, fluid viscosity and gas cap size have significant impacts on oil recovery after 20 years of simulation.
3. Results and discussion
3.1. Selection of best well placement strategy
The performance of horizontal well based on oil recovery at different oil rim heights after 10 years of simulation for the three placement strategies evaluated in the study is illustrated in Figure . It is observed that oil rim height presents significant uncertainty for oil recovery. The recovery from all the strategies increases with increasing oil rim height. However, placing the horizontal well near the OWC recorded higher oil recovery. Figure shows the sensitivity of horizontal permeability to the cumulative oil recovery for the different oil rim heights. Oil recovery increases with permeability for a fixed oil rim height. Therefore, horizontal permeability was observed to have a significant impact on cumulative production from our analysis.
To select best the well placement for the purpose of field development, 30-year simulations were run for a fixed permeability of 1000 mD and an oil rim height of 80 ft. The choice of 1000 mD is evident in Figure where a further increase of permeability from 1000mD to 2000 mD resulted in a fractional change in cumulative recovery for a mid-oil rim case. Figure shows the evolution of cumulative recovery for various placement strategies. It is evident that placing the horizontal well near the OWC shows highest recovery efficiency with about 24 MMSTB recoverable reserves relative to other strategies evaluated in this study.
3.2. Uncertainty quantification of well placement near the OWC
For practical applications in realistic field cases, the bottom-hole pressure (BHP) and vertical-to-horizontal permeability anisotropy ratio, Kv/Kh, were included in the uncertainty analysis. These two parameters were not significant according to the pareto chart of Figure . However, to develop the most robust surrogate model, there is a need to include them to avoid leaving some uncertain parameters behind. The overall increase in the number of experiments as a result of this inclusion was offset and taken care of by judicious selection of appropriate experimental design used for this task. The strength of the aquifer was found to be an important factor impacting oil recovery. The study considered two aquifer strengths—weak and strong based on a qualitative measure of the degree of pressure maintenance in the reservoir. Strong aquifers provide relatively higher pressure support.
3.3. Experimental design
The goal here is to select the best design without compromising the efficiency. A uniform experimental design was implemented by modifying Latin Hypercube design. The results of the simulations are shown in Tables and for weak and strong aquifers, respectively. Each of the variables employed in the simulations and the recovery for each experimental run are recorded in the Tables.
Table 2. Recovery under uncertainty (Weak aquifer case)
Table 3. Recovery under uncertainty (strong aquifer case)
3.4. Surrogate model development
Tables and are the ANOVA tables for both model and factor selected in the weak and strong aquifers. The model F-value of 369.23 and 7970.88 for weak and strong aquifer cases implies that the model is significant. There is only a 0.01%chance that a “model F0-value” this large could occur due to noise. Values of “Prob > F” less than 0.0500 indicates that the model terms are significant. In this work, the parameters labelled A, B, C, D, E, F and G are significant in both cases. Also, the interactions, labelled AB, AC, AD, AF, AG, BD, and ABD are significant in both cases at a 90% confidence limit. Equation 1 and Equation 2 are the final equations for the weak and strong aquifer systems, respectively. The correlation coefficients for the two model equations for the weak and strong aquifer systems are 0.998 and 1.00, respectively.
Table 4. Analysis of variance of cumulative recovery for oil rim model development (Weak Aquifer)
Table 5. Analysis of variance of cumulative recovery for oil rim model development (Strong Aquifer)
Figures and are parity plots for the two models. These graphs plot the predicted production forecast as a function of the experimental reserves. If the prediction method was a perfect fit of the experimental data, then all the points would lie on the x= y line.
The terms in EquationEquations 3.1(3.1)
(3.1) and Equation3.2
(3.2)
(3.2) are defined as:
A = height of the oil rim
B = horizontal permeability (Permx)
C = oil viscosity (Viscosity)
D = bottom-hole pressure (BHP)
E = horizontal-to-vertical permeability anisotropy ratio (Kv/Kh)
F = Length of the horizontal well (Well Length)
G = size of the gas cap (m)
AB = A × B
AC = A × C
AD = A × D
AF = A × F
AG = A × G
BD = B × D
ABD = A × B × D
3.4.1. Validation of response surface models
To assess the validity and accuracy of the response surface models, the estimates from Equationequations (3.1(3.1)
(3.1) ) and (Equation3.2
(3.2)
(3.2) ) were compared to the recovery from history-matched YAAD oil rim reservoirs in the Niger Delta. The YAAD oil rims had thicknesses ranging from 10–50 ft, horizontal permeability from 100 to 2000 mD, viscosity from 0.3 to 1.3 cp, oil gravity from 20° to 40°API, gas cap size, m-factor from 0.2 to 5 (dimensionless); and aquifer strength ranging from 1 to 100. Figure shows the comparison of the actual field oil recovery with the model prediction. The history-matched model of YAAD was used for the forecast after 30 years under the following conditions: H = 80 ft, Permx = 1500 mD, viscosity = 2 cp, BHP = 1200 psi, kv/kh = 0.070, well horizontal length = 800 m and gas cap size of 4.0. Figure shows that the developed models captured the field oil recovery very well and can be used to provide information to support proper management of the field.
3.5. Monte carlo simulation
The Monte Carlo technique (Hammersley & Handscomb, Citation1983) was used to combine the uncertain attributes and to generate values for model input variables. One million iterations were made while assuming the uniform distribution functions for the input parameters. The risk curves generated were in terms of cumulative oil production.
Cumulative probability distribution and sensitivity of different parameters on the forecast reserves are shown in Figure . The impact of the major uncertain parameters for the two aquifer systems was quantified. It clearly shows that the horizontal well length is the dominant factor in both aquifer cases. The spread in production profiles (P10–P90 range) from the deterministic forecast volume (P50) from the two aquifer cases is shown in Table which indicates the uncertainty in the forecast. The extreme quantities to a large extent are investment indicators; these govern the decision on whether to develop the oil rim and how to develop it.
Table 6. Production Forecast distribution
4. Conclusions
The following conclusions can be drawn from this study.
This study showed that numerical simulation using surrogate models is a cost-effective alternative to full-field simulation for the evaluation of thin oil rim reservoir development. Selection and optimal placement of wells within oil rim reservoirs are critical for reservoir development and management. Placing the well just above the oil-water contact offers more economic value compared to other placement strategies considered in this study. The use of modern experimental design methods such as uniform design as demonstrated in this study can offer more reliable proxy modelling for uncertainty quantification. It is shown that horizontal permeability is the dominant reservoir factor in recovery from thin column reservoirs in the short term. However, in the long term, the lateral length and reservoir anisotropy exhibited greater influence on the reserve forecast. This impact of the uncertainty on the forecast reserves was demonstrated using the Monte Carlo simulation. The posterior summaries of the parameters alongside their uncertainties given by P0, P10, P50, P70, and P90 quartiles were obtained. The results show that the aquifer strength plays an important role in oil recovery from thin column reservoirs. The approach used in this study and uncertainty analysis can serve as a framework for evaluating similar oil rim reservoirs.
Acknowledgements
The authors acknowledged the use of the Schlumberger software at the African University of Science and Technology (AUST) for the simulations.
Additional information
Funding
Notes on contributors
Yetunde M. Aladeitan
Yetunde M. Aladeitan is a lecturer at the department of Chemical Engineering at the University of Abuja in Nigeria and a PhD student of petroleum engineering at the African University of Science and Technology Abuja. Her area of specialization in reservoir simulation and modelling.
Akeem O. Arinkoola
Akeem O. Arinkoola holds a PhD in Petroleum engineering and lectures at the Ladoke Akintola University of Technology, his areas of research interest are uncertainty analysis and reservoir modelling.
Okhiria D. Udebhulu
Okhiria D. Udebhulu a PhD student of petroleum engineering at the Department of Mineral Engineering, Universidade de Sao Paulo, Sao Paulo-SP Brazil, his research area are in Petroleum Production Engineering.
David O. Ogbe
David O. Ogbe is a visiting Professor of petroleum engineering at the African University of Science and Technology Abuja. He holds a PhD in petroleum Engineering rom the prestigious Stanford University in California. His research interests includes reservoir simulation, reservoir modelling, formation evaluation, oil and gas reservoir engineering, well testing, Reservoir modelling and simulation and reservoir characterization.
References
- Chugbo, A. I., Roux, G. D., & Bosio, J. C. (1989). Thin oil columns: Most people think horizontal wells, Obagi field case suggest the contrary. Paper SPE 19599, Society of Petroleum Engineers. doi:10.2118/SPE-19599-MS
- Ehlig-Economides, C. A., Chan, K. S., & Spath, J. B. (1996). Production enhancement strategies for strong bottom water reservoirs. Paper SPE 36613, Society of Petroleum Engineers. doi:10.2118/36613-MS.
- Hammersley, M., & Handscomb, D. C. (1983). Monte Carlo Methods. In M. S. Bartleth (Ed.), Chapman and Hall. London and Norwich: Fletcher and Son Limited.
- Inikori, O. S., & Wojtanowicz, A. K. (2002). New concepts of dual completion for water cresting control and improved oil recovery in horizontal wells. Paper SPE 77416, Society of Petroleum Engineers. doi:10.2118/77416-MS.
- Kabir, M., Mckenzie, P., Cornell, C., & O’Sullivan, T. (1998). Gas injection technique to develop rim oil, Mereenie Field, Australia. Paper SPE 50050, Society of Petroleum Engineers. doi:10.2118/50050-MS.
- Lawal, K. A., Inewari, A., & Adenuga, A. O. (2010). Preliminary assessment of oil-rim reservoirs. “A review of current practices and formulation of new concepts”. Paper SPE 136955, Society of Petroleum Engineers. doi:10.2118/136955-MS.
- Mohamed, N., Ezuka, I., & Ghasen, G. N. (2010). Economic evaluation of intelligent wells in simultaneous production of oil and gas reserves from Single Wellbore. Paper SPE 132925, Society of Petroleum Engineers. doi:10.2118/132925-MS.
- Onyeukwu, I. H., Peacock, A., & Matemilola, S. A. (2012). Improving recovery from oil rim reservoirs by simultaneous gas and water injection. Paper SPE 162956, Society of Petroleum Engineers. doi:10.2118/162956-MS.
- Yang, W., & Wattenbarger, R. A. (1991). Water coning correlation for vertical and horizontal wells. Paper SPE 22931, Society of Petroleum Engineers. doi:10.2118/22931-MS.