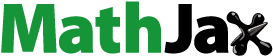
Abstract
Every year severity of road crash shows substantial evidence that Bangladesh is a crash-prone country. Different factors are directly and indirectly linked with crash occurrences. Thus, developing a crash prediction model is of necessity to explore the effects of various structural and non-structural elements associated with transportation system on crashes in the context of Bangladesh. Considering all districts (64) of Bangladesh, 13 types of road infrastructures, different types of roads and highways, socio-economic and demographic factors, and weather conditions have been taken as predictors to identify their inimical effect on crash occurrence. Poisson Regression models have been developed for different crash types described in the crash data. In this research, an apt focus is given on the effect of road infrastructures on crash severity. The result displays that Reinforced Cement Concrete (RCC) and Pre-stressed Concrete (PC) built infrastructures are harmful for fatal and grievous crashes whereas structures designed with both concrete and steel, e.g., Steel Beam and RCC Slab (SBRS), Truss with RCC Slab (TRS), Baily with Steel Deck (BSD) are found less detrimental to any type of crash severity. National highways, non-surveyed roads, paved roads are found hazardous. Also, sex ratio and large household size tend to increase fatal crash. Moreover, rainfall and fog, both have strong influence on crash occurrence.
PUBLIC INTEREST STATEMENT
This study attempts to develop a statistical crash prediction model considering different explanatory factors, e.g., road infrastructures, weather conditions, socio-economic and demographic characteristics. With these factors, empirical equations of count model are developed to predict crashes based on different severity levels considering 64 districts of Bangladesh. These models are expected to help the policymakers and transport authorities to identify appropriate materials in constructing road infrastructures in Bangladesh from road safety perspective.
1. Introduction
To assist in managing the frequency and severity of crashes, road safety study is of preliminary requirement. From the world status report on traffic road accidents and injuries, it is perceived that the total number of road traffic deaths remains unacceptably high at 1.24 million per year. Only 7% of the world’s population have adequate laws addressing five factors related to accidents (i.e. speed, drink-driving, helmets, seatbelts, and child restraints) (Global status report on Road safety, Citation2013). About 1.2 million people are killed and 50 million are injured worldwide. It is expected that collision injury will move from 9th place to 3rd place by 2020 (Jahan, Citation2006). In case of developing countries, the situation is more alarming. Among all crashes, eighty-six (86%) percent occurs in the developing countries (Peden et al.,Citation2004). Road crashes scenario of developing countries is worse comparing with that of developed countries, even if these countries only account one-third of the total motor vehicle fleet (Rifaat et al., Citation2014). Accident rates in developing countries are often 10–70 times higher than those of developed countries (Sheikh, Citation2004). In developing countries, the situation is becoming worsened due to the rapid growth of motorized vehicles relative to their population (Khanal & Sarkar, Citation2014). Also, the heterogeneous traffic modes make the traffic and safety situation much more complicated in these countries (Anowar et al., Citation2014).
As a developing country, Bangladesh faces a similar kind of problem. Each year, road accidents claim on average 12,000 lives and lead to about 35,000 injuries (ARI, Citation2013). The fatality rate in Bangladesh is almost 25 times higher comparing with that of developed countries, 8 times higher than Thailand and 3 times higher than India (Jahan, Citation2006). Moreover, the annual economic loss caused by traffic accidents is estimated to be in the order of 2 to 3 percent of the GDP (ARI, Citation2013). The situation seems out of control when the report on vehicles’ growth trend says that, in between the years 2009–2013, 10–12 percent of vehicles have been increased whereas the road length and road infrastructure have been increased approximately 0.12–0.62 percent on an average per year (Bangladesh Bureau of Statistics Annual Report, Citation2013). In Bangladesh, a considerable number of crashes occur every year due to bad weather. Especially, in the coastal region due to heavy rainfall and turbulent wind speed, and the northern region for heavy fog several crashes occur every year. If the data of crash occurrence due to bad weather is seen, it is observed that 55.2%, 3.3%, and 41.5% crashes occur due to rain, wind and foggy weather, respectively (ARI, Citation2013).
It is, therefore, necessary to quantify the causes of crashes in terms of crash occurrence factors that depend on road infrastructures, geometric factors, traffic characteristics, socio-economic and demographic factors, weather factors and interactions among these factors. Thus, developing a statistical model comprising all the significant variables in an equation that can predict crash occurrence is one of the solutions for this quantification. It can enable to make and design highway standards and policy that are essential to highway safety. Also, this can help to compare different design alternatives of the highway system with limited resources and other constraints. Furthermore, the contribution of this paper is to create a relationship of different types of infrastructure with several crash types based on severities which is one of the noble attempts in developing countries’ context. In this paper, the main objective is to find out the significant effects of different road infrastructures, geometric factors, socio-economic and demographic factors and weather conditions on different crashes classified by severity.
While investigating the earlier studies on crash occurrence and severity, it is found that dominant explanatory variables such as geometry, operations, and weather (Kopelias et al., Citation2007), crash factors including crash type and location (Schneider & Savolainen, Citation2011), hazardous roadside furniture (Ray et al., Citation2014) have been considered and modeled with crash severity. Various studies suggest that there is a relationship among road infrastructure, geometric design and road crash and number of fatalities and reported accidents varies due to the change of these parameters (Wang et al., Citation2013). Street patterns have also different effects on traffic collisions (Rifaat & Tay, Citation2009; Rifaat et al., 2009b). Several potential causes were found linking with crash injury severity, for example, changing lane width (C. Lee et al., Citation2015), the impact of fixed roadside (Holdridge et al., Citation2005), effect of design criteria of roads and road environment (Lindermann, Citation2007), planning and management of road networks (Gomes, Citation2013). Moreover, road sections with high traffic volume, a high number of curves per kilometer and the absence of shoulder were found to be hazardous (Thakali et al., Citation2009).
The effects of demographic characteristics on road safety have also been explored. Lack of education and enforcement play a substantial role in the occurrence of crashes. Economic growth is greatly reduced due to crash occurrences (Flahaut, Citation2004). The relationship between traffic fatality and per capita income was developed to forecast traffic (Kopits & Cropper, Citation2005; Traynor, Citation2008). Interestingly, the inclusion of demography was found insignificant in changing of fatalities and reported crashes according to the work of Noland and Oh (Citation2004). A study showed that the increase in household population results in increasing accident number and male population decreases the crash number and female population shows vice versa (Rifaat et al., Citation2014)
The effect of weather on crashes was examined in several studies. Some studies investigated weather effects on free-flow speed (Camacho et al., Citation2010), effect of saturation flow rate that is influenced by various weather conditions (Asmer & Zuylen, Citation2011), effect of precipitation that increases crash frequency (Theofilatos & Yannis, Citation2014). Moreover, a study on crash severity to find the effects of different weather conditions revealed that adverse weather is associated with an increase of 9% to 73.7% of all crash types (El-Basyouny et al., Citation2014). In Bangladesh, a study reveals that rainy, foggy and windy conditions are linked to increase intersection crash injury severity (Anowar et al., Citation2014)
In Bangladesh, several disaggregated studies were performed describing the effect of road infrastructures, socioeconomic-demography, and weather condition on the crash occurrence at intersections (Anowar et al., Citation2014), and on the National highways (Alam et al., Citation2011; Hoque, Citation2008). Some studies identified several non-geometric attributes as significant contributors to the crash (Salehin, Citation2015). Recently, a study was also conducted on the effect of road infrastructures on district wise casualty occurrences in Bangladesh, however, this study only focused relationship between casualties and road infrastructures rather than focusing on crash severity (Anik & Sadeek, Citation2018).
Recently, several studies have been carried out for developing crash prediction models using statistical and artificial intelligence (AI) methods. In 2014, a study examined the effect of road infrastructure, socio-economic and demographic factors on crash occurrence by using Negative binomial regression model. However, an aggregated number of all crashes was considered in this study ignoring the different levels of crash severity. Also, weather factor was not considered in their model (Rifaat et al., Citation2014). A partially constrained generalized ordered logit model was developed in a study on the factors influencing the severity of intersection crashes in Bangladesh (Anowar et al., Citation2014). A bayesian crash prediction model was employed to assess the safety risk of urban intersections (Salehin, Citation2015), generalized linear modeling was used to predict crashes at different levels of crash severity for major arterial segments (Hadiuzzaman et al., Citation2016).
Papadimitriou et al. (Citation2019) and Mohammed et al. (Citation2019) identified several factors of crash occurrence with the explanation of how these variables affect on crash injury severity by finding their positive or negative effects on the crash severity. Tang et al. (Citation2019) applied different AI methods and compared their performances in terms of prediction accuracy. They concluded that the stacking model, i.e.,, a combination of different classification methods distributed into two layers gives better results compared to single-based classification. Gomes et al. (Citation2019) made an assessment of crash prediction performance based on enriched data. This study raised the issue of unavailability of data and suggests enhancing the explanatory variables as a function of a more comprehensive dataset. La Torre et al. (Citation2019) developed two accident prediction models for single and multi-vehicle fatal and injury crashes based on the procedure of the Highway Safety Manual (HSM) and introduced a new procedure that gains the capability of transferability in other European roadways.
Artificial neural network was considered to develop accident prediction model using (Chakraborty et al., Citation2019) road geometrical characteristics, network planning, traffic exposure, and operational characteristics. Machine learning algorithms (i.e., decision tree, random forest, ANN) were used to forecast crash severity considering weather condition (Lee et al., Citation2020). Dong et al. (Citation2019) developed a support vector regression (SVR) model based on state and space to identify risk factors considering changes of roadway from time-to-time and they found that the developed model can respond well for accounting the high variation of roadway environment.
Several review papers have been published that focused on the crash prediction models. For example, Mohammed et al. (Citation2019) reviewed accident scenarios of different countries such as Turkey, India, Iran, Indonesia, Thailand, Africa, etc. and they also investigated different types of crash prediction models those are previously developed based on different independent variables formed from geometric and traffic characteristics, access road, road segment length, speed, economic and social factors. Pradhan and Sameen (Citation2019) reviewed traffic accident prediction models based on neural networks. This study compared the technical detail of statistical modeling of crash occurrence with neural networks. Prediction and modeling power of both methods were compared and was concluded that it is preferable to model crash prediction using statistical methods, however, when things get complicated, for example, if non-linearity is present in the model then neural networks could be used. They also suggested that count data models are preferable for frequency modeling of traffic accident whereas artificial intelligence such as deep learning or other fusion algorithms could be a better choice for forecasting the severity of crashes.
However, very few studies have developed crash prediction model considering road infrastructures in an elaborative way. Papadimitriou et al. (Citation2019) identified some risk factors from road infrastructures, namely, road type, road surface, road environment (e.g., lighting and weather), road segments, junctions, traffic control, cross-sectional elements, cross-slopes, lanes, median deficiencies, shoulder deficiencies, etc. Mukherjee and Mitra (Citation2019) also used road infrastructure as variables with land use. They have considered the width of the carriageways, absence of designated bus stop facilities, adequate sight distance, etc. under the category of land use and road infrastructural variables.
An aggregated study to find the effect of road infrastructures, geometric factors, demographic factors and weather conditions on different types of crashes based on severity has not been explored yet. As a result, this paper fills the gap by developing crash prediction models for fatal, grievous, minor, and PDO crashes separately in the context of a developing country.
2. Data collection and study area
In our study, district-level data has been taken as the unit of analysis. Crash severity data of 64 districts in Bangladesh are employed in this study. Accident Research Institute (ARI), BUET had provided all the district-wise data of Fatal, Grievous, Minor and Property Damage crashes from 2009 to 2013. The aggregated sample from 2009 to 2013 is 8578, 1692, 286, 326 for fatal, grievous, simple (minor) and collision types (PDO-Property Damage Only) crashes respectively are used for developing models. Figure represents a frequency-based crash severity chart of each district considering the year from 2009 to 2013. It is important to admit that in Bangladesh crash report data is biased as less severe crashes such as Property Damage Only (PDO) or Minor Injury crashes are not often reported and recorded (Ahsan et al., Citation2011; Raihan et al., Citation2017).
Figure 1. Crash frequency by severity in all 64 districts in Bangladesh (Aggregated data from 2009 to 2013)
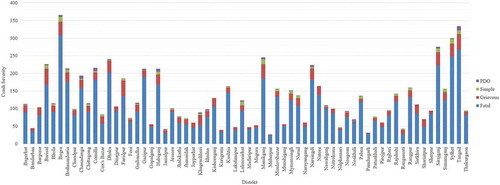
Apart from the number of crashes, this study also includes data of different road infrastructures, different road networks, socio-economic, demographic characteristics and weather conditions of all districts in Bangladesh. The data are structured in an unbalanced-panel data type and are divided among districts. The reason for unbalanced-panel data is that it is very difficult to collect historical data which accounts changes in road infrastructure and demography for every year. Thus, the data for road infrastructures, road networks, and demographic characteristics were considered same for all 5 years. However, crash data based on severity levels and weather data were obtained for every year from 2009 to 2013.
2.1. Road infrastructure
From the RMMS database of Roads and Highways Department (RHD), 13 types of infrastructure data have been collected. The data includes the types of infrastructure and the length of each type of structure for 64 districts. Also, information of different road networks, i.e.,, National highways, Regional highways, and Zilla roads are taken for consideration in the model. In Table , description for each road infrastructure is given. Moreover, Figure represents the road network in Bangladesh which shows National, Regional and Zilla roads in all districts.
Figure 2. Road network in Bangladesh (Source: HDM circle, roads and highways department of Bangladesh)
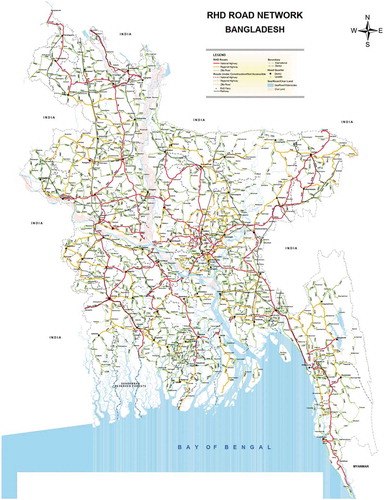
Table 1. Description of road infrastructure, socio-economic, demographic and weather data
2.2. Socio-economic and Demographic Data
District wise Socio-economic and demographic factors have been extracted from the Bangladesh Bureau of Statistics (BBS) Year Book, 2013. Different Information is observed on general households, institutional households, and the number of male and female, size of households, sex ratio, literacy rate, temporary insolvency, and percentage of savings of income, etc. The definition and explanation of different road infrastructures, socio-economic, demographic and weather data are shown in Table .
2.3. Weather data
Bangladesh Meteorological Department (BMD) provided the daily data of fog, rainfall, maximum temperature and minimum temperature, wind intensity and its direction. They provided data of 35 stations around the country. In our study, fog, rainfall, maximum temperature, and minimum temperature are taken as predictors of crashes. Data has been taken from the year 2009 to 2013. Daily data are aggregated into yearly data for all 64 districts in Bangladesh.
3. Methodology
Count data modeling is generally used for crash frequency analysis as the number of crashes on a roadway is a non-negative integer (Anastasopoulos et al., Citation2008). If event “” occurs according to a Poisson process with parameter
, then the Poisson distribution can be written as:
where is the Poisson parameter for individual districts of “
” in year “
”, which is district
’s expected number of crash,
it
, “
” is the probability of “
” accidents occurring in that district “
” in the year “
”. Poisson regression specifies the Poisson parameter
as a function of explanatory variables by using the log-linear function:
where Xit is a vector of explanatory variables and β is a vector of estimable parameters. The model was estimated using maximum likelihood method. In case of Poisson distribution, the likelihood of the expected number of event , when the observed number of
is known and can be expressed as
The log-likelihood function then given by-
Generally, count models (e.g., Poisson) assume that coefficients are fixed across the observation. However, it may have a problem of overestimation of the t-value of the estimated parameters from an underestimated standard error due to unobserved heterogeneity across all observations. To tackle this situation, the random parameter count model can be used. In this case, the parameter works as the following equations:
Here, is a randomly distributed term. For this model the log-likelihood can be written as the following equation:
Here, refers to the probability density function of
(Washington et al., Citation2003).
3.1. Model Evaluation
To measure the overall goodness of fit of the model, the log-likelihood ratio index has been calculated which can be written as:
where L(β) = log-likelihood value of the fitted model, L(0) = log-likelihood value of the model with a constant term.
In this analysis, an initial investigation found no significant unobserved heterogeneity. Because both mean and standard deviation of the variables coming from random parameter density are found statistically insignificant. It is worth to mention here that a random parameter is only valid when both average and standard deviation of the parameter density are statistically significant. If the estimated mean and standard deviation are not statistically different from zero, the parameter is constant across the observations (Park & Lee, Citation2017). Thus, in this paper, the fixed parameters models are used to estimate the final model.
4. Results and discussion
Initially, 98 variables were taken for developing crash prediction models. Firstly, negative binomial regression is applied to the datasets. Separate models have been developed for Fatal, Grievous, Minor and PDO crashes. All the insignificant variables are excluded from each of the models and the variables that are statistically significant with a 10% level of significance have been taken as the predictors for each type of response variable. Then, the over-dispersion parameter is checked whether it is significantly different from zero or not. In this study, the over-dispersion parameter is not statistically significant for any cases. Therefore, the Poisson regression model was again developed with significant factors. The estimation result from the final model is summarized and reported in Table . In general, the four individual crash severity models fitted the data well with fairly large chi-square goodness of fit. The pseudo R square is reported for all models as well. A variable is considered statistically significant in this study if the p-value is ≤0.1. The statistically significant variables are presented in three major categories, i.e., district wise infrastructure, socio-economic and demographic characteristics, and weather conditions to find out their effects. Descriptive statistics of these variables are given in Table . Discussions on the effects of these significant variables are presented among three categories as well.
Table 2. Descriptive statistics of four types of crash, road infrastructures, socio-economic and demographic conditions, and weather conditions of all districts in Bangladesh
Table 3. Parameter estimates of all 4 crashes
4.1. Road Infrastructure
From Table , it can be inferred from the model result that on National highways, Box culverts and RCC made infrastructures (RCC Bridge) have a severe impact on the crash occurrences. Both of them increase the fatal and grievous crash if their length increase in a district. Several reasons may cause these findings for example, the narrow width of these structures likely plays an important role. If carriageway and shoulder are narrow in these structures, the overtaking of vehicles is difficult which often ends up to severe crashes on these high-speed roads. Also, damaged or missing sections in an infrastructure, collision with the railing or curbs may promote crashes on these structures. Slab culvert also resembles the result as those of the previous two. But PC girder type structures show opposite result. The reason behind it perhaps, this structure may be constructed with proper care along with appropriate operational management of traffic on them. Also, the structural strength is high enough to assure it as a safe and reliable structure.
However, in Regional highways and Zilla roads, no significant effect is found for both structures. Both arch masonry and RCC bridge are likely to decrease fatal crash, however, RCC bridge tends to increase grievous crash. Steel Beam and RCC Slab (SBRS) and Baily with Steel Deck (BSD) exhibit a mixed effect on the grievous and PDO type crashes. SBRS tends to increase grievous crash and decrease PDO type crash whereas BSD exhibits opposite result. Experiencing obstruction, collision with guard rails, entrance posts and wing walls may be the reason for fatal and injury crashes on these structures.
On Zilla roads, girder made bridges (PC and RCC girder) tend to increase all types of crashes and SBRS, BTD and BSD tend to decrease grievous and minor crashes. The presence of PC girder type structures often end up with fatal, minor and PDO types crash on Zilla roads. Driver’s attitude perhaps an important factor for the involvement of these types of crashes. Drivers may face less hindrance of speeding up on Regional and Zilla roads as the traffic volume on those roads are less than this of the National roads.
Most of the composite steel structures (i.e. Steel and concrete are used for different components of structure) decrease crash severity. Among these, SBRS is found statistically significant for all types of highways (i.e. National, Regional, Zilla). SBRS decreases fatal and grievous crash on National and Regional highways. Moreover, BSD decreases the grievous crash on Zilla roads and minor crash and PDO on Regional highways. Perhaps this finding indicates safe road condition where these structures are constructed. However, it tends to increase grievous collision on Regional highways.
Pre-stressed concrete made bridges on Zilla roads are found negatively associated with crashes that means with the increase of these infrastructures’ length crash occurrence decreases. Low vehicle speed due to less width of Zilla road may be the reason of these findings.
Our result indicates that when the length of the National roads and roads that are not surveyed increases, it enhances the chances of fatal, grievous and minor crashes. Roads that are not surveyed mean those roads in National/Regional/Zilla network are not classified, pavement materials used for those roads are not inspected and AADT data for those roads are not surveyed. Furthermore, if National highways increase, the likelihood of fatal and minor crashes increases which is consistent with the finding of study by Hoque (Citation2008). This may occur due to the drivers' inattentiveness and unconsciousness. Additionally, on National highways drivers have more opportunity to speed up their vehicles that may exceed the speed limit of those roads. Also, in some areas, it is found open-air markets besides the National highways. Thus, pedestrians are at risk while crossing the roads as chances being hit by vehicles increase. It may be reduced by providing cautionary signs on the highways. When roads are made of rigid pavement at Union level, chances of fatal and minor crash increase whereas brick pavements decrease the chances of grievous crashes on Union road but increase on Upazilla roads. It is important to mention that if the number of structural span increases on Union, Upazilla and Village roads, the likelihood of the occurrence of fatal, grievous and collision type crash increases.
In this study, it is observed that the existing gap number is negatively associated with fatal crashes on Union roads whereas it is positively associated with those of village roads. The existing gap means the gap in the continuous road system. This gap decreases the traffic volume by providing alternatives traffic routes and modes such as ferries. Perhaps less traffic volume due to the presence of these gaps may reduce crash. This result is also consistent with a previous study (Rifaat et al., Citation2014).
4.2. Socioeconomic and demographic characteristics
If the number of institutional household size increases, the chance of a fatal crash also increases. An institutional household defines hospitals, clinics, educational institutions, etc. Usually traffic demand and pedestrian movement assumed to be high near these institutions. Thus, fatality may increase due to lack of road sign, lack of proper pedestrian facilities around these institutions. Poor traffic management may promote vehicle-pedestrian collision. On the contrary, the presence of other households tends to decrease grievous crash perhaps due to less traffic.
4.3. Weather Condition
Interestingly, with the increase of rainfall in the year 2009, 2011 and 2013 fatal, minor and collision type crashes are likely to decrease. Conscious driving and hindrance of increasing speed by rain may be the reason for this finding. The average minimum temperature is found negatively associated with the grievous crash in 2009 and visibility in 2009 also exhibits the same result for a fatal crash. However, from 2012 to 2013 minimum temperature enhances the occurring of grievous and PDO type crash. Minimum temperature indicates a winter season that means the presence of foggy weather. Drivers’ discomfort in winter, vehicle speed, vehicle lighting system directly affect the crash occurrences. Moreover, if there is no median on roads, for reducing travel time the tendency of the drivers is to drive along the wrong way using opposite lanes. In foggy weather, this situation often enhances the chance of a crash. This study did not find any significant effect of temperature on the fatal and minor crash.
5. Conclusion
From this study, it is clear that many factors related to road infrastructures, socioeconomic and demographic features, and weather conditions have a significant impact on crash occurrence. The models’ result reveals the factors which increase or decrease the district level crash severity. From the study, it is found that any types of concrete made infrastructure (RCC Bridge, Arch masonry, Box culvert, RCC girder, and PC girder) are detrimental to fatal and grievous injury crashes on National, Regional and Zilla (district) roads. Highway infrastructure designed with composite materials (Steel and concrete) i.e. Steel beam and RCC slab (SBRS), Truss with RCC slab (TRS), Baily with steel deck (BSD) is found less harmful to any type of crash. Also, SBRS and TRS are found less vulnerable for National highways and Zilla roads.
National highways are found risk-prone locations for occurring fatal and minor injury crashes. Roads that are not surveyed have been found hazardous for grievous injury crashes. On the contrary, Zilla roads and Village roads are found less threat of occurring fatal crashes relative to those on the National highway. Upazilla and Union roads mostly are paved road or earthen type found increasing the fatal injury crashes whereas roads made by brick pavements are found having a positive effect on grievous injury crashes and negative effect on the same injury crashes when they are used to build Union roads. Moreover, variation in the number of road structures in union and village influences occurring fatal as well as grievous crashes. Additionally, a number of existing gap has a negative effect on the occurrence of fatal crashes in Union and positive effect on Village roads.
While considering socio-economic and demographic characteristics, it is found that the number of other households (e.g., shops, messes, offices) hardly promotes grievous crashes. If the institutional household size increases, the probability of fatal crashes also increases.
While considering weather conditions, it is observed that rainfall decreases fatal crash and property damage only crash during the year 2009 and 2013. Effect of average minimum temperature is not found significant for fatal crash and minor injury crashes, however, is found to increase grievous crashes and decrease PDO crashes. Visibility also influences crash occurrence.
Based on this information proper countermeasures can be developed for the reduction of crashes by selecting appropriate road construction materials and road structures. This study is beneficial for the developing countries like Bangladesh, which is experiencing a significant number of road casualties in recent times because of increased population, increased traffic, less development in roads and highways sector. Based on the findings it is suggested that in addition to taking initiatives to increase safety, improvement of existing infrastructures and roads is also needed. Material selection, thickness design for the road pavement, regular inspection of structural cracks should be monitored with proper care. The results of this study will provide important information to policymakers as well as transportation engineers and planners while constructing any road infrastructure particularly in Bangladesh or any other similar developing countries. Box culverts, RCC bridges, SBRS, PC girder, and RCC girder made bridges are found detrimental to crash occurrence according to the results of this study. Appropriate traffic signs, provisions of bus bays on the National, Regional and Zilla roads, sufficient widening of the roads, speed restriction, improvement of sight distance should be ensured to avoid human and economic losses.
Some limitations are also observed in this study. This study could not include some important factors which might affect the crash occurrence. Due to lack of information in district level data, the number of registered vehicles, licensing information, solvency rate, vehicles’ miles traveled, literacy rate, etc., were not considered in this study.
Additional information
Funding
Notes on contributors
Soumik Nafis Sadeek
Soumik Nafis Sadeek works as a lecturer in the Department of Civil Engineering of International University of Business Agriculture and Technology(IUBAT), Bangladesh. He received his B.Sc in Civil Engineering from Islamic University of Technology in 2015 and doing his masters in Transportation Engineering in the same university. His research interests include application of econometric modeling, artificial intelligence and reliability theories in road safety.
Shakil Mohammad Rifaat
Shakil Mohammad Rifaat works as a Professor in the Department of Civil and Environmental Engineering of Islamic University of Technology, a subsidiary organ of Organisation of Islamic Cooperation (OIC), Bangladesh. He received his PhD degree in transportation engineering from the University of Calgary, Alberta, Canada, in 2010 and Masters degree in Transportation Engineering from the National University of Singapore in 2004. He did his B.Sc. in Civil Engineering from Bangladesh University of Engineering and Technology in 2000. His research interests include traffic safety, transportation and econometric modeling.
References
- Accident Research Institute (ARI). Annual Report 2013. BUET.
- Ahsan, H. M., Raihan, M. A., Rahman, M. S., & Arefin, N. H. 2011. Reporting and recording of road traffic accidents in Bangladesh. 4th annual paper meet and 1st civil engineering congress, Decmber 22-24, Dhaka, Bangladesh
- Alam, M. S., Mahmud, S. M., & Hoque, M. S. 2011. Road accident trends in Bangladesh: A comprehensive study, 4th annual paper meet and 1st civil engineering congress, December 22-24, 2011, Dhaka, Bangladesh
- Anastasopoulos, P. C., Tarko, A., & Mannering, F. (2008). Tobit analysis of vehicle accident rates on interstate highways. Accident Analysis and Prevention, 40(2), 768–18. https://doi.org/10.1016/j.aap.2007.09.006
- Anik, A. H., & Sadeek, S. N. (2018). Effect of road infrastructures on casualty occurrence in Bangladesh, Proceedings of the 4th International Conference on Civil Engineering for Sustainable Development (ICCESD 2018), 9-11 February 2018, KUET, Bangladesh
- Anowar, S., Yasmin, S., & Tay, R. (2014). Factors influencing the severity of intersection crashes in Bangladesh. Asian Transport Studies, 3(2), 1–12. https://doi.org/10.11175/eastsats.3.143
- Asmer, J., & Zuylen, H. V. (2011). Saturation flow under adverse weather conditions. Transportation Research Record: Journal of Transportation Research Board, 2258(1), 103–109. https://doi.org/10.3141/2258-13
- Bangladesh Bureau of Statistics Annual Report. 2013.
- Camacho, F., Garcia, A., & Belda, E. (2010). Analysis of impact of adverse weather on freeway free-flow speed in Spain. Transportation Research Record: Journal of Transportation Research Board, 2169(1), 150–159. https://doi.org/10.3141/2169-16
- Chakraborty, A., Mukherjee, D., & Mitra, S. (2019). Development of pedestrian crash prediction model for a developing country using artificial neural network. International Journal of Injury Control and Safety Promotion, 26(3), 283–293. https://doi.org/10.1080/17457300.2019.1627463
- Dong, C., Xie, K., Sun, X., Lyu, M., & Yue, H. (2019). Roadway traffic crash prediction using a state-space model based support vector regression approach. PLos ONE, 14(4), e0214866. https://doi.org/10.1371/journal.pone.0214866
- El-Basyouny, K., Barua, S., Islam, M., & Li, R. (2014). Assessing the effect of weather states on crash severity and type by use of full Bayesian multivariate safety models. Transportation Research Record: Journal of Transportation Research Board, 2432(1), 65–73. https://doi.org/10.3141/2432-08
- Flahaut, B. (2004). Impact of infrastructure and local environment on road unsafety: Logistic modeling with spatial autocorrelation. Accident Analysis and Prevention, 36(6), 1055–1066. https://doi.org/10.1016/j.aap.2003.12.003
- Global status report on Road safety. 2013.
- Gomes, M. M., Pirdavani, A., Brijs, T., & Pitombo, C. S. (2019). Assessing the impacts of enriched information on crash prediction performance. Accident Analysis and Prevention, 122(January), 162–171. https://doi.org/10.1016/j.aap.2018.10.004
- Gomes, S. V. (2013). The influence of the infrastructure characteristics in urban road accidents occurrence. Accident Analysis and Prevention, 60, 289–297. https://doi.org/10.1016/j.aap.2013.02.042
- Hadiuzzaman, M., Karim, M. A., Rahman, M. M., & Hasan, T. (2016). Planning level regression models for prediction of the number of crashes on urban arterials in Bangladesh. International Journal of Transportation Engineering, 3(4), 267–275. doi: 10.22119/IJTE.2016.16177
- Holdridge, J. M., Shankar, V. N., & Ulfarsson, G. F. (2005). The crash severity impacts of fixed roadside objects. Journal of Safety Science, 36(2), 139–147. https://doi.org/10.1016/j.jsr.2004.12.005
- Hoque, M. M. (2008). The prevention of road traffic accidents: An overview of behavioural modification techniques. Journal of Scientific Research, 22(2), 85–97.
- Jahan, A. A. 2006. Road safety countermeasures and traffic calming practices in roads and highways department in Bangladesh. In Proceedings of international conference on road safety, Dhaka, Bangladesh, pp.120–123
- Khanal, M., & Sarkar, P. (2014). Road safety in developing countries. Journal of Civil and Environmental Engineering, S2, 001. https://doi.org/10.4172/2165-784X.S2-001
- Kopelias, P., Papadimitriou, F., Papandreou, K., & Prevedouros, P. (2007, December). Urban freeway crash analysis: Geometric, Operational and weather effects on crash number and severity. Transportation Research Record: Journal of Transportation Research Board, 2015(1), 123–131. https://doi.org/10.3141/2015-14
- Kopits, E., & Cropper, M. (2005). Traffic fatalities and economic growth. Accident Analysis and Prevention, 37(1), 169–178. https://doi.org/10.1016/j.aap.2004.04.006
- La Torre, F., Meocci, M., Domenichini, L., Branzi, V., Tanzi, N., & Paliotto, A. (2019). Development of an accident prediction model for Italian freeways. Accident Analysis and Prevention, 124(March 2019), 1–11. https://doi.org/10.1016/j.aap.2018.12.023
- Lee, C., Aty, M. A., Park, J., & Wang, J. H. (2015). Development of crash modification factors for changing lane width on roadway segments using generalized nonlinear models. Accident Analysis and Prevention, 76(March 2015), 83–91. https://doi.org/10.1016/j.aap.2015.01.007
- Lee, J., Yoon, T., Kwon, S., & Lee, J. (2020). Model evaluation for forecasting traffic accident severity in rainy seasons using machine learning algorithms: Seoul city study. Applied Sciences, 10(1), 129. https://doi.org/10.3390/app10010129
- Lindermann, H. (2007). The design of roads and of the road environment in small rural communities. Transportation Research Record: Journal of Transportation Research Board, 2025(1), 53–62. https://doi.org/10.3141/2025-05
- Mohammed, A. A., Anbak, K., Mosa, A. M., & Syamsunur, D. (2019). A review of the traffic accidents and related practices word-wide. The Open Transportation Journal, 13(1), 65–83. https://doi.org/10.2174/1874447801913010065
- Mukherjee, D., & Mitra, S. (2019). Impact of road infrastructure land use and traffic operational characteristics on pedestrian fatality risk: A case study of Kolkata, India. Transportation in Developing Economies, 5(6), 1–9. https://doi.org/10.1007/s40890-019-0077-5
- Noland, R. B., & Oh, L. (2004). The effect of Infrastructure and demographic change on traffic-related fatalities and crashes: A case study of illinois-level data. Accident Analysis & Prevention, 36(4), 525–532. https://doi.org/10.1016/S0001-4575(03)00058-7
- Papadimitriou, E., Filtness, A., Theofilatos, A., Ziakopoulos, A., Quigley, C., & Yannins, G. (2019). Review and ranking of crash risk factors related to the road infrastructure. Accident Analysis and Prevention, 125(April 2019), 85–97. https://doi.org/10.1016/j.aap.2019.01.002
- Park, M., & Lee, D. (2017). Development of accident frequency models with random parameters on interstate roadway segments with and without lighting systems. Journal of Advanced Transportation, 2017, 1–8. https://doi.org/10.1155/2017/4740532
- Peden, M., Scurfield, R., Sleet, D., Jarawan, E., & Mathers, CA2004. World Report on Road Traffic Injury Prevention, World Health Organization.
- Pradhan, B., & Sameen, M. I. (2019). Review of traffic accident predictions with neural networks (Chapter 8). In Laser scanning systems in highway and safety assessment in science, technology and innovation, 97-109. Springer Nature Switzerland.
- Raihan, M. A., Hossain, M., & Hasan, T. (2017). Data mining in road crash analysis: The context of developing countries, 41-52. International Journal of Injury Control and Safety Promotion. https://doi.org/10.1080/17457300.2017.1323929
- Ray, M., Carrigan, C., & Plaxico, C. (2014). Method of modeling crash severity with observable crash data. Transportation Research Record: Journal of Transportation Research Board, 2437(1), 1–9. https://doi.org/10.3141/2437-01
- Rifaat, S., Rahman, H., & Mohammad, P. M. (2014). Study on the effect of road infrastructure, socio economic and demographic features on road crashes in Bangladesh. World Academy of Science, Engineering and Technology, International Journal of Social, Education, Economics and Management Engineering, 8(4): 1192-1197. doi: 10.5281/zenodo.1093466
- Rifaat, S., Tay, R., Perez, A., & de Barros, A. (2009). Effects of neighborhood street patterns on traffic crash frequency. Journal of Transportation Safety and Security, 1(4), 241–253. https://doi.org/10.1080/19439960903328595
- Rifaat, S. M., & Tay, R. (2009). Effects of street patterns on injury risks in two-vehicle crashes. Transportation Research Record, 2102(1), 61–67. https://doi.org/10.3141/2102-08
- Salehin, M. F. (2015). Bayesian crash prediction models to assess the safety risk of urban intersections in metro Dhaka, M.Sc Thesis, Department of Civil Engineering, Bangladesh University of Engineering and Technology (BUET)
- Schneider, W., & Savolainen, P. (2011). Comparison of severity of Motorcyclist Injury by Crash Types. Transportation Research Record: Journal of Transportation Research Board, 2265(1), 70–80. https://doi.org/10.3141/2265-08
- Sheikh, M. M. R. 2004. A statistical analysis of road traffic accidents and casualties in Bangladesh, M.S. thesis, Dept. Civil Eng., Napier University.
- Tang, J., Liang, J., Han, C., Li, Z., & Huang, H. (2019). Crash injury severity analysis using a two-layer stacking framework. Accident Analysis and Prevention, 122(January 2019), 226–238. https://doi.org/10.1016/j.aap.2018.10.016
- Thakali, L., Hossain, M., & Kanitpong, K. (2009). Development of accident prediction models, their possibilities and efficacy for the developing countries; A Thai experience. Proceedings of the Eastern Asia Society for Transportation Studies, 7: 335. https://doi.org/10.11175/eastpro.2009.0.355.0
- Theofilatos, A., & Yannis, G. (2014). A review of the effect of traffic and weather characteristics on road safety. Accident Analysis and Prevention, 72(November 2014), 244–256. https://doi.org/10.1016/j.aap.2014.06.017
- Traynor, T. L. (2008). Regional economic conditions and crash fatality rates – A cross country analysis. Journal of Safety Research, 39(1), 33–39. https://doi.org/10.1016/j.jsr.2007.10.008
- Wang, C., Quddus, M. A., & Ison, S. G. (2013). The effect of traffic and road characteristics on road safety: A review and future research direction. Safety Science, 57(March 2013), 264–275. https://doi.org/10.1016/j.ssci.2013.02.012
- Washington, S., Karlaftis, M., & Mannering, F. (2003). Statistical and econometric methods for transportation data analysis. Chapman and Hall/CRC.