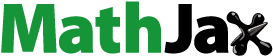
Abstract
This paper focused on applying social network analysis techniques to co-authorship network in order to discover the influencers in Civil engineering research field in Nigeria. It further applies the Latent Dirichlet allocation (LDA) algorithm to uncover the major research topics in this field. The research used 663 publications downloaded from the Scopus database, with the year of publication ranging from 1968 to 2018, using Nigeria as the case study, Civil and Structural engineering as the field of research. The study was carried out using the centrality measures in network analysis such as degree centrality, closeness centrality, and betweenness centrality for co-authorship network analysis of authors and text mining using the LDA algorithm to discover the research focus of the authors. Also, the relationship between the centrality measures and authors’ performance, measured in terms of citation was investigated using regression analysis. The results showed that there was a significantly positive relationship with betweenness centrality and closeness centrality for performance, but a negative relationship with degree centrality. Also the topics discovered using the LDA algorithm helped to reveal the major focus of Civil Engineering research in Nigeria. In conclusion, it is recommended that based on the co-authorship network of civil engineering research in Nigeria, which was found to be a healthy small-world community, the environment discovered can be improved upon to support collaboration and sharing of ideas between researchers in the civil engineering field.
PUBLIC INTEREST STATEMENT
In order to provide valuable interventions that will improve academic research, there are current waves of study which focuses on co-authorship networks and co-innovator networks where patents are the focus of the research. In this paper, the developed co-authorship network discovers the influencers in civil engineering research. The study was able to use centrality measures in social network analysis, and a semantic text clustering approach to identify major civil engineering research influencers and topics. In the research, it was discovered that academic performance has a significantly positive relationship with betweenness centrality and closeness centrality but a negative relationship with degree centrality. It is therefore recommended that based on the co-authorship network of civil engineering research in Nigeria, which was found to be a healthy small-world community, the environment discovered can be improved upon to support collaboration and sharing of ideas between researchers in the civil engineering field.
1. Introduction
Civil Engineering is the branch of engineering that deals with planning, design, and construction of structures for the need of the people (Ricketts et al., Citation2003) while Structural Engineering is a branch of Civil Engineering that deals with the study and application of structural theory to the design, analysis and evaluation of civil engineering structures (Chen & Liew, Citation2002). Very important engineering structures in everyday life need a touch of Civil and Structural Engineering: bridge construction, airport construction, highway engineering, water resources engineering, and community and regional planning are all notable mentions (Ricketts et al., Citation2003). Research in civil and structural engineering is important in Nigeria since the country is still developing and standard structures existing in developed countries are not fully visible yet in the country. From literature, it has been stated that one of the most important factors that causes construction delay in Nigeria are architect’s incomplete drawing and incomplete structural drawing (Aibinu & Odeyinka, Citation2006). In the same study, it was reported that 88% of the delay factors caused 90% of the overall delays on the building projects surveyed (Aibinu & Odeyinka, Citation2006). The impact of a lot of delay factors on buildings in Nigeria was also confirmed in a recent study (Ogunde et al., Citation2017). Ogunsemi and Jagboro (Citation2006) also reported an issue in the building projects in Nigeria in their study. Hence, the need for civil and structural engineering research in Nigeria.
Co-authorship network analysis on the other hand involves analyzing the inter-connectedness of authors with respect to how they collaborate on research projects (Racherla & Hu, Citation2010). Research in co-authorship network analysis typically involves the use of centrality measures which include degree centrality, closeness centrality, and betweenness centrality (Abbasi et al., Citation2012b) to measure the characteristics of authors in a co-authorship network. Co-authorship network analysis has been done on “steel structures”, an emerging research discipline in Civil engineering (Uddin et al., Citation2012) and also on infrastructure resilience in disasters (Nazarnia & Sarmasti, Citation2018). Other studies on co-authorship network analysis on the Civil engineering discipline can be found in literature (Abbasi et al., Citation2012b; Cimenler et al., Citation2014; Franceschet & Costantini, Citation2010). Some studies have directed their research efforts to focus on individual countries and regions of the world, some countries of focus in recent studies include Slovenia (Cugmas et al., Citation2016), Spain (Bordons et al., Citation2015), UK (Crescenzi et al., Citation2016), USA (Velden et al., Citation2010) and Italy (Abramo et al., Citation2011a; Franceschet & Costantini, Citation2010).
The research area is also not void of new techniques and innovation, this is also evident in literature. He et al. (Citation2013) proposed a new method for extracting information from a co-authorship network called Diversity Subgraph Extraction (DSE) using data primarily for studying interdisciplinary collaboration. The method was compared against another method based on breadth first search (BFS) and the results favoured DSE in terms of capturing richer information from a network. Oliveira et al. (Citation2017) proposed a Bayesian inference approach to measure the reliability of nodes (researchers) in a network and concluded in their study that the contribution of each researcher is relevant to the maintenance of the research groups.
This research work takes a step further from co-authorship and co-innovator network analysis by introducing semantic analysis to discover the focus of the research outputs in order to provide valuable interventions that will further improve academic research and collaboration.
2. Literature review
The review is divided into two major sections, the first section discusses the recent works done on co-authorship networks with the focus solely on research papers as the product of academicians while the second section focuses on co-innovator networks where patents are the focus of the research products.
In the first section, Racherla and Hu (Citation2010) did a social network analysis in the field of tourism using data from an identified top three journals in the field which are Annals of Tourism Research, Journal of Travel Research, and Tourism Management. They concluded that researchers in the tourism field restrict their collaborations to a specific group of interest that is; prominent researchers did not show the tendency to connect disparate groups even though researchers tend to favour collaborations with highly successful researchers. Franceschet and Costantini (Citation2010) studied how collaboration affect co-authored papers in terms of impact and quality using dataset across various disciplines in Italian Universities. The measure of impact was achieved through the citation metrics on retrieved papers while quality was measured based on the judgement of peer reviewers on the same set of papers. The national research assessment exercise of Italian universities (a peer review exercise) was used as the major source of data gathering and most of the articles were also indexed in the Web of Science database. They concluded that in terms of impact (citation metrics), in-house papers garnered less citations than those ones with contributions from external collaborators (collaborators from other universities). Velden et al. (Citation2010) obtained data from Web of Science (WoS) for three research fields in the discipline of Chemistry over 20-year period to carry out a mesoscopic analysis for some research groups in Europe and the USA. The authors combined the use of participant interview (qualitative) and network analysis (quantitative) methods as viable approaches for co-authorship analysis. Abramo et al. (Citation2011a) did a bibliometric analysis on Italian researchers in the field of hard sciences with data gathered from Web of Science over the period of 2001–2005 to answer the questions relating to the link between the level of internationalization of a single researcher and research performance. The authors used some indicators that can be broadly grouped into two (research performance indicators and internalization indicators) for the required analysis. The results from their analysis confirmed that researchers with more international collaborations have the best research performance. Abramo et al. (Citation2011b) studied collaboration networks among Italian researchers for the period between 2001 and 2005 and how they affect each researcher’s research performance. Like the work of Franceschet and Costantini (Citation2010), it was discovered that the discipline of Physics have the highest degree of international collaboration among researchers and that a researcher with a high level of international collaboration enjoys more productivity (publication volume). Productivity was judged to have more impact on the intensity of international collaboration than the average quality of research papers of scientists although both measurement factors have similar relative importance on the disposition to collaborate internationally. Abbasi et al. (Citation2012a) carried out another study on scholars in the discipline of Information Science and Library Science using data gathered from Scopus within the time frame of 2000 to 2009. The authors sought to look for the relationship between researchers’ collaboration network and their citation performance using structural holes theory. They concluded from their results that research performance is positively correlated with a researcher’s ego-network structure. Also, it was concluded that researchers with high betweenness centrality (high shortest path among collaborators) exhibit higher performance in their research. Finally, it was suggested that researchers should connect to more diverse research groups to improve their efficiency in research. Abbasi et al. (Citation2012b) carried out a study within the domain of “steel structures” to investigate how authors evolve during the expansion of the co-authorship network using three common centrality measures: degree centrality, closeness centrality and betweenness centrality. The authors set out to answer three research questions and came up with the following conclusions: (1) authors with higher betweenness centrality tend to attract more co-authors than those with higher degree centrality, (2) relatively small number of new authors attach themselves to existing authors, this coincides with the fact that existing authors also prefer to collaborate with their existing co-authors, (3) as one of the main highlights of the paper, the authors identified a “new” property for authors called brokering roles which characterises PhD supervisors who tend to create new collaborations as nodes with the highest degree of betweenness centrality over the years as they get new PhD students. Li et al. (Citation2013) investigated how six indicators of social capital interact and affect citations of Information Systems (IS) researchers using data gotten from the Social Science Citation Index (SSCI). Their results favours betweenness centrality in correspondence to the work of Yan and Ding (Citation2009) and Abbasi et al. (Citation2012b) by concluding that it significantly affect citation count for publications. Also, co-authoring with highly productive researchers has a huge tendency to increase the centrality of a researcher and positively affect their citation counts. The top five pure IS journals were selected for this study and the data gathered spans through the year 1999–2003. Finally, the result presented in the paper also concluded that team exploration and publishing tenure do not have any effect on author citations. Liao (Citation2010) made the same conclusion on team exploration. Benckendorff and Zehrer (Citation2013) studied the influence pioneer authors in tourism research along with their seminal works have on recent works. Scopus was used as the primary data source for data gathered within the spheres of the following leading journals: Annals of Tourism Research (ATR), Journal of Travel Research (JTR) and Tourism Management (TM) between 1996 and 2010. The following data points were gathered from their research: most frequently cited journals, most cited lead authors, co-citation network of most cited authors, most cited individual works and co-citation network of most influential works. De Stefano et al. (Citation2013) carried out a co-authorship analysis among Italian statisticians using three different data sources: Web of Science (WoS), Current Index to Statistics (CIS) and nationally funded research projects (PRIN). All the hypothesis tested in the research study were confirmed leading to the following conclusions: the number of co-authored publications is growing faster than single-authored publications in the field of statistics, the collaboration style of Italian statisticians is similar to that obtained in Social Sciences, the sub-fields have different collaboration styles and finally, the performance of Italian authors in the field of statistics is related to their collaboration styles in the co-authorship network. Didegah and Thelwall (Citation2013) examined whether some factors affect citation counts of articles. The factors include research collaboration, journal and reference impact, abstract readability, and article size. The number of authors and the number of countries positively affect citation counts in the fields studied except that there is no significant correlation between the number of countries and citation counts in the Social Sciences field. The authors also concluded that each additional author causes an increase in the average number of citations and that each additional country in the international collaboration network caused the same positive effect on the citation metric. Ortega (Citation2014) made some main conclusions as answers to the research questions raised in his work include: (1) authors within sparse and thin networks have a higher citation per document count than their counterparts within dense networks. (2) The sparse networks are more frequent in the Mathematics, Social Sciences and Economics, and Business disciplines while the dense networks occur frequently among the Physics, Engineering and Geosciences disciplines. (3) The collaboration type, research impact, and network structure are not correlated. These findings were gotten after the co-authorship analysis was done on data gotten from the Microsoft Academic Search bibliographic source. Cimenler et al. (Citation2014) carried out a regression analysis on gathered data for the College of Engineering of University of South Florida, USA to investigate the effect social network metrics have on their citation performance. Another study by Manganote et al. (Citation2014) was done based on the Scimago Institutions Ranking 2012 focusing on three indicators including internationalisation (international collaboration) which is closely related to our focus in this study. The result revealed a significant difference in the behaviours of Northern American and Western European institutions towards international collaboration. The study also revealed that Chinese institutions have low propensity to collaborate internationally which also reflected in their research impact (which was low). Ebadi and Schiffauerova (Citation2015) studied the impact of some factors on the position of researchers in a collaboration network at individual level. The dataset gathered was from Canadian researchers during the period of 1996 to 2010. It was concluded that funding is an important element in driving researchers to collaborate more. Bordons et al. (Citation2015) analysed the structure of co-authorship networks in three fields: Nanoscience, Pharmacology and Statistics for scientists that reside in Spain over a period of 3 years (2006 to 2008) the data were gathered from the Web of Science database. In the two experimental fields (Nanoscience and Pharmacology), the network structure is dense unlike that of the Statistics field that is less connected. For the three fields, the strengths of links among the co-authors have a positive relationship with the g-index. The authors used a Poisson regression model to explore the relationship between authors’ performance and the different measures of the collaboration network. It was also observed that there is a strong relationship between the positions of authors in the collaboration network and their research performance, a very glaring picture was portrayed in Nanoscience and Pharmacology. Finally, answering the third research question in the paper, it was concluded that there exist a significant difference in the “average citedness” of research papers among the three fields used for the study. Sadoughi et al. (Citation2016) studied co-authorship networks of Iranian researchers in the field of parasitology covering the period between 1972 and 2013. Data gathering was done with the Web of Science database. They concluded that the co-authorship network consists of 78 authors with a particular researcher leading in all measures of centrality (degree centrality, betweenness centrality, and closeness centrality) and that the collaboration with international colleagues is mostly done with researchers in England and the United States of America. Guan et al. (Citation2017) took into consideration the node attributes of knowledge elements in a paper in addition to the author attributes to study their effects on paper citations using papers in the research field of wind energy from the Web of Science and Journal Citation Reports databases. Dehdarirad and Nasini (Citation2017) applied a two-mode regression model to capture simultaneously, the effect of paper properties and authors’ behavioural characteristics and demography on the success and impact of the academic careers of authors based on the assumption that paper impact is not only affected by the paper characteristics but also on the characteristics of the authors.
The second section of the review focused on co-innovator networks. Beaudry and Schiffauerova (Citation2011) gathered data for Canadian inventors in the field of nanotechnology to study the impact of co-inventorship network characteristics on the quality of inventions (patent quality). They studied 5067 patents from the Nanobank database where at least one of the inventors resides in Canada with the aim of confirming four research hypotheses. Based on previous studies that highlighted the negative impact of geographical distance on R&D collaborations, Morescalchi et al. (Citation2015) carried out a study on data gathered from European Patent Office (EPO) for OECD countries to verify the claim. The study analysed four different networks: co-inventor network, patent citations network, inventor mobility network, and applicant-inventor network within the period of 1988 to 2009. The summary of their findings collaborated with previous findings: the distance effect has really had an impact on the two networks of concern to this study: co-inventor and patent citations. Guan et al. (Citation2015) studied the effects of a multi-level collaboration on innovators’ innovation performance, a drift from other studies that analyzed a single network. They used patent records in the field of alternative energy. The inter-country network structure was able to moderate the relationship between the inter-city network structure and innovation performance. The authors opined that their results were consistent with the interaction theory (Paruchuri, Citation2010). Crescenzi et al. (Citation2016) studied the characteristics of the collaborations among inventors in the UK, based on three research questions. They concluded that geographical proximity seems to be paramount to the inventor’s inventions though there is a potential advantage in creating a sparse network through collaborations with innovators outside the geographical boundaries. Guan and Liu (Citation2016) studied the effects of not just collaboration networks but also knowledge networks have on exploitative and exploratory innovations using data for the nano-energy field. The authors considered both types of networks based on two previous works (Wang et al., Citation2014; Yayavaram & Ahuja, Citation2008) where it was stated that technological innovation is influenced not only by collaboration networks but also in knowledge networks. This research work takes a step further from co-authorship and co-innovator network analysis by introducing semantic analysis to discover the focus of the research outputs.
3. Data collection and preprocessing
In order to retrieve the publications used for this research, “Civil and Structural Engineering” was used as the search criteria with the region restricted to Nigeria. A total of 663 records or publications were retrieved and 1461 unique authors were identified using their Scopus Identification Number (Author Ids). The publications retrieved for this research ranged from 1969 to 2018 as revealed in Table . The records retrieved had the following attributes; Publication Identity in Scopus, Author Identity in Scopus, Citations, Title, Year, Source title, Affiliations, Abstract, and Keywords. The unidirectional graph generated contained 1461 nodes (authors) and 12,273 edges (collaborations). Each node (author) in the network has a unique identification number and each edge has weight representing the citation of the collaboration. The dataset was retrieved from the Scopus website (https://www.scopus.com), which is an abstract and indexing database with full-text links that is produced by the Elsevier Co. The data were downloaded on the 5th of October 2018. To convert the raw data downloaded from the Scopus database to Edgelist which is the input to the network x API in python and Gephi, a script was written in MATLAB. The network graph for civil engineering research in Nigeria was constructed using network x API in python library and visualized using Gephi (https://gephi.org). The author identity number was used as nodes for the network construction to avoid name variation of the authors.
Table 1. Yearly publication Distribution
4. Research methodology
4.1. Research structure
In this study, the analysis is broken down into two sections: Scientific co-authorship Network Analysis on Civil Engineering Research (Section 4.2) and Identification of major civil Engineering research fields (Section 4.3).
The first section includes methods and theories of identifying major influencers of the civil engineering research in Nigeria using Social Network Analysis. Section two includes the description of the research field identification method which is basically text mining. For this research, LDA (Latent Dirichlet allocation) algorithm will be used for Topic Modeliing. The LDA algorithm was applied on the abstracts retrieved for the publications.
4.2. Social networks analysis
Social Network Analysis is from Sociology, but based on mathematical notions of graph theory (Arif et al., Citation2012). According to Kolaczyk and Csárdi (Citation2014), network graph modeling can be used to test the significance of the characteristics of observed network graphs, and also to study proposed mechanisms of real-world networks such as degree distributions and small-world effects. In this research, centrality measures from network analysis was used to find out the important authors in the network, signifying the most important or influential researchers in the civil engineering research in Nigeria. According to (Marsden, Citation2002), centrality is most often used to explain an actor’s structural position in a network. Centrality can therefore be defined as the extent to which a node is connected to other nodes within a specific network (Wasserman & Faust, Citation1994). Several studies have been carried out on the centrality measures of a co-authorship network and these include (Arif et al., Citation2012; Badar et al., Citation2013). Some researches such as Abbasi et al. (Citation2011) investigated and identified positive influences of centrality on performance outcomes in co-authorship network. In this research, we explore questions such as “Who are the major influencers of the civil engineering community in Nigeria?”, Are there correlations between performance measures in terms of citation and centrality measures, i.e., does the importance or influence of the researchers correlate with their output in terms of citation. The centrality measures used in this paper includes Degree Centrality, Closeness centrality, and Betweenness Centrality.
Degree Centrality aims at finding centrality based on the notion that important nodes have many connections and is expressed in (1) (Zachary, Citation1977).
is the degree of centrality of node V
is the number of nodes in the network
is the degree of node V
Closeness centrality aims at finding centrality based on the notion that nodes that are important are going to be a short distance away from all other nodes in the network. EquationEquation 2(2)
(2) is used to express the closeness centrality of node V (Zachary, Citation1977).
is the closeness centrality of node V
is the number of nodes in the network
is the sum of all the other nodes in the network and the distance between node v and those nodes.
Betweenness centrality aims at finding centrality based on the assumption that nodes that connect other nodes are important nodes. It is the number of shortest paths between nodes that go through a particular author. It relates to the perspective that importance relates to where a vertex is located with respect to the paths in the network graph (Azondekon et al., Citation2018). Therefore, the betweenness value gives the conclusion that nodes are important because they connect other nodes. EquationEquation (3)(3)
(3) is used to express betweenness centrality (Zachary, Citation1977).
is the importance of node V using betweenness
is the sum over all possible s and t
is the number of shortest paths between nodes s and t
is the number of shortest paths between nodes s and t that pass through node v
In this research, the research performance of the authors is measured using citation of authors. According to Garfield (Citation2014), “Citations are the currency of scholarship”. Other researchers such as Bornmann et al. (Citation2008) and Badar et al. (Citation2013) also collaborated this belief. According to existing research by Soheili et al. (2015) and Khasseh et al. (Citation2017), regression analysis has been widely used to explore the relationship between centrality measures and also performance measures in order to make recommendations for improvement in co-authorship collaboration for improved research. In this research, we report the results of analysis of variance (ANOVA) for regression analysis for centrality measures and performance measures.
4.3. Text mining
According to Irfan et al. (Citation2015), text mining is described as an intersection of techniques from information retrieval, text analysis, Natural Language Processing (NLP), and information classification domains which can be used to provide computational intelligence. It involves a complex procedure, involving automated discovery of useful and interesting knowledge from unstructured and ambiguous textual data (Basu et al., Citation2001; Han, Citation2005). Several existing research have attempted text clustering in various forms in order to discover non-trivial knowledge some of which include Liu and Xiong (Citation2011) and Afolabi et al. (Citation2017). In this research the basic text mining process employed is topic modelling which is a text clustering problem where document and words are clustered simultaneously (Blei et al., Citation2003). The main process is to transform keywords (or terms) contained in text documents (i.e., abstracts) into document-keyword matrix, which is then used to extract the topics in the document collection. According to Song and Suh (Citation2018), various data mining methods have been applied for document-keyword matrix such as clustering, latent semantic analysis, and sentimental analysis. According to Blei et al. (Citation2003), LDA is considered the most useful method for basic tasks of the natural language processes such as classification, novelty detection, summarization, and similarity and relevance judgments. This informed the selection for the LDA algorithm. The text mining methodology employed in this research is based on three major steps. i. Text pre-processing; ii. Creation of document matrix; iii. Building LDA models on the document-term matrix. The text preprocessing step consists of tokenization, normalization, and removal of stop words. The final stage of the pre-processing is the lemmatization using the wordnet dictionary. All the pre-processing was implemented using the python NLTK library. The tokenized words were then converted to document matrix using gensim library in Python. Finally, the LDA models were built using the gensim library. According to Blei et al. (Citation2003), the LDA algorithm can be described as a generative probabilistic model for topic modeling, and it is based on collections of discrete data such as frequency and text corpora. It is an algorithm that is useful for basic natural language processing tasks which involves classification, novelty detection, summarization, and similarity and relevance judgments. The main focus of LDA algorithms is that, documents are represented as random mixtures over latent topics where each topic is determined by a distribution over words (terms) (song & Suh, Citation2018). The algorithm is able to derive latent topics from topic probability conditioned on the document distributions and word probability conditioned on the topic distribution. LDA algorithm is useful in the case that the topic probabilities indicate an explicit representation of words contained in documents (Lee et al., Citation2015).
The number of keywords used to represent the latent topics in this work is 10 because it is difficult to characterize the latent topics based on the small number of keywords. Also, five latent topics were selected for each text category examined.
The text mining section is presented in the flow chart in Figure .
5. Result and discussion
5.1. Descriptive analysis
The degrees of the undirected graph ranged from 1 to 128 with an average degree distribution of 15.943. The degree distribution graph is presented in Figure . Mean Closeness centrality was discovered to be 0.711283 and the top 10 authors based on closeness centrality values are revealed in Table . Betweenness measures range between 0 and 9605 and the top 10 broker authors, i.e., authors with the highest betweenness centrality measure are also revealed in Table . The degree Centrality values ranged from 0.000684 to 0.087671 and the most connected authors, i.e., the top 10 authors based on degree centrality measure are also revealed in Table .
Table 2. Top 10 Authors in the Civil Engineering Co-Authorship network based on the Centrality Measures
5.2. Network cohesion
The number of cliques or communities identified in the network totalled 227 and Figure represents the size distribution of the communities (represented as modularity class). The network is not connected and a census of all the communities within the network reveals the existence of a giant community containing 166 authors.
Figure is a visualization of the largest community in the network. Clustering coefficient measures the tendency for authors who share connections in the network to become connected which is also known as triadic closure. For co-authorship network the higher the clustering coefficient the higher the possibility of author who share connections in the network to become connected. In this research the average clustering coefficient was calculated using Latapy (Citation2008) to give 0.911 with total triangles of 358,850. The civil engineering co-authorship network has a density of 0.011 which is a measure of how close the network is to complete.
The largest community in the network, consisting of 166 authors visualized in Figure , is an indication that the community has authors with high number of collaboration symbolizing a very active research community.
5.3. Relationship between performance (i.e. citation) and centrality measures
Regression analysis was used to study the relationship between peformance measures in terms of citation and the centrality measures (Betweenness, Closeness Centrality, and Degree Centrality). The result of analysis of variance (ANOVA) for regression analysis is presented in Table .
Table 3. ANOVA for regression analysis of performance (citation) and centrality measures
The regression analysis result shows that the centrality measures explain just 4.3% of variance of performance measure in terms of citation (R Square = 0.043905). The Significant F is 4.042E-14 which is less than 0.5 indicating a strong regression. The regression coefficients indicate that there is a positive relationship between performance and Betweenness (Coefficients = 7094.659, P-Value = 4.24E-09) and closeness centrality (Coefficients = 114.6901, P-Value = 0.009965) and a negative relationship between performance and degree centrality (Coefficients = −207.761, P-Value = 2.77E-05). The regression coefficients of each predicting variable showed that Closeness centrality and Betweenness centrality measure can significantly explain the variance of performance.
5.4. Identification of major civil engineering research topics
The goal of the text mining section is to discover the latent topics researched on in the most recent year, that is 2018, the topics researched on in the largest research community and finally, the topics focused on in the civil engineering research in Nigeria overall. The topics of the research extracted by the text mining and LDA algorithm are presented in Table .
Table 4. Topic trend in the most recent years
Although unnecessary keywords have been eliminated by the semantic text pre-processing step, the title of topics is determined with the help of experts and some of the topics consist of common words. From Table , it can be seen that in 2018, Topic 1 focuses on studying the strength of concrete, material, there is also interest in waste, material, and property with emphasis on using models for this study. Topic 2 also focuses on strength of concrete but in addition, words like cement is featured in this topic. Topic 3 still revolves around the strength of material but in addition, steel, construction, and Nigeria is added to the keywords. Topic 4 focuses on new words like building, agricultural, structure, and water. The new keyword introduced to topic 5 is mainly alloy. For the largest community, it can be seen from the list of keywords in the topics that they are similar to that discovered for topics in 2018 with few addition such as ceramic, glass. The topics from the total publications signifying the overall research content of civil engineering research in Nigeria as retrieved from Scopus database focuses are as follows: Topic 1 is focused on concrete, strength, cement, compressive, result, aggregate, property, study, and content. Topic 2 adds words such as, value, material, sample, and compaction Topic 3 is revolves around factors, models, analysis of buildings in Nigeria. Topic 4 centres on groundwater, magnetic field, source, quality, and control. Finally, Topic 5 is focused on building designs, construction.
6. Conclusion
This study reveals the structural characteristics of the civil engineering research co-authorship network in Nigeria over a period of 38 years. These 38 years of data collected is the overall publications of civil & structural engineering research in Nigeria as contained in the Scopus database, given a wide range of the data sample. This research demonstrates that the civil engineering collaboration network in Nigeria is quite complex and displays small-world properties which is sometimes referred to as “six degrees of separation”.
Khasseh et al. (Citation2017) carried out research using centrality measures to discover the influence of researchers and they were able to prove that centrality is effective in indicating scientific influence. In this study, it was discovered that K.N.A, Maulud, Afolabi, Ayo Samuel, Mgbemena, Chinedum Ogonna, Nnaji, Chidozie Charles, Soboyejo, Wolé O. ranked highest with respect to closeness centrality. The top broker authors based on betweenness centrality are Kupolati, Williams Kehinde, Busari, Ayobami Adebola, Popoola, Abimbola Patricia Idowu, Ede, Anthony N., and Awoyera, Paul Oluwaseun. These authors facilitate scientific collaborations within and outside the scientific community, signifying their importance in facilitating interdisciplinary research. For degree centrality, the top authors were discovered to be Mosaddeghi, Mohammad Reza, Ottoni Filho, Theophilo Benedicto, Hatzigiannakis, Evangelos G., Eskandari, Iraj, and Rotunno Filho, Otto Corrêa.
Also previous research by Liu et al. (2007), Cimenler et al. (Citation2014) and Khasseh et al. (Citation2017) has revealed that centrality measures have an effect on the performance of authors in a co-authorship network. This is further confirmed by our research, which discovered that there is significant positive relationship between performance and betweenness centrality and closeness centrality.
Unlike most studies on co-authorship network, our research combines the semantic text mining approach to further discover the research focus of the discovered co-authorship network. The text mining approach addresses issues of semantics in text clustering and provides better inference according to Blei et al. (Citation2003).
In the text mining section of the research, we were able to discover both the current research topics and the overall topics focused on in civil engineering research in Nigeria. For further work, since this study used only data collected from the Scopus database which can be considered as a limitation, other research can attempt to include publication data from Web of Science and Google Scholar.
Availability of data and material
The data used for the research are available on request.
Code availability
The code for analysing the data is available on request.
Disclosure statement
There is no conflict of interest that is known to the authors.
Additional information
Funding
Notes on contributors
Ibukun T Afolabi
Ibukun T. Afolabi holds a B.Sc in Engineering Physics, an M.Sc in Computer Science and a Ph.D. in Computer Science. Her specific area of research is in the field of Data & Text Mining, Programming and Business Analytics. She has supervised several undergraduate and postgraduate students in computer science research and published over 39 articles in International high impact journals and conferences indexed in Scopus and Web of Science. She is a member of the Computer Profession of Nigeria (CPN) and Nigeria Computer Society (NCS).
References
- Abbasi, A., Altmann, J., & Hossain, L. (2011). Identifying the effects of co-authorship networks on the performance of scholars: A correlation and regression analysis of performance measures and social network analysis measures. Journal of Informetrics, 5(4), 594–17. https://doi.org/10.1016/j.joi.2011.05.007
- Abbasi, A., Chung, K. S. K., & Hossain, L. (2012a). Egocentric analysis of co-authorship network structure, position and performance. Information Processing & Management, 48(4), 671–679. https://doi.org/10.1016/j.ipm.2011.09.001
- Abbasi, A., Hossain, L., & Leydesdorff, L. (2012b). Betweenness centrality as a driver of preferential attachment in the evolution of research collaboration networks. Journal of Informetrics, 6(3), 403–412. DOI:10.1016/j.joi.2012.01.002
- Abramo, G., D’Angelo, C. A., & Solazzi, M. (2011a). Are researchers that collaborate more at the international level top performers? An investigation on the Italian university system. Journal of Informetrics, 5(1), 204–213. https://doi.org/10.1016/j.joi.2010.11.002
- Abramo, G., D’Angelo, C. A., & Solazzi, M. (2011b). The relationship between scientists’ research performance and the degree of internationalization of their research. Scientometrics, 86(3), 629–643. https://doi.org/10.1007/s11192-010-0284-7
- Afolabi, I. T., Ezenwoke, A., & Ayo, C. K. (2017). Competitive analysis of social media data in the banking industry. International Journal of Advertising, 11(3), 183-201. DOI:10.1504/IJIMA.2017.085644
- Aibinu, A. A., & Odeyinka, H. A. (2006). Construction delays and their causative factors in Nigeria. Journal of Construction Engineering and Management, 132(7), 667–677. https://doi.org/10.1061/(ASCE)0733-9364(2006)132:7(667)
- Arif, T., Ali, R., & Asger, M. (2012). Scientific co-authorship social networks: A case study of computer science scenario in India. International Journal of Computer Applications, 52(12), 38–45. https://doi.org/10.5120/8257-1790
- Azondekon, R., Harper, Z. J., Agossa, F. R., Welzig, C. M., & McRoy, S. (2018). Scientific authorship and collaboration network analysis on malaria research in Benin: Papers indexed in the web of science (1996–2016). Global Health Research and Policy, 3(1), 11. https://doi.org/10.1186/s41256-018-0067-x
- Badar, K., Hite, J. M., & Badir, Y. F. (2013). Examining the relationship of co-authorship network centrality and gender on academic research performance: The case of chemistry researchers in Pakistan. Scientometrics, 94(2), 755. https://doi.org/10.1007/s11192-012-0764-z
- Basu, S., Mooney, R. J., Pasupuleti, K. V., & Ghosh, J. (2001, August 26–29). Evaluating the novelty of text mined rules using lexical knowledge. In Proceedings of the 7th ACM SIGKDD international conference on knowledge discovery and data mining (pp. 233–238). CAACM.
- Beaudry, C., & Schiffauerova, A. (2011). Impacts of collaboration and network indicators on patent quality: The case of Canadian nanotechnology innovation. European Management Journal, 29(5), 362–376. https://doi.org/10.1016/j.emj.2011.03.001
- Benckendorff, P., & Zehrer, A. (2013). A network analysis of tourism research. Annals of Tourism Research, 43, 121–149. https://doi.org/10.1016/j.annals.2013.04.005
- Blei, D. M., Ng, A. Y., & Jordan, M. I. (2003). Latent dirichlet allocation. Journal of Machine Learning Research, 3(Jan), 993–1022.
- Bordons, M., Aparicio, J., González-Albo, B., & Díaz-Faes, A. A. (2015). The relationship between the research performance of scientists and their position in co-authorship networks in three fields. Journal of Informetrics, 9(1), 135–144. https://doi.org/10.1016/j.joi.2014.12.001
- Bornmann, L., Mutz, R., Neuhaus, C., & Daniel, H. D. (2008). Citation counts for research evaluation: Standards of good practice for analyzing bibliometric data and presenting and interpreting results. Ethics in Science and Environmental Politics, 8(1), 93–102. https://doi.org/10.3354/esep00084
- Chen, W. F., & Liew, J. R. (2002). The civil engineering handbook. CRC Press.
- Cimenler, O., Reevesa, K. A., & Skvoretz, J. (2014). A regression analysis of researchers’ social network metrics on their citation performance in a college of engineering. Journal of Informetrics, 8(3), 667–682. https://doi.org/10.1016/j.joi.2014.06.004
- Crescenzi, R., Nathan, M., & Rodríguez-Pose, A. (2016). Do inventors talk to strangers? On proximity and collaborative knowledge creation. Research Policy, 45(1), 177–194. https://doi.org/10.1016/j.respol.2015.07.003
- Cugmas, M., Ferligoj, A., & Kronegger, L. (2016). The stability of co-authorship structures. Scientometrics, 106(1), 163–186. https://doi.org/10.1007/s11192-015-1790-4
- De Stefano, D., Fuccella, V., Vitale, M. P., & Zaccarin, S. (2013). The use of different data sources in the analysis of co-authorship networks and scientific performance. Social Networks, 35(3), 370–381. https://doi.org/10.1016/j.socnet.2013.04.004
- Dehdarirad, T., & Nasini, S. (2017). Research impact in co-authorship networks: A two-mode analysis. Journal of Informetrics, 11(2), 371–388. https://doi.org/10.1016/j.joi.2017.02.002
- Didegah, F., & Thelwall, M. (2013). Which factors help authors produce the highest impact research? Collaboration, journal and document properties. Journal of Informetrics, 7(4), 861–873. https://doi.org/10.1016/j.joi.2013.08.006
- Ebadi, A., & Schiffauerova, A. (2015). How to become an important player in scientific collaboration networks? Journal of Informetrics, 9(4), 809–825. https://doi.org/10.1016/j.joi.2015.08.002
- Ferligoj, A., Kronegger, L., Mali, F., Snijders, T. A., & Doreian, P. (2015). Scientific collaboration dynamics in a national scientific system. Scientometrics, 104(3), 985–1012. https://doi.org/10.1007/s11192-015-1585-7
- Franceschet, M., & Costantini, A. (2010). The effect of scholar collaboration on impact and quality of academic papers. Journal of Informetrics, 4(4), 540–553. https://doi.org/10.1016/j.joi.2010.06.003
- Garfield, E. (2014). 50 years of citation indexing: A visit with Dr. Eugene Garfield. Thompson Reuters. https://www.youtube.com/watch?v=2kZ0_5HTYDQ
- Guan, J., & Liu, N. (2016). Exploitative and exploratory innovations in knowledge network and collaboration network: A patent analysis in the technological field of nano-energy. Research Policy, 45(1), 97–112. https://doi.org/10.1016/j.respol.2015.08.002
- Guan, J., Yan, Y., & Zhang, J. J. (2017). The impact of collaboration and knowledge networks on citations. Journal of Informetrics, 11(2), 407–422. https://doi.org/10.1016/j.joi.2017.02.007
- Guan, J., Zhang, J., & Yan, Y. (2015). The impact of multilevel networks on innovation. Research Policy, 44(3), 545–559. https://doi.org/10.1016/j.respol.2014.12.007
- Han, J. (2005). Data mining: Concepts and techniques. Morgan Kaufmann Publishers Inc.
- He, B., Ding, Y., Tang, J., Reguramalingam, V., & Bollen, J. (2013). Mining diversity subgraph in multidisciplinary scientific collaboration networks: A meso perspective. Journal of Informetrics, 7(1), 117–128. https://doi.org/10.1016/j.joi.2012.09.005
- Irfan, R., King, C. K., Grages, D., Ewen, S., Khan, S. U., Madani, S. A., Tziritas, N., Chen, D., Rayes, A., Tziritas, N., Xu, C.-Z., Zomaya, A. Y., Alzahrani, A. S., Li, H., & Kolodziej, J. (2015). A survey on text mining in social networks. The Knowledge Engineering Review, 30(2), 157–170. https://doi.org/10.1017/S0269888914000277
- Khasseh, A. A., Soheili, F., & Mousavi Chelak, A. (2017). Co-authorship network analysis of iMetrics researchers. Library Philosophy and Practice (E-journal), 1496, 1-16. http://digitalcommons.unl.edu/libphilprac/1496
- Kolaczyk, E. D., & Csárdi, G. (2014). Statistical analysis of network data with R (Vol. 65). Springer.
- Latapy, M. (2008). Main-memory triangle computations for very large (Sparse (Power-Law)) graphs. Theoretical Computer Science, 407(1–3), 458–473. https://doi.org/10.1016/j.tcs.2008.07.017
- Lee, W. S., Han, E. J., & Sohn, S. Y. (2015). Predicting the pattern of technology convergence using big-data technology on large-scale triadic patents. Technological Forecasting and Social Change, 100, 317–329. https://doi.org/10.1016/j.techfore.2015.07.022
- Li, E. Y., Liao, C. H., & Yen, H. R. (2013). Co-authorship networks and research impact: A social capital perspective. Research Policy, 42(9), 1515–1530. https://doi.org/10.1016/j.respol.2013.06.012
- Liao, C. H. (2010). How to improve research quality? Examining the impacts of collaboration intensity and member diversity in collaboration networks. Scientometrics, 86(3), 747–761. https://doi.org/10.1007/s11192-010-0309-2
- Liu, F., & Xiong, L. (2011, July 15–17). Survey on text clustering algorithm: Research present situation of text clustering algorithm. In Proceedings of the IEEE 2nd international conference software engineering and service science (pp. 196–199). IEEE.
- Manganote, E. J., Araujo, M. S., & Schulz, P. A. (2014). Visualization of ranking data: Geographical signatures in international collaboration, leadership and research impact. Journal of Informetrics, 8(3), 642–649. https://doi.org/10.1016/j.joi.2014.05.005
- Marsden, P. V. (2002). Egocentric and sociometric measures of network centrality. Social Networks, 24(4), 407–422. https://doi.org/10.1016/S0378-8733(02)00016-3
- Morescalchi, A., Pammolli, F., Penner, O., Petersen, A. M., & Riccaboni, M. (2015). The evolution of networks of innovators within and across borders: Evidence from patent data. Research Policy, 44(3), 651–668. https://doi.org/10.1016/j.respol.2014.10.015
- Mostafa, M. M. (2013). More than words: Social networks’ text mining for consumer brand sentiments. Expert Systems with Applications, 40(10), 4241–4251. https://doi.org/10.1016/j.eswa.2013.01.019
- Nazarnia, H., & Sarmasti, H. (2018). Characterizing infrastructure resilience in disasters using dynamic network analysis of consumers’ service disruption patterns. Civil Engineering Journal, 4(10), 2356–2372. https://doi.org/10.28991/cej-03091165
- Ogunde, A. O., Dafe, O. E., Akinola, G. A., Ogundipe, K. E., Oloke, O. C., Ademola, S. A., Akuete, E., & Olaniran, H. F. (2017). Factors militating against prompt delivery of construction projects in Lagos megacity, Nigeria: Contractors’ perspective. Mediterranean Journal of Social Sciences, 8(3), 233. https://doi.org/10.5901/mjss.2017.v8n3p233
- Ogunsemi, D. R., & Jagboro, G. O. (2006). Time cost model for building projects in Nigeria. Construction Management and Economics, 24(3), 253–258. https://doi.org/10.1080/01446190500521041
- Oliveira, S. C., Cobre, J., & De Paula Ferreira, T. (2017). A Bayesian approach for the reliability of scientific co-authorship networks with emphasis on nodes. Social Networks, 48, 110–115. https://doi.org/10.1016/j.socnet.2016.06.005
- Ortega, J. L. (2014). Influence of co-authorship networks in the research impact: Ego network analyses from Microsoft Academic Search. Journal of Informetrics, 8(3), 728–737. https://doi.org/10.1016/j.joi.2014.07.001
- Paruchuri, S. (2010). Intraorganizational networks, interorganizational networks, and the impact of central inventors: A longitudinal study of pharmaceutical firms. Organization Science, 21(1), 63–80. https://doi.org/10.1287/orsc.1080.0414
- Perc, M. (2010). Growth and structure of Slovenia’s scientific collaboration network. Journal of Informetrics, 4(4), 475–482. https://doi.org/10.1016/j.joi.2010.04.003
- Racherla, P., & Hu, C. (2010). A social network perspective of tourism research collaborations. Annals of Tourism Research, 37(4), 1012–1034. https://doi.org/10.1016/j.annals.2010.03.008
- Ricketts, J. T., Loftin, M. K., & Merritt, F. S. (2003). Standard Handbook for civil engineers (Handbook). McGraw-Hill Professional.
- Sadoughi, F., Valinejadi, A., Shirazi, M. S., & Khademi, R. (2016). Social network analysis of Iranian RESEARCHERS ON MEDICAL parasitology: A 41 year co-authorship survey. Iranian Journal of Parasitology, 11(2), 204.
- Song, B., & Suh, Y. (2018). Identifying convergence fields and technologies for industrial safety: LDA based network analysis.. Technological Forecasting and Social Change, 138,(2019), 115-126. https://doi.org/10.1016/j.techfore.2018.08.013
- Suh, Y., Kim, G., & Seol, H. (2017). Roadmapping for prioritisation of smartphone feature requirements based on user experiences. Technology Analysis & Strategic Management, 29(8), 886–902. https://doi.org/10.1080/09537325.2016.1259468
- Uddin, S., Hossain, L., Abbasi, A., & Rasmussen, K. (2012). Trend and efficiency analysis of co-authorship network. Scientometrics, 90(2), 687–699. https://doi.org/10.1007/s11192-011-0511-x
- Velden, T., Haque, A. U., & Lagoze, C. (2010). A new approach to analyzing patterns of collaboration in co-authorship networks: Mesoscopic analysis and interpretation. Scientometrics, 85(1), 219–242. https://doi.org/10.1007/s11192-010-0224-6
- Wang, C., Rodan, S., Fruin, M., & Xu, X. (2014). Knowledge networks, collaboration networks, and exploratory innovation. Academy of Management Journal, 57(2), 484–514. https://doi.org/10.5465/amj.2011.0917
- Wasserman, S., & Faust, K. (1994). Structural analysis in the social sciences. Social network analysis: Methods and applications. Cambridge University Press. https://doi.org/10.1017/CBO9780511815478
- Yan, E., & Ding, Y. (2009). Applying centrality measures to impact analysis: A coauthorship network analysis. Journal of the American Society for Information Science and Technology, 60(10), 2107–2118. https://doi.org/10.1002/asi.21128
- Yayavaram, S., & Ahuja, G. (2008). Decomposability in knowledge structures and its impact on the usefulness of inventions and knowledge-base malleability. Administrative Science Quarterly, 53(2), 333–362. https://doi.org/10.2189/asqu.53.2.333
- Zachary, W. W. (1977). An information flow model for conflict and fission in small groups. Journal of Anthropological Research, 33(4), 452–473. https://doi.org/10.1086/jar.33.4.3629752