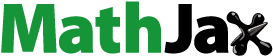
Abstract
This study focuses on the impact of the unexpected events of the marine casualties in Taiwan international ports and aims to develop an undesirable-output Data Envelopment Analysis (DEA) model to evaluate navigation safety and acquires the improvement in marine casualties that affect the performance of port safety. Moreover, the major difficulties in this study are the data collection and classification for each marine casualties, the selection of key parameters and weighted value for assessment model. Then, the undesirable-output DEA can conquer the dilemma and render marine casualties be negative factor. This paper searches the main cause about marine casualties adopted by R.O.C. Ministry of Transportation and Communications (MOTC). Based upon 899 sets of marine casualties collected from 2010 to 2019 in Taiwan international ports water, an assessment model comprising four international ports is built, each of which is composed of an input variable and eleven undesirable-output variables. Finally, the results show that: (1) The method which was demonstrated on Taiwan international ports indicates navigation safety ordering is Hualien port, Keelung port, Taichung port and Kaohsiung port. (2) All of the marine casualties, collision and structural damage/ship sinking are the severest casualty that should be improved. Furthermore, the findings of this study correspond to reality so it can help the port authority to take effective risk control actions to increase the navigation safety of international ports.
PUBLIC INTEREST STATEMENT
These days the maritime accidents are more frequently around the world at sea. With the growth of the demand for shipping, maritime accidents are occurring more frequently and seriously, the value of marine insurance claims are getting higher, and marine pollution is becoming more serious. In order to ensure safety at sea, for instance, preventing human injury or death and to protect the marine environment, many studies dedicated a lot of resources to discuss how to promote navigation safety. However, the risk of navigation at sea was found very high. This article utilized undesirable-output DEA Model to evaluate the port safety in Taiwanese international ports. The results of this study are that the undesirable-output DEA model could apply to evaluate the port navigation safety, and acquire the respective performance and improvement of each port’s safety. In the end, appropriate recommendations have been forwarded in this paper.
1. Introduction
Shipping brings huge economical effect, there are about 90% of the goods traded worldwide (UNCTAD, Citation2016; Corporate, Citation2019), which is one of the most important transportation approaches (Christiansen et al., Citation2007). The shipping industry has a huge economic impact, and it is responsible for the flow of about 90% of all goods traded worldwide. There are around 60,000 merchant ships worldwide transporting cargo of every kind. The world fleet is registered in over 150 nations, and manned by over a million seafarers; naturally, this makes the safety of the vessels extremely critical (Corporate, Citation2019). Moreover, the international seaborne trade gathered momentum, with volumes expanding by 4%; the growth was the fastest in five years (UNCTAD, Citation2018). Shipping was also known as maritime transportation via ships and seafarers to complete its process. While merchant vessels have had continuously increases in demand over the past decades with the development of the global economy, it has also encountered massively increasing risks and uncertainties (Chai et al., Citation2017). IMO indicates that while shipping is perhaps the most international of all the world great industries, it is also one of the most dangerous (Faghih-Roohi et al., Citation2014; IMO, Citation2004; Sang et al., Citation2015; Wang & Lee, Citation2012a; Zhang et al., Citation2016a, Citation2015). As the result, IMO establishes that the best method of improving safety at sea is by developing international regulations that are followed by all shipping nations. While enormous endeavors are devoted towards the objective of navigation safety, the occurrence of merchant vessel tragedies remains at a high level worldwide.
An overview published by European Maritime Safety Agency (EMSA) states that between 2011 and 2017 there were a total of 405 accidents leading to a total of 683 lives lost, which represents a total of 3,301 maritime casualties through 2017 (EMSA, Citation2018). Crew represent the largest category of persons injured at sea, there were 5,329 cases of crew injury during the 2011–2017 period. According to Safety and Shipping Review 2019, there have been 8,862 machinery damage incidents within the last 10 years, up by more than a third over this period. The value of 230,000 marine insurance industry claims in five years amounts to almost $10 billions. Ship sinking/collision incidents account for 16% of this total, 174 fire incidents were reported in 2018 (Corporate, Citation2019). Failure in operations would cause marine casualties and conduct in the marine environment may pose various major risks in terms of environmental pollution and cause shipping companies to lose assets as well as cause human casualties. Therefore, a great deal of research on the improvement of marine safety is necessary (Tseng et al., Citation2017).
This paper spent 3 months to collect and classify 899 sets of unexpected marine casualties occurred from 2010 to 2019 within Taiwanese international ports. According to R.O.C. MOTC the marine casualties were separate into eleven kinds of event, there were collision, structural damage/ship sinking, stranding, fire, explosion, leaking, capsized, mechanical failure, extra-ordinary, others and injured/death. These were 60 injured or death events, caused much marine insurance claims and pollution. For the purpose of evaluating the maritime risk performance, and rendering the unexpected marine casualties be undesirable-output variables, this study adapted DEA to navigation safety assessment model.
Data Envelopment Analysis (DEA) is a linear programming technique initially developed by Charnes, Cooper and Rhodes to evaluate the efficiency of public sector non-profit organizations (Charnes et al., Citation1978). DEA developed by Charnes et al., provides a nonparametric methodology for evaluating the efficiency of each set of comparable decision making units (DMUs) (Cooper et al., Citation2001). In the original model of Charnes et al., efficiency is represented by the ratio of weighted outputs to weighted inputs. An important feature of DEA is that it can provide efficiency scores, while taking account of both multiple inputs and multiple outputs (Sathye, Citation2003). DEA has been widely applied to address various decision analysis problems due to its usefulness in evaluating multi-criterion systems and providing improvement targets for such system (J. Liu et al., Citation2000). It can transform multiple resources into multiple problems. The efficiency has been calculated using variable returns to scale input-oriented model of the DEA methodology.
This paper aims to analyse the characteristics of the maritime casualties that occurred along international ports in Taiwan and to develop a navigation safety assessment and prediction model based on a data-driven undesirable-output DEA model. With the statistics from R.O.C. Ministry of Transportation and Communications (MOTC), the study constructs a logical structure based on the undesirable-output DEA model providing the relationship between international ports and marine casualties. The conclusions gained from this study is valuable for shipping companies, seafarers who provide services on a ship, academia and government in the aspect of enhancing navigation safety around ports.
The remainder of this article is organized as follows. Section 2 provides background about the research of marine casualties, in particular its integration with methodology. Section 3 presents the description of the research problem. In Section 4, an introduction to undesirable-output DEA model, the database in the context of R.O.C. MOTC are presented with assessment and case description. Section 5 demonstrates the application of the methodology in a real case study and Section 6 concludes the paper and provides the main findings and recommendations for possible future studies.
2. Literature review
2.1. Maritime casualties and incidents
For collecting information on ship casualties, IMO has established a module database called the Global Integrated Shipping Information System (GISIS). It includes a maritime casualties and incidents module database, which has data on Maritime Casualties and Incidents (MCI). In order to collect all types of casualties and populate the validity of GISIS module, IMO classifies ship casualties as “very serious casualties”, “serious casualties”, “less serious casualties” and “marine incidents”.Footnote1
European Maritime Safety Agency (EMSA) defines casualty events as “unwanted events in which there was some kind of energy release with impact on people and/or ship including its equipment and its cargo or environment” (EMSA, Citation2018). They are classified as Capsizing/Listing, Collision, Contact, Damage to equipment, Grounding/stranding, Fire/explosion, Flooding/foundering. Allianz Global Corporate & Specialty (AGCS) uses classification similar to EMSA, its marine casualty involves Foundered (sunk), Wrecked/stranded (grounded), Fire/explosion, Machinery damage/failure, Collision (involving vessels), Hull damage (holed cracks etc.), Miscellaneous, Contact (e.g., port wall), Piracy, Missing/overdue. Furthermore, according to the Allianz Safety & Shipping Review 2019, foundered (sunk/submerged), wrecked/stranded, fire/ explosion, machinery damage, and collision are the most frequent causes of total losses over the past decade, accounting for over 90% of all reported cases (Corporate, Citation2019; EMSA, Citation2018). A marine casualty analysis for ship disasters in Turkish territorial waters between 2005 and 2015 indicates that collision is the most common accident type (Bolat, Citation2016).
2.2. Previous research
The causes of maritime accidents mainly include environmental effects, human factors, and ship conditions or a combination of these factors (Jiang et al., Citation2020). Recently, ship sizes of new deliveries continued to be larger than the existing fleet (EMSA, Citation2018). The increasing maritime transportation activities can cause higher maritime traffic density and more complex maritime traffic, thereby increasing occurrence rate of maritime accidents, especially collision accidents (Z. Liu et al., Citation2019; Twrdy et al., Citation2013). Ung (Citation2019) demonstrated that in Canada from 2007 to 2016 the most frequent type of shipping accidents was collision with the occurrence rate of 30.20% in 2649 casualties. According to Allianz Safety & Shipping Review 2019 and Annual Overview of Marine Casualties and Incidents 2018 many statistics, while many types of maritime casualties took place worldwide, the most frequent type to happen is collision. Then, this phenomenon also had occurred in Taiwan so this study devoted immense efforts to enhance navigation safety wherever possible.
With the increasing number of casualties and incidents occurring around the world, many scholars began to study the causes or accident investigations of the events. A common orientation to safety-focused investigations, especially the independent accident investigation were established that can provide a powerful diagnostic tool for reducing the peril of drowning (Stoop, Citation2003). The structure of the offshore platform has a certain influence on the safety of maritime navigation (Qasim & Hasan, Citation2020), which will affect the safety of Taiwan’s waters with the increasing offshore wind power project along the western coast of Taiwan. The rising levels of seas and oceans could directly affect the serviceability of infrastructures of coastal regions; effects may include damaging port facilities, and port safety (Nazarnia et al., Citation2020). Especially, Ship Registries play an important role in the maintenance of ship safety, for example, of Covid-19 pandemic, some of the actions taken can be regarded as a temporary reply of the maritime industry to avoid the threats imposed by the disease (Saviolakis & Pazarzis, Citation2021).
Many researches describe collisions as the most common type of accident, moreover the limitation and restrictions of the port was significant (Goerlandt et al., Citation2017; Szlapczynski, Citation2015; Tian et al., Citation2020; Ung, Citation2019; Zhang et al., Citation2018a, Citation2016b). J Chen et al. (Citation2019) used an improved entropy weight-TOPSIS model and selected the total-loss marine accidents that occurred in the world from 1998 to 2018, to find the main influential factors are foundering, stranding and fires/explosions. By the way, Twrdy et al. (Citation2013) adopt ship-handling simulator to canvass maneuvering safety. In accordance with the provisions of the United Nations Convention on the Law of the Sea (United Nations, Citation1982), several states have implemented additional mechanisms to ensure the safety of navigation in their waters, due to specific local conditions such as the presence of sea ice, restricted waters, bad weather or busy water (Wang & Lee, Citation2012b; Zhang et al., Citation2018b).
In terms of maritime accident risk analysis, the IMO proposed a structured and systematic framework called formal safety assessment (FSA). The FSA is a structured and systematic methodology, aimed at improving maritime safety, including the protection of life, health, the marine environment and property, by using risk analysis and cost-benefit assessment (Jiang et al., Citation2020, IMO). Many researchers have utilized it to identify risk factors in special waters (Balmat et al., Citation2009; Banda et al., Citation2016; Fera & Macchiaroli, Citation2010; Hu et al., Citation2007; Hu & Zhang, Citation2012; J. Wang & Foinikis, Citation2001). Although it is widely used, FSA has some drawbacks, such as the inability to accurately quantify risks and the lack of reliability and effectiveness, when subjective knowledge is used in the absence of historical data (Jiang et al., Citation2020).
In order to ensure safety at sea, for instance, preventing human injury or death and to protect the marine environment, many studies dedicated large amounts of resources to discuss how to promote navigation safety in international port waters. A number of indexes have been used to evaluate ship navigation safety such as the navigational features, the geographical environment, the navigation process, the maritime navigation safety, efficiency system, the number of marine accidents, death of seafarers and missing, the density of vessel traffic and weather conditions (Akten, Citation2004; ElSayed et al., Citation2011; C. P. Liu et al., Citation2006; Twrdy et al., Citation2013; Urbański et al., Citation2008; Wang & Lee, Citation2012a, Citation2012b).
Recently, some researchers employed the DEA model for navigation safety application, which got a practicable result, and according to the structure of the model, the input variable was the amount of berth or dummy variable, output variables were a variety of marine casualties occurred at sea by ships (Wang & Lee, Citation2012a, Citation2012b). In the same way, Gan et al. (Citation2017) proposed using undesirable outputs DEA model as a means to evaluate Changjiang maritime safety. The model covered six varieties of accident that were collision, contact, stranding, fire, foundering, and all seem as undesirable outputs, and no explicit input variable.
Navigation safety is a critical issue that has led many researchers to discuss how to promote and develop many different kinds of model via methodology such as SWOT, Fuzzy Analytic Hierarchy Process, Event Sequence Diagram, simulator, Data Envelopment Analysis, Gray Relation Analysis, Artificial potential field, Bayesian network approach, Cognitive Reliability Error Analysis, Success Likelihood Index Method, Fault Tree Analysis, Human Error Assessment and Reduction Technique, Analytic Hierarchy Process (Afenyo et al., Citation2018; Akyuz, Citation2015, Citation2016; Akyuz & Celik, Citation2014, Citation2015; Randić et al., Citation2015; Twrdy et al., Citation2013; Ung, Citation2019; Wang & Lee, Citation2012a, Citation2012b, Citation2010; Wang et al., Citation2020; Xie et al., Citation2019; Yang et al., Citation2013). While the aforementioned studies have the capability of establishing prediction modelling particularly in situations where the lack of data exists, but such models lack the consideration of input weights and marine casualties are undesirable.
3. Description of the research problem
Clearly, along with the development of the navigation technology, the demand for shipping transportation and international logistics is significantly increased. This increase results in heavier traffic along the ports, which in return causes higher navigational safety and security concerns. As increasing numbers of merchant ships navigate in Taiwan waters, the safety in port waters is necessary for both economic and environmental purposes. This paper aims to provide a comprehensive analysis of navigation safety efficiency and improvement strategy of port waters safety.
This phenomenon reflected in Taiwan waters, from 1992 to 2016 where 34.98% ~40.31% of the shipping disasters taking place of Taiwanese merchant shipping tragedies are attributed to collision according to Ministry of Transportation and Communications (MOTC). Despite the massive investment to improve the safety and security of Taiwan international port waters in the past years, we have seen a large number of shipping disasters and casualties occurred along the port waters.
After consulting previous studies, there were some dilemmas such as lack of objective weights to assess variables, loss of reliability from expert, insufficient information for casualty risk and immoderation descriptive statistical analysis (Gan et al., Citation2017; Huang et al., Citation2021; Wang & Lee, Citation2012a, Citation2012b). However, marine casualties do not occur ordinarily or regularly and researchers rarely take marine casualties as undesirable output to determine navigation safety. As a result, traditional methodologies for data analysis are not suited to evaluate navigation safety. For that purpose, this study chose undesirable-output DEA model as the tool for the study on navigation safety in order to analyze marine accidents in relation to accident sites.
Some researchers employed the DEA model for navigation safety application which got a practicable result, and according to the structure of the model the input variable was the amount of port physical facility, output variables were marine casualties. The undesirable outputs DEA model can readily apply to estimate port safety performance. Moreover, the undesirable-output DEA model can conquer the aforementioned puzzles by providing objective weights and subsequently applied to assess the performance of port safety. In order to examine the correlation between the international ports where the casualties occurred and types of marine casualties, this study demonstrates on ports in Taiwan. Based on the DEA model the suitable assumption is that the DMUs are Taiwan international ports, the casualty types are undesirable output variables, and the input variable is the amount of berths. The prototype of DEA has been widely applied to address various decision analysis problems due to its usefulness in evaluating multi-criterion systems and providing improvement targets. Therefore, this study applies undesirable-output DEA model and considered its effects on negative factors to assess the safety efficiency of Taiwan international ports.
4. Research methodology
4.1. Data envelopment analysis
In contrast to the parametric approach, non-parametric methods disregard measurement errors without assumption of the functional form (Huang et al., Citation2021). Data envelopment analysis (DEA), first introduced by Charnes et al. in 1978 is an effective non-parametric mathematical optimization technique for measuring the relative efficiency of a group of peer decision making units (DMUs) with multiple inputs and outputs (Charnes et al., Citation1978). The most well-known DEA models are CCR model and BCC model those all based upon the constant returns to scale (CRS) assumption. Therefore, DEA is a mathematical method for measuring the relative efficiencies of peer DMUs with multiple inputs and outputs. It maximizes the efficiency score with a range from zero to one that determine efficient performance for each DMU by choosing a set of weights for all inputs and outputs under evaluation among all DMUs (Färe et al., Citation1989). DEA is considered as a well appropriate holistic approach when analyzing port production and performance and has been used by more and more scholars (Gan et al., Citation2017; Huang et al., Citation2021; Wang & Lee, Citation2012a, Citation2012b).
However, the traditional DEA model neglects evaluating the undesirable output to lead to the failure to ranking all DMUs. Whereas multilateral productivity comparisons of firms producing multiple outputs, some of which are undesirable, Färe et al. obtained to make the modification of nonparametric approach to measure the undesirable outputs (Färe et al., Citation1989). The modification permits an asymmetric treatment of inputs, desirable outputs and undesirable outputs. Moreover, the enhanced multilateral productivity index utilizes proxies of the implied prices as the undesirable outputs. Färe and Grosskopf adopted a directional distance function that may be estimated by using the usual linear programming techniques employed in undesirable outputs DEA (Färe & Grosskopf, Citation2004).
In DEA literature, there already existed much research concerning applications with undesirable outputs. Furthermore, this approach holds that undesirable outputs can be reduced to increase the efficiency score at will of management (Guo & Wu, Citation2013). DEA is an efficient approach for exploring the internal relationships between output and input variables. The main contribution of this approach is to provide quantitative data regarding methods of improvement (W. B. Liu et al., Citation2010; You & Yan, Citation2011). Then, this study attempted to provide a multiple outputs evaluation method for the safety performance in Taiwan international ports as well as improving safety management levels. Our study established a DEA model for the evaluation of port safety performance based on undesirable output data.
Based on the concept of sustainable design efficiency, this study consider a set of n different designs of a particular product class (motor vehicles, music devices, smart phones, etc.) as the decision making units (DMUs) (C. Chen et al., Citation2012). Assume that for each product design, denoted by DMUj (j = 1, …, n), there are m relevant product specifications as inputs as well as s performance attributes as the desirable outputs (e.g. size of a motor vehicle, storage capacity of a portable music devices) and undesirable outputs (e.g. environmental impacts such as carbon footprint and the amount of e-waste). Let
denote the index of desirable outputs and
denote the index of undesirable outputs. The ith input of the kth DMU is denoted by
. The rth desirable output of the kth DMU is denoted by
, and the
undesirable output of the kth DMU is denoted by
. To increase the desirable outputs and decrease undesirable outputs simultaneously, Färe et al. modified the variable returns to scale (VRS) model of Banker, Charnes, and Cooper output oriented model into the following non-linear programming problem, which could be modelled as the formula (1) (Banker et al., Citation1984; Färe & Grosskopf, Citation2004; Färe et al., Citation1989).
Where
the weight for output and input
the amount of output
of DMUj;
the amount of input
of DMUj;
the number of outputs;
the number of inputs;
the number of DMUs;
the
desirable output of DMUk; and
the
undesirable output of DMUk.
Inspired by the above nonlinear model, this study developed the following new model for treating desirable and undesirable outputs differently under the assumption of variable returns to scale (VRS) by using as the surrogate of
in the constraints of desirable outputs and
as the surrogate of
in the constraints of undesirable outputs when maximizing the scaling factor (Färe et al., Citation1989). Then, function (1) can convert into a linear programming problem as the formula (2):
Since both formula (1) and (2) do not consider slacks, an efficient DMU may have nonzero slacks. The following formula incorporates slacks into the formula (2):
The output-oriented efficiency score from formula (3) can calculate as:
4.2. Assessment model description
According to some researches using DEA to evaluate navigation safety, they selected collision, contact, grounding, stranding, fire, mechanical failure, injured/death and foundering to be the decisive variables (Gan et al., Citation2017; Wang & Lee, Citation2012a, Citation2012b). The undesirable outputs DEA model proposed to be a means of evaluating Changjiang maritime safety (Gan et al., Citation2017). Similarly, the DEA model evaluated the security of international commercial harbours in Taiwan (Wang & Lee, Citation2012a, Citation2012b). These authors have asserted that their research results could demonstrate the correlation between the conditions of their investigation and practical scenarios. Referring to the database of MOTC, the classification of maritime casualty are collision; structural damage/ship sinking, stranding, fire, explosion, leaking, capsized, mechanical failure, extra-ordinary, others, and injured/death. Meanwhile, this model adopted maritime casualty to be the output variables.
The input variables were another key thing to remember in this assessment model. Meanwhile, the total quay length, terminal area, the number of gantry cranes, yard gantry crane, straddle carriers, container throughput and infrastructure construction are most suitable to incorporate input variables (Huang et al., Citation2021). According to the reality and public information of Taiwanese port and, the input variable of this study were the amount of berth. Then, the four international ports in Taiwan consisting of Keelung port, Kaohsiung port, Taichung port, and Hualien port were transformed into DMUs. According to above, the proposed undesirable outputs DEA model furnished clearly study design to evaluate navigation safety as shown in .
4.3. Case description
The surroundings safety of international ports is vital import to transportation. Meanwhile, this study has collected shipping accident data from the R.O.C. MOTC. This database has records of marine casualties that have occurred in Taiwan’s international ports and describes what kind of maritime casualty it was. For illustrating the navigation safety of international ports, this study selected complete date from MOTC to assess between 2010 and 2019; each set of the recorded data includes the following information: (i) Berth; (ii) Collision; (iii) Structural damage/ship sinking; (iv) stranding; (v) Fire; (vi) Explosion; (vii) Leaking; (viii) Capsized; (ix) Mechanical failure; (x) Extra-ordinary; (xi) Others; (xii) Injured/death.
A total number of shipping casualties occurred in Taiwan waters between 2010 and 2019 are 899. Of these casualties, Keelung port had 56 berths, 62 collision, 69 structural damage/ship sinking, 8 stranding, 1 fire, 3 mechanical failure, 2 extra-ordinary, 24 others, 8 injured/death. The total amount of casualties in Keelung port is 177, 472 in Kaohsiung, 202 in Taichung, and 48 in Hualien. The above descriptive statistics of input and output variables selected for our study comprehensively summarized in .
Table 1. Descriptive statistics of input and output variables
The collision and structural damage/ship sinking are the main types of marine casualties in Keelung port, Kaohsiung port and Taichung port. Meanwhile, the amount of marine casualties of Kaohsiung port had occupied nearly half proportion of the marine casualties in Taiwan international ports. Besides collision, structural damage/ship sinking is the most frequent marine casualty, subsequent is others. Moreover, collisions have been one of the most common types of accidents in Kaohsiung port, and structural damage/ship sinking have been one of the most common types of accidents in Taichung port, the same as Hualien port and Keelung port. From the overall perspective, collision and structural damage/ship sinking are ranking in the front of the other casualties.
It is worth noting that in regards to structural damage/ship sinking, Kaohsiung and Taichung port is higher than overall average performance. Compared to all other international ports in Taiwan, the performance of Kaohsiung port is about the total number of collision, structural damage/ship sinking, mechanical failure, and injured/death casualties is the highest. In Kaohsiung port, the highest marine casualties were collision and structural damage/ship sinking (as shown in ).
5. Discussion
This research adapted undesirable-output DEA to evaluate navigation safety in Taiwan international ports. All types of marine casualties are rendering the undesirable output variables. The collected statistical data ranges from 2010 to 2019, derives from four international ports in Taiwan consisting of Keelung port, Kaohsiung port, Taichung port, and Hualien port which were then transformed into decision making units (DMUs). In terms of assessing effectiveness, it is quite obvious that real input variables are better than dummy variables (Wang & Lee, Citation2012a, Citation2012b). However, considering the sensitivities of these parameters on the results, the undesirable-output DEA model is better than traditional DEA model (Gan et al., Citation2017). Based on previous researches, this study applied undesirable-output DEA model and selected the amount of berth to be the input variable. This study constructed an undesirable-output DEA model with one input variable, eleven output variables and four DMUs.
For recognizing the distribution state of input variable and output variables related to each DMU, this study integrates a statistical analysis (as shown in ) linked to the statistical data, in terms of the maximum, minimum, average and standard deviation, respectively. For example, the maximum value of input variable is 118, minimum value is 25, average value is 62.25, and the value of the standard deviation is 34.223.
Table 2. Statistical analysis of input and output variables
So as to obtain the navigation safety performance of all international ports in Taiwan, this paper utilized DEA model with undesirable outputs (as shown in formula (3)). presents the projections, constraints and slacks of each variables that compared by all DMUs, reference to the undesirable-output DEA model. The input variable amount of projection, constraint and slack is 25, −3.2E-14, 31. Then, shows the value of , efficiency score and ranking that were calculated by formula (3).
Table 3. The value of projection, constraint and slack of undesirable-output DEA model
Table 4. The calculated result of , efficiency score and ranking
According to the extension from formula (1) and formula (2), we utilized formula (3) that corrected with the incorporated slacks to calculate each DMU’s efficiency score. After being calculated using formula (3), we obtain value and efficiency score of each DMUs. A series of
value for Keelung, Kaohsiung, Taichung and Hualien port are determined to be 0.411329, 0.673235, 0.517328 and 0. The efficiency score for Keelung, Kaohsiung, Taichung and Hualien port are determined to be 0.708552, 0.597645, 0.659053 and 1.0. The result being ranking the efficiency score from high to low is Hualien port, Keelung port, Taichung port and Kaohsiung port.
In spite of the difference of input and output variables between Keelung, Kaohsiung, Taichung, and Hualien port are conspicuous, formula (3) and (4) can calculate the value of ,efficiency Score and Ranking of all DMUs. Having said that the
value and the efficiency score for Keelung, Kaohsiung, Taichung and Hualien port are a little difference, the DEA model could calculate the correction of undesirable outputs to ameliorate the efficiency score of each DMUs.
The DEA model would not only provide efficiency score, but also demonstrate the improvement quantity of each DMUs to advance the performance of navigation safety. The value of slack means the further reduction in outputs that could be gained beyond that implied by the radial projection. According to , the slack of each outputs in Keelung port, collision is 14, structural damage/ship sinking is 14, stranding is 4, mechanical failure is 3, others is 22, injured/death is 3 and the other outputs are 0. Furthermore, the improvement quantity of each DMUs is displayed in , the undesirable outputs of collision structural damage/ship sinking, Stranding and Fire could be reduced to 48, 55, 4 and1, the performance of navigation safety within Keelung port waters would be improved significantly. The navigation safety efficiency score of Hualien port are 1, in other words, the safety management level of their operations is relatively efficient. However, Kaohsiung, Taichung and Keelung port obtain the efficiency score with 0.597645, 0.659053, 0.708552 respectively. The assessment result demonstrates that among of three ports have great optimized and improved spaces on the navigation safety management.
Table 5. The slack value of all output variables among all DMUs
Table 6. The optimum value of all output variables among all DMUs
From the results in , during the period 2009 to 2019, we demonstrated undesirable-output DEA model on Taiwan international ports. The outcome of the undesirable-output DEA model was as follows:
The navigation safety ranks from the highest to the lowest is Hualien port, Keelung port, Taichung port, Kaohsiung port.
Four of the Taiwanese international ports, the lowest efficiency score of navigation safety is Kaohsiung port, and Hualien port is the highest.
For the purpose of increasing navigation safety of all marine casualties, collision and structural damage/ship sinking should be the first priority to be improved.
Based on the algorithm of the proposed undesirable-output DEA model, the results accord with reality.
This model can be adapted to the present problem to yield a variety of efficiency measures that treat undesirable outputs asymmetrically. This efficiency measure provides the basis for making multilateral productivity comparisons conditioned on different assumptions about undesirable outputs. Due to the restrictions of time and resources, this study only investigated 11 output variables and 1 input variable in the 4 DMUs of Keelung port, Kaohsiung port, Taichung port, and Hualien port. Future research may address such issues as oil leaks from ships, or the exhaust emissions of SOx, NOx, COx, VOCs from ships in the port (Tseng et al., Citation2017). Furthermore, the study limitations include the fact that MOTC’s casualty records do not include the injury level and loss value. Besides this, the input variables does not account for prime cost, the benefits, and manpower of the port. Although this would incorporate some discrepancies into the study findings for efficiency score, these assumptions would be accurate in most cases due to the discrepancies being small. Further work of this study may set up more input and output variables to assess based on this model. Each input variables of ports such as prime cost and manpower would be proposed and take into consideration. These issues are however beyond the scope of the current work, and left for future research.
6. Conclusions
This paper describes the application of an undesirable-output DEA model for the navigation safety. The paper demonstrates a useful research methodology, the construction of an undesirable-output DEA structure, and the empirical study of navigation safety. The important strengths of this study are that the undesirable-output DEA model could apply to evaluate the port navigation safety, and acquire the respective efficiency score and improvement of each port’s safety. As a result, each evaluated port can obtain ways to improve its safety performance. Comparing of undesirable-output DEA results with reality, the assumption of this algorithm evaluated and justified.
The strength of DEA is that it can provide efficiency scores of all DMUs by dealing with multiple inputs and multiple outputs, and demonstrate the improvements on each DMU as well. According to Wang and Lee (Citation2012b) the DEA-CCR Model evaluates the navigation safety of commercial harbours, nevertheless, DEA-CCR Model could not identify the differences between two equal efficiency DMUs. On the other hand, the above-mentioned model did not concern about the output variables are negative factor. For illustrating that the navigation safety assessment of the undesirable-output DEA is obviously better than the prototype of DEA, this study introduced the undesirable-output DEA model and viewed marine casualties as undesirable outputs to calculate.
According to the empirical research results, the navigation safety efficiency score rankings from high to low are Hualien port, Keelung port, Taichung port, Kaohsiung port. Furthermore, the result indicated that the port containing high collision and structural damage/ship sinking has low efficiency scores, leading to the high risk of navigation. Therefore, reducing the probability of collision and structural damage/ship sinking will greatly increase the safety of ports. Based on the undesirable-output DEA model, the assessment of the navigation safety can investigate to identify the key outputs that trigger port risks. For validating the effectiveness of the proposed model, the differences between reality and experiments that carried out by undesirable-output DEA model are consistent. This study aimed at furnishing a proposed model that can be generally applicable for port safety assessment. Incidentally, this paper can establish an assessment model of navigation safety with undesirable-output DEA. Further, it hopes to provide some suggestions for maritime authorities with similar safety statuses, to help improve the level of safety and maintain a safe navigation environment.
Finally yet importantly, this paper provides recommendations concerning follow-up research. Future research may utilize other optimal formula to eliminate the limitations about undesirable-output DEA model. It therefore hopes that future researchers concerning about this issue can provide even more efficient multilateral productivity comparisons conditioned on different assumptions about undesirable outputs to evaluate the port safety.
Disclosure statement
No potential conflict of interest was reported by the author(s).
Additional information
Funding
Notes on contributors
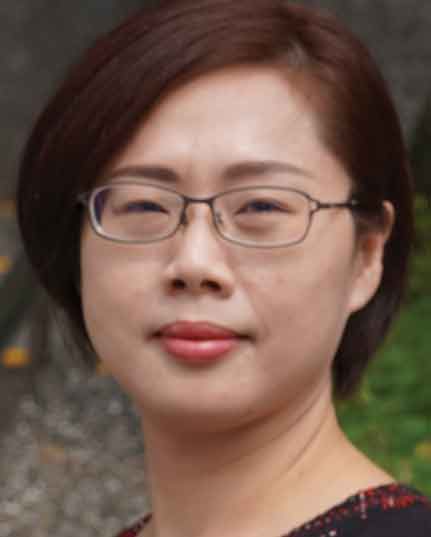
Hui-Chiao Wang
Hui-Chiao Wang is an Assistant Professor at Bachelor Program in Ocean Tourism Management of National Taiwan Ocean University. She had master’s degree in Merchant Marine Management and PhD in Business Administration at National Taiwan Ocean University. She worked for the Teaching and Learning Center of Academic Affair from 2013 to 2015. Afterwards she continued her scholarly career at Bachelor Program in Ocean Tourism Management. Her research interests include teaching practice research, navigation safety, maritime casualty analysis, and data envelopment analysis. She used to be a seafarer, so she devoted herself to the study the cause of maritime accidents in the hope of reducing the marine loss of life and property. In addition to analyzing maritime accidents, she used the hydraulic model for the analysis of evacuations for passenger Ship. Hui-Chiao Wang has published in Journal of Ship Production and Design and Journal of Marine Engineering and Technology.
Notes
1. According to MSC-MEPC.3/Circ.1.
References
- Afenyo, M., Khan, F., Veitch, B., & Yang, M. (2018). Arctic shipping accident scenario analysis using Bayesian network approach. Ocean Engineering, 133(15), 224–20. https://doi.org/10.1016/j.oceaneng.2017.02.002
- Akten, N. (2004). Analysis of shipping casualties in the Bosphorus. The Journal of Navigation, 57(3), 345–356. https://doi.org/10.1017/S0373463304002826
- Akyuz, E. (2015). Quantification of human error probability towards the gas inerting process onboard oil tankers. Safety Science, 80, 77–86. https://doi.org/10.1016/j.ssci.2015.07.018
- Akyuz, E. (2016). Quantitative human error assessment during abandon ship procedures in maritime transportation. Ocean Engineering, 120(1), 21–29. https://doi.org/10.1016/j.oceaneng.2016.05.017
- Akyuz, E., & Celik, M. (2014). Application of CREAM human reliability model to cargo loading process of LPG tankers. Safety Science, 34, 39–48. https://doi.org/10.1016/j.jlp.2015.01.019
- Akyuz, E., & Celik, M. (2015). A methodological extension to human reliability analysis for cargo tank cleaning operation on board chemical tanker ships. Safety Science, 75, 146–155. https://doi.org/10.1016/j.ssci.2015.02.008
- Balmat, J. F., Lafont, F., Maifret, R., & Pessel, N. (2009). MAritime RISk Assessment (MARISA), a fuzzy approach to define an individual ship risk factor. Ocean Engineering, 36(15–16), 1278–1286. https://doi.org/10.1016/j.oceaneng.2009.07.003
- Banda, O. A. V., Goerlandt, F., Kuzmin, V., Kujala, P., & Montewka, J. (2016). Risk management model of winter navigation operations. Marine Pollution Bulletin, 108(1–2), 242–262. https://doi.org/10.1016/j.marpolbul.2016.03.071
- Banker, R. D., Charnes, A., & Cooper, W. W. (1984). Some models for estimating technical and scale inefficiencies in data envelopment analysis. Management Science, 30(9), 1078–1092. https://doi.org/10.1287/mnsc.30.9.1078
- Bolat, F. (2016). Analysis of ship accidents in Turkey from 2005 to 2015. International Journal of Engineering Research and Technology, 5(12), 478–481.
- Chai, T., Weng, J., & De-qi, X. (2017). Development of a quantitative risk assessment model for ship collisions in fairways. Safety Science, 91, 71–83. https://doi.org/10.1016/j.ssci.2016.07.018
- Charnes, A., Cooper, W. W., & Rhodes, E. (1978). Measuring the efficiency of decision making units. European Journal of Operational Research, 2(6), 429–444. https://doi.org/10.1016/0377-2217(78)90138-8
- Chen, C., Zhu, J., Yu, J. Y., & Noori, H. (2012). A new methodology for evaluating sustainable product design performance with two-stage network data envelopment analysis. European Journal of Operational Research, 221(2), 348–359. https://doi.org/10.1016/j.ejor.2012.03.043
- Chen, J., Bian, W., Wan, Z., Yang, Z., Zheng, H., & Wang, P. (2019). Identifying factors influencing total-loss marine accidents in the world: Analysis and evaluation based on ship types and sea regions. Ocean Engineering, 191, 106495. https://doi.org/10.1016/j.oceaneng.2019.106495
- Christiansen, M., Fagerholt, K., Nygreen, B., & Ronen, D. (2007). Maritime transportation. In Handbooks in operations research and management science Vol. 14. (pp. 189–284). https://doi.org/10.1016/S0927-0507(06)14004-9.
- Cooper, W. W., Seiford, L. M., & Tone, K. (2001). Data envelopment analysis: A comprehensive text with models, applications, references and DEA-solver software. Journal of the Operational Research Society, 52(12), 1408–1409. https://doi.org/10.1057/palgrave.jors.2601257
- Corporate, A. G. (2019). Specialty: Safety & shipping review 2019. An annual review of trends and developments in shipping losses and safety.
- ElSayed, T., Leheta, H., & Gadar, H. (2011). Assessment of safety performance indicators: A case study for the Bouri field. Proceedings of the Institution of Mechanical Engineers, Part M: Journal of Engineering for the Maritime Environment, 225(1), 60–67. https://doi.org/10.1177/1475090210396025
- EMSA. (2018). Annual overview of marine casualties and incidents.
- Faghih-Roohi, S., Xie, M., & Ng, K. M. (2014). Accident risk assessment in marine transportation via Markov modelling and Markov chain Monte Carlo simulation. Ocean Engineering, 91, 363–370. https://doi.org/10.1016/j.oceaneng.2014.09.029
- Färe, R., & Grosskopf, S. (2004). Modelling undesirable factors in efficiency evaluation: Comments. European Journal of Operational Research, 157(1), 242–245. https://doi.org/10.1016/S0377-2217(03)00191-7
- Färe, R., Grosskopf, S., Lovell, C. A. K., & Pasurka, C. (1989). Multilateral productivity comparisons when some outputs are undesirable: A nonparametric approach. The Review of Economics and Statistics, 71(1), 90–98. https://doi.org/10.2307/1928055
- Fera, M., & Macchiaroli, R. (2010). Appraisal of a new risk assessment model for SME. Safety Science, 48(10), 1361–1368. https://doi.org/10.1016/j.ssci.2010.05.009
- Gan, G. Y., Lee, H. S., Chung, C. C., & Chen, S. L. (2017). Performance evaluation of the security management of changjiang maritime safety administrations: Application with undesirable outputs in data envelopment analysis. Journal of Marine Science and Technology, 25(2), 213–219. https://doi.org/10.6119/JMST-016-1207-1
- Goerlandt, F., Goite, H., Banda, O. A. V., Höglund, A., Ahonen-Rainio, P., & Lensu, M. (2017). An analysis of wintertime navigational accidents in the Northern Baltic Sea. Safety Science, 92, 66–84. https://doi.org/10.1016/j.ssci.2016.09.011
- Guo, D., & Wu, J. (2013). A complete ranking of DMUs with undesirable outputs using restrictions in DEA models. Mathematical and Computer Modelling, 58(5–6), 1102–1109. https://doi.org/10.1016/j.mcm.2011.12.044
- Hu, S., Fang, Q., Xia, H., & Xi, Y. (2007). Formal safety assessment based on relative risk model in ship navigation reliability engineering & system safety. Reliability Engineering and System Safety, 92(3), 369–377. https://doi.org/10.1016/j.ress.2006.04.011
- Hu, S., & Zhang, J. (2012). Risk assessment of marine traffic safety at coastal water area. Procedia Engineering, 45, 31–37. https://doi.org/10.1016/j.proeng.2012.08.116
- Huang, T., Chen, Z., Wang, S., & Jiang, D. (2021). Efficiency evaluation of key ports along the 21st-century maritime silk road based on the DEA–SCOR model. Maritime Policy & Management, 48(3), 1–13. https://doi.org/10.1080/03088839.2020.1773558
- International Maritime Organization, Introduction to IMO, Retrieved 14/5, 2004.
- Jiang, M., Lu, J., Yang, Z., & Li, J. (2020). Risk analysis of maritime accidents along the main route of the maritime silk road: A Bayesian network approach. Maritime Policy & Management, 47(6), 815–832. https://doi.org/10.1080/03088839.2020.1730010
- Liu, C. P., Liang, G. S., Su, Y., & Chu, C. W. (2006). Navigation safety analysis in Taiwanese ports. The Journal of Navigation, 59(2), 201–211. https://doi.org/10.1017/S0373463306003687
- Liu, J., Ding, F. Y., & Lall, V. (2000). Using data envelopment analysis to compare suppliers for supplier selection and performance improvement. Supply Chain Management: An International Journal, 5(3), 143–150. https://doi.org/10.1108/13598540010338893
- Liu, W. B., Meng, W., Li, X. X., & Zhang, D. Q. (2010). DEA models with undesirable inputs and outputs. Annals of Operations Research, 173(1), 177–194. https://doi.org/10.1007/s10479-009-0587-3
- Liu, Z., Wu, Z., & Zheng, Z. (2019). A cooperative game approach for assessing the collision risk in multi-vessel encountering. Ocean Engineering, 187, 106175. https://doi.org/10.1016/j.oceaneng.2019.106175
- Nazarnia, H., Nazarnia, M., Sarmasti, H., & Wills, W. O. (2020). A systematic review of civil and environmental infrastructures for coastal adaptation to sea level rise. Civil Engineering Journal, 6(7), 1375–1399. https://doi.org/10.28991/cej-2020-03091555
- Qasim, R. M., & Hasan, A. Q. (2020). Investigating the behavior of offshore platform to ship impact. Civil Engineering Journal, 6(3), 495–511. https://doi.org/10.28991/cej-2020-03091486
- Randić, M., Matika, D., & Možnik, D. (2015). SWOT analysis of deficiencies on ship components identified by port state control inspections with the aim to improve the safety of maritime navigation. Brodogradnja: Teorija I Praksa Brodogradnje I Pomorske Tehnike, 66 (3), 61–72. 0000-0001-8202-4097.
- Sang, L. Z., Wall, A., Mao, Z., Yan, X. P., & Wang, J. (2015). A novel method for restoring the trajectory of the inland waterway ship by using AIS data. Ocean Engineering, 110, 83–194. https://doi.org/10.1016/j.oceaneng.2015.10.021
- Sathye, M. (2003). Efficiency of banks in a developing economy: The case of India. European Journal of Operational Research, 148(63), 662–671. https://doi.org/10.1016/S0377-2217(02)00471-X
- Saviolakis, P., & Pazarzis, M. (2021). The effect of Covid-19 pandemic on the maritime industry and the role of the ship registries. Emerging Science Journal, 5, 77–85. https://doi.org/10.28991/esj-2021-SPER-06
- Stoop, J. A. (2003). Maritime accident investigation methodologies. Injury Control and Safety Promotion, 10(4), 237–242. https://doi.org/10.1076/icsp.10.4.237.16776
- Szlapczynski, R. (2015). Evolutionary planning of safe ship tracks in restricted visibility. The Journal of Navigation, 68(1), 39–51. https://doi.org/10.1017/S0373463314000587
- Tian, W., Ma, Q., Zhang, J., Meng, B., Gan, Z., Wan, H., & He, Y. (2020). Ship collision risk assessment model for Qinzhou port based on event sequence diagram. Brodogradnja: Teorija I Praksa Brodogradnje I Pomorske Tehnike, 71(2), 1–14. https://doi.org/10.21278/brod71201
- Tseng, W. J., Ding, J. F., & Chang, K. H. (2017). Evaluating key environmental risk factors for pollution at international ports in Taiwan. Brodogradnja: Teorija I Praksa Brodogradnje I Pomorske Tehnike, 68(1), 1–15. https://doi.org/10.21278/brod68101
- Twrdy, E., Petrovič, M., & Batista, M. (2013). Limitation and restrictions on the admission of postpanamax container ships in the port of Koper. Brodogradnja: Teorija I Praksa Brodogradnje I Pomorske Tehnike, 64 (4), 456–471. 0000-0002-7937-3162.
- UNCTAD (United Nations Conference on Trade and Development). (2016). Review of maritime transport 2016.
- UNCTAD (United Nations Conference on Trade and Development). (2018). Review of maritime transport 2018.
- Ung, S. T. (2019). Evaluation of human error contribution to oil tanker collision using fault tree analysis and modified fuzzy Bayesian network based CREAM. Ocean Engineering, 179(1), 159–172. https://doi.org/10.1016/j.oceaneng.2019.03.031
- United Nations. (1982). United Nations convention on the law of the sea. International Legal Materials Treaties and Agreements, 21(6), 1261–1354. https://doi.org/10.1017/S0020782900057363
- Urbański, J., Morgaś, W., & Kopacz, Z. (2008). The safety and security system of maritime navigation. The Journal of Navigation, 61(3), 529–553. https://doi.org/10.1017/S0373463308004712
- Wang, H. C., & Lee, H. S. (2010). Application of grey relational analysis to evaluate port safety in Keelung harbor. Journal of Ship Production and Design, 26(3), 206–210. https://doi.org/10.5957/jspd.2010.26.3.206
- Wang, H. C., & Lee, H. S. (2012a). The impact of navigation safety in Kaohsiung harbor. Journal of Marine Engineering & Technology, 11(1), 45–50. https://doi.org/10.1080/20464177.2012.11020260
- Wang, H. C., & Lee, H. S. (2012b). Evaluating navigation safety for harbours in Taiwan: An empirical study. Journal of Marine Engineering & Technology, 11(3), 31–37. https://doi.org/10.1080/20464177.2012.11020270
- Wang, J., & Foinikis, P. (2001). Formal safety assessment of containerships. Marine Policy, 25(2), 143–157. https://doi.org/10.1016/S0308-597X(01)00005-7
- Wang, L., Wang, J., Shi, M., Fu, S., & Zhu, M. (2020). Critical risk factors in ship fire accidents. Maritime Policy & Management, 1–19. https://doi.org/10.1080/03088839.2020.1821110
- Xie, L., Xue, S., Zhang, J., Zhang, M., Tian, W., & Haugen, S. (2019). A path planning approach based on multi-direction A* algorithm for ships navigating within wind farm waters. Ocean Engineering, 184(15), 311–322. https://doi.org/10.1016/j.oceaneng.2019.04.055
- Yang, Z., Bonsall, S., Wall, A., Wang, J., & Usman, M. (2013). A modified CREAM to human reliability quantification in marine engineering. Safety Science, 58(15), 293–303. https://doi.org/10.1016/j.oceaneng.2012.11.003
- You, S., & Yan, H. (2011). A new approach in modelling undesirable output in DEA model. Journal of the Operational Research Society, 62(12), 2146–2156. https://doi.org/10.1057/jors.2011.1
- Zhang, J., Teixeira, Â. P., Guedes Soares, C., & Yan, X. (2018a). Quantitative assessment of collision risk influence factors in the Tianjin port. Safety Science, 110, 363–371. https://doi.org/10.1016/j.ssci.2018.05.002
- Zhang, J., Teixeira, Â. P., Guedes Soares, C., Yan, X., & Liu, K. (2016b). Maritime transportation risk assessment of tianjin port with Bayesian belief networks. Risk Analysis, 36(6), 1171–1187. https://doi.org/10.1111/risa.12519
- Zhang, W., Goerlandt, F., Kujala, P., & Qi, Y. (2018b). A coupled kinematics model for icebreaker escort operations in ice-covered waters. Ocean Engineering, 167(1), 317–333. https://doi.org/10.1016/j.oceaneng.2018.08.035
- Zhang, W., Goerlandt, F., Kujala, P., & Wang, Y. (2016a). An advanced method for detecting possible near miss ship collisions from AIS data. Ocean Engineering, 124, 141–156. https://doi.org/10.1016/j.oceaneng.2016.07.059
- Zhang, W., Goerlandt, F., Montewka, J., & Kujala, P. (2015). A method for detecting possible near miss ship collisions from AIS data. Ocean Engineering, 107, 60–69. https://doi.org/10.1016/j.oceaneng.2015.07.046