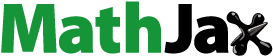
Abstract
The aim of the research was to identify and evaluate the main determinants of small-scale biogas technology adoption and its impact on crop yields. The case study is based on cross-sectional data gathered from 335 rural households in Ethiopia’s East Gojjam Zone. This study made use of both primary and secondary data. A questionnaire-based survey was used to collect primary data from 197 biogas adopter (treated) and 138 non-adopter (control) households. Propensity score matching (PSM) methods were used to estimate the determinants and impact of biogas technology adoption on cereal crop yields. According to probit model estimates, the main determinants were cattle head, follow-up and support, extension contact, training access, distance to water sources, and distance to the market. Ethiopian agricultural and rural development policies should consider the impact of household biogas technology adoption behavior when developing policy actions under the Agricultural Transformation Plan.
Public Interest Statement
Biogas technology provides many irreversible benefits to society, such as bio-slurry, cooking, and lighting as eco-friendly resources. Bio-slurry from biogas is utilized as one of the best organic fertilizers, greatly improving soil fertility and, as a result, crop productivity and income of small-holder farmers. Despite its versatile resources, agricultural and rural development agencies have paid little attention to the technology’s diffusion. Until 2020, only 482 rural households out of 67,966 in the study areas adopted and benefited from biogas technology, demonstrating that the technology has not been broadly adopted by farmers. Consequently, the actual output of cereal crops is lower than the potential production. Therefore, this study explores the factors of small-scale biogas technology adoption and its impact on crop yields. To realize the potential benefits of the technology, all development agents should collaborate to consider biogas technology as one of the strategies of agricultural and rural development policies.
1. Introduction
A key issue in economic development theory is a historical examination of how individuals can break free from the cycle of poverty. Poverty is primarily a rural phenomenon around the world. That is, approximately 80% of the world’s poor live in rural areas; 64% work in agriculture (Ermiyas et al., Citation2019); and one-tenth of the world’s population lives on less than 1.9 dollars per day (Roser & Ortiz-Ospina, Citation2013). Similarly, 79% of Ethiopia’s total population lives in rural areas, and agriculture is the primary source of income, accounting for the greatest proportion of employment opportunities and a source of foreign earnings (NBE, Citation2021). Ethiopia has a rapidly growing population and a long history of food insecurity. A series of production failures, as well as an imbalance between agricultural productivity and population growth, have made a significant contribution to food insecurity (Kaluski et al., Citation2001).
As a result, increasing agricultural production is the best option for alleviating poverty and achieving food security by increasing the amount of food available for consumption (Wolde, Citation2021). Extensification (i.e., increasing crop yields) and intensification (i.e., expanding acreage) are two methods for increasing agricultural output (i.e., by using more inputs and technologies per unit of land). However, because land is scarce in Ethiopia, where severe population pressure is a significant bottleneck, extensification is not a viable option (Menmeru, Citation2014). Intensification, which includes investments in modern inputs and technologies, is a better option for increasing agricultural output and decreasing food insecurity. In the 1970s, several Asian countries successfully implemented this option, which was dubbed the “green revolution” (Mulugeta et al., Citation2012).
Since 1991, the Ethiopian government has used the green revolution to increase agricultural production (Rohne Till, Citation2021; Wolde, Citation2021). This method emphasized technology packages that included credit, fertilizers (both organic and synthetic), improved seeds, and improved management procedures to increase cereal yields (Endale, Citation2010). To maintain this high rate of agricultural growth, new yield-increasing technologies must be successfully implemented (Dadi et al., Citation2004; Dorosh & Rashid, Citation2012). To that end, chemical fertilizers (DAP and UREA), one of the major agricultural inputs, are imported into Ethiopia in large quantities every year (NBE, Citation2020), and have been used for major crop production in Ethiopia for several decades (Biramo et al., Citation2019).
Although fertilizer use is the primary strategy for addressing soil depletion, the long-term and continuous application of chemical fertilizers has a significant and irreversible impact on agricultural production by degrading soil fertility (Gelmesa et al., Citation2012; Rahman & Tetteh, Citation2014). The resulting issues, such as soil organic matter depletion, deteriorating soil structure, nutrient imbalances, and loss of soil biodiversity, may cause agricultural production to decline (Bansal et al., Citation2020; Chen et al., Citation2020; Fan et al., Citation2020; Haile & Ayalew, Citation2018; Kamran et al., Citation2021). Such degradation has largely dominated the major crop production area, which is primarily cultivated using an intensive annual crop rotation of wheat and maize (Wang et al., Citation2019; Zhu et al., Citation2021). Low organic matter content and poor soil fertility may severely limit crop yields, exposing many Ethiopian farmers to food insecurity and poverty and necessitating food aid (Zheng et al. Citation2021; Biramo et al., Citation2019).
Later, in order to find the best alternative to chemical fertilizer, the Ethiopian government launched the National Biogas Program of Ethiopia (NBPE) in 2007 with technical assistance from the Netherlands Development Organization (SNV) for the development and dissemination of biogas technology for rural households (Eshete & Stoop, Citation2007; Shallo & Sime, Citation2019). Biogas is created in the absence of oxygen through anaerobic digestion of animal waste and crop residue, and it is primarily composed of methane and carbon dioxide (Balgah et al, Citation2018). Biogas is made from cow dung in Ethiopia, and biogas slurry is a byproduct that is used as an organic fertilizer. Despite the fact that bio-slurry is a waste product, it appears that the efforts have paid off.
By adding organic matter to the soil, bio-slurry improves the physical, chemical, and biological quality of the soil by enhancing its structure, water holding capacity, and microbial activity (Goberna et al., Citation2011; Guta, Citation2014). The bio-slurry from biogas is the most important soil conditioner because it contains humic acid, which increases the soil’s nutrient supply and hygroscopicity, stimulating plant growth (Warnars & Oppenoorth, Citation2014; Yu et al., Citation2010). Bio-slurry, in particular, contains all of the primary nutrients for the development of soil organisms as well as an organic fungicide that fights plant diseases, reduces soil erosion by improving soil water retention capacity, and produces probiotic microbial-derived bio-activator to stimulate microbial activity, promote plant growth, and reduce soil-borne diseases. As a result, biogas slurry improves soil fertility for crop production and causes a spring increase in crop yields on adopters’ fields (Shaheb et al., Citation2015). It also improves yield quality and quantity, food security, and farmer profitability (Clemens et al., Citation2018; Tah & Ngwa, Citation2015).
At the pilot level, biogas experts agreed that biogas slurry increased productivity. A variety of factors, including improved seed, soil type, and proper technological imitation, among others, influence productivity (Wolde, Citation2021). Farmers may not receive adequate development assistance or ongoing follow-up from development agents. As a result, once the technology is available to farmers, an analysis at the farm level should be conducted to determine its impact on productivity. The farm level impact study will provide useful information for determining whether or not farmers can effectively use the technology. So far, evidence on the effect of bio slurry application on cereal crop yields in Ethiopia, particularly in the East Gojjam Zone, is lacking. As a result, the goal of this research is to evaluate the impact of biogas technology adoption on crop yield and other factors influencing adoption decisions in the East Gojjam Zone.
2. Research methodology
2.1. Study population
The research was carried out in three randomly selected woredas in the Amhara region’s East Gojjam Zone. Ethiopia’s National Domestic Biogas Programme has recently introduced domestic biogas technology in Gozamin, Awabel, and Enebse Sar Midir. Most importantly, in 2013, biogas technology was introduced in the East Gojjam Zone (EGZ, Citation2021). The study areas are distributed spatially across three woredas in order to collect heterogeneous data on socioeconomic attributes, infrastructure level, agricultural production level, and the status of households’ biogas technology adoption potential. The research locations are additionally distinguished by a range of meteorological and topological domains, such as altitude variations, temperature variations, and rainfall variations, as well as the presence of biomass energy sources. The study districts have a total of 67,966 rural households whose primary source of livelihood is agriculture (EGZ, Citation2021). The farming method, which was seen to be a combination of raising animals and producing crops at all research sites, uses chemical fertilizer continuously, which reduces soil fertility and, as a result, crop yield.
2.2. Sample design, procedure, and data collection
The study was cross-sectional in nature. The two-stage sampling technique was used to choose the study area. Three woredas were chosen at random in the first stage: Gozamin, Awabel, and Enebsie Sar Midir. With the assistance of the water and energy officers of the study woredas, sample homes from the three woredas that use the byproducts of domestic biogas technology (bio-slurry) for fuel and fertilizer were purposefully chosen for the second stage. As a sampling frame, the sample for user households with bio-digesters that were finished and operational at least 10 months before the survey period was employed.
An effort was made to incorporate this time lag so that households would have enough time to gain the knowledge necessary to operate a digester and profit from owning the technology (bio-slurry) for boosting crop output. In addition, because of the frequent information sharing between neighbors in rural areas, a sample of non-user households that are close to user households was taken (Ghimire, Citation2013). According to the East Gojjam Zone water and energy office (2020), there were 482 biogas adopter homes in the three woredas. Gozamin Woreda accounted for 305 of the biogas adopters, the majority. Additionally, there were 122 and 55 biogas adopters in the woredas of Awabel and Enebsie Sar Midir, respectively.
To calculate the sample size for the families using biogas, the general formula created by Tara Yamane (1967) was used. The study included a + 5% margin of error and a 95% confidence interval. Thus, the sample size was calculated using the following formula:
Where e (0.05) denotes the desired level of precision, i.e. the margin of error, and n denotes the required sample size, N is the total household population under investigation that is involved in agriculture.
Thus, ,
,
n = 218.59 ˷ 219
Based on each woreda’s proportional proportion of biogas consumers to the entire sampling frame, as stated in Table , the sample size was distributed throughout the woreda.
Table 1. Proportional sample distribution
To eliminate biases that may result from a difference in sample size, the researcher intended to employ sample sizes of 219 from adopter families and another 219 from non-adopter households from Table . Despite this, a total of 335 houses, including 197 biogas user (treated) and 138 non-user (control) observations, were gathered by taking into account only biogas user households for the adopter side and cleaning the data by removing incomplete replies. The data for the three woredas was gathered between February and March of 2021.
Structured questioners were used to randomly choose the selected household heads and obtain the necessary data from them. In-depth qualitative data was also gathered by conducting focus groups, key informant interviews, and field observations in addition to validating the household survey. A pre-tested questioner was used to collect information on a variety of socioeconomic factors of households and the impacts of bio-slurry fertilization on crop output. The questioner also included a part on the justifications for using biogas or not, the source of the money for the digester, and follow-up and technical assistance from the water and energy office’s technological experts. Furthermore, focus groups and key informant interviews were used to gather qualitative information on the potential and challenges facing the biogas industry.
The managers of biogas programs, database officers, planning, monitoring, and evaluation officers, energy experts, and renewable energy consultants at various levels are examples of key informants who are competent and open-minded. Secondary data was gathered from peer-reviewed journals and unpublished official reports and records, particularly the reports of the East Gojjam Zone Biogas Program Coordination with SNV and the National Biogas Programme of Ethiopia.
2.3. Data analysis
The foundation of the analysis was comprised of descriptive statistics and propensity score matching techniques. The PSMATCH2 technique and STATA 14 software were used to analyze the collected data. The T-test was used to determine whether there was a statistically significant difference between families using and not using biogas. Utilizing treatment and control observations together with their determinant variables, econometric analysis was used to assess the determinants of small-scale biogas technology adoption and the impact of this technology on agricultural yield. Propensity Score Matching (PSM) was used to analyze the effect of biogas technology on agricultural productivity in order to minimize potential sample bias because participation in the residential biogas program is not random (Liu et al., Citation2020; Becker & Ichino, Citation2002; Wu et al., Citation2010; Caliendo & Kopeinig, Citation2008).
Using the propensity score matching approach, households who utilize bio-slurry from biogas were essentially compared to those that have not yet taken part in order to identify a bias problem. In this situation, biogas users who utilize bioslurry as a treatment saw a change in crop yield. The impact of residential biogas technology adoption on the outcome variables was examined in two steps of the study. In the beginning, selection EquationEq. 1(1)
(1) , which employs a probit model, was used to estimate the propensity score, also known as the probability of building a domestic biogas plant, for the treated and control observations, in accordance with; Table ). The Average Treatment Effect on Treated (ATT) over the result of Equation Four was calculated in the second phase. After matching, the ATT suggests the typical yield differential between houses using biogas technology and those without. This was accomplished by applying nearest neighbor, radius, kernel, and stratification matching algorithm methods to match biogas user and non-user homes in accordance with the propensity score.
Table 2. Probit estimates of the propensity to biogas technology adoption (Dependent variable: adoption [1/0])
In the study, the treatment D is a binary variable that determines if the household uses bio-slurry or not, D = 1 for users and D = 0 otherwise.
Xi denotes the pre-treatment characteristics (characteristics of households that might affect the adoption decision of biogas technology).
According to EquationEq. 1(1)
(1) , the likelihood that families will choose to use biogas technology depends on their pre-treatment characteristics.
Y stands for the treatment effects in EquationEq. 2(2)
(2) . (impact of biogas technology adoption on crop yield). After matching, the outcome Y among users (Y1) and non-users (Y0) was compared to see what effect the outcome variables (crop yield) would have on the households not using bio-slurry. The treatment effect for a single household I can be written as:
Caliendo and Kopeinig (Citation2008) outline the two most commonly estimated parameters in the literature. The first is the average population treatment effect (ATE), which is calculated by comparing the average crop yield use among bio-slurry user and non-user households without matching, as shown in EquationEq.3(3)
(3) .
The above equation depicts the expected effect of technology adoption on the outcome variable (crop yield) if the population’s households were assigned at random (Caliendo & Kopeinig, Citation2008). However, it is possible that it is irrelevant to my analysis because it includes the effect of the household for which the program was never intended. As shown in EquationEq.4(4)
(4) , the most prominent evaluation of the pure effect of the treatment observation is the average treatment effect on the treated (ATT).
The ATT is a hypothetical situation that compares crop yield between bio-slurry user and non-user households after matching based on their propensity scores to adopt biogas energy technology.
On the basis of propensity scores, different matching criteria can be used to assign adopters and non-adopters. According to Khandker et al. (Citation2009) and Desai et al. (Citation2017), the different matching techniques will affect the estimation results. As a result, the ATT results are presented using four matching techniques: Nearest Neighbor Matching (NNM), Radius, Kernel, and stratification matching methods. Based on the nearest propensity score, the NNM method pairs each treated individual with an untreated individual. Typically, the matching procedure was used by allowing for replacement, implying that the same untreated individual could be used as a match for multiple treated individuals. However, the use of NNM revealed that the difference in propensity score between the treated and its nearest untreated may still be significant. The Radius matching approach, which requires a fixed range distance of propensity score between treated and untreated individual matches, can be used to avoid the situation that causes poor matching. Replacement is permitted only between propensity scores within a fixed range of distance. However, because of the high number of dropped observations due to outranged common support, this procedure may increase the risk of sampling bias. To avoid dropped observations, a kernel matching procedure can be used, which involves weighting the average of all untreated individuals to be matched by each treated individual. This method, however, has a high bias risk because only a small subset will meet the criteria. Furthermore, the stratification method produced greater imbalances in covariate distributions between statin-exposed and unexposed matches, resulting in a higher bias and lower precision. Because each matching procedure has limitations, this study used all four matching criteria to demonstrate estimation consistency.
As a result, the complete lists of explanatory variables included in this study are shown in Table below.
Table 3. Definition of variables and their measurement
3. Results and discussion
3.1. Descriptive analysis
Table summarizes the socioeconomic and demographic characteristics of the sampled households. The survey included 335 sample households, 197 of which are bio-slurry beneficiaries of biogas technology and 138 of which are not. In terms of percentages, bio-slurry is used by 59% of households, while 41% of them do not.
Table 4. Mean value of basic socio-economic and demographic variables of respondents (Standard errors in parentheses)
As shown in Table , there is a group mean difference in a number of characteristics between biogas adopter and non-adopter households. The average age, level of income, land size, and cattle head of the treated cases (biogas users), for example, were found to be significantly higher than those of the comparison observations. More specifically, adopter households are significantly older than non-adopter households. This gives the impression that older households are more likely than younger households to use better bio-slurry due to the possibility of greater wealth accumulation to adopt the technology. Similarly, biogas users had higher annual income and land size than non-user households, indicating that higher income may be associated with the use of better organic fertilizer from bio-slurry. Other farm characteristics were higher among biogas adopter households, including awareness, adequate water supply, follow-up and support from technology transfer experts, access to training, and frequency of extension contact. Furthermore, the distance to water and the distance to the market create a significant difference between adopter and non-adopter households.
Table also includes average information about the respondents in this study. For example, the average age of respondents is 49 years old, and their average income is 1,674.10 USD, while the average income of adopter households is not more than 2,126.60 USD. Each household has an average of 1.8 hectares of land and 5.42 TLU in their herd, which exceeds the requirements for biogas technology adoption (SNV, Citation2017). The average walking distance from home to water is 8.4 minutes, and the average distance to the nearest market with biogas technology is 12.4 minutes. Furthermore, nearly three-quarters (74%) of respondents are aware of the technology, and nearly half (54%) have an adequate water supply. However, only 46% of respondents receive follow-up and support from biogas technology dissemination stakeholders, and 34% have access to training, indicating a very low exposure to new information. Furthermore, the average frequency of agricultural extension contact per year is 2.9 times, which is far below the requirements for small-holder farmers.
3.2. Impacts of biogas technology adoption on the outcome variable
Prior to implementing the propensity score matching model, the study used a two-test sample paired t-test to determine the statistical difference between treatment and control groups, as shown in Table below. The null hypothesis assumes that the mean crop yield of the treatment and control groups is the same. According to Table , the null hypothesis of a difference in mean crop yield between biogas users and non-user households is rejected. As a result, the mean crop yield of non-user households is 33.9 quintals/year lower than that of biogas-user households, and the estimated difference was statistically significant at the 1% level of significance. This is due to the increased crop yield produced by using bio-slurry as an organic fertilizer, which contributed to the difference between the treatment and control groups.
Table 5. The t-test of crop yield: average crop yield by technology adoption
According to the findings above, technology adoption improves agricultural productivity and thus crop yield. Households that use biogas produce more agricultural products than non-users. The change is statistically significant, and it is in a positive direction. The findings are supported by Kelebe’s (Citation2018) previous studies, which discovered that bio-slurry user households can increase crop yield.
However, because technology adoption is endogenous, a simple comparison of the outcome variable does not provide a causal interpretation. That is, the difference in crop yield may not be due to a lack of biogas adoption. Other factors, such as differences in household characteristics and endowments, may be to blame. Thus, propensity score matching methods were used in the study to avoid such a problem and to determine the pure impact of technology adoption.
Various econometric assumptions were tested using appropriate techniques prior to the estimation of the Probit regression and propensity scores. Post estimation variance inflation factors (VIF) were used to test the multicollinearity of all explanatory variables. Based on the VIF (Xi) values, the value of VIF is far less than 10. As a result, all significant explanatory variables were included in the model (Appendix Table ).
The model specification was validated using linktest, a non-significant test of hatsq is 0.934. This means that linktest failed to reject the assumption that the model is correctly specified. As a result, it is confirmed that this study does not contain a specification error (Appendix).
3.3. Estimating propensity score
Table shows the estimation of propensity scores using the Probit model (EquationEq.1)(1)
(1) to predict the determinants of biogas technology adoption. As previously stated, the dependent variable in this model is binary, indicating whether the household uses the end products of bio-slurry, with a value of 1 indicating use and 0 indicating non-use.
Table displays the estimated propensity score coefficients, which are required for the estimation of the outcome variables. The diagnostic test confirms the model’s overall fitness, with Prob > chi2 = 0.000. The LR χ2 test was based on the assumption that at least one of the regression predictor coefficients was not equal to zero. The estimated LR χ2 test value was 409.6, indicating that the predictor coefficients differed from zero. The model’s goodness-of-fit measures revealed that the independent variables were related to the log odds of adoption at the same time. Furthermore, for the entire observed data set, the chosen independent variables correctly predicted households’ biogas adoption conditions.
Six of the 15 explanatory variables identified had a significant impact on the household’s decision to use biogas technology. Cattle heads, in particular, were significant variables influencing biogas technology adoption (P < 0.05). A certain number of livestock of a certain size is required to generate adequate manure, energy, and bio-slurry (Shallo et al., Citation2020; SNV, Citation2017). Thus, a large number of cattle, expressed in cow equivalents, has a positive impact on the adoption of biogas technology. The distance from the residence to water sources and the nearest market also had a significant (p < 0.01) negative impact on technology adoption. The likelihood of households adopting biogas technology decreased by 0.179 when the distance to water sources from the residence increased by 1 minute. This implies that the distance to a water source influences the adoption of biogas technology. A study conducted by Shallo et al. backed up the findings (CitationCentral Statistics Agency (CSA), 2020/2021). Water is required for the production of bio-slurry and the use of biogas technology (J. Mwirigi et al., Citation2014).
Furthermore, the distance between a home and the nearest market is negatively associated with the adoption of biogas technology (Kelebe, Citation2018; Mengistu et al., Citation2016). The likelihood of households adopting biogas technology decreased by 0.288 when the distance to the market from the residence for purchasing spare parts increased by 1 minute. That is, the further away the household homestead is from modern energy technology providers, the less likely the household is to adopt biogas technology because it reduces the likelihood of obtaining spare parts for the technology. The most inconvenient condition that appears to have a negative impact on the adoption and functionality of bio-digesters is the scarcity of biogas key spare parts near farm households (Shallo & Sime, Citation2019).
Institutional follow-up for technical support influenced the functional status of bio-digesters significantly (P < 0.01). As a result, the dissemination of biogas technology supported by trained technicians who can provide standard construction, support for arranging spare parts, and proper maintenance services of installed bio digesters increases the functional status of bio-digesters (Ghimire, Citation2013; Nasery & Rao, Citation2011; Shallo & Sime, Citation2019). However, in Africa, bio-digester malfunction is exacerbated by a poor dissemination strategy, a lack of project monitoring and follow-up by promoters, a lack of technical support and spare parts, and a lack of ownership responsibility by adopters (Bensah & Brew-Hammond, Citation2010; Berhe et al., Citation2017). As a result, increasing a household’s likelihood of receiving adequate follow-up and technical support was discovered to improve the functional status of biogas technology.
Access to training was discovered to have a positive and significant (P < 0.05) influence on technology adoption. This means that as households receive technical training on how to use biogas technology, the likelihood of their maintaining operational bio-digesters installed bio-digesters increases (SNV, Citation2017; Agbarevo & Benjamin, Citation2013). Masons and energy supervisors lack the necessary skills to provide adequate maintenance service. Despite user households’ limited understanding of bio-digester operation principles, digesters stop working due to minor issues such as water pipe and lamp damage, and substrate drying out in digesters. Such technical ability of adopter households may improve the technology’s reputation among potential adopting rural communities and the working efficiency of bio-digesters (Parawira, Citation2009; J. W. Mwirigi et al., Citation2009).
Similarly, an increase in the availability of extension services has a positive and significant (p 0.05) impact on the adoption of biogas technology. Contacting agricultural extension agents on a regular basis with rural households increases the likelihood of receiving relevant information about the benefits and drawbacks of biogas technology and thus its adoption (Kelebe, Citation2018). The likelihood of households adopting biogas technology increased by 0.4 unit for every unit increase in service from the extension agents. However, the lack of effective extension services on bio-slurry treatment represents a significant knowledge gap for pig farmers (Vu et al., Citation2007).
3.4. Checking balance test and common support and choice of matching algorism
Following t-test analysis, the next step in PSM is a covariate balance test to determine the common support region. The balancing test identifies where the observed characteristics of the treatment and control groups overlap. The balancing assumption is met for crop yield covariates using propensity score algorithms. The estimated propensity score distribution of biogas user and non-user households ranges between 0.16989555 and 1, with a mean score of 0.9493965 (SD = 0.1453964). As a result, observations with propensity scores that fall outside of this range of common support are removed from the sample.
The next step is to calculate the impact of biogas technology on crop yield by comparing the effects of tread and control variables on crop yield using the four PSM matching algorithms, namely the nearest neighbor, radius, kernel, and stratification matching methods.
The results of the four PSM techniques mentioned above, as shown in Table , reveal that using bio-slurry has a positive impact on crop yield. As a result of the application of a bio-slurry treatment on crop fields, the annual crop yield of biogas user households was found to be higher on average by 43, 40.8, 42.4, and 39.6 quintals/year/household compared to non-users, respectively. According to the propensity score match result, biogas technology adoption had a significant and positive impact on crop yields.
Table 6. ATT estimation results of the impact of biogas technology adoption on crop yield (Bootstrapped standard errors in parenthesize)
The impact of bio-slurry application is comparable to that of chemical fertilizer application. Crop yields treated with bio-slurry are significantly higher than those treated with chemical fertilizer. Despite the fact that the productivity effect of bio-slurry varies from crop to crop, households that used it increased crop yield (Warnars & Oppenoorth, Citation2014; SNV, Citation2017 Clemens et al., Citation2018; Tah & Ngwa, Citation2015; Lansing et al., Citation2010; Ferdous et al., Citation2020).
Table shows the estimation results for the average treatment effect on the treated (ATT) of the outcome variable using PSM techniques.
According to the literature, combining bio-slurry from cow dung with chemical fertilizer has a significant effect on increasing all crop yields (Warnars & Oppenoorth, Citation2014). For example, bio-slurry application increased maize yield (Ferdous et al., Citation2020), green bean yield (Musse et al., Citation2020), radish root crops (Shaheb et al., Citation2015), fruit yields (Biramo et al., Citation2019), and wheat yield (Garg et al., Citation2005).
According to Ferdous et al. (Citation2020), the combined application of biogas slurry and chemical fertilizer resulted in a 20–24 percent higher grain yield, a 22–23 percent higher gross return, and a 52–53 percent higher gross margin when compared to the traditional farmer’s practice. Warnars and Oppenoorth (Citation2014) also discovered that biogas slurry application increases the average yield of rice, maize, wheat, and cotton when compared to farmyard manure (FYM) application. While treated with compost, the average yield of Teff increases from 12 q/ha to 25 q/ha and 30 q/ha when treated with chemical fertilizer. Moreover, the combination of bio-slurry and chemical fertilizers improves nitrogen transformation on the crop and increases corn yield significantly more than chemical fertilizer treatment (SNV, Citation2017) and farm yard manure (FYM; Lansing et al., Citation2010). Garg et al. (Citation2005) also found that when plots were amended with biogas slurry, the leaf area index, root length density, and grain yield of wheat were higher than in unamended plots.
Furthermore, farmers observed a significant increase in financial assets after implementing biogas technologies, owing to increased sales of surplus production, particularly maize and teff, the two most cultivated crops in the study area. Their savings increased as a result of higher agricultural produce sales, lower chemical fertilizer costs, and reduced expenditure on fuel wood.
Thus, this study confirmed that combining biogas slurry with chemical fertilizer can achieve the goal of small-holder farmers. This arose as a result of the two opposing justifications. First, if only chemical fertilizers are applied to the soil without the addition of organic manure, land productivity will decline (Haque et al., Citation2015). Second, adding only organic manure to the soil will not result in the desired increase in crop yield. To address the issue, an integrated fertilization strategy (bio-slurry and chemical fertilizer) should be pursued in order to increase smallholder farmers’ access to soil fertility management. The wise use of organic and inorganic fertilizers to increase production and productivity of smallholder farmers is known as Integrated Nutrient Management (Karki & Expert, Citation2006). That is, if our agriculture is to improve in terms of productivity and quality, we must use all available inputs wisely.
However, the sustainable production of biogas has been hampered by a lack of technical follow-up and support from technology transfer stakeholders; corruption in access to technical training; a lack of water supply; and the high cost of installing biogas and purchasing spare parts.
The majority of respondent households state that when bio-digesters fail to function properly due to a lack of regular follow-up and support from technology dissemination stakeholders. As a result, they are unable to produce bio-slurry fertilizer. Energy experts did not provide them with continuous assistance. They also stated that officials come when they need information for a report or when the digester is being installed, but there is no continuous support or follow-up. As a result, digesters are unable to function properly. Due to such issues, sustainable biogas production was difficult.
4. Conclusions
The study uses PSM to look at how small-scale biogas technology uptake affects crop productivity. As the number of cattle heads increases, the likelihood of biogas technology adoption rises. Similar to this, households are more likely to embrace biogas technology if they have access to institutional support and follow-up, extension contacts, and training. The distance from markets and water sources, however, has a detrimental impact on the adoption of technology. A lack of regular follow-up and support, a lack of training, poor extension contact, a disproportionately greater distance to water sources, and a distance to the market where the technology spare parts are available are barriers for households that do not benefit from the technology.
The PSM comparison of agricultural yield between households that utilize and those that don’t utilize it reveals a significant difference. The use of bio-slurry fertilizer on their crop fields results in a favourable crop production premium of 41.5 quintals/year/household, according to the study. Between households that adopted and those that did not, there was a significant and favorable change in crop production on average. The principal organization, the National Biogas Programme of Ethiopia, should collaborate with agricultural and rural development offices to promote adequate community adoption of the biogas technology and its numerous economic benefits. In order to boost soil fertility, increase crop production, lower the cost of chemical fertilizer; assure food security, and increase household income, players participating in rural development and poverty reduction should incorporate biogas technology into the agricultural input policy. The desire for this stems from the absence of bio-slurry from biogas technology in previous agricultural input policies, which is now being added to become one of the most precious alternatives to chemical fertilizer.
There were only a few houses using biogas technology during the study period. Thus, to maximize the technology’s beneficial effects, development agents should encourage farmers to use biogas technology by offering fundamental infrastructure, continuous follow-up and support, and access to technical training. Therefore, there is a pressing need to promote the use of biogas technology in order to assist smallholders.
Disclosure statement
No potential conflict of interest was reported by the author(s).
Additional information
Funding
Notes on contributors
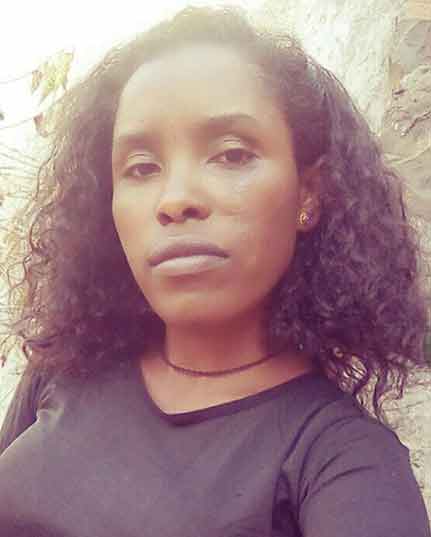
Fasika Chekol
Fasika Chekol Mekonnen, holds an MSc in Economics from Addis Ababa University, with a specialization in Economic Policy Analysis. The author is currently working as a lecturer in Economics at Debre Markos University. The author is interested in conducting research on the areas of technology adoption, production efficiency, agricultural marketing,, renewable energy sources, nonfarm business for women rural empowerment, employee training, and business networking alliance strategy for firm performance.
References
- Agbarevo, M. N. B., & Benjamin, N. (2013). Farmers’ perception of effectiveness of agricultural extension delivery in cross-river state, Nigeria. IOSR Journal of Agriculture and Veterinary Science, 2(6), 1–18.
- Balgah, R. A., Mbue, I. N., & Ngwa, K. A. (2018). The impacts of renewable energy on livelihoods: A case study of biogas adoption in Cameroon. Int. J. Dev. Sustain, 7, 220–239.
- Bansal, S., Yin, X., Savoy, H. J., Jagadamma, S., Lee, J., & Sykes, V. (2020). Long‐term influence of phosphorus fertilization on organic carbon and nitrogen in soil aggregates under no‐till corn–wheat–soybean rotations. Agronomy Journal, 112(4), 2519–2534. https://doi.org/10.1002/agj2.20200
- Becker, S. O., & Ichino, A. (2002). Estimation of average treatment effects based on propensity scores. The Stata Journal, 2(4), 358–377. https://doi.org/10.1177/1536867X0200200403
- Bensah, E. C., & Brew-Hammond, A. (2010). Biogas technology dissemination in Ghana: History, current status, future prospects, and policy significance. International Journal of Energy and Environment, 1(2), 277–294.
- Berhe, T. G., Tesfahuney, R. G., Desta, G. A., & Mekonnen, L. S. (2017). Biogas plant distribution for rural household sustainable energy supply in Africa. Energy and Policy Research, 4(1), 10–20. https://doi.org/10.1080/23815639.2017.1280432
- Biramo, G., Abera, G., & Biazin, B. (2019). Effects of dry bioslurry and chemical fertilizers on tomato growth performance, fruit yield and soil properties under irrigated condition in Southern Ethiopian. African Journal of Agricultural Research, 14(33), 1685–1692. https://doi.org/10.5897/AJAR2019.14372
- Caliendo, M., & Kopeinig, S. (2008). Some practical guidance for the implementation of propensity score matching. Journal of Economic Surveys, 22(1), 31–72. https://doi.org/10.1111/j.1467-6419.2007.00527.x
- Central Statistics Agency (CSA). (20202021). Statistical bulletin for crop and livestock product utilization (Private Peasant Holdings, Meher Season).
- Chen, L., Li, F., Li, W., Ning, Q., Li, J., Zhang, J., Zhang, J., Ma, D., & Zhang, C. (2020). Organic amendment mitigates the negative impacts of mineral fertilization on bacterial communities in Shajiang black soil. Applied Soil Ecology, 150, 103457. https://doi.org/10.1016/j.apsoil.2019.103457
- Clemens, H., Bailis, R., Nyambane, A., & Ndung’u, V. (2018). Africa biogas partnership program: A review of clean cooking implementation through market development in East Africa. Energy for Sustainable Development, 46, 23–31. https://doi.org/10.1016/j.esd.2018.05.012
- Dadi, L., Burton, M., & Ozanne, A. (2004). Durati on analysis of technology adoption in Ethiopian agriculture. Journal of Agricultural Economics, 55(3), 613–631. https://doi.org/10.1111/j.1477-9552.2004.tb00117.x
- Desai, R. J., Rothman, K. J., Bateman, B. T., Hernandez-Diaz, S., & Huybrechts, K. F. (2017). A propensity score based fine stratification approach for confounding adjustment when exposure is infrequent. Epidemiology (Cambridge, Mass), 28(2), 249. https://doi.org/10.1097/EDE.0000000000000595
- Dorosh, P., & Rashid, S. (2012). Introduction in food and agriculture in Ethiopia. Progress and Policy Challenges.
- EGZ. (2021). East Gojjam Zone, Agricultural and Rural Development Office, 2020. Ethiopia: Debre Markos.
- EGZ. (2021). East Gojjam Zone, Water, and Energy Office. Ethipia: Debre Markos.
- Endale, K. (2010). Genetic variability, heritability, correlation coefficient and pathanalysis for yield and yield related traits in upland rice (Oryzasativa L.).
- Ermiyas, A., Batu, M., & Teka, E. (2019). Determinants of rural poverty in Ethiopia: A household level analysis in the case of Dejen woreda. International Journal of Arts and Social Science, 10(2), 1–6.
- Eshete, G., & Stoop, C. (2007). Biogas for better life, brief programme profile. Biogas for Better Life. Brief Programme Profile.
- Fan, R., Du, J., Liang, A., Lou, J., & Li, J. (2020). Carbon sequestration in aggregates from native and cultivated soils as affected by soil stoichiometry. Biology and Fertility of Soils, 56(8), 1109–1120. https://doi.org/10.1007/s00374-020-01489-2
- Ferdous, Z., Ullah, H., Datta, A., Attia, A., Rakshit, A., & Molla, S. H. (2020). Application of biogas slurry in combination with chemical fertilizer enhances grain yield and profitability of maize (Zea Mays L.). Communications in Soil Science and Plant Analysis, 51(19), 2501–2510. https://doi.org/10.1080/00103624.2020.1844728
- Garg, R. N., Pathak, H., Das, D. K., & Tomar, R. K. (2005). Use of flyash and biogas slurry for improving wheat yield and physical properties of soil. Environmental Monitoring and Assessment, 107(1), 1–9. https://doi.org/10.1007/s10661-005-2021-x
- Gelmesa, D., Abebie, B., & Desalegn, L. (2012). Regulation of tomato (Lycopersicon esculentum Mill.) fruit setting and earliness by gibberellic acid and 2, 4-dichlorophenoxy acetic acid application. African Journal of Biotechnology, 11(51), 11200–11206.
- Ghimire, P. C. (2013). SNV supported domestic biogas programmes in Asia and Africa. Renewable Energy, 49, 90–94. https://doi.org/10.1016/j.renene.2012.01.058
- Goberna, M., Podmirseg, S. M., Waldhuber, S., Knapp, B. A., García, C., & Insam, H. (2011). Pathogenic bacteria and mineral N in soils following the land spreading of biogas digestates and fresh manure. Applied Soil Ecology, 49, 18–25. https://doi.org/10.1016/j.apsoil.2011.07.007
- Guta, D. D. (2014). Effect of fuelwood scarcity and socio-economic factors on household bio-based energy use and energy substitution in rural Ethiopia. Energy Policy, 75, 217–227.
- Haile, A., & Ayalew, T. (2018). Comparative study on the effect of bio-slurry and inorganic N-fertilizer on growth and yield of kale (Brassica oleracea L.). African Journal of Plant Science, 12(4), 81–87. https://doi.org/10.5897/AJPS2018.1639
- Haque, M. A., Jahiruddin, M., Rahman, M. M., & Saleque, M. A. (2015). Usability of bioslurry to improve system productivity and economic return under potato-rice cropping system. Research in Agriculture Livestock and Fisheries, 2(1), 27–33. https://doi.org/10.3329/ralf.v2i1.23026
- Kaluski, D. N., Ophir, E., & Tilahun, A. (2001). Food security and nutrition – The Ethiopian case for action. Public Health Nutrition, 5(3), 373–381. https://doi.org/10.1079/PHN2001313
- Kamran, M., Huang, L., Nie, J., Geng, M., Lu, Y., Liao, Y., Zhou, F., & Xu, Y. (2021). Effect of reduced mineral fertilization (NPK) combined with green manure on aggregate stability and soil organic carbon fractions in a fluvo-aquic paddy soil. Soil and Tillage Research, 211, 105005. https://doi.org/10.1016/j.still.2021.105005
- Karki, A. B., & Expert, B. (2006). Country report on the use of Bio-slurry in Nepal, Kathmandu: BSP-Nepal.
- Kelebe, H. E. (2018). Returns, setbacks, and future prospects of bio-energy promotion in northern Ethiopia: The case of family-sized biogas energy. Energy, Sustainability and Society, 8(1), 1–14. https://doi.org/10.1186/s13705-018-0171-2
- Khandker, S. R., Koolwal, G. B., & Samad, H. A. (2009). Handbook on impact evaluation: Quantitative methods and practices. World Bank Publications.
- Lansing, S., Martin, J. F., Botero, R. B., Da Silva, T. N., & Da Silva, E. D. (2010). Wastewater transformations and fertilizer value when co-digesting differing ratios of swine manure and used cooking grease in low-cost digesters. Biomass and Bioenergy, 34(12), 1711–1720. https://doi.org/10.1016/j.biombioe.2010.07.005
- Liu, Y., Kim, C., Wu, A. D., Gustafson, P., Kroc, E., & Zumbo, B. D. (2020). Investigating the performance of propensity score approaches for differential item functioning analysis. Journal of Modern Applied Statistical Methods, 18(1), 18. https://doi.org/10.22237/jmasm/1556669280
- Mengistu, M. G., Simane, B., Eshete, G., & Workneh, T. S. (2016). Factors affecting households’ decisions in biogas technology adoption, the case of Ofla and Mecha Districts, northern Ethiopia. Renewable Energy, 93, 215–227. https://doi.org/10.1016/j.renene.2016.02.066
- Menmeru, T. (2014). Population growth and cultivated land in rural Ethiopia: Land use dynamics, access, farm size, and fragmentation.
- Mulugeta, S., Sentayehu, A., & Kassahun, B. (2012). Genetic variability, heritability. Correlation Coefficient and Path Analysis for Yield and Yield Related Traits in Upland Rice (Oryzasativa L.).
- Musse, Z. A., YosephSamago, T., & Beshir, H. M. (2020). Effect of liquid bio-slurry and nitrogen rates on soil physico-chemical properties and quality of green bean (Phaseolus vulgaris L.) at Hawassa Southern Ethiopia. Journal of Plant Interactions, 15(1), 207–212. https://doi.org/10.1080/17429145.2020.1781270
- Mwirigi, J., Balana, B. B., Mugisha, J., Walekhwa, P., Melamu, R., Nakami, S., & Makenzi, P. (2014). Socio-economic hurdles to widespread adoption of small-scale biogas digesters in Sub-Saharan Africa: A review. Biomass and Bioenergy, 70, 17–25. https://doi.org/10.1016/j.biombioe.2014.02.018
- Mwirigi, J. W., Makenzi, P. M., & Ochola, W. O. (2009). Socio-economic constraints to adoption and sustainability of biogas technology by farmers in Nakuru Districts, Kenya. Energy for Sustainable Development, 13(2), 106–115. https://doi.org/10.1016/j.esd.2009.05.002
- Nasery, V., & Rao, A. (2011). Biogas for rural communities.Center for Technology Alternatives for Rural Areas, Indian Institute of Technology Bombay. www.cse.iitb.ac.in/~sohoni/pastTDSL/BiogasOptions.pdf Accessed: 31 May 2012
- NBE. (2020). National Bank of Ethiopia Annual report on Ethiopian Economy. Ethiopia: Addis Ababa.
- NBE. (2021). National Bank of Ethiopia Annual report on Ethiopian Economy.
- Parawira, W. (2009). Biogas technology in sub-Saharan Africa: Status, prospects and constraints. Reviews in Environmental Science and Bio/Technology, 8(2), 187–200. https://doi.org/10.1007/s11157-009-9148-0
- Rahman, N., & Tetteh, F. M. (2014). Improved quality protein maize response to NPK fertilizer in Sudan Savanna Agro-ecological Zone of Ghana. Journal of Agricultural and Biological Science, 9(5), 166–171.
- Rohne Till, E. (2021). A green revolution in sub‐Saharan Africa? The transformation of Ethiopia’s agricultural sector. Journal of International Development, 33(2), 277–315. https://doi.org/10.1002/jid.3523
- Roser, M., & Ortiz-Ospina, E. (2013). Global extreme poverty. Our World in Data.
- Shaheb, M. R., Nazrul, M. I., Zonayed-Ull-Noor, A. K. M., Hossain, K. M. F., & Saha, D. (2015). Bio-slurry influences the yield and profitability of radish. Journal of Sylhet Agricultural University, 2, 9–14.
- Shallo, L., Ayele, M., & Sime, G. (2020). Determinants of biogas technology adoption in southern Ethiopia.Energy. Sustainability and Society, 10(1), 1–13.
- Shallo, L., & Sime, G. (2019). Determinants of functional status of family size bio-digesters: Empirical evidence from southern Ethiopia. International Journal of Sustainable Energy, 38(5), 493–510. https://doi.org/10.1080/14786451.2018.1538145
- SNV. (2017) . Biogas Production and Utilization in Ethiopia–Challenges and Opportunities. Netherland Development Organization (SNV).
- Tah, C. K., & Ngwa, K. A. (2015). Biogas Production: Impact on Farmers’ Incomes. Int. J. Emerg. Trends Multidiscip, 1, 199–210.
- Vu, T. K. V., Tran, M. T., & Dang, T. T. S. (2007). A survey of manure management on pig farms in Northern Vietnam. Livestock Science, 112(3), 288–297. https://doi.org/10.1016/j.livsci.2007.09.008
- Wang, X., Qi, J. Y., Zhang, X. Z., Li, S. S., Virk, A. L., Zhao, X., Zhang, X.-Z., Xiao, X.-P., & Zhang, H. L. (2019). Effects of tillage and residue management on soil aggregates and associated carbon storage in a double paddy cropping system. Soil and Tillage Research, 194, 104339. https://doi.org/10.1016/j.still.2019.104339
- Warnars, L., & Oppenoorth, H. (2014). Bioslurry: A supreme fertilizer. A Study on Bioslurry Results and Uses. Hivos. https://www.hivos.org/sites/default/files/bioslurry_book.pdf
- Wolde, T. G. (2021). Impact of row-seeding technology adoption on teff productivity, household welfare and asset holding: Evidence from South West Shoa Zone. Oromia Regional State, Using Propensity Score Matching.
- Wu, H., Ding, S., Pandey, S., & Tao, D. (2010). Assessing the impact of agricultural technology adoption on farmers’ well‐being using propensity‐score matching analysis in rural China. Asian Economic Journal, 24(2), 141–160. https://doi.org/10.1111/j.1467-8381.2010.02033.x
- Yu, F. B., Luo, X. P., Song, C. F., Zhang, M. X., & Shan, S. D. (2010). Concentrated biogas slurry enhanced soil fertility and tomato quality. Acta Agriculturae Scandinavica Section B–Soil and Plant Science, 60(3), 262–268.
- Zhu, L., Zhang, F., Li, L., & Liu, T. (2021). Soil C and aggregate stability were promoted by bio-fertilizer on the North China Plain. Journal of Soil Science and Plant Nutrition, 21(3), 2355–2363. https://doi.org/10.1007/s42729-021-00527-8
Appendix
Table 1. Multicollinearity test
Table 2. Functional specification test