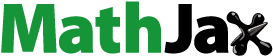
Abstract
This study explores the adoption of blockchain technology within the Ethiopian National Quality Infrastructure (NQI). Data from 178 professionals representing various organizations and roles were collected to investigate key adoption factors. By integrating the Technology Acceptance Model (TAM) and the Technology-Organization-Environment (TOE) framework, external factors (Technology Compatibility, Relative Advantage, Government Support, and Policy) and internal factors (perceived usefulness, perceived ease of use, and intention to use) were examined. A dual-stage analytical approach involving partial least square-based structural equation model (PLS-SEM) and artificial neural network (ANN) analyses was employed. The findings emphasize the significance of technological compatibility, perceived usefulness, and top management support as determinants of blockchain adoption in the NQI. Particularly, the compatibility of the existing system emerges as the most influential factor in adopting blockchain technology within the Ethiopian NQI. This study enhances the understanding of blockchain adoption within the NQI context, providing valuable insights for successful implementation. It contributes to the existing knowledge in this area and offers practical implications for quality infrastructure management.
REVIEWING EDITOR:
1. Introduction
Blockchain technology, a ground-breaking innovation that utilizes cryptographic techniques to link data in blocks, is transforming the digital landscape rapidly. Positioned as the fourth-generation disruptive technology after steam engines, electricity, and the Internet (Kulkarni & Patil, Citation2020), it is regarded as the fifth evolution in computing (Attaran & Gunasekaran, Citation2019). Blockchain has emerged as a powerful driver of innovation within the business landscape because of its potential to reshape organizational structures, transactional practices, and business operations (Behnke & Janssen, Citation2019; Cermeño, Citation2016; Kulkarni & Patil, Citation2020). This is a novel paradigm that renders existing models obsolete (Hervey, Citation2023).
Blockchain technology operates on a distributed data structure, utilizing a peer-to-peer network (Marsal-Llacuna, Citation2018) in which blocks are interconnected through cryptographic hashes with copies stored across all nodes (Al-Saqaf & Seidler, Citation2017). It offers notable features, such as data security, tamper-proof transactions, and data validation within a distributed ledger among network members, resulting in virtually immutable transaction records (Grewal et al., Citation2018; Zhang et al., Citation2020). This results in virtually immutable transaction records, making it a valuable tool for ensuring secure management of national quality infrastructure (NQI).
The challenges of transparency and trust caused by outdated administrative systems, which are prevalent in developing countries such as Ethiopia, underscore the need for the implementation of blockchain technology in the national quality infrastructure (Kim, Citation2023). Moreover, nonconformance cost of export products accounts for a substantial portion (99%) of the national cost of quality in the Export-Import sector in Ethiopia (Beshah et al., Citation2017). By leveraging blockchain technology, Ethiopia can enhance transparency, traceability, and accountability throughout the activities under the NQI, mitigating the risk of nonconformance and bolstering trust among stakeholders. Blockchain’s immutable and decentralized nature allows for secure and tamper-proof record-keeping, reducing the likelihood of fraud, counterfeiting, and quality-related issues (Queiroz et al., Citation2019). Therefore, adopting blockchain technology in the national quality infrastructure holds great potential to improve multidimensional quality management issues. Furthermore, given that Quality Infrastructure (QI) plays a crucial role in domestic and international markets by ensuring proper functioning and facilitating market entry (Runde, Citation2017), it is essential to consider the integration of blockchain technology within the existing QI framework. The World Bank defines quality infrastructure as legal and regulatory structures and processes related to standardization, accreditation, metrology, and conformity assessments (The World Bank Ethiopia, Citation2016), indicating the various elements that collectively form the foundation of QI. By incorporating blockchain technology into the QI framework, Ethiopia can address the challenges of transparency, trust, and nonconformance, ultimately enhancing the overall quality management system.
While previous studies have explored the potential of blockchain in enhancing transparency and trust and combating trade discrimination (Díaz et al., Citation2021), a research gap exists in the specific application of blockchain to the National Quality Infrastructure (NQI). Limited research exists on individual NQI components, such as Metrology (Miličević et al., Citation2022) and the Accreditation Process (Chivu et al., Citation2022), and no study has examined the adoption of blockchain in the NQI context. As blockchain is still a novel concept within NQI, challenges in its implementation may exist. Therefore, there is a pressing need to comprehensively understand the influencing factors and adoption mechanisms of successful integration at a fundamental level. To address this gap, this study aims to empirically examine blockchain adoption in the national quality infrastructure sector using integration of two well-known adoption theories- Technology Acceptance Model (TAM) and Technology-Organization-Environment (TOE).
In recent years, various adoption theories, including the TAM (Wang et al., Citation2022), Theory of Diffusion of Innovation (DOI) (Wamba & Queiroz, Citation2022), Theory of Planned Behavior (TPB) (Soomro et al., Citation2022), Theory of Reasoned Action (TRA) (Marikyan et al., Citation2022), Unified Theory of Acceptance and Use of Technology (UTAUT) (Abu Afifa et al., Citation2022), and TOE Framework (Malik et al., Citation2021; Wong et al., Citation2020), have been employed to study the acceptance behavior of blockchain technology in to different systems. TAM offers insights into users’ acceptance and utilization of technology by considering user attitudes, technical features, and social impact. The TOE framework comprehensively explains the adoption and implementation of technological innovations by considering technological, organizational, and environmental factors. The integrated model draws on the strengths of both TAM in explaining individual-level adoption, and TOE in considering organizational-level adoption from multiple perspectives (Wang et al., Citation2022). This makes it suitable to analyze blockchain adoption behavior in the NQI sector. Scholars have applied this integrated approach in various research contexts, including the adoption of blockchain technology (Kumar Bhardwaj et al., Citation2021; Ullah et al., Citation2021; Wang et al., Citation2022), e-commerce (Awa et al., Citation2015), and other trending technologies such as AI (Na et al., Citation2022). However, there is still a gap in the literature regarding the adoption of blockchain technology in specific domains and different geographical and cultural settings. Cultural differences and economic variations significantly influence technological adoption, and there is a lack of comprehensive research examining the implementation and impact of blockchain technology on the National Quality Infrastructure (NQI) domain.
This study aims to identify the key factors driving the adoption of blockchain technology in Ethiopian National Quality Infrastructure. By integrating the Technology Acceptance Model (TAM), a theoretical framework that explains the factors influencing the acceptance and adoption of new technology, and the technology-organization-environment (TOE) framework, a conceptual model that examines the factors influencing technology adoption and implementation within an organizational context, it seeks to comprehensively understand users’ perceptions of blockchain adoption in this sector.
To address these research objectives, the study will address the following research questions:
RQ1: What are the key factors that directly influence the adoption of blockchain technology in the National Quality Infrastructure, with an emphasis on the Ethiopian context?
RQ2: Among these key factors, which has a greater association with intentions to adopt and use blockchain technology in the Ethiopian National Quality Infrastructure?
2. Theoretical background
2.1. Blockchain technology
Blockchain technology initially gained prominence in the realm of crypto-currencies, particularly Bitcoin, as a means of facilitating secure transactions (Nakamoto, Citation2018). However, the inherent features of blockchain, such as traceability, transparency, smart contracts, and security, have led to its widespread adoption in domains beyond cryptocurrency (Taherdoost, Citation2022). Industries such as finance (Kabra et al., Citation2020; Tsai et al., Citation2018), healthcare (Choudhury et al., Citation2019), personal identity protection (Nokhbeh Zaeem & Barber, Citation2020), supply chain management (Pranav et al., Citation2019), Internet of Things (IoT) (Minoli & Minoli, Citation2019), and even government activities like e-voting (Al-Rawy & Elci, Citation2019)have embraced blockchain technology, highlighting its disruptive potential across various industries. One notable feature of blockchain is its decentralized nature, which eliminates the need for a central authority, making it suitable for applications where trust and transparency are crucial (Manoj et al., Citation2022). For example, in supply chain management, blockchain can be integrated to create an immutable record of transactions, ensuring traceability and authenticity of products from their origin to the end consumer (Chittipaka et al., Citation2023; Kumar Bhardwaj et al., Citation2021; Wamba & Queiroz, Citation2022). This feature can help combat counterfeit goods and enhance consumer confidence (Sun et al., Citation2023).
Another feature of blockchain is its ability to provide enhanced security through cryptographic algorithms (Albshaier et al., Citation2024; Taherdoost, Citation2023). This makes it particularly valuable in sectors such as healthcare (Choudhury et al., Citation2019; Kouhizadeh et al., Citation2021), where sensitive patient data needs to be securely stored and shared. By implementing blockchain technology, healthcare systems can ensure the privacy and integrity of patient records, prevent unauthorized access or tampering, and enable seamless and secure sharing of data between healthcare providers.
Furthermore, blockchain’s smart contract functionality enables the automation and execution of predefined contractual terms without the need for intermediaries (Manoj et al., Citation2022; Vionis & Kotsilieris, Citation2023). This feature can be leveraged in areas such as finance and insurance, where complex transactions and agreements are common. By utilizing blockchain-based smart contracts, organizations can reduce costs, improve efficiency, and minimize the potential for fraud or errors.
Consequently, the attributes of blockchain technology, such as trust, transparency, traceability, authenticity, enhanced security, and automation of contractual terms, can have a profound impact on national quality infrastructure (NQI). By integrating blockchain into this context, it would enable the creation of a decentralized and transparent system that ensures trust and reliability in quality-related transactions and processes. The traceability and authenticity features would enhance the ability to track and verify the origin and quality of products or services, improving overall quality control and consumer confidence. The enhanced security measures provided by blockchain’s cryptographic algorithms would safeguard sensitive data, ensuring privacy and data integrity. The automation of contractual terms through smart contracts would streamline and simplify quality-related agreements and processes, reducing administrative burdens and minimizing the potential for errors or fraud.
2.2. Technology adoption theories
2.2.1. The TAM
The TAM is a widely recognized and extensively used theoretical framework for understanding the acceptance and utilization of technology (Davis, Citation1989). TAM posits that user attitudes toward technology, including perceived usefulness and ease of use, significantly influence their intention to adopt and use the technology. Additionally, social influence, such as the opinions of peers or experts, plays a crucial role in shaping individuals’ perceptions and adoption decisions.
Numerous studies have demonstrated the applicability and relevance of TAM in various research contexts (Namahoot & Rattanawiboonsom, Citation2022; Nuryyev et al., Citation2020; Sciarelli et al., Citation2022). In the study (Sciarelli et al., Citation2022), the authors delve into the factors influencing blockchain adoption among Italian companies. They employ an extended TAM model to develop a conceptual framework and test hypotheses using PLS-SEM on a sample of 250 firms. The findings shed light on the determinants of blockchain adoption and reveal the moderating role of firm size. This research offers valuable insights into the adoption of blockchain technology in the Italian context. Another noteworthy paper (Nuryyev et al., Citation2020), investigated the factors influencing the intention of tourism and hospitality SMEs to adopt cryptocurrency payments. The study integrated strategic orientation, social influence, and owner/manager characteristics into the technology acceptance model. By examining their impact on perceived usefulness and ease of use, the paper provides an empirical contribution to understanding technology adoption in SMEs within the tourism and hospitality sector. Furthermore (Namahoot & Rattanawiboonsom, Citation2022), examined consumers’ intention to adopt cryptocurrency platforms in Thailand. The authors extended the TAM to assess key determinants such as perceived usefulness, ease of use, and innovativeness. This research contributes to our understanding of consumer behavior and their attitudes towards an emerging financial technology in cases considered.
2.2.2. The TOE Framework
The TOE Framework is a comprehensive theoretical model that explains the adoption and implementation of technological innovations within organizations (Nguyen et al., Citation2022). This framework considers the interplay between technological factors, organizational characteristics, and environmental influences. Technological factors encompass the attributes and complexity of the technology itself, while organizational characteristics include factors such as organizational structure, culture, and resources. Environmental influences refer to external factors, such as market conditions and regulatory requirements. The TOE Framework has been widely used in research to examine technology adoption and implementation across various industries (Malik et al., Citation2021; Chittipaka et al., Citation2023). By leveraging the TOE Framework, we can gain a holistic understanding of the factors that shape the adoption and success of blockchain technology within organizations.
In the empirical study by Chittipaka et al. (Citation2023), the authors focus on the adoption of blockchain technology in supply chain management within the Indian context. They employed the TOE framework to examine factors influencing adoption. Through the development of hypotheses related to technological, organizational, and environmental dimensions, the research model is tested using survey data from 525 professionals. The findings offer valuable insights into the considerations for blockchain adoption and carry both theoretical and practical implications. Moreover, this study contributes to the growing body of literature on blockchain adoption within supply chain management, specifically in the Indian context. Similarly Malik et al. (Citation2021), investigated the adoption of blockchain technology among organizations in Australia. The study extended the TOE framework to examine the factors influencing adoption. By developing well-founded hypotheses and utilizing a quantitative research method, the authors provide valuable insights into the adoption dynamics. However, it is suggested that the study could further discuss the generalizability of the findings and consider additional contextual factors specific to the case area’s setting.
2.2.3. Integrated approaches
Integrated approaches in technology adoption leverage multiple technology adoption theories to provide a comprehensive understanding of the factors influencing the acceptance and utilization of new technologies. By integrating these theories, researchers can capture a broader range of factors and perspectives in their analysis (Ullah et al., Citation2021; Wang et al., Citation2022; Wamba & Queiroz, Citation2022). In the paper (Wang et al., Citation2022), the authors systematically explored the factors influencing the adoption of blockchain technology in the construction industry. They integrated the TOE framework and TAM to develop a theoretical model. By formulating well-grounded hypotheses on technical, organizational, and environmental dimensions, the study investigated the impact on perceived usefulness and ease of use. The findings have the potential to provide valuable insights for promoting blockchain adoption in the construction sector. Another paper of significance that further contributed to the literature is Ullah et al. (Citation2021), which explored an expanded TAM to assess the factors affecting the adoption of blockchain technology in smart learning environments. By integrating constructs from the Diffusion of Innovation theory (DOI) with TAM, the authors developed hypotheses related to trialability, relative advantage, compatibility, perceived ease of use, and usefulness. Through an online survey and validation of the conceptual model, the study offers useful insights into the adoption of emerging technologies in educational settings. Furthermore, Wamba and Queiroz (Citation2022), proposed a multi-stage model to understand the diffusion of blockchain technology across supply chains. Drawing upon established five adoption theories, the model examines factors influencing intention to adopt, the adoption process, and routinization of blockchain at different stages. The empirical validation of the model using a large dataset from India and the US provides valuable insights for both researchers and practitioners seeking to understand the adoption dynamics of blockchain technology in supply chain contexts.
Despite the efforts made in the past researches in different perspectives of technology adoption in different setups, there is an existing gap in the literature regarding the adoption of blockchain technology, specifically in the domain it encompasses, as well as in the geographical and cultural setups in the cases considered, as technological adoption is significantly affected by cultural differences and differences in economic status across the globe (Lee et al., Citation2013; Oke et al., Citation2014). Moreover, although previous studies have explored blockchain adoption in various industries (), with different analytical techniques used to test their respective hypotheses and research models, there is a lack of comprehensive research examining its implementation and impact on NQI.
Table 1. Summary of previous studies on blockchain adoption.
This study employs an integrated theoretical approach by utilizing TAM to assess individual-level perceptions of the usefulness and ease of use of blockchain for NQI tasks. Additionally, this research incorporates the TOE framework to consider the organizational and contextual factors that influence adoption (Wang et al., Citation2020, Citation2022). By combining micro- and macro-perspectives, this integrated application of TAM and TOE provides valuable insights into the determinants of blockchain adoption for national quality governance, within Ethiopian context, benefiting both researchers and practitioners.
3. Hypothesis development and research model
In this section, we establish a conceptual research model that forms the foundation for examining and testing the developed hypotheses. visually represents this model, providing a clear illustration of the relationships and variables under investigation. Subsequently, we present the hypotheses concerning the specific aspects addressed in the subsequent sub-sections.
3.1. The Technology-Organization-Environment (TOE) framework
3.1.1. The technological dimension
3.1.1.1. Technological Compatibility
Technological compatibility (TC) refers to how well a new technology aligns and integrates with the adopter’s existing practices, needs, values, beliefs, and experiences (Chittipaka et al., Citation2023; Kumar Bhardwaj et al., Citation2021; Rehman et al., Citation2022; Ullah et al., Citation2021). In the context of this study on blockchain technology adoption in the NQI, TC reflects how well blockchain technology is perceived as compatible with specific requirements, work processes, and existing systems within the NQI sector.
Previous studies have consistently demonstrated that TC play a crucial role in shaping the intention to adopt new technology. These findings are supported by those of various studies (Chittipaka et al., Citation2023; Dutta et al., Citation2020; Koh et al., Citation2020; Kumar Bhardwaj et al., Citation2021; Ullah et al., Citation2021; Yadav et al., Citation2020). TC has been identified as a key factor influencing the adoption of blockchain technology in smart learning environments (Ullah et al., Citation2021). Furthermore, the positive impact of TC on intention to adopt blockchain technology extends to different sectors and applications. For instance, it has been found to have a significant positive effect on the intention to adopt blockchain in supply chain operations (Dutta et al., Citation2020), transportation and logistics (Koh et al., Citation2020), agricultural supply chains (Yadav et al., Citation2020), and supply chain management (Chittipaka et al., Citation2023; Kumar Bhardwaj et al., Citation2021). These consistent findings across diverse contexts highlight the vital role of TC in driving the adoption of blockchain technology in various domains.
Prior research has consistently indicated that TC plays a significant role in shaping the perceived usefulness of new technologies. This finding is supported by previous studies (Karjaluoto & Vaccaro, Citation2009; Li et al., Citation2019; Schmidthuber et al., Citation2020). Specifically, TC was found to have a significant positive effect on the perceived usefulness of B2B green marketing (Karjaluoto & Vaccaro, Citation2009). Additionally, it has been identified as a significant factor that influences the perceived usefulness of smart wearables (Li et al., Citation2019). These findings highlight the importance of TC in enhancing the perceived usefulness of innovative technologies across different domains.
Building upon the results of previous studies and the discussion above, we propose the following hypotheses:
H1: Technological compatibility (TC) has a positive influence on the behavioral intention to adopt and use Blockchain Technology in the National Quality Infrastructure (BCT in NQI).
H2: Technology compatibility (TC) has a positive influence on the perceived usefulness of Blockchain Technology in the National Quality Infrastructure (BCT in NQI).
In this study, RA refers to the perceived superiority of blockchain technology over existing technologies and practices. It encompasses the positive difference between the benefits that NQI organizations can obtain from adopting blockchain and the efforts required for its implementation. These benefits include improved transparency, enhanced traceability, increased reliability, and potential gains in efficiency. Non-tangible advantages, such as enhanced reputation, heightened customer satisfaction, and improved response speed, are also part of the relative advantage within the NQI sector.
Previous studies have consistently revealed a significant positive relationship between RA and intention to adopt and use technology. These findings are supported by studies such as Wong et al. (Citation2020), Kumar Bhardwaj et al. (Citation2021), Clohessy and Acton (Citation2019), Kapoor et al. (Citation2015), Puklavec et al. (Citation2018), Albrecht et al. (Citation2018). Importantly, RA has been found to exert a positive influence on the intention of Small and Medium Enterprises (SMEs) to adopt blockchain in supply chains (Kumar Bhardwaj et al., Citation2021). Additionally, perceived RA has been identified as a key factor influencing customer intention to use technology (Albrecht et al., Citation2018; Alkhateeb et al., Citation2009; Hardgrave et al., Citation2003). Moreover, the positive relationship between RA and intention to adopt and use technology extends to specific applications within different domains. Notably, research has established a significant positive relationship between RA and the intention to adopt and use interbank mobile payments (Kapoor et al., Citation2015), business intelligence systems (Puklavec et al., Citation2018), and blockchain in the energy sector (Orji et al., Citation2020). These consistent findings across diverse technological contexts underscore the importance of RA in shaping users’ intention to adopt and use innovative technologies. Consequently, studying the relationship between RA and behavioral intention to adopt and use blockchain technology in the NQI sector is crucial.
Therefore, drawing on the conclusions of prior research and the aforementioned rationale, we propose the following hypotheses:
H3: Relative advantage (RA) has a positive influence on the behavioral intention to adopt and use blockchain technology in the NQI.
H4: Relative advantage (RA) has a positive influence on the perceived usefulness of blockchain technology in NQI.
3.1.2. Organizational dimension
Top Management Support (TMS): Top management support (TMS) refers to the extent to which employees perceive their supervisors’ care, value their contributions, and receive support in terms of resources, guidance, mentorship, and problem-solving related to the adoption of new technologies (Abu-Akel & Ibrahim, Citation2023; Al-Saqaf & Seidler, Citation2020; Haneem et al., Citation2019; Nasongkhla & Shieh, Citation2023; Premkumar & Roberts, Citation1999). In the context of the NQI, the understanding and active involvement of senior executives in embracing new technologies such as blockchain is a crucial aspect to consider when examining the behavioral intention to adopt and use blockchain technology (Kumar Bhardwaj et al., Citation2021). Previous studies have consistently shown that TMS directly influences the intention to adopt and use new technologies (Kouhizadeh et al., Citation2021). Furthermore, research has highlighted the vital role of top management in resource allocation, facilitating technology adoption, and ensuring its successful implementation (Kumar Bhardwaj et al., Citation2021). Leadership support provided by top management plays a crucial role in allocating financial and human resources for technology adoption and conveying the importance of blockchain adoption to employees (Al-Kubaisy & Al-Somali, Citation2023; Guan et al., Citation2023; Wong et al., Citation2020). Scholars have recognized top management as the primary variable responsible for ensuring the successful approval of new technologies within an organization (Al-Saqaf & Seidler, Citation2020; Abu-Akel & Ibrahim, Citation2023; Premkumar & Roberts, Citation1999; Wu et al., Citation2003). Moreover, TMS helps overcome organizational change resistance, which is a common barrier to technological adoption (Al-Kubaisy & Al-Somali, Citation2023; Clohessy & Acton, Citation2019). By actively supporting and advocating blockchain technology, top management can create an environment that encourages its successful implementation and integration within the NQI, addressing resistance, and ensuring a smooth adoption process.
Furthermore, the significance of the relationship between TMS and perceived ease of use of new technology has been firmly established in prior research (Al-Saqaf & Seidler, Citation2020; Abu-Akel & Ibrahim, Citation2023; Haneem et al., Citation2019; Nasongkhla & Shieh, Citation2023). Previous studies have consistently demonstrated that higher levels of TMS contribute to users’ perceptions of ease of use and confidence in the system (Abu-Akel & Ibrahim, Citation2023; Nasongkhla & Shieh, Citation2023). This understanding is crucial for ensuring successful technology acceptance and implementation efforts within the NQI, emphasizing the importance of establishing appropriate support structures to facilitate the staff uptake of crucial technologies (Nasongkhla & Shieh, Citation2023). Furthermore, research on technology adoption highlights the positive influence of TMS on perceived ease of use in various applications (Al-Saqaf & Seidler, Citation2020; Haneem et al., Citation2019). Notably, TMS positively impacts the perceived ease of use of e-filing adoption and mastering data management (Al-Saqaf & Seidler, Citation2020; Haneem et al., Citation2019), underscoring its critical role in promoting the ease of use of new technology across different domains. These findings reinforce the need for effective support structures to facilitate successful blockchain technology adoption within the NQI, while recognizing the importance of TMS. Therefore, considering the results of previous studies and the aforementioned line of reasoning, we propose the following hypothesis:
H5: Top management support (TMS) positively influences the behavioral intention to adopt and use blockchain technology in NQI.
H6: Top management support (TMS) positively influences the perceived ease of use of blockchain technology in NQI.
3.1.3. Environmental dimensions
Government Support and Policy (GSP): Government support and policy (GSP) encompassing regulations, incentives, and measures are crucial for promoting blockchain adoption (Shi & Yan, Citation2016). In this study, government support refers to the regulations and initiatives promoting blockchain adoption in NQI (Al-Kubaisy & Al-Somali, Citation2023). This includes norms, guidance, and regulations shaping individuals’ perceptions of the difficulty and value of adopting blockchain technology within the NQI (Wang et al., Citation2022). Additionally, government policies and support provided by relevant agencies encourage blockchain adoption in the logistics industry (Montecchi et al., Citation2019). Previous research has consistently shown their significant influence, particularly in the supply chain and logistics sector, as influential factors shaping behavioral intentions to adopt blockchain technologies (Orji et al., Citation2020; Al-Kubaisy & Al-Somali, Citation2023). Understanding the relationship between government support, policy, and behavioral intention to adopt and use blockchain technology in the NQI is crucial. This finding provides insights for policymakers to strategize successful technology adoption (Al-Kubaisy & Al-Somali, Citation2023). Government agencies offer resources, create a conducive policy environment for adoption, and motivate industries to adopt emerging technologies through incentives and regulations (Orji et al., Citation2020). Thus, in line with these facts, we propose the following hypothesis:
H7: Government support and policy (GSP) positively influence the behavioral intention to adopt and use blockchain technology in NQI.
H8: Government support and policy (GSP) positively influence the perceived ease of use of blockchain technology in the NQI.
3.2. The technology acceptance model (TAM)
3.2.1. Perceived ease of use (PEU)
TAM provides a framework for understanding users’ acceptance and adoption of new technologies. In the context of Blockchain Technology Adoption in National Quality Infrastructure (NQI), perceived ease of use (PEU) refers to an individual’s subjective assessment of the system’s effortless nature and user-friendliness (Davis, Citation1989; Nuryyev et al., Citation2020). This reflects the degree to which users perceive the system as intuitive, convenient, and free from complexities or obstacles (Esfahbodi et al., Citation2022; Nuryyev et al., Citation2020; Sohaib et al., Citation2020; Wang et al., Citation2022). Similarly, the Behavioral Intention to Adopt (BIA) represents individuals’ willingness and readiness to use blockchain technology in the NQI (Taylor & Todd, Citation1995).
Numerous studies have consistently demonstrated the positive influence of PEU on users’ intention to adopt and utilize new technologies (Bach et al., Citation2022; Lin & Chang, Citation2011; Nuryyev et al., Citation2020; Sohaib et al., Citation2020; Salahshour Rad et al., Citation2018; Wang et al., Citation2022). These findings highlight the importance of PEU in driving technological adoption. For instance, in the context of small- and medium-sized enterprises (SMEs), a higher PEU directly influences the intention to adopt blockchain technology (Nuryyev et al., Citation2020). Similarly, within the realm of cryptocurrency, PEU has been observed to significantly enhance the behavioral intention to use cryptocurrencies (Sohaib et al., Citation2020). Moreover, in the construction industry, a stronger perception of ease of use of blockchain technology is associated with a higher willingness to adopt it (Wang et al., Citation2022). These empirical findings underscore the critical role of perceived ease of PEU as a determining factor in technology adoption decisions.
Furthermore, the link between PEU and perceived usefulness in technology adoption has been elucidated in previous studies (Abdou & Jasimuddin, Citation2020; Almuraqab et al., Citation2021; Bach et al., Citation2022; Esfahbodi et al., Citation2022; Grover et al., Citation2019; Huang & Liao, Citation2015). These investigations have identified positive associations between PEU and perceived usefulness, for instance, in the context of cryptocurrency payments (Nuryyev et al., Citation2020) and the impact of ease of use on usefulness in distributed ledger technology (Rehman et al., Citation2022). Notably, the findings indicate that the easier the use of blockchain technology, the more users perceive it to be useful (Wang et al., Citation2022). Comprehending the influence of PEU on perceived usefulness is crucial for understanding users’ acceptance behavior, particularly in the adoption of emerging technologies such as blockchain (Esfahbodi et al., Citation2022). Examining this relationship provides valuable insights into user adoption behaviors and decision-making processes. This facilitates the identification of factors contributing to users’ perceptions of ease of use and usefulness, thereby shedding light on adoption patterns and potential barriers associated with blockchain technology. Consequently, considering the outcomes derived from previous studies and the reasoning provided above, we present the following hypotheses:
H9: Perceived ease of use (PEU) significantly influences the behavioral intention to use blockchain technology in the NQI.
H10: Perceived ease of use (PEU) significantly influences the perceived usefulness of blockchain technology in NQI.
3.2.2. Perceived usefulness (PU)
Perceived usefulness (PU) refers to the belief that utilizing a specific technology will enhance job performance (Davis, Citation1989; Esfahbodi et al., Citation2022; Nuryyev et al., Citation2020; Rehman et al., Citation2022; Shi & Yan, Citation2016; Sohaib et al., Citation2020; Sternad Zabukovšek et al., Citation2019). In this study, PU represents users’ perceptions of how adopting blockchain technology can improve their work outcomes within the NQI context. Previous research has consistently demonstrated the positive impact of PU on intention to adopt blockchain technology across various domains. This has been supported by previous studies (Nuryyev et al., Citation2020; Rehman et al., Citation2022; Shi & Yan, Citation2016; Sternad Zabukovšek et al., Citation2019). Notably, PU plays a significant role in the intention to adopt blockchain technology in e-commerce (Esfahbodi et al., Citation2022) and in the domain of SMEs, which positively influences the intention to adopt cryptocurrency payments (Nuryyev et al., Citation2020). Individuals who perceive technology as more useful are more likely to express their intention to use it (Sohaib et al., Citation2020). Furthermore, the perceived usefulness of Enterprise Resource Planning (ERP) systems has been found to positively influence users’ attitudes towards their usage (Sternad Zabukovšek et al., Citation2019). PU has also been identified as a crucial factor in the intention to adopt various technologies within specific areas. For instance, research has highlighted its positive impact on consumers’ intention to adopt blockchain acceptance (Knauer & Mann, Citation2020). Moreover, studies have consistently shown a positive influence of PU on the intention to adopt fitness mobile apps (Huang & Ren, Citation2020) and smart wearable devices (Park, Citation2020). These consistent findings across diverse technological domains underscore the significance of PU in shaping users’ intentions to adopt innovative technologies, including blockchains. Thus, considering the findings of earlier research and the aforementioned arguments, we formulate the following hypothesis:
H11: Perceived usefulness significantly influences the behavioral intention to use blockchain technology in the NQI.
4. Methodology
4.1. Research methodology
4.1.1. Sample size and sampling technique
A combination of sampling techniques was used in this study to determine the minimum required sample size (Priyanath et al., Citation2020; Tilahun et al., Citation2023). The ‘R-square minimum technique’ considered factors such as the minimum R-squared value, the maximum number of arrows pointing to a construct, and the chosen significance level. With a minimum R-squared value of 0.25 and a significance level of 0.05, the minimum sample size was determined using () to achieve 80% predictive power (Priyanath et al., Citation2020). This resulted in a minimum sample size of 75 participants. Additionally, the ‘10 times rule’ suggested a minimum sample size of 70 participants, which is ten times the number of constructs in the conceptual research model. Notably, the actual sample size in this study exceeded the minimum requirements, with a total of 178 participants included (Wong et al., Citation2020; Hair et al., Citation2014).
4.1.2. Data collection: Instrument and process
The data collection instrument used in this empirical study was a 7-point Likert-scale questionnaire with closed-response questions (Malik et al., Citation2021; Wang et al., Citation2022). It was adapted from validated measurement scales used in similar research to ensure appropriate construct coverage and benefit from the established reliability and validity. The questionnaire underwent peer review, pilot testing, and necessary adjustments to enhance its clarity and eliminate redundancy.
Purposive sampling, a non-probability sampling method, was used in this study (Kim, Citation2022). The self-administered primary data collection involved individuals with varying levels of responsibility, diverse educational backgrounds, and work experience from NQI, government, manufacturing, banking, finance, universities, and research institutions. Participants were selected based on their information technology literacy, understanding of blockchain technology, and NQI, with additional information provided as necessary. Moreover, Participants were informed that completion and submission of the questionnaire would serve as an indication of their voluntary consent to participate in the study. Verbal consent was obtained from each participant prior to their involvement in the study. The consent process involved providing participants with detailed information about the study objectives, procedures, the voluntary nature of participation, and the assurance of confidentiality. Furthermore, the study was approved and received ethical clearance from the Institutional Review Board (IRB) of Addis Ababa Institute of Technology (AAiT), Addis Ababa University. This approval signifies that the study adheres to the ethical standards outlined in the 1964 Declaration of Helsinki and its subsequent amendments.
A total of 194 survey questionnaires were initially obtained from the target population, and 16 incomplete responses were excluded from the data analysis. Thus, the final sample size for the subsequent analysis was 178.
4.1.3. Measurement of constructs
The research model consists of seven factors (): technology compatibility, relative advantages, top management support, government support and policy, perceived usefulness, perceived ease of use, and behavioral intention to adopt blockchain technology. To ensure content validity, all measurement items were adapted from scales previously validated in other studies. Each item was assessed on a seven-point Likert scale ranging from ‘very strongly disagree’ to ‘very strongly agree’. By leveraging established measurement instruments and modifying them appropriately for the current study context, we aimed to develop a questionnaire that not only captured the key constructs, but also benefited from prior validation, strengthening the validity and reliability of the findings.
Table 2. The constructs, the sources, the measurement items and the resulting loading, composite reliability, average variance extracted and the Cronbach’s Alpha.
4.2. Demographic profile of respondents
This study included 178 participants in the demographic distribution analysis. Regarding sex, 75.8% of the participants were male, while 24.2% were female. In terms of age, 6.2% of the participants were under 25 years old, 52.8% were between 25 and 35 years old, 37.1% were between 36 and 45 years old, and only 3.9% were above 45 years old. Moreover, when considering the highest level of education, 7.3% of participants had completed college or below, 44.9% had an undergraduate degree, 43.8% had a master’s degree, and 3.9% held a PhD. In terms of the industry or institution with which they were affiliated, 28.1% were associated with NQI institutions, 17.4% with government and public administration, 23.0% with manufacturing and production, 2.8% with banking and finance, and 28.7% with universities and research institutions. Regarding years of experience, 28.1% of participants had 2–5 years of experience, 34.3% had 6–10 years of experience, 23.6% had 11–15 years of experience, and 14.0% had more than 15 years of experience. Lastly, in terms of management positions, 56.7% of the participants held non-managerial positions, 24.2% were entry-level managers, 10.1% were middle-level managers, and 9.0% were senior-level managers.
5. Dual-stage analysis and results
5.1. Preliminary analysis
5.1.1. Kolmogorov-Smirnov (K-S), ANOVA and multicollinearity tests
We conducted a preliminary analysis of our conceptual research model by employing three statistical tests. One-sample K-S test for normality of distribution was conducted on various variables, in which all variables had an asymp. sig. value of 0.000, indicating that the observed distributions deviated significantly from the normal distribution. The ANOVA tests, , revealed highly significant linear relationships (p < 0.001) between the variables in all cases analyzed. The evidence suggests strong and statistically significant linear associations, highlighting the importance of considering linearity when exploring relationships among variables. Furthermore, for testing for multicollinearity, upon the PLS-SEM algorithm run, SmartPLS 4 (Ringle et al., Citation2022) yielded all Variance Inflation Factor (VIF) less than 10, ranging from 1.215 to 1.686 for the inner model, and from 1.380 to 2.555 for the outer model, indicating no significant multicollinearity issues among the variables (Hew & Syed Abdul Kadir, Citation2016; Rehman et al., Citation2022; Wong et al., Citation2020).
5.1.2. Common method bias (CMB)
To address common method bias in PLS-SEM, the VIF was assessed prior to testing the structural model to identify constructs with high correlation. The results indicated that the highest VIF value was 2.555 (), which is below the threshold of 5.00 (Ullah et al., Citation2021), and is even less than the more conservative value suggested by (Kock & Lynn, Citation2012), which is 3.3. Furthermore, Harman’s single-factor analysis was conducted. The results revealed that a single factor accounted for only 35.2% of the total variance in our analysis, which is below the required threshold of 50% (Dang Quan et al., Citation2024; Nguyen et al., Citation2023; Podsakoff et al., Citation2003). This indicates that common method bias is unlikely to be a significant issue in our study.
Table 3. Fornell-Lacker’s criterion (above diagonal), Heterotrait-Monotrait ratio (below diagonal).
5.2. Stage-1: Structural equation model analysis
5.2.1. Assessment of the measurement model
The assessment of the measurement model in the study indicates strong convergence validity, with constructs demonstrating good internal consistency and capturing a substantial amount of variance in their indicators (). The reliability is supported by composite reliability values ranging from 0.784 to 0.897 and Cronbach’s alpha values ranging from 0.783 to 0.891 (Hew et al., Citation2018; Leong et al., Citation2020). Additionally, average variance extracted (AVE) values ranging from 0.664 to 0.754 confirm the reliability and effectiveness of the measurements (Hair et al., Citation2017; Leong et al., Citation2018; Nordman & Tolstoy, Citation2016; Rehman et al., Citation2022). Furthermore, discriminant validity is established, , through correlations lower than the square root of AVE values and HTMT values, below 0.9. This confirms the distinctiveness of the constructs, as supported by Fornell-Lacker’s criterion and the HTMT ratio (Fornell & Larcker, Citation1981; Henseler et al., Citation2016; Latif et al., Citation2020). Moreover, the factor loadings () of all the items in the model have a value greater than the acceptable value of 0.70 (Hair et al., Citation2010; Esposito et al., Citation2010).
5.2.2. Structural model analysis
Path Analysis: In the initial stage, hypothesis testing was carried out through PLS-SEM bootstrapping on SmartPLS 4 (Ringle et al., Citation2022) on 5000 subsamples with a two-tailed test of 0.05 significance level. The results of the path analysis reveal important insights into the relationships between the constructs with a 63.64% significant path percentage, as shown in the path analysis () and the total effects (). depicts the structural model, showcasing the T statistics for the outer model and the t-values for the inner model. Accordingly, the path from Technology Compatibility (TC) to Behavioral Intention to Apply (BIA) shows a significant positive relationship (β = 0.307, p < 0.000), supporting H1. However, the path from TC to Perceived Usefulness (PU) is not significant (β = –0.015, p = 0.841), indicating that TC does not significantly influence PU. On the other hand, the path from Relative Advantage (RA) to PU demonstrates a strong positive relationship (β = 0.478, p < 0.000), supporting H4. The path from RA to BIA is not significant (β = –0.011, p = 0.915), suggesting no significant impact of Relative Advantage on Behavioral Intentions to Apply and use blockchain technology. The paths from Top Management Support (TMS) to BIA (β = 0.281, p = 0.000) and TMS to Perceived Ease of Use (PEU) (β = 0.313, p = 0.000) support hypotheses H5 and H6, respectively. Similarly, the path from Government Support and Policy (GSP) to PEU is significant (β = 0.346, p = 0.000), supporting Hypothesis H8, but the path from GSP to BIA is not significant (β = 0.002, p = 0.980). The paths from PU to BIA (β = 0.293, p = 0.003) and PEU to PU (β = 0.233, p = 0.008) are significant, supporting hypotheses H11 and H10, respectively; however, the path from PEU to BIA (β = 0.096, p = 0.295) is not significant. These results provide valuable insights into the relationships between the constructs, allowing for a better understanding of the factors influencing behavioral intention and perceived usefulness in the context of the study.
Table 4. Path analysis.
5.2.2.1. Predictive power, predictive relevance and effect size (f2)
demonstrates the strong predictive power of the independent variables for the constructs. The R2 values indicate that 48.2%, 32.3%, and 34.7% of the variance in BIA, PEU, and PU, respectively, can be explained by the independent variables of the model. These percentages (>10%) suggest a substantive and satisfactory predictive power (Wong et al., Citation2020; Eom et al., Citation2006). Q2 values (>0) indicate moderate predictive relevance, indicating the model’s ability to predict constructs beyond the estimation sample (Latif et al., Citation2020). The findings highlight the effectiveness of the independent variables in predicting the dependent variables and the model’s accuracy in out-of-sample situations. The effect size (f2) values () revealed moderate effects, such as TC and BIA (0.128), GSP and PEU (0.136), and RA and PU (0.288), while other relationships showed weaker effects (BIA and GSP: 0.005) and no effects in GSP and BIA and three other relations, illustrating varying magnitudes of construct relationships (Hair et al., Citation2010).
Table 5. Predictive power (R2) and predictive relevance (Q2).
Table 6. Effect size f2.
5.3. Stage-2: Artificial neural network analysis
5.3.1. Artificial neural networks (ANN)
Artificial Neural Networks (ANN) are powerful modelling techniques that resemble the human brain and offer exceptional performance enhancements through training (Hew et al., Citation2018; Talukder et al., 2020). ANNs establish connections between the input and output data using artificial neurons in hidden layers (), enabling prediction without relying on a theoretical model. This approach revolutionizes data analysis owing to its efficiency and robustness (Leong et al., Citation2019).
Figure 3. Architecture of a typical Neural Network [Source: Sternad Zabukovšek et al., Citation2019].
![Figure 3. Architecture of a typical Neural Network [Source: Sternad Zabukovšek et al., Citation2019].](/cms/asset/3b11efdb-2074-4584-8d65-6b65f1c79c91/oaen_a_2369220_f0003_c.jpg)
In this study, to enhance the learning depth, we incorporated two hidden layers into the deep learning ANN architecture (Gillis, Citation2023; Mahdavifar & Ghorbani, Citation2019; Rehman et al., Citation2022). The ANN model utilizes a multilayer perceptron (MLP) with nonlinear mapping (Liébana-Cabanillas et al., Citation2017; Sohaib et al., Citation2020; Sternad Zabukovšek et al., Citation2019; Uluskan, Citation2023). SPSS 23 with ten-fold cross-validation was used to mitigate overfitting (Wong et al., Citation2020; Leong et al., Citation2020; Ooi & Tan, Citation2016). The activation functions were a hyperbolic tangent for the hidden layer and an identity for the output layer. The number of neurons was generated automatically (Sternad Zabukovšek et al., Citation2019; Uluskan, Citation2023).
The loading scores obtained from the SEM stage served as inputs for the ANN analysis. The data were split, with 90% allocated for training the neural network model and the remaining 10% for testing the prediction accuracy (Lee et al., Citation2020). Significant paths from the PLS-SEM analysis formed three models (Model 1, Model 2, and Model 3), with PU, PEU, and BIA as endogenous constructs (, , and ). The results () show variations in performance and variable importance across models. The model evaluation used metrics such as the Sum of Squared Errors (SSE) and Root Mean Square Error (RMSE). The mean SSE and RMSE provided average performance measures, whereas the standard deviations indicated variability among the models. The RMSE was computed using EquationEquation (1)(1)
(1) based on the SSE values from the ANN analysis (Lee et al., Citation2020; Sohaib et al., Citation2020).
(1)
(1)
The Normalized Variable Relative Importance section shows the relative importance of the input and output variables in each model. The models exhibited low SSE and RMSE values, indicating a good performance. For example, in Model 3, the mean SSE was 1.959 (SD = 0.255) for the training data, and the mean RMSE was 0.109 (SD = 0.007). These findings underscore the models’ accuracy and consistency in capturing the underlying patterns and relationships (Leong et al., Citation2020; Uluskan, Citation2023).
5.3.2. Sensitivity analysis
The sensitivity analysis of the ANN models () revealed the relative importance of constructs in predicting endogenous constructs related to BCT application in the NQI (Sohaib et al., Citation2020; Sternad Zabukovšek et al., Citation2019). In Model 1, PU as an endogenous construct, RA, and PEU played significant roles with average importance values of 0.631 and 0.369, respectively. RA demonstrated a strong influence, whereas PEU had a moderate impact. Model 2, with PEU as the endogenous construct, highlighted TMS (0.351) and GSP (0.649) as influential factors, indicating their respective significance levels. Model 3, focusing on BIA as the endogenous construct, identified PU (0.303), TC (0.374), and TMS (0.345) as crucial constructs, with varying levels of impact.
Table 7. Sensitivity analysis.
5.4. Comparative analysis
The study found that The ANN models exhibited low SSE and RMSE values, indicating good performance in predicting the endogenous constructs of PU, PEU, and BIA. Sensitivity analysis revealed the relative importance of constructs, with RA and PEU playing significant roles in predicting PU, and TC and TMS were important in predicting BIA.
The comparison between the ANN and SEM models () shows similarities and differences in their representations of variable relationships (Foo et al., Citation2018; Sohaib et al., Citation2020). Both models highlight the importance of input variables, such as PEU, RA, TMS, GSP, and TC, although their rankings and relative importance may differ. Generally, the models agreed on the significant influence of these variables on the outputs, with some discrepancies in the PU and TMS ranks in the third model.
Table 8. Comparison of PLS-SEM results with ANN results.
The observed discrepancies between the ANN and SEM models, especially in Model 3 with three independent variables, can be attributed to increased complexity. The ANN model captures complex nonlinear relationships, but sacrifices interpretability (Foo et al., Citation2018; Oparaji et al., Citation2017; Sohaib et al., Citation2020). On the other hand, SEM provides a structured framework and a better understanding of theoretical constructs but may struggle with complex interactions. These discrepancies reflect different approaches and trade-offs between interpretability and predictive accuracy (Dziugaite et al., Citation2020; Deng et al., Citation2018; Linardatos et al., Citation2020).
Employing a two-stage approach with SEM and an ANN offers several advantages. PLS-SEM establishes a theoretical foundation, tests hypotheses, and validates a model. It provides insights into variable relationships and the overall fit. In the subsequent stage, the use of loading scores from SEM as ANN inputs leveraged the ANN's ability to capture nonlinear relationships and make accurate predictions. This approach combines the interpretability and theoretical grounding of SEM with the flexibility and predictive power of ANN, enhancing data comprehension, uncovering hidden patterns, potentially improving prediction accuracy, and bringing rigor to the analysis (Elareshi et al., Citation2022; Leong et al., Citation2020; Rehman et al., Citation2022; Wong et al., Citation2020).
6. Discussion
The findings of this study have several broader implications for the adoption of blockchain technology in the context of national quality infrastructure. Firstly, the positive impact of Technological Compatibility (TC) on Behavioral Intention to Apply (BIA), supporting H1, validates the notion that when the technology aligns well with existing systems and processes, individuals are more likely to adopt it. This finding is consistent with previous studies (Kumar Bhardwaj et al., Citation2021; Ullah et al., Citation2021; Chittipaka et al., Citation2023), highlighting the importance of considering compatibility factors when implementing blockchain technology. However, the non-significant relationship between TC and Perceived Usefulness (PU) contradicts previous research (Ullah et al., Citation2021; Wang et al., Citation2022). This suggests that while compatibility may influence individuals’ intention to apply blockchain, it may not directly impact their perception of its usefulness. Nonetheless, the Artificial Neural Network (ANN) analysis identified TC as a key factor, further emphasizing its significance in the adoption of blockchain technology. Furthermore, the strong positive relationship between Relative Advantage (RA) and PU, supporting H3, reinforces the idea that individuals perceive blockchain as more useful when they recognize its relative advantages over existing systems. This finding aligns with previous studies (Kumar Bhardwaj et al., Citation2021), underscoring the importance of highlighting the benefits and advantages of blockchain to potential adopters. However, the non-significant influence of RA on BIA differs from other studies (Kumar Bhardwaj et al., Citation2021; Wong et al., Citation2020). This suggests that while individuals may recognize the advantages of blockchain, it may not necessarily translate into their intention to adopt it. Nevertheless, the higher normalized relative importance of RA in the ANN Model-1 indicates that it still plays a crucial role in shaping individuals’ perception of usefulness of the technology.
The significant paths from Top Management Support (TMS) to BIA and Perceived Ease of Use (PEU), supporting H5 and H6, respectively, confirm the crucial role of top management in promoting adoption. This aligns with previous study (Kumar Bhardwaj et al., Citation2021) and highlights the significance of leadership support in driving the acceptance and implementation of blockchain technology. However, it is worth noting that the normalized relative importance of TMS in the ANN Model-2 was relatively lower compared to other variables, suggesting that its importance may vary depending on the analytical approach used.
Furthermore, the significant path from Government Support and Policy (GSP) to PEU validates the idea that government initiatives and policies can influence individuals’ perception of how easy it is to use blockchain technology. However, the non-significant path from GSP to BIA does not support H8, consistent with some previous studies (Kumar Bhardwaj et al., Citation2021; Ullah et al., Citation2021). This suggests that while government support and policies may impact individuals’ perception of ease of use, they may not directly translate into their intention to adopt the technology. Further investigation is warranted to better understand the direct impact of government support on adoption intentions.
The significant path from PEU to PU, supporting H10, corroborates the idea that individuals perceive blockchain technology as more useful when they find it easy to use. This finding is consistent with some previous studies (Esfahbodi et al., Citation2022; Nuryyev et al., Citation2020; Rehman et al., Citation2022; Sciarelli et al., Citation2022; Ullah et al., Citation2021; Wang et al., Citation2022), and emphasizes the importance of considering ease of use as a factor that can influence individuals’ perception of usefulness. However, it is worth noting that this finding contradicts other studies (Sternad Zabukovšek et al., Citation2019; Shrestha & Vassileva, Citation2019), suggesting that the relationship between ease of use and perceived usefulness may be more nuanced and context-dependent. Thus, further investigation is needed to better understand the direct impact of ease of use on perceived usefulness. Additionally, the non-significant path from PEU to BIA, not supporting H11, aligns with some previous research (Esfahbodi et al., Citation2022; Kumar Bhardwaj et al., Citation2021; Shrestha & Vassileva, Citation2019), indicating that while individuals may perceive blockchain as easy to use, it may not directly translate into their intention to adopt the technology. However, this finding contrasts with other studies (Nuryyev et al., Citation2020; Sohaib et al., Citation2020; Wang et al., Citation2022), suggesting that the relationship between ease of use and adoption intentions may vary across different contexts or sample populations, necessitating further research to explore the direct impact of ease of use on adoption intentions. Furthermore, the significant paths from PU to BIA, supporting H9, substantiates the idea that individuals are more likely to adopt blockchain technology when they perceive it as useful. This finding aligns with several previous studies (Esfahbodi et al., Citation2022; Nuryyev et al., Citation2020; Rehman et al., Citation2022; Sciarelli et al., Citation2022; Sohaib et al., Citation2020; Sternad Zabukovšek et al., Citation2019), highlighting the importance of emphasizing the benefits and advantages of blockchain to potential adopters. However, there are a few exceptions (Shrestha & Vassileva, Citation2019), suggesting that the relationship between perceived usefulness and adoption intentions may not be universally consistent. The high normalized relative importance of perceived usefulness as an exogenous construct in Model-3 indicates its moderate influence on adoption intentions, further emphasizing its significance.
Research Question 1 (RQ1) aimed to determine the key factors that directly influence the adoption of blockchain technology in the context of the National Quality Infrastructure, with a particular focus on the Ethiopian context. The analysis conducted in this study identifies Perceived Usefulness, Technology Compatibility, and Top Management Support as the key factors that directly influence the adoption of blockchain technology in the National Quality Infrastructure. Understanding the importance of these factors can assist policymakers, organizations, and stakeholders in the Ethiopian National Quality Infrastructure in designing strategies and interventions that promote the successful adoption and implementation of blockchain technology. By emphasizing the usefulness of the technology, ensuring compatibility with existing systems, and garnering top management support, organizations can enhance the likelihood of successful blockchain adoption and leverage its potential benefits in the context of the National Quality Infrastructure.
Research Question 2 (RQ2) aimed to determine which of the key factors identified in the study has a greater association with intentions to adopt and use blockchain technology in the Ethiopian National Quality Infrastructure (NQI). Upon comparing the significance levels of the identified direct influencers of behavioral intentions to adopt blockchain technology in the Ethiopian NQI, it was found that Technological Compatibility (TC) exhibited a stronger association with intentions to adopt and use blockchain technology. Moreover, the comparative analysis of the Partial Least Squares-Structural Equation Modeling (PLS-SEM) and Artificial Neural Network (ANN) results consistently found Technological Compatibility to be the most influencing factor. This convergence of findings from two different analytical approaches provides robust support for the significance of Technological Compatibility in shaping adoption intentions in the context of the Ethiopian NQI. These results underscore the importance of considering technological compatibility as a key factor when promoting the adoption of blockchain technology in the Ethiopian NQI. By ensuring that the technology aligns well with the existing infrastructure and processes, organizations can enhance the likelihood of adoption and reduce barriers associated with compatibility issues.
6.1. Theoretical implications
The use of the TAM-TOE framework in assessing the adoption of blockchain technology within Ethiopia’s NQI, along with the application of dual-stage analysis using PLS-SEM and ANN, has important theoretical implications. Firstly, the adoption of the TAM-TOE framework in this study demonstrates its relevance and applicability in understanding the factors influencing the adoption of blockchain technology within the NQI context. By incorporating elements of technology acceptance, organizational characteristics, and external factors, the framework provides a comprehensive lens through which to analyze the adoption process. This theoretical implication suggests that the TAM-TOE framework can serve as a valuable tool for researchers and practitioners in studying and promoting the adoption of emerging technologies, such as blockchain, in similar contexts. Secondly, the use of dual-stage analysis, combining PLS-SEM and ANN, showcases the advantages of employing multiple analytical techniques to gain a deeper understanding of the adoption phenomenon. PLS-SEM enables the examination of complex relationships and latent constructs, while ANN offers the ability to model nonlinear relationships and capture intricate patterns in the data. Indeed, the literature on use of PLS-SEM and ANN integrated approach are applied in such domains as adoption of Metaverse Banking Service (Nguyen et al., Citation2023), Adoption of blockchain in operations and SCM (Wong et al., Citation2020), quality of university cafeteria services (Uluskan, Citation2023). The integration of these two techniques allows for a more robust analysis and provides a comprehensive perspective on the factors influencing blockchain adoption within the NQI. This theoretical implication highlights the importance of employing diverse analytical approaches to enhance the understanding of complex adoption processes, such as the NQIs.
Furthermore, the application of PLS-SEM and ANN in this study contributes to the advancement of methodological approaches in adoption research. PLS-SEM, as a variance-based structural equation modeling technique, offers flexibility and efficiency in handling small sample sizes and complex models (Abu Afifa et al., Citation2022; Nuryyev et al., Citation2020; Wang et al., Citation2022). ANN, on the other hand, provides a powerful tool for capturing and modeling nonlinear relationships and patterns in large datasets (Nguyen et al., Citation2023; Uluskan, Citation2023; Wong et al., Citation2020). The combination of these techniques showcases the importance of methodological pluralism in adoption research and encourages researchers to leverage various analytical approaches to gain a comprehensive understanding of adoption phenomena, especially in the NQI sector.
6.2. Practical implications
The findings of this study have significant practical implications for policymakers and organizations within Ethiopia’s National Quality Infrastructure (NQI) concerning the adoption of blockchain technology. Firstly, policymakers should recognize the influence of factors such as perceived usefulness, technological compatibility, and top management support on blockchain adoption within the NQI. These factors should be given priority when formulating strategies and initiatives to promote adoption.
Addressing technological compatibility challenges is crucial based on the research findings. Policymakers should prioritize efforts to align blockchain technology with existing systems and processes, facilitating seamless integration and minimizing compatibility barriers. Comprehensive assessments of the current infrastructure will help identify areas where blockchain technology can be effectively integrated. Moreover, policymakers should emphasize the relative advantages of blockchain technology compared to existing systems within the NQI. By highlighting its benefits, such as increased efficiency, transparency, and security, policymakers can shape individuals’ perception of the technology’s usefulness and encourage adoption.
Additionally, cultivating a supportive organizational culture that fosters top management support is vital. Active involvement and endorsement from top management play a pivotal role in facilitating blockchain adoption. Policymakers should create an empowering environment that enables top management to champion blockchain adoption within the NQI. This can be achieved through the provision of necessary resources and training, as well as promoting a culture that values and supports blockchain initiatives.
7. Conclusions, limitations and future research avenue
This study fills a significant literature gap by examining the adoption of blockchain technology in the Ethiopian National Quality Infrastructure (NQI). By integrating the TAM and TOE frameworks, it comprehensively analyzes the factors influencing blockchain adoption at both individual and organizational levels. The findings validate the importance of technological compatibility, top management support and policy, and perceived usefulness as primary drivers of blockchain adoption in the NQI, shaping stakeholders’ intentions. The study emphasizes the need to align existing infrastructure with blockchain systems for successful implementation. The two-stage PLS-SEM and ANN analyses validate prior relationships and provide new insights, strengthening the understanding of blockchain adoption in the NQI context. These findings have practical implications for researchers, policymakers, and practitioners involved in blockchain adoption. The study contributes to the existing literature by highlighting the interplay between technological, organizational, and environmental factors in adoption decisions within the NQI.
7.1. Limitations and future research agenda
Although some achievements have been made, the study still has the following shortcomings: Firstly, the TAM-TOE framework relies on self-reported measures and subjective perceptions, which can introduce biases and potential measurement errors. To tackle this, future research endeavors could focus on employing mixed-methods approaches that combine qualitative and quantitative data can provide a more comprehensive understanding of the factors influencing technology adoption. Secondly, the current study is focused on a specific period, providing limited insights into the dynamics and changes in adoption patterns over time. Consequently, conducting longitudinal studies can help overcome this limitation by tracking the adoption of blockchain technology within the NQI over an extended period. Furthermore, the current study lacked comparative analyses that examine the contextual factors influencing blockchain adoption. By comparing the adoption of blockchain technology in the NQI with other sectors or industries in Ethiopia or other countries, researchers can identify sector-specific challenges, opportunities, and best practices.
Authors’ contribution
Ayele Legesse: substantially contributions and designed the study, collected, and analysed data, and wrote the initial draft of the manuscript and enhanced the final draft of the manuscript. Dr. Birhanu Beshah: Provided expertise in statistical analysis, critically reviewed and edited the manuscript and final approval of the manuscript to be published. Dr. Ing. Eshetie Berhan: Provided expertise in statistical analysis, assisted in data interpretation, and critically reviewed and edited the manuscript and final approval of the manuscript to be published. Dr. Ermias Tesfaye: Provided expertise in statistical analysis, contributed to critically review the manuscript for intellectual content and enhanced the final draft of the manuscript.
Disclosure statement
No potential conflict of interest was reported by the author(s).
Data availability statement
The data supporting the findings of this study are available from the corresponding author upon reasonable request.
Additional information
Notes on contributors
Ayele Legesse
Ayele Legesse a PhD student in Industrial Engineering at Addis Ababa University, holds an MSc in Mechanical Engineering. He has lectured at Haramaya University and coordinated several programs there. His research interests include blockchain technology, national quality infrastructure, assembly line balancing, and workplace safety.
Birhanu Beshah
Birhanu Beshah an Associate Professor of Industrial Engineering at Addis Ababa University, has served as CEO of Ethiopian Railways Corporation and leads the African Railway Education and Research Center. He has over 50 publications and his research interests include quality management, logistics, and emerging technologies like AI, Industry 4.0 and Blockchain.
Eshetie Berhan
Eshetie Berhan an Associate Professor of Industrial Engineering with a PhD in Industrial and Mechanical Engineering, has published over 115 research papers. His expertise includes manufacturing, supply chain management, AI, and organizational studies. He has held leadership roles at Addis Ababa Institute of Technology, contributing to various engineering and socio-economic projects.
Ermias Tesfaye
Ermias Tesfaye an Assistant Professor of Industrial Engineering at Addis Ababa University, holds a PhD in Mechanical Engineering. He has published 7 research papers and specializes in operational excellence and lean manufacturing. He also serves as a consultant and previously held the position of Associate Director for Post Graduate Program.
References
- Abdou, D., & Jasimuddin, S. M. (2020). The use of the UTAUT model in the adoption of E-learning technologies: An empirical study in France based banks. Journal of Global Information Management, 28(4), 38–51. https://doi.org/10.4018/JGIM.2020100103
- Abu Afifa, M. M., Vo Van, H., & Le Hoang Van, T. (2022). Blockchain adoption in accounting by an extended UTAUT model: Empirical evidence from an emerging economy. Journal of Financial Reporting and Accounting, 21(1), 5–44. https://doi.org/10.1108/JFRA-12-2021-0434
- Abu-Akel, S. A., & Ibrahim, M. (2023). The effect of relative advantage, top management support and IT infrastructure on E-Filing adoption. Journal of Risk and Financial Management, 16(6), 295. https://doi.org/10.3390/jrfm16060295
- Albrecht, S., Strüker, J., Reichert, S., Neumann, D., Schmid, J., & Fridgen, G. (2018). Dynamics of Blockchain implementation-A case study from the energy sector. Retrieved from http://hdl.handle.net/10125/50334
- Albshaier, L., Almarri, S., & Hafizur Rahman, M. M. (2024). A review of Blockchain’s role in E-Commerce transactions: Open challenges, and future research directions. Computers, 13(1), 27. https://doi.org/10.3390/computers13010027
- Alkhateeb, F. M., Khanfar, N. M., & Loudon, D. (2009). Physicians’ adoption of pharmaceutical E-Detailing: Application of Rogers’ innovation-diffusion model. Services Marketing Quarterly, 31(1), 116–132. https://doi.org/10.1080/15332960903408575
- Al-Kubaisy, Z. M., & Al-Somali, S. A. (2023). Factors influencing blockchain technologies adoption in supply chain management and logistic sectors: Cultural compatibility of blockchain solutions as moderator. Systems, 11(12), 574. https://doi.org/10.3390/systems11120574
- Almuraqab, N. A. S., Jasimuddin, S. M., & Mansoor, W. (2021). An Empirical Study of Perception of the End-User on the Acceptance of Smart Government Service in the UAE. Journal of Global Information Management, 29(6), 1–29. https://doi.org/10.4018/JGIM.20211101.oa11
- Al-Rawy, M., & Elci, A. (2019). A design for blockchain-based digital voting system. Springer International Publishing. https://doi.org/10.1007/978-3-030-02351-5
- Al-Saqaf, W., & Seidler, N. (2017). Blockchain technology for social impact: Opportunities and challenges ahead. Journal of Cyber Policy, 2(3), 338–354. https://doi.org/10.1080/23738871.2017.1400084
- Al-Saqaf, W., & Seidler, N. (2020). The factors effecing E-Filing adoption among Jordanian Firms: The moderating role of trust. PalArch s Journal of Archaeology of Egypt/Egyptology, 17(6), 17–31.
- Attaran, M., & Gunasekaran, A. (2019). Applications of blockchain technology in business challenges and opportunities. Springery.
- Awa, H. O., Ojiabo, O. U., & Emecheta, B. C. (2015). Integrating TAM, TPB and TOE frameworks and expanding their characteristic constructs for e-commerce adoption by SMEs. Journal of Science & Technology Policy Management, 6(1), 76–94. https://doi.org/10.1108/JSTPM-04-2014-0012
- Bach, M. P., Zoroja, J., & Čeljo, A. (2022). An extension of the technology acceptance model for business intelligence systems: Project management maturity perspective. International Journal of Information Systems and Project Management, 5(2), 5–21. https://doi.org/10.12821/ijispm050201
- Behnke, K., & Janssen, M. F. W. H. A. (2019). Boundary conditions for traceability in food supply chains using blockchain technology. International Journal of Information Management. https://doi.org/10.1016/j.ijinfomgt.2019.05.025
- Beshah, B., Gidey, E., & Leta, A. (2017). National cost of quality in Ethiopian import–export. Total Quality Management and Business Excellence, 28(1-2), 118–129. https://doi.org/10.1080/14783363.2015.1050177
- Cermeño, J. S. (2016). Blockchain in financial services: Regulatory landscape and future challenges for its commercial application. BBVA Research.
- Chittipaka, V., Kumar, S., Sivarajah, U., Bowden, J. L. H., & Baral, M. M. (2023). Blockchain Technology for Supply Chains operating in emerging markets: An empirical examination of technology-organization-environment (TOE) framework. Annals of Operations Research, 327(1), 465–492. https://doi.org/10.1007/s10479-022-04801-5
- Chivu, R. G., Popa, I. C., Orzan, M. C., Marinescu, C., Florescu, M. S., & Orzan, A. O. (2022). The role of blockchain technologies in the sustainable development of students’ learning process. Sustainability, 14(3), 1406. https://doi.org/10.3390/su14031406
- Choudhury, O., Fairoza, N., Sylla, I., & Das, A. (2019). A blockchain framework for managing and monitoring data in multi-site clinical trials. ArXiv, abs/1902.03975.
- Clohessy, T., & Acton, T. (2019). Investigating the influence of organizational factors on blockchain adoption: An innovation theory perspective. Industrial Management & Data Systems, 119(7), 1457–1491. https://doi.org/10.1108/IMDS-08-2018-0365
- Dang Quan, T., Wei-Han Tan, G., Aw, E. C. X., Cham, T. H., Basu, S., & Ooi, K. B. (2024). Can you resist the virtual temptations? Unveiling impulsive buying in metaverse retail. Asia Pacific Journal of Marketing and Logistics, 2024, 911. https://doi.org/10.1108/APJML-09-2023-0911
- Davis, F. D. (1989). Perceived usefuness, perceived ease of use and user acceptance of information technology. MIS Quarterly, 13(3), 319. https://doi.org/10.2307/249008
- Deng, L., Yang, M., & Marcoulides, K. M. (2018). Structural equation modeling with many variables: A systematic review of issues and developments. Frontiers in Psychology, 9(APR), 580. https://doi.org/10.3389/fpsyg.2018.00580
- Díaz, R. M., Valdés Figueroa, L., & Pérez, G. (2021). Blockchain implementation opportunities and challenges in the Latin American and Caribbean logistics sector. FAL Bulletin, No. 387. Economic Commission for Latin America and the Caribbean (ECLAC). Santiago, Chile.
- Dutta, P., Choi, T. M., Somani, S., & Butala, R. (2020). Blockchain technology in supply chain operations: Applications, challenges and research opportunities. Transportation Research. Part E, Logistics and Transportation Review, 142, 102067. https://doi.org/10.1016/j.tre.2020.102067
- Dziugaite, G. K., Ben-David, S., & Roy, D. M. (2020). Enforcing Interpretability and its Statistical Impacts: Trade-offs between Accuracy and Interpretability. pp. 1–12. http://arxiv.org/abs/2010.13764
- Elareshi, M., Habes, M., Youssef, E., Salloum, S. A., Alfaisal, R., & Ziani, A. (2022). SEM-ANN-based approach to understanding students’ academic-performance adoption of YouTube for learning during Covid. Heliyon, 8(4), e09236. https://doi.org/10.1016/j.heliyon.2022.e09236
- Eom, N., Wen, S. B., & Ashill, H. J. (2006). The determinants of students’ perceived learning outcomes and satisfaction in university online education: An empirical investigation. Decision Sciences Journal of Innovative Education, 4(2), 215–235. https://doi.org/10.1111/j.1540-4609.2006.00114.x
- Esfahbodi, A., Pang, G., & Peng, L. (2022). Determinants of consumers’ adoption intention for blockchain technology in E-commerce. Journal of Digital Economy, 1(2), 89–101. https://doi.org/10.1016/j.jdec.2022.11.001
- Esposito V., Chin, W. W., Henseler, J., & Wang, H. (Eds.). (2010). Handbook of partial least squares. Springer Berlin Heidelberg. https://doi.org/10.1007/978-3-540-32827-8
- Foo, P.-Y., Lee, V.-H., Tan, G. W.-H., & Ooi, K.-B. (2018). A gateway to realising sustainability performance via green supply chain management practices: A PLS–ANN approach. Expert Systems with Applications, 107, 1–14. https://doi.org/10.1016/j.eswa.2018.04.013
- Fornell, C., & Larcker, D. F. (1981). Evaluating structural equation models with unobservable variables and measurement error. Journal of Marketing Research, 18(1), 39–50. https://doi.org/10.2307/3151312
- Gillis, A. S. (2023). Deep Learning. TechTarget. Retrieved October 21, 2023, from https://www.techtarget.com/searchenterpriseai/definition/deep-learning-deep-neural-network.
- Grewal, D., Motyka, S., & Levy, M. (2018). The evolution and future of retailing and retailing education. Journal of Marketing Education, 40(1), 85–93.
- Grover, P., Kar, A. K., Janssen, M., & Ilavarasan, P. V. (2019). Perceived usefulness, ease of use and user acceptance of blockchain technology for digital transactions–insights from user-generated content on Twitter. Enterprise information system, 13(6), 771–800. https://doi.org/10.1080/17517575.2019.1599446
- Guan, W., Ding, W., Zhang, B., & Verny, J. (2023). The role of supply chain alignment in coping with resource dependency in blockchain adoption: Empirical evidence from China. Journal of Enterprise Information Management, 36(2), 605–628. https://doi.org/10.1108/JEIM-11-2021-0491
- Hair, R., Black, J. F., Babin, W. C., Anderson, B. J., & Tatham, R. E. (2010). Multivariate Data Analysis. Pearson.
- Hair, M., Hult, J. F., Ringle, G. T. M., & Sarstedt, C. M. (2017). A Primer on Partial Least Squares Structural Equation Modeling (PLS-SEM) (2nd ed.). Sage Publications Inc.
- Hair, J., Jr, Sarstedt, M., Hopkins, L., & Kuppelwieser, V. (2014). Partial least squares structural equation modeling (PLS-SEM). European Business Review, 26(2), 106–121. https://doi.org/10.1108/EBR-10-2013-0128
- Haneem, F., Kama, N., Taskin, N., Pauleen, D., & Abu Bakar, N. A. (2019). Determinants of master data management adoption by local government organizations: An empirical study. International Journal of Information Management, 45, 25–43. https://doi.org/10.1016/j.ijinfomgt.2018.10.007
- Hardgrave, B. C., Davis, F. D., & Riemenschneider, C. K. (2003). Investigating determinants of software developers’ intentions to follow methodologies. Journal of Management Information Systems, 20(1), 123–151. https://doi.org/10.1080/07421222.2003.11045751
- Henseler, J., Hubona, G., & Ray, P. A. (2016). Using PLS path modeling in new technology research: updated guidelines. Industrial Management & Data Systems, 116(1), 2–20. https://doi.org/10.1108/IMDS-09-2015-0382
- Hervey, A. (2023). Blockchain is A new model that makes the existing model obsolete. Retrieved October 25, 2023, from medium.com. Blockchain is A new model that makes the existing model obsolete.
- Hew, J.-J., Leong, L.-Y., Tan, G. W.-H., Lee, V.-H., & Ooi, K.-B. (2018). Mobile social tourism shopping: A dual-stage analysis of a multi-mediation model. Tour Manag, 66, 121–139. https://doi.org/10.1016/j.tourman.2017.10.005
- Hew, T.-S., & Syed Abdul Kadir, S. L. (2016). Predicting instructional effectiveness of cloud-based virtual learning environment. Industrial Management & Data Systems, 116(8), 1557–1584. https://doi.org/10.1108/IMDS-11-2015-0475
- Huang, T. L., & Liao, S. (2015). A model of acceptance of augmented-reality interactive technology: the moderating role of cognitive innovativeness. Electronic Commerce Research, 15(2), 269–295. https://doi.org/10.1007/s10660-014-9163-2
- Huang, G., & Ren, Y. (2020). Linking technological functions of fitness mobile apps with continuance usage among Chinese users: Moderating role of exercise self-efficacy. Computers in Human Behavior, 103, 151–160. https://doi.org/10.1016/j.chb.2019.09.013
- Kabra, N., Bhattacharya, P., Tanwar, S., & Tyagi, S. (2020). MudraChain : Blockchain-based framework for automated cheque clearance in financial institutions. Future Generation Computer Systems, 102, 574–587. https://doi.org/10.1016/j.future.2019.08.035
- Kapoor, K. K., Dwivedi, Y. K., & Williams, M. D. (2015). Examining the role of three sets of innovation attributes for determining adoption of the interbank mobile payment service. Information Systems Frontiers, 17(5), 1039–1056. https://doi.org/10.1007/s10796-014-9484-7
- Karjaluoto, H., & Vaccaro, V. L. (2009). B2B green marketing and innovation theory for competitive advantage. Journal of Systems and Information Technology, 11(4), 315–330. https://doi.org/10.1108/13287260911002477
- Kim, A. (2023). How blockchain is providing sustainable coffee. Ledger insight. Retrieved February 15, 2023, from https://www.ledgerinsights.com/how-blockchain-is-providing-sustainable-coffee/.
- Kim, K. S. (2022). Methodology of non-probability sampling in survey research. American Journal of Biomedical Science & Research, 15(6), 616–618. https://doi.org/10.34297/AJBSR.2022.15.002166
- Knauer, F., & Mann, A. (2020). What is in it for me? Identifying drivers of blockchain acceptance among german consumers. The Journal of the British Blockchain Association, 3(1), 1–16. Jan https://doi.org/10.31585/jbba-3-1-(1)2020
- Kock, N., & Lynn, G. (2012). Lateral Collinearity and misleading results in variance-based SEM: An illustration and recommendations. Journal of the Association for Information Systems, 13(7), 546–580. https://doi.org/10.17705/1jais.00302
- Koh, L., Dolgui, A., & Sarkis, J. (2020). Blockchain in transport and logistics–paradigms and transitions. International Journal of Production Research, 58(7), 2054–2062. https://doi.org/10.1080/00207543.2020.1736428
- Kouhizadeh, M., Saberi, S., & Sarkis, J. (2021). Blockchain technology and the sustainable supply chain: Theoretically exploring adoption barriers. International Journal of Production Economics, 231, 107831. https://doi.org/10.1016/j.ijpe.2020.107831
- Kulkarni, M., & Patil, K. (2020). Block chain technology adoption using TOE framework. International Journal of Scientific & Technology Research, 9(02), 1109–1117.
- Kumar Bhardwaj, A., Garg, A., & Gajpal, Y. (2021). Determinants of blockchain technology adoption in supply chains by Small and Medium Enterprises (SMEs) in India. Mathematical Problems in Engineering, 2021, 1–14. https://doi.org/10.1155/2021/5537395
- Latif, K. F., Sajjad, A., Bashir, R., Shaukat, M. B., Khan, M. B., & Sahibzada, U. F. (2020). Revisiting the relationship between corporate social responsibility and organizational performance: The mediating role of team outcomes. Corporate Social Responsibility and Environmental Management, 27(4), 1630–1641. https://doi.org/10.1002/csr.1911
- Lee, V.-H., Hew, J.-J., Leong, L.-Y., Tan, G. W.-H., & Ooi, K.-B. (2020). Wearable payment: A deep learning-based dual-stage SEM-ANN analysis. Expert Systems with Applications, 157, 113477. https://doi.org/10.1016/j.eswa.2020.113477
- Lee, S. G., Trimi, S., & Kim, C. (2013). The impact of cultural differences on technology adoption. Journal of World Business, 48(1), 20–29. https://doi.org/10.1016/j.jwb.2012.06.003
- Leong, L.-Y., Hew, T.-S., Ooi, K.-B., Lee, V.-H., & Hew, J.-J. (2019). A hybrid SEM-neural network analysis of social media addiction. Expert Systems with Applications, 133, 296–316. https://doi.org/10.1016/j.eswa.2019.05.024
- Leong, L. Y., Hew, T. S., Ooi, K. B., & Wei, J. (2020). Predicting mobile wallet resistance: A two-staged structural equation modeling-artificial neural network approach. International Journal of Information Management, 51(vember), 102047. https://doi.org/10.1016/j.ijinfomgt.2019.102047
- Leong, L.-Y., Jaafar, N. I., & Ainin, S. (2018). The effects of Facebook browsing and usage intensity on impulse purchase in f-commerce. Comput Human Behav, 78, 160–173. https://doi.org/10.1016/j.chb.2017.09.033
- Li, J., Ma, Q., Chan, A. H., & Man, S. S. (2019). Health monitoring through wearable technologies for older adults: Smart wearables acceptance model. Applied Ergonomics, 75, 162–169. https://doi.org/10.1016/j.apergo.2018.10.006
- Liébana-Cabanillas, F., Marinković, V., & Kalinić, Z. (2017). A SEM-neural network approach for predicting antecedents of m-commerce acceptance. International Journal of Information Management, 37(2), 14–24. https://doi.org/10.1016/j.ijinfomgt.2016.10.008
- Lin, J. S. C., & Chang, H. C. (2011). The role of technology readiness in self-service technology acceptance. Managing Service Quality, 21(4), 424–444. https://doi.org/10.1108/09604521111146289
- Linardatos, P., Papastefanopoulos, V., & Kotsiantis, S. (2020). Explainable AI: A review of machine learning interpretability methods. Entropy, 23(1), 18. https://doi.org/10.3390/e23010018
- Mahdavifar, S., & Ghorbani, A. A. (2019). Application of deep learning to cybersecurity: A survey. Neurocomputing, 347, 149–176. https://doi.org/10.1016/j.neucom.2019.02.056
- Malik, S., Chadhar, M., Vatanasakdakul, S., & Chetty, M. (2021). Factors affecting the organizational adoption of blockchain technology: Extending the technology–organization– environment (TOE) framework in the Australian context. Sustainability, 13(16), 9404. https://doi.org/10.3390/su13169404
- Manoj, T., Makkithaya, K., & Narendra, V. G. (2022). A Blockchain based decentralized identifiers for entity authentication in electronic health records. Cogent Engineering, 9(1), 134. https://doi.org/10.1080/23311916.2022.2035134
- Marikyan, D., Papagiannidis, S., Rana, O. F., & Ranjan, R. (2022). Blockchain adoption: A study of cognitive factors underpinning decision making. Comput Human Behav, 131(October 2021), 107207. https://doi.org/10.1016/j.chb.2022.107207
- Marsal-Llacuna, M.-L. (2018). Future living framework: Is blockchain the next enabling network? Technol. Forecast. Soc. Change, 128, 226–234.
- Miličević, K., Omrčen, L., Kohler, M., & Lukić, I. (2022). Trust model concept for IoT Blockchain applications as part of the digital transformation of metrology. Sensors, 22(13), 4708. https://doi.org/10.3390/s22134708
- Minoli, D., & Minoli, D. (2019). Positioning of blockchain mechanisms in IoT-powered Smart Home Systems : A gateway-based approach. Internet of Things, 10, 100147. https://doi.org/10.1016/j.iot.2019.100147
- Montecchi, M., Plangger, K., & Etter, M. May (2019). It’s real, trust me! Establishing supply chain provenance using blockchain. Business Horizons, 62(3), 283–293. https://doi.org/10.1016/j.bushor.2019.01.008
- Na, S., Heo, S., Han, S., Shin, Y., & Roh, Y. (2022). Acceptance Model of Artificial Intelligence (AI)-based technologies in construction firms: Applying the Technology Acceptance Model (TAM) in Combination with the Technology–Organisation–Environment (TOE) Framework. Buildings, 12(2), 90. https://doi.org/10.3390/buildings12020090
- Nakamoto, S. (2018). Bitcoin: A peer-to-peer electronic cash system. bitcoin.org. Retrieved March 28, 2018, from https://bitcoin.org/bitcoin.pdf%0A.
- Namahoot, K. S., & Rattanawiboonsom, V. (2022). Integration of TAM Model of consumers’ intention to adopt cryptocurrency platform in Thailand: The mediating role of attitude and perceived risk. Human Behavior and Emerging Technologies, 2022, 98. https://doi.org/10.1155/2022/9642998
- Nasongkhla, J., & Shieh, C. J. (2023). Using technology acceptance model to discuss factors in university employees’ behavior intention to apply social media. Online Journal of Communication and Media Technologies, 13(2), e202317. https://doi.org/10.30935/ojcmt/13019
- Nguyen, L. T., Duc, D. T. V., Dang, T. Q., & Nguyen, D. P. (2023). Metaverse banking service: Are we ready to adopt? A deep learning-based dual-stage SEM-ANN analysis. Human Behavior and Emerging Technologies, 2023, 371. https://doi.org/10.1155/2023/6617371
- Nguyen, T. H., Le, X. C., & Vu, T. H. L. (2022). An Extended Technology-Organization-Environment (TOE) Framework for online retailing utilization in digital transformation: Empirical evidence from Vietnam. Journal of Open Innovation, 8(4), 200. https://doi.org/10.3390/joitmc8040200
- Nokhbeh Zaeem, R., & Barber, K. S. (2020). How much identity management with blockchain would have saved us? A longitudinal study of identity Theft. Lecture Notes in Business Information Processing, 158–168. https://doi.org/10.1007/978-3-030-61146-0_13
- Nordman, E. R., & Tolstoy, D. (2016). The impact of opportunity connectedness on innovation in SMEs’ foreign-market relationships. Technovation, 57–58, 47–57. https://doi.org/10.1016/j.technovation.2016.04.001
- Nuryyev, G., Wang, Y.-P., Achyldurdyyeva, J., Jaw, B.-S., Yeh, Y.-S., Lin, H.-T., & Wu, L.-F. (2020). Blockchain technology adoption behavior and sustainability of the business in tourism and hospitality SMEs: An empirical study. Sustainability, 12(3), 1256. https://doi.org/10.3390/su12031256
- Oke, A., Walumbwa, F., Yan, T., Idiagbon-Oke, M., & A. Ojode, L. (2014). Linking economic status with technology adoption in three emerging economies of Sub-Saharan Africa. Journal of Manufacturing Technology Management, 25(1), 49–68. https://doi.org/10.1108/JMTM-02-2012-0013
- Ooi, K.-B., & Tan, G. W.-H. (2016). Mobile technology acceptance model: An investigation using mobile users to explore smartphone credit card. Expert Systems with Applications, 59, 33–46. Oct https://doi.org/10.1016/j.eswa.2016.04.015
- Oparaji, U., Sheu, R.-J., Bankhead, M., Austin, J., & Patelli, E. (2017). Robust artificial neural network for reliability and sensitivity analyses of complex non-linear systems. Neural Networks, 96, 80–90. https://doi.org/10.1016/j.neunet.2017.09.003
- Orji, I. J., Kusi-Sarpong, S., Huang, S., & Vazquez-Brust, D. (2020). Evaluating the factors that influence blockchain adoption in the freight logistics industry. Transp Res E Logist Transp Rev, 141, 102025. https://doi.org/10.1016/j.tre.2020.102025
- Park, E. (2020). User acceptance of smart wearable devices: An expectation-confirmation model approach. Telematics and Informatics, 47, 101318. https://doi.org/10.1016/j.tele.2019.101318
- Podsakoff, P. M., MacKenzie, S. B., Lee, J. Y., & Podsakoff, N. P. (2003). Common method biases in behavioral research: A critical review of the literature and recommended remedies. Journal of Applied Psychology, 88(5), 879–903. https://doi.org/10.1037/0021-9010.88.5.879
- Pranav, S., Singh, K., Nandi, R., & Kumar, S. (2019). Managing smart home appliances with proof of authority and blockchain (vol. 2). Springer International Publishing. https://doi.org/10.1007/978-3-030-22482-0
- Premkumar, G., & Roberts, M. (1999). Adoption of new information technologies in rural small businesses. Omega, 27(4), 467–484. https://doi.org/10.1016/s0305-0483(98)00071-1
- Priyanath, H. M. S., Rvspk, R., & Rgn, M. (2020). Methods and rule-of-thumbs in the determination of minimum sample size when appling structural equation modelling: A review. Journal of Social Science Research, 15, 102–107. https://doi.org/10.24297/jssr.v15i.8670
- Puklavec, B., Oliveira, T., & Popovič, A. (2018). Understanding the determinants of business intelligence system adoption stages an empirical study of SMEs. Industrial Management & Data Systems, 118(1), 236–261. https://doi.org/10.1108/IMDS-05-2017-0170
- Queiroz, M. M., Telles, R., & Bonilla, S. H. (2020). Blockchain and supply chain management integration: A systematic review of the literature. Supply Chain Management, 25(2), 241–254. https://doi.org/10.1108/SCM-03-2018-0143
- Rehman, I. H., Ahmad, A., Akhter, F., & Aljarallah, A. (2022). A dual-stage SEM-ANN analysis to explore consumer adoption of smart wearable healthcare devices. Journal of Global Information Management, 29(6), 1–30. https://doi.org/10.4018/JGIM.294123
- Ringle, J.-M., Christian, M., & Wende., S. (2022). SmartPLS Release: 4. SmartPLS GmbH, Oststeinbek, Germany. Retrieved from https://www.smartpls.com.
- Rogers, E. M. (1983). Diffusion of innovations. Free Press.
- Runde, D. F. (2017). Quality Infrastructure: Ensuring sustainable economic growth. Center for Strategic and International Studies (CSIS). (January 2017), 1–6. http://www.jstor.org/stable/resrep23247%0A.
- Salahshour Rad, M., Nilashi, M., & Mohamed Dahlan, H. (2018). Information technology adoption: A review of the literature and classification. Universal Access in the Information Society, 17(2), 361–390. https://doi.org/10.1007/s10209-017-0534-z
- Schmidthuber, L., Maresch, D., & Ginner, M. (2020). Disruptive technologies and abundance in the service sector – Toward a refined technology acceptance model. Technological Forecasting and Social Change, 155, 119328. https://doi.org/10.1016/j.techfore.2018.06.017
- Sciarelli, M., Prisco, A., Gheith, M. H., & Muto, V. (2022). Factors affecting the adoption of blockchain technology in innovative Italian companies: An extended TAM approach. Journal of Strategy and Management, 15(3), 495–507. https://doi.org/10.1108/JSMA-02-2021-0054
- Shi, P., & Yan, B. (2016). Factors affecting RFID adoption in the agricultural product distribution industry: Empirical evidence from China. Springerplus, 5(1), 2029. https://doi.org/10.1186/s40064-016-3708-x
- Shrestha, A. K., & Vassileva, J. (2019). User acceptance of Usable Blockchain-based research data sharing system: An extended TAM Based Study. 2019 First IEEE International Conference on Trust, Privacy and Security in Intelligent Systems and Applications (TPS-ISA), 203–208.
- Sohaib, O., Hussain, W., Asif, M., Ahmad, M., & Mazzara, M. (2020). A PLS-SEM Neural network approach for understanding cryptocurrency adoption. IEEE Access, 8, 13138–13150. https://doi.org/10.1109/ACCESS.2019.2960083
- Soomro, B. A., Shah, N., & Abdelwahed, N. A. A. (2022). Intention to adopt cryptocurrency: A robust contribution of trust and the theory of planned behavior. Journal of Economic and Administrative Sciences, 40(2), 419–433. https://doi.org/10.1108/JEAS-10-2021-0204
- Sternad Zabukovšek, S., Kalinic, Z., Bobek, S., & Tominc, P. (2019). SEM–ANN based research of factors’ impact on extended use of ERP systems. Central European Journal of Operations Research, 27(3), 703–735. https://doi.org/10.1007/s10100-018-0592-1
- Sun, Z., Xu, Q., & Liu, J. (2023). Dynamic supervision of counterfeit products based on blockchain technology: A differential game on goodwill accumulation. PLoS One, 18(10), e0293346. https://doi.org/10.1371/journal.pone.0293346
- Taherdoost, H. (2022). A critical review of blockchain acceptance models – Blockchain Technology adoption frameworks and applications. Computers, 11, 24. https://doi.org/10.3390/computers11020024
- Taherdoost, H. (2023). Blockchain and machine learning: A critical review on security. Information, 14(5), 295. https://doi.org/10.3390/info14050295
- Talukder, M. S., Sorwar, G., Bao, Y., Ahmed, J. U., & Palash, M. A. S. (2020). Predicting antecedents of wearable healthcare technology acceptance by elderly: A combined SEM-Neural Network approach. Technol Forecast Soc Change, 150, 119793. https://doi.org/10.1016/j.techfore.2019.119793
- Taylor, S., & Todd, P. A. (1995). Understanding information technology usage: A test of competing models. Information Systems Research, 6(2), 144–176. https://doi.org/10.1287/isre.6.2.144
- The World Bank Ethiopia. (2016). The World Bank Ethiopia: National Quality Infrastructure Development Project (P160279).
- Tilahun, M., Berhan, E., & Tesfaye, G. (2023). Determinants of consumers’ purchase intention on digital business model platform: Evidence from Ethiopia using partial least square structural equation model (PLS-SEM) technique. Journal of Innovation and Entrepreneurship, 12(1), 23. https://doi.org/10.1186/s13731-023-00323-x
- Tsai, W., Deng, E., Ding, X., & Li, J. (2018). Application of Blockchain to trade clearing. In 2018 IEEE International Conference on Software Quality, Reliability and Security Companion (QRS-C), pp. 154–163. https://doi.org/10.1109/QRS-C.2018.00039
- Ullah, N., Al-Rahmi, W. M., Alzahrani, A. I., Alfarraj, O., & Alblehai, F. M. (2021). Blockchain technology adoption in smart learning environments. Sustainability, 13(4), 1801. https://doi.org/10.3390/su13041801
- Uluskan, M. (2023). Structural equation modelling – Artificial neural network based hybrid approach for assessing quality of university cafeteria services. The TQM Journal, 35(4), 1048–1071. https://doi.org/10.1108/TQM-01-2022-0001
- Vionis, P., & Kotsilieris, T. (2023). The potential of blockchain technology and smart contracts in the energy sector: A review. Applied Sciences, 14(1), 253. https://doi.org/10.3390/app14010253
- Wamba, S. F., & Queiroz, M. M. (2022). Industry 4.0 and the supply chain digitalisation: A blockchain diffusion perspective. Production Planning and Control, 33(2-3), 193–210. https://doi.org/10.1080/09537287.2020.1810756
- Wang, X., Liu, L., Liu, J., & Huang, X. (2022). Understanding the Determinants of Blockchain Technology Adoption in the Construction Industry. Buildings, 12(10), 1709. https://doi.org/10.3390/buildings12101709
- Wong, L. W., Leong, L. Y., Hew, J. J., Tan, G. W. H., & Ooi, K. B. (2020). Time to seize the digital evolution: Adoption of blockchain in operations and supply chain management among Malaysian SMEs. International Journal of Information Management, 52(March), 101997. https://doi.org/10.1016/j.ijinfomgt.2019.08.005
- Wong, L. W., Tan, G. W. H., Lee, V. H., Ooi, K. B., & Sohal, A. (2020). Unearthing the determinants of Blockchain adoption in supply chain management. International Journal of Production Research, 58(7), 2100–2123. https://doi.org/10.1080/00207543.2020.1730463
- Wu, B., & Chen, X. (2017). Continuance intention to use MOOCs: Integrating the technology acceptance model (TAM) and task technology fit (TTF) model. Computers in Human Behavior, 67, 221–232. https://doi.org/10.1016/j.chb.2016.10.028
- Wu, F., Mahajan, V., & Balasubramanian, S. (2003). An analysis of E-Business adoption and its impact on business performance. Journal of the Academy of Marketing Science, 31(4), 425–447. https://doi.org/10.1177/0092070303255379
- Yadav, V. S., Singh, A. R., Raut, R. D., & Govindarajan, U. H. (2020). Blockchain technology adoption barriers in the Indian agricultural supply chain: An integrated approach. Resources, Conservation and Recycling, 161, 104877. https://doi.org/10.1016/j.resconrec.2020.104877
- Yeoh, W., Lee, A. S. H., Ng, C., Popovic, A., & Han, Y. (2023). Examining the acceptance of blockchain by real estate buyers and sellers. Information Systems Frontiers, 26(3), 1121–1137. https://doi.org/10.1007/s10796-023-10411-8
- Zhang, J., Tan, R., Su, C., & Si, W. (2020). Design and application of a personal credit information sharing platform based on consortium blockchain. Journal of Information Security and Applications, 55(2019), 102659. https://doi.org/10.1016/j.jisa.2020.102659
Appendix A
Table A1. Parameters for selecting minimum sample size (Source: Priyanath et al., 2020).
Table A2. ANOVA test for linearity.
Table A3. Variance Inflation Factor (VIF) for Inner and outer model.
Table A4. Total effect.
Table A5. The resulting RMSE for each model and each network.