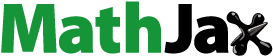
Abstract
Relationship of weather parameters viz., maximum temperature (Tmax, °C), minimum temperature (Tmin, °C), rainfall (RF, mm), morning relative humidity (RH1, %), evening humidity (RH2, %), and sunshine hours (SSH), during seven years at Mandya (Karnataka) was individually explored with peaks of rice yellow stem borer (YSB) Scirpophaga incertulas (Walker) light trap catches. The peaks of YSB trap catches exhibited significant correlation with Tmax of October 3rd week, Tmin of November 1st week, RF of October 2nd week, RH1 of November 4th and RH2 of November 1st week, and SSH of October 4th week. Weather-based prediction model for YSB was developed by regressing peaks of YSB light trap catches on mean values of different weather parameters of aforesaid weeks. Of the weather parameters, only Tmin, RF, and RH1 were found to be relevant through stepwise regression. The model was validated satisfactorily through 8-year independent data on weather parameters and YSB light trap catch peaks (R 2 = 0.90, p < 0.0002).
Public Interest Statement
Insect pests are a major bottleneck in realizing yield potential of the crop. Accurate prediction of insect emergence and development is essential for effective pest management despite being quite challenging. Improperly timed pesticide applications are expensive, harmful to natural enemy populations, and also contaminate the environment. Proper forewarning and monitoring techniques are indispensible in pest management, their absence is only felt when sudden pest outbreaks catch use unaware.
Pest forecasting models facilitate understanding of factors that affect pest population dynamics. These can thus be used for predicting pest outbreaks. In our paper, we developed a forewarning model for yellow stem borer, a serious pest of rice at Mandya, Karnataka, India. This would prove useful in forewarning likely occurrence of the pest in Mandya region, thereby paving way for timely action and prevention of yield losses due to the pest.
1. Introduction
Rice is the world’s most important food crop and is the staple food for 50% of the global population (Barrion, Joshi, Barrion-Dupo, & Sebastian, Citation2007). The crop is attacked by several insect pests from nursery to harvest, which cause severe yield loss in one region of the country or another (Asghar, Suhail, Afzal, & Khan, Citation2009). Yellow stem borer (YSB) Scirpophaga incertulas (Walker) has emerged as one of the most important pests of rice during post green revolution years throughout the country (Alam, Sing, Yadav, & Goel, Citation1992; Bandong & Litsinger, Citation2005; Krishnaiah, Lakshmi, Pasalu, Katti, & Padmavathi, Citation2008). The extent of rice yield losses due to YSB has been estimated as 20–70 % (Catling, Islam, & Pattrasudhi, Citation1987; Chelliah, Benthur, & Prakasa Rao, Citation1989).
Both biotic and abiotic factors are believed to be responsible for pest population dynamics (Singh et al., Citation2009). Climatic factors such as temperature, rainfall, and relative humidity greatly influence the outbreak of the insect population (Chen, Liu, & Zeno, Citation1968; Heong, Manza, Catindig, Villareal, & Jacobsen, Citation2007; Siswanto, Dzolkhifli, & Elna, Citation2008). Population dynamics of YSB like any other species are thus liable to fluctuate according to the dynamic condition of its environment (Chen et al., Citation1968; Khaliq, Javed, Sohail, & Sagheer, Citation2014). Quantification of important mortality factors, both biotic and abiotic is needed to reliably forecast insect populations. Besides, knowledge of the seasonal abundance and population build up trend is essential to ensure timely preparedness to tackle impending pest problems and prevent crop losses (Das et al., Citation2008).
Pest population density may be the resultant of weather parameters of several preceding weeks or months. It thus becomes worthwhile to explore relationship of pest population with weather parameters of several preceding weeks. Several workers have analyzed influence of weather factors on YSB population and observed temperature, humidity, and rainfall to be important ones (Bhatnagar & Saxena, Citation1999; Das et al., Citation2008; Joshi, Ram, & Singh, Citation2009; Mandal, Roy, & Saha, Citation2011; Sharma, Atsedewoin, & Fanta, Citation2011). However, such a study has not been attempted for Mandya region of Karnataka. As pest–weather relations are empirical and location-specific in nature and thus need to be established individually for different regions.
Keeping this in view, present study was undertaken to develop and validate weather-based YSB prediction model for the Cauvery Command areas of Mandya, Karnataka, India.
2. Materials and methods
Data on YSB light trap catches and weather parameters viz., maximum temperature (Tmax) (°C), minimum temperature (Tmin) (°C), rainfall (RF) (mm), morning relative humidity (RH1) (%), evening humidity (RH2) (%), and sunshine hours (SSH) for 15 years across 1991–2005 for Mandya region (76.19′–76.20′ S and 12.13′–13.4′ E) of Karnataka, India was collected from Annual Progress Reports of Directorate of Rice Research (DRR), Hyderabad, India. Weekly weather data and log transformed YSB light trap catches for seven years (N = 7) were used for model formulation. Linear correlation coefficients were computed between the YSB trap catch peaks during different years and weekly mean values of each of the weather parameters individually. The correlation coefficients between YSB light trap catch peaks and weekly values of weather parameters were explored from 1st week of July onwards until attainment of peak YSB catch. Most important week with respect to each of the weather factors could thus be identified and relevant values were used to develop a multiple linear pest–weather model by regression of the YSB trap catch peaks on different weather parameters using SAS statistical software. The model was validated through eight-year independent data (N = 8) set on weather and YSB trap catch peaks. Model performance was evaluated by comparing observed and predicted YSB trap catch peaks.
3. Results and discussion
The YSB trap catch peaks exhibited significant correlation with Tmax of October 3rd week, Tmin of November 1st week, RF of October 2nd week, RH1 and RH2 of November 4th and 1st week, respectively, and SSH of October 4th week (Table ). As peaks of YSB light trap catches during different years occurred between December 1st week and December 4th week, weather parameters only up to November 4th week were considered for developing regression relationship. Pest–weather model between YSB light trap catches and all weather parameters was established as follows (Equation 1, Table ).
Table 1. Correlation coefficient between yearly peaks of YSB light trap catches and weather parameters (prior to peak catches) during seven years at Mandya, Karnataka
Table 2. Different relationships established between YSB light traps catches and meteorological parameters so as to develop a forecasting model for the pest.
Weather parameters explained 97% variability in YSB trap catches (p = 0.0001). However, removal of SSH did not result in an appreciable reduction in coefficient of determination (R 2 = 0.99) rather it increased, thereby indicating insignificant influence of SSH on YSB light trap catches (Equation 2, Table ). Likewise, exclusion of RH2 did not cause any reduction in R 2 (0.98) suggesting insignificant role of RH2 on YSB light trap catches (Equation 3, Table ). However, exclusion of RH1 caused a significant reduction in R 2 (0.64), suggesting significant role of RH1 on YSB light trap catches (Equation 4, Table ).
Further, rainfall was excluded and model with Tmax, Tmin, and RH1 could account for only 51% variability in YSB trap catches, thereby suggesting significant role of rainfall (Equation 5, Table ). With the removal of Tmin, the model with Tmax, rainfall, and RH1 explained only 60% variability in YSB light trap catches, thus suggesting significant influence of Tmin on YSB light trap catches (Equation 6, Table ). However, Tmax removal did not result in much reduction in R
2 thus indicating Tmax to be insignificant in YSB light trap catches (Equation 7, Table ). Out of different weather parameters, only Tmin, RF, and RH1were thus revealed to be important with respect to their influence on YSB light trap catch peaks. These three factors could explain 92% variability in YSB light trap catches (p = 0.0310). Final model with Tmin, rainfall, and RH1was thus established as follows (Equation 7, Table ) and its parameters are shown in Table .
Table 3. Multiple linear regression between yearly peaks of YSB light trap catches and weekly mean values of weather factors (Final model) during seven years at Mandya, Karnataka
The pest–weather model was validated satisfactorily (R 2 = 0.90; p = 0.0001) with independent eight-year data on weather and YSB trap catches (Figure ).
Pest–weather model clearly suggested the Tmin, RF, and RH1 to be important weather parameters that influenced YSB light trap catches at Mandya, Karnataka. Besides, satisfactory validation of the model (R 2 = 0.90, p = 0.0001) with 8-year data endorsed importance of these parameters in affecting YSB population dynamics. On the other hand, Tmax, RH2, and SSH were not found to influence the YSB light trap catches. The Tmin, RF, and RH1 exhibited negative relationship with YSB light trap catches. Indirectly, YSB light trap catches were indicative of its population level on rice crop in the region. The YSB has been designated as a resident pest as it hibernates in rice stubbles in the same field and previous season’s pest density has influence on ensuing season’s density. It thus follows that after initial emergence of YSB adults; interfield migration is important for its population build up and spread in an area. Insects being cold-blooded animals, temperature plays a crucial role in their development and distribution (Bale et al., Citation2002; Kinoshita & Yagi, Citation1930), and YSB is no exception to this thermal principle. Significant influence of Tmin on YSB light trap catches is understandable because moth movement and population build in a particular area takes place during night. Cooler nights seemed to have favored YSB movement.
Rainfall negatively affected YSB light trap catches directly as well indirectly through relative humidity. Heavy rainfall might adversely affect YSB population through physical destruction of its development stages, while high humidity might promote entomopathogens.
Similar results on interactive effect of rainfall, relative humidity, and mean minimum temperature on rice stem borer were also reported (Abraham, Thomas, Karunakaran, & Gopala Krishnan, Citation1972; Bhatnagar & Saxena, Citation1999). Rehman, Inayatullah, and Majid (Citation2002) found minimum temperature, rainfall, and relative humidity to be important factors that influenced the YSB outbreaks. As reliable forewarning and monitoring tools are indispensible for pest management, the YSB prediction model would prove useful in forewarning likely occurrence of the pest in Mandya region, thereby paving way for timely action and prevention of yield losses due to the pest.
Cover image
Adult, Yellow stem borer of rice crop.
Source: Dr L. Vijay kumar.
Acknowledgment
Authors are grateful to Dr. Mallikarjun Jeer for his help to undertake this study. We also thank the Directorate of Rice Research, Hyderabad, India for having used the light trap catches and meteorological data from their Annual Progress Reports.
Additional information
Funding
Notes on contributors
N.R. Prasannakumar
N.R. Prasannakumar obtained his PhD in Entomology from Indian Agricultural Research Institute (IARI), New Delhi. He is currently working as a scientist in Entomology at IARI, Regional station, Katrain (Kullu Valley), Himachal Pradesh, India. He is presently engaged in research on development of decision support systems for pest management and climate change impacts on insect pests. His contributions are as below: Developed spectral signatures and spectral indices for rice brown plant hopper with remote sensing techniques, Assessed impact of effect of elevated CO2 on rice BPH in open top chambers (OTCs), Developed forewarning thumb rule for rice BPH for Mandya region, Karnataka, India, Documented the wild pollinators in broccoli, and studied the importance of wild pollinators in broccoli pollination and seed setting.
References
- Abraham, C. C. , Thomas, B. , Karunakaran, K. , & Gopala Krishnan, R. (1972). Effect of planting season and associated weather conditions on the incidence of rice stem borer Tryporyza incertulas (Walkar). Agricultural Research Journal of Kerala , 10 , 141–151.
- Alam, N. , Sing, R. , Yadav, R. P. , & Goel, S. C. (1992). Population dynamics of borer complex in deep water rice. Bioecology and control of insect pests. In Proceedings of the National Symposium on growth development and control Technology of Insect pests (pp. 95–99). Muzaffarnagar, India..
- Asghar, M. , Suhail, A. , Afzal, M. , & Khan, M. A. (2009). Determination of economic threshold levels for the stem borers (Scirpophaga sp.) and leaf folder (Cnaphalocrosis medinalis) of rice (Oryza sativa) in the Kallar tract of Punjab, Pakistan. International Journal of Agricultural Biology , 11 , 717–720.
- Bale, J. S. , Masters, G. J. , Hodkinson, I. D. , Awmack, C. , Bezemer, T. M. , Brown, V. K. , … Whittaker, J. B. (2002). Herbivory in global climate change research: Direct effects of rising temperature on insect herbivores. Global Change Biology , 8 (1), 1–16.10.1046/j.1365-2486.2002.00451.x
- Bandong, J. P. , & Litsinger, J. A. (2005). Rice crop stage susceptibility to the rice yellow stemborer Scirpophaga incertulas (Walker) (Lepidoptera: Pyralidae). International Journal of Pest Management , 51 , 37–43.10.1080/09670870400028276
- Barrion, A. T. , Joshi, R. C. , Barrion-Dupo, A. L. A. , & Sebastian, L. S. (2007). Systematics of the Philippine rice black bug, Scotinophara stål (Hemiptera: Pentatomidae). In R. C. Joshi , A. T. Barrion , & L. S. Sebastian (Eds.), Rice black bugs: Taxonomy, ecology & management of invasive species (p. 3). Manila: Philippine Rice Research Institute.
- Bhatnagar, A. , & Saxena, R. R. (1999). Environmental correlates of population buildup of rice insect pests through light trap catches. Orza , 36 , 241–245.
- Catling, H. D. , Islam, Z. , & Pattrasudhi, R. (1987). Assessing yield losses in deepwater rice due to yellow stem borer Scirpophaga incertulas (Walker) in Bangladesh and Thailand. Crop Protection , 6 , 20–27.10.1016/0261-2194(87)90023-8
- Chelliah, A. , Benthur, J. S. , & Prakasa Rao, P. S. (1989). Approaches to rice management—Achievements and opportunities. Oryza , 26 , 12–26.
- Chen, C. M. , Liu, C. L. , & Zeno, X. Z. (1968). Study on the regularity of outbreaks of the paddy borer in Hunan Province. I. Ecological geographical distribution. Acta Entomologica Sinica , 1 , 115–124 [in Chinese, English translation of original journal 1965, 14(2): 118].
- Das, D. K. , Behera, K. S. , Dhandapani, A. , Trivedi, T. P. , Chona, N. , & Bhandari, P. (2008). Development of forewarning systems of rice pests for their management. In A. Prakash , A. Sasmal , J. Rao , S. N. Tewari , K. S. Behera , S. K. Singh , & V. Nandagopal (Eds.), Rice pest management (pp. 187–200). Cuttack: Applied Zoologist Research Association.
- Heong, K. L. , Manza, A. , Catindig, J. , Villareal, S. , & Jacobsen, T. (2007). Changes in pesticide use and arthropod biodiversity in the IRRI research farm. Outlooks on Pest Management , 18 , 229–233.10.1564/18oct11
- Joshi, G. , Ram, L. , & Singh, R. (2009). Population dynamics of paddy stem borers in relation to biotic and abiotic factors. Annals of Biology , 25 , 47–51.
- Khaliq, A. , Javed, M. , Sohail, M. , & Sagheer, M. (2014). Environmental effects on insects and their population dynamics. Journal of Entomology and Zoology studies , 2 (2), 1–7.
- Kinoshita, S. , & Yagi, N. (1930). Notes on the northern limit of distribution of the paddy stem borer. Nihon Gakuzyutsu Kyokai Hokoku , 6 , 546–548.
- Krishnaiah, N. V. , Lakshmi, V. J. , Pasalu, I. C. , Katti, G. R. , & Padmavathi, C. (2008). Insecticides in rice-IPM, past, present and future (p. 148). Hyderabad: Directorate of Rice Research, ICAR.
- Mandal, P. , Roy, K. , & Saha, G. (2011). Weather based prediction model of Scirpophaga incertulus (Walker). Annals of Plant Protection Sciences , 19 , 20–24.
- Rehman, A. , Inayatullah, C. , & Majid, A. (2002). Descriptive model to predict the outbreaks of yellow rice stem borer, Scirpophaga incertulas . Pakistan Journal of Agricultural Research , 17 , 282–289.
- Sharma, M. K. , Atsedewoin, A. , & Fanta, S. (2011). Forewarning models of the insects of the paddy crop. International Journal of Biodiversity and Conservation , 3 , 367–375.
- Singh, S. P. , Sekhon, B. S. , Brar, J. S. , Dhaliwal, L. K. , & Chahal, S. K. (2009, November 26–27). Effect of weather parameters and plant geometry on sucking pests dynamics in Bt and non Bt cotton. In 4th National Seminar on agro-meteorology-needs approaches and linkages for rural development (pp. 12–13). Hisar, India.
- Siswanto, R. M. , Dzolkhifli, O. , & Elna, K. (2008). Population fluctuation of Helopeltis antonii Signoret on cashew Anacarcium occidentalle L. in Java Indonesia. Pertanika Journal of Tropical Agriculture Science , 31 , 191–196.