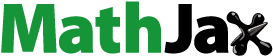
Abstract
This study seeks to estimate technical and resource-use efficiency in the Northern Region. Multistage sampling technique was used to collect cross-sectional data from 126 smallholder rice farmers in Tolon District of Northern Region, Ghana. The stochastic frontier analysis (SFA) was used to analyse the driving factors of rice output and measure the technical efficiency level of farmers while the marginal value product–marginal factor cost (MVP-MFC) approach was used to estimate the efficiency of resource use in rice production. The results from the SFA show that, apart from weedicide, factors such as farm size, quantity of weedicide, and fertiliser used have positive effects on output of rice. However, apart from farm size, factors such as weedicide, fertiliser, and seed were overutilised in production. Technical efficient varied widely among rice farmers, ranging from 11% to 98% with a mean of 75%. Technical inefficient of farmers was influenced by age, extension, household size, years of education, and credit. The study recommends that a farm-level policy directed towards the stimulation of extension work through motivation to give the rural farm households the needed training on farm management to improve productivity.
PUBLIC INTEREST STATEMENT
Rice is one of the most consumed staple in Ghana. However, over the years, even though yield has been increasing, there is still a significant gap between actual yield and potential yield. This demands for measures to increase the efficiency of farmers. A country like Ghana, which has shortage of all factors of production except labour, obviously cannot afford to make an inefficient use of resources. It is therefore important to estimate allocative efficiency of inputs at the farm level and to identify the sources of inefficiency. The study revealed that, apart from farm size, factors such as weedicide, fertiliser, and seed were overutilised in production. As expected, extension services and access to credit played an important role in boosting the efficiency of farmers. Therefore, farm-level policy directed towards the stimulation of extension work through motivation to give the rural farm households the needed training on farm management to improve productivity.
Competing Interests
The authors declare no competing interests.
1. Introduction
The contribution of the agricultural sector to the overall development of Ghana cannot be underscored. Over the years, the agricultural sector which employs the majority of the labour force has contributed significantly to the gross domestic product (GDP) of Ghana. The sector remains key to overall economic growth and development of the country, and it is expected to lead to the growth and structural transformation of the economy. In the year 2017, the agricultural sector contributed 18.3% to GDP as against 25.5% industry sector and 56.2% service sector (Ghana Statistical Service (GSS), Citation2018). Although the agricultural sector’s quota to the GDP of the country is low, the trickle-down effect of this sector on the livelihood of farmers with regard to food security and other gains cannot be underscored.
The crop subsector remains the largest (14.2%) contributor of the agricultural sector to GDP (GSS, Citation2018). Despite such significant role, crop productivity in Ghana has remained low. Growth in agricultural output over the years has come as a result of increase in land under cultivation rather than improvement in yields. Studies in Ghana and other parts of Africa have shown that most farm yields are lower than their achievable yields. For instance, the yields of cereal in Africa are lower than half the world average in which rice is no exception (Macauley & Ramadjita, Citation2015). Moreover, the average yield of rice, maize, sorghum, and soybeans in Ghana is estimated at 3.01 Mt/ha, 2.05 Mt/ha, 1.25 Mt/ha, and 1.70 Mt/ha, respectively, whereas the potential has been estimated to be 6.00 Mt/ha, 5.50 Mt/ha, 2.00 Mt/ha, and 3.00 Mt/ha, respectively [Ministry of Food and Agriculture (MoFA), (Citation2017)]. Input utilisations, socioeconomic factors, management practices, climatic conditions, policy and institutional constraints, biophysical factors, and poor adoption rate of improved technologies were identified as some of the major reasons ascribed to these yield gaps. According to Rockstrom et al. (Citation2003), low yield of crops under rain-fed production is not certain due to low physical potential, but largely to inefficient allocation of inputs.
Rice (Oryza sativa L.) is one of the agronomical cereals grown in the northern part of Ghana. Among all the cereals produced and consumed in Ghana, rice is ranked second after maize (MoFA, Citation2011;ISSER, Citation2014). According to Ministry of Food and Agriculture (MoFA), (Citation2016), the per capita consumption of rice is increasing steadily in the country. This increase is driven by urbanisation and related changes in eating habits and population growth (United State Department of Agriculture (USDA), Citation2014; and Seck, Diagne, Mohanty, & Wopereis, Citation2012). However, for the past years, the country has not produced sufficiently to meet consumers demand. About 55% of rice was imported in 2017 to compensate the demand deficit in the country (MoFA, Citation2017). The inability of farmers to obtain the expected yields could be attributed to the low efficiency level of resource use (Roetter, Van Kuelen, Kuiper, Verhagen, & Van Laar, Citation2008; Donkoh & Awuni, Citation2011). Enhancing farm productivity would help to increase Ghana’s per capita food production and self-sufficiency in rice.
A number of studies have been done on the efficiency of Ghana’s rice industry but with diverse interests. Some of the studies directed their attention towards the adoption of improved rice varieties (see Donkoh & Awuni, Citation2011; Oladele, Kolawole, & Wakatsuki, Citation2011; Wiredu et al., Citation2010; Ragasa et al., Citation2013), whilst others focused on technical efficiency (TE) (Donkoh, Ayambila, & Abdulai, Citation2013; Yiadom-Boakye, Owusu-Sekyere, Nkegbe, & Ohene-Yankyera, Citation2013; Abdulai, Nkegbe, Donkoh, Citation2018; Mabe, Donkoh, & Al-hassan, Citation2018). However, this study extended to estimating the individual productivity of the inputs used in rice production. Resource-use efficiency (RUE) studies on rice production in Ghana is limited but extensively researched in other parts of sub-Saharan Africa. Studies on RUE in other parts of sub-Saharan Africa include (see Nargis & Lee, Citation2013; Onubuogu, Esiobu, Nwosu, & Okereke, Citation2014; Sanusi, Ogungbile, Yakasai, Ahmad, & Daneji, Citation2016; Okoye et al., Citation2016;; Sujan, Islam, Azad, & Rayhan, Citation2017). Besides this, the implementation of 50% subsidy policy on farm inputs through the Planting for Food and Jobs programme in Ghana has increased farmers' access to productive inputs. A study of this kind will provide pertinent information to make comprehensive management decision in resource allocation and reorient the formulation of agricultural policies and institutional improvement.
Allocative efficiency of resource use is critical to enhanced productivity and incomes (Girei, Dire, Yuguda, & Salihu, Citation2014; Ohen, Ene, & Umeze, Citation2014; Onubuogu et al., Citation2014). The major goal of any production system is the attainment of an optimally high level of output with a given amount of effort or input. In the attainment of the optimal level of output, resource productivity is pivotal. An input is said to be efficiently utilised when it is put to the best possible use and at minimum cost. It is necessary to examine whether or not smallholder rice farmers in Ghana are making maximum use of the inputs available to them. Motivated by these arguments, this study seeks to estimate the level of technical and RUE of smallholder rice farmers at the farm level and to identify the determinants of TE in production.
2. Literature review
The aim of a firm in production is to maximise output and minimise cost. In order to achieve this goal in production, all various components of efficiency must be assured. The conventional notion of efficiency can be associated with the work of Farrell (Citation1957) who indicated that the various components of efficiency include TE, allocative efficiency, and economic efficiency. The concept of TE relates to the question of whether a firm uses the best available technology in its production process. TE means producing the maximum amount of output with the incorporation of minimum possible amount of inputs. Technical inefficiency arises when less than the maximum output is obtained from a given package of factors. It is generally assumed to reflect inefficiency due to timing and method of application of production inputs. Allocative efficiency deals with the extent to which farmers make efficient decisions by using inputs up to a level where their marginal contribution to production value is equal to the factor cost (Rukuni, Citation1994). Allocative efficiency is evaluated from the producer’s maximisation point of view. Allocative or price inefficiency arises when factors are used in proportions which do not minimise the cost of producing a given level of output. However, technical and allocative efficiencies are the main components of economic efficiency.
According to Battese (Citation1992) and Zhu (Citation2003), parametric and non-parametric approaches are the two core ways of estimating the efficiency of a production firm. However, the parametric is characterised into two core components, namely, the stochastic frontier models and deterministic frontier models. The data envelope analysis (DEA) is the most common non-parametric analytical approach. The parametric method of estimation involves the econometric modelling of the production frontier. The parametric accounts for measurement errors in both output and stochastic elements by decomposing the effects of noise from the inefficiency effects. Unlike the parametric, the non-parametric method has the ability to measure the efficiency of multi-output cases and requires no functional form to specify the relationship between inputs and outputs (Battese & Broca, Citation1997; Coelli, Rao, O’Donnell, & Battese, Citation2005).
Korir, Serem, Sulo, and Kipsat (Citation2013) examined the determinants of Bambara groundnut production in Western Kenya using the stochastic frontier analysis (SFA) and found out that farmers' farm size, amount of labour used, and quantity of seeds were the major factors influencing Bambara groundnut production in the study area. The empirical results also indicated that, on the average, groundnut farms in the study area could increase their output by 62% using the same input level. That is, the study found the mean TE to be 38%. Another study on the determinants of production and TE among cotton farmers in the Northern part of Ghana was conducted by Adzawla, Fuseini, and Donkoh (Citation2013). The transcendental (translog) production frontier was used to estimate the production function. The empirical results revealed that farm size, labour, and fertiliser utilisations are the main determinants of cotton production in the Northern part of Ghana. Danso-Abbeam, Dahamani, and Bawa (Citation2015) also used the translog production frontier to analyse their data set. The results from the SFAindicated that labour and quantity of seeds exerted significant and positive effects on groundnut output, whilst the area of land allocated to groundnut cultivation had a negative and significant effect on groundnut output. Groundnut farmers in the study area had a mean TE score of about 84%, indicating an output loss of 16% due to inefficiency.
Many researchers have also demonstrated that institutional factors, demographic factors, and socioeconomic factors influence the efficiency of a firm in production (Danso-Abbeam et al., Citation2015; Mabe et al., Citation2018;; A-lhassan, Citation2012). Danso-Abbeam et al. (Citation2015) found that various sources of efficiency include education, farming experience, household size, membership of farmer-base organisation, and farmers' contact with extension personnel in groundnut production in Northern region of Ghana. Mabe et al. (Citation2018) also indicated that factors such as age, sex, household size, years of education, extension visits, contract farming, access to improved seeds, access to irrigation, high rainfall amount, and less lodging of rice influence the level of TE of rice farmers in Ghana. Another study was conducted on TE in smallholder paddy farms in Ghana; an analysis based on different farming systems and gender by A-lhassan (Citation2012) showed that credit availability, family size, and non-farm employment significantly determine the TE of smallholders.
Over the years, allocative efficiency of resources in production has been analysed in the agricultural field by researchers. For instance, Awunyo‑Vitor, Wongnaa, and Aidoo (Citation2016) looked at resource-use efficiency among maize farmers in Ghana. The results showed that generally, maize farmers in Ghana were inefficient in their use of resources available to them. Fertiliser, herbicide, pesticide, seed, manure, and land were underutilised, while labour and capital were overutilised by the farmers. The results further showed that maize farmers in Ghana exhibit increasing returns to scale, indicating that the famers can increase their output by increasing the use of some of the key resources. Ishiaku, Haruna, Danwanka, and Suleiman (Citation2017) also conducted their study on resource-use efficiency of fadama III small-scale rice farmers in Nasarawa State, Nigeria. The research demonstrated that the overall production elasticity of the inputs used was 1.045 and 1.356 for participants and non-participants. Participants used all the resources more efficiently than the non-participants with a ratio of 6.40 as against 9.04 for labour, 6.71 as against 7.60 for fertiliser, 3.74 as against 7.11 for seed, 7.00 as against 10.92 for herbicide, and 3.25 as against 5.27 for farm size. Also, Kadiri, Eze, Orebiyi, and Onyeagocha (Citation2014) analysed RUE and allocative efficiency of paddy rice production in Niger Delta Region of Nigeria. Result of the allocative efficiency of inputs confirmed that rice producers in the area did not attain optimal allocative efficiency, seed input (0.94) had the highest allocative efficiency, while land input (0.05) showed the least allocative efficient input. Current studies of this kind either focussed on other crops or were conducted in a different country. Also, there is a lack of studies on RUE in rice production in Ghana. Therefore, this research seeks to estimate the level of technical and RUE of smallholder rice farmers at the farm level and to identify the determinants of TE in production.
3. Materials and methods
3.1. Study area
The study was conducted in Tolon District in the Northern Region of Ghana. The district covers a total land area of 2,741 km2 with an estimated population of 72,990, with 36,360 males and 36,630 females in 2010 (GSS, Citation2012). Tolon district lies between latitude 9° 15ʹ 10° 02ʹ North and longitude 0° 53ʹ and 1° 25 West. The district shares boundaries with North Gonja District to the West, Kumbungu District to the north, Central Gonja District to the south, and to the East with Tamale Metropolitan. The main vegetation is grassland, interspersed with guinea savannah woodland, characterised by drought-resistant trees such as acacia, mango, baobab, shea-nut, dawadawa, and neem. The soils are generally of the sandy loam type except in the lowlands where alluvial deposits are found and support the cultivation of crops like rice, yam, cowpea, millet, sorghum, groundnut, etc. Majority of the inhabitants of the Tolon District are full-time farmers.
3.2. Sampling technique and sample size
The data were collected from primary sources through field survey, mainly from smallholder rice farmers’ households in the 2018 cropping season. A three-stage systematic random sampling technique was employed for this study. In the first stage, a simple random sampling technique was used to select Tolon district from the Northern region. In the second stage, five communities (i.e., Nyankpala, Tibogu, Tolon Township, Gburimani, and Gbanjogla) were randomly selected from the major rice-producing communities. In the last stage, about 26 rice farm households from each community were simple randomly selected, where every household has the chance of being included. A sample size of 126 rice farm households was obtained and used for this study.
3.3. Data analysis
The study employed descriptive statistics, inferential statistics, stochastic production frontier, and marginal value product–marginal factor cost (MVP-MFC) approach to analyse the data and the results are presented in Table . The SFA was used to examine the driving factors of rice output and measure the TE level of farmers, whereas allocative efficiency of the factor inputs was computed using the MVP-MFC. The SFA was used to measure the ability of rice farmers to use a minimum quantity of inputs under a given technology to achieve a maximum level of output (TE), whereas the MVP-MFC was used to measure their ability in achieving the best combination of different inputs in producing a given level of output considering the relative prices of these inputs (allocative efficiency).
3.3.1. The SFA
The stochastic frontier production function was employed to analyse the determining factors of rice output. The stochastic frontier function was independently proposed by Aigner, Lovell, and Schmidt (Citation1977) and Meeusen and van Den Broeck (Citation1977). SFA is based on an econometric specification of a production frontier. The specification of SFA allows for a non-negative random component in the error term to generate a measure of technical inefficiency. According to Aigner et al. (Citation1977), and Meeusen and van Den Broeck (Citation1977), the stochastic frontier production function is defined by;
where i = 1,2,3,4 … .N
where Yi is the output level of the ith farm household, is the production function of the vector, xi is the inputs for the ith farm household and a vector β is the parameter to be estimated. ei represents an error term made up of two components vi and ui. The error term vi accounts for random effects as a result of measurement errors and other factors are not under control of farmers in production. ui is a non-negative error term associated with farmer-specific factors, making farmers unable to obtain optimum efficiency in production. Thus, ui measures the technical inefficiency effects that fall within the control of the decision-making unit.
In recent times, SFA has been used by researchers such as Mabe et al. (Citation2018), Abdulai et al. (Citation2018), Danso-Abbeam, (Citation2015), and Bempomaa and De-Graft (Citation2014) in Ghana. These authors specified the TE of an individual farm household as the ratio of the observed output to the corresponding frontier output conditioned on the level of inputs used by the farm household. TE means producing the maximum amount of output with the incorporation of minimum possible amount of inputs. Technical inefficiency, therefore, refers to the margin with which the level of output for the farmer falls below the frontier output. In the description above, TE of ith farm household can be specified as:
The error term vi is assumed to be identically, independently and normally distributed with zero mean and a constant variance, . The error term ui is also assumed to be distributed as truncation of normal distribution with mean ui and variance
, such that the inefficiency error term can be explained by exogenous variables. Following Battese and Coelli (Citation1995), the inefficiency distribution parameter can be specified as;
where Zi is a vector of farmer characteristics, is a vector of parameters to be estimated, and
is unobservable random variables. STATA provides a joint estimation of the parameters in the stochastic frontier production function and those of variables in the inefficiency model as well as variance parameters.
The empirical stochastic frontier translog production model for identifying the factors influencing the output levels of ith rice farm household is specified as:
The model estimating the determinants of technical inefficiency is also given as:
3.4. RUE analysis
A research on RUE fundamentally commences with the assumption regarding the goal of producers. The classical assumption is the motive of profit maximisation, which is an ideal framework against which various forms of efficiencies of production can be adequately measured. RUE is related to the ability of a firm to choose its inputs in a cost-minimising way. In order to achieve this objective, the study employs the MVP-MFC analysis approach. This approach has been used by researchers such as Ishiaku et al. (Citation2017), Awunyo‑Vitor et al. (Citation2016), Danso-Abbeam et al. (Citation2015), and Kadiri et al. (Citation2014) where the MVPs for each input used were computed and such computed MVPs were then compared with their respective acquisition cost, MFC.
When the translog production function (in Equation (6)) is linearised and differentiated with respect to the input (Xi), the marginal physical product (MPP) can be obtained as shown below:
where
where Yi = mean value of output, Xi = mean value of input employed in the production of a product, MPPx = marginal physical product of input X, and Py = unit price of rice output. i is output elasticity of input X. The RUE of each of the measurable input used in rice production was computed by the ratio of the MVP to that of the MFC).
where RUE denotes resource-use-efficiency and MFC represents the price of the measurable factor inputs at their geometric means.
3.4.1. Decision rule
, implies that resources are used efficiently by rice farmers in the study area.
, implies resources are underutilised and increasing the rate of use of that resource will help increase productivity.
, implies resources are overutilised and reducing the rate of use of that resource will help improve productivity.
4. Results and discussions
4.1. Demographic and farm-specific characteristics of farm households
Table shows the statistical distribution of demographic and farm-specific characteristics of rice farmers in the study area. From the table, the average age of rice farmers was 45 years. The implication is that these younger farmers are likely to adopt new innovations to increase efficiency level than the older ones in production (Onubuogu et al., Citation2014; Girei et al., Citation2014; Mabe et al., Citation2018). The study also revealed that the majority (80%) of the farmers were males, while 20% were females. Also, the majority (79%) of the farmers were married, whiles 21% were unmarried. Married rice farmers tend to have easy access to production inputs such as land and large family size which are traditionally owned to enhance production (Onubuogu et al., Citation2014; Danso-Abbeam et al., Citation2015; Mabe et al., Citation2018). Again, the study found an average household size of eight persons. Large household size ensures the availability of labour and expansion of farm size. The mean household size of 8 number of persons is higher than the national average value of 4.0 (Ghana Statistical Service (GSS), Citation2014). Esiobu, Nwosu, and Onubuogu (Citation2014) found that large household size complement labour to enhance production and reduce the cost of hired labour.
Table 1. Description of variables in the stochastic frontier translog production model
Table 2. Descriptive statistics of variables in the stochastic frontier production model
The average years of education of rice farmers in the study area were 5.76 years. The result implies that approximately the farmers had formal education up to the primary school level. An exposure to high level of education is an added advantage in terms of achieving high yield/output and marketing efficiency in production (Esiobu et al., Citation2014). Again, the average farming experience of the farmers was 14 years. Onubuogu et al. (Citation2014) and Esiobu et al. (Citation2014) reported that farmers with more experience would have a better knowledge of efficient allocation of resources and market situation and are thus expected to run a more efficient and profitable enterprise. A majority (60%) of the rice farmers were members of farmer-based organisation (FBO), while 40% were not FBO members. Being a member of FBO affords farmers the opportunity of sharing information on modern production techniques, purchasing inputs in bulk as well as exchanging labour. Similarly, whereas 64% of the farmers received extension service, 34% did not receive. Access to extension service enables farmers to receive education on agricultural innovations. According to Evenson (Citation2001) and Gautam (Citation2000), a well-functioning agricultural extension system is pivotal to increasing the productivity of staple food crops and thus presents a credible avenue for moving millions of people out of poverty.
About 35% of the farmers had access to financial credit for rice production. Access to financial credit will increase the ability of farmers to purchase the needed inputs to increase productivity (Mabe et al., Citation2018). The study also showed that the average quantity of labour used for rice production was 76.46 man-days. This value is higher than an average of 44.5 man-day recorded by Mabe et al. (Citation2018). Also, an average of 3.42 l of weedicide was used for rice production. This value is higher than an average of 2.22 l recorded by Konja, Mabe, and Oteng-Frimpong (Citation2019). From the table, an average of 335.2 kg of fertiliser was used for an acre production of rice in the study area. Onubuogu et al. (Citation2014) observed that output was dependent on the amount of soil fertility in crop production. In other words, soil fertility is stimulated by the quantity of fertiliser applied to the soil.
The study found an average farm size of 2.37 acres for rice production. This implies that the farmers in the study area are mainly smallholder farmers operating on less than a hectare of farmland. This could be as a result of the land tenure system predominant in the study area. Large farm size increases agricultural productivity and improves farmers technical, allocative, and RUE. The average quantity of seed used for rice production in the study area was 82.6 kg/acre which is similar to the result of Nimoh (Citation2012). The study also indicated that the average output of rice farmers in the study area was 573.3 kg/acre. However, Mabe et al. (Citation2018) recorded an average output of 795.75 kg/acre. Rice production is dependent on the quantity and quality of seed used. Good quality and optimum quantity of planting material used in production increases output.
4.2. Determinants of technical efficiency
Table shows the maximum likelihood estimates for parameters of the stochastic translog production frontier. Following Coelli et al. (Citation2005), all the variables were normalised through mean correction and hence can be interpreted as partial elasticities. Since the sum of the model’s first-order coefficients is positive, the monotonicity condition is met.
Table 3. Maximum likelihood estimates of the stochastic translog production frontier model
The study shows that fertiliser, farm size, and quantity of weedicide used significantly affect the level of rice output in the study area. From the table, the coefficient of farm size was 0.695 and statistically significant at 1%. This implies that when farm size increases by 100%, holding other variable inputs constant, the output would increase by about 69%. This result is consistent with Abdulai et al. (Citation2018) and Amaechina & Eboh, (Citation2016). The study also indicates that the coefficient of fertiliser was 0.370 and statistically significant at 1%. This connotes that when the quantity of fertiliser used increases by 100%, holding other variable inputs constant, the output would increase by about 37%. This finding conforms to the results of Amaechina & Eboh (Citation2016) and Mabe et al. (Citation2018) but contrary to the result of Abdulai et al. (Citation2018). The coefficient of weedicide was −0.198 and statistically significant at 5%. This means that when the quantity of weedicide used increases by 100%, the output would decrease by 20%, holding other variable inputs constant. This result is in line with the finding of Amaechina & Eboh (Citation2016).
From the results, there are significant input complementary effects between “farm size and fertiliser’’ and “farm size and weedicide’’ in rice production in the study area. This implies that, when the level of the pairs of factors is jointly increased, the rice output level will increase. Statistically, there are significant substitution effects on rice output level in production. The factors that are substitutes include “seed and fertiliser’’ and “fertiliser and weedicide.” This implies that, when the pairs of these factors are jointly increased, the output level of rice will reduce in the study area.
4.3. Determinants of TE in rice production
Table shows the results explaining the determinants of technical inefficiency in rice production. From the result, age, extension access, household size, years in education, and access to credit are significant determinants of technical inefficient in the study area. As expected, the age of farmers was significant at 5% and inversely affected technical inefficiency in rice production. This implies that a unit increase of rice farmers’ age increases their TE level. This finding conforms to Ishiaku et al. (Citation2017) but contradicts the result of Yiadom-Boakye et al. (Citation2013).
Table 4. Determinants of technical inefficiency in rice production
As expected, access to extension service was statistically significant at 5% and positively affected technical inefficiency in production. This connotes that rice farmers who have access to extension service are more technically efficient than their counterparts. This result agrees with the findings of (Danso-Abbeam et al., Citation2015; Abdulai et al., Citation2018). Contrary to a prior expectation, household size was statistically significant at 1% and positively influenced the level of technical inefficiency in production. This implies that farmers with large household size are less technically efficient than those with small household size.
Also, the result indicates that years in education of rice farmers were significant at 1% and showed a negative association with technical inefficiency. This means that an increase in the year of education of farmers increases TE level in production. The result conforms with Danso-Abbeam et al. (Citation2015) who also found that access to education affects technical inefficiency negatively. As expected, access to credit was statistically significant at 10% and negatively affected the level of technical inefficiency in production. This implies that farmers who have access to credit are more technically efficient than those without. This result is consistent with the finding of Yiadom-Boakye et al. (Citation2013) but contradicts the result of Adamu et al. (2015).
4.4. Summary statistics distribution of TE of rice farmers
Table is a frequency distribution table showing the TE scores of rice producer in the study area. Rice farmers displayed a wide range of TE, ranging from 11% to 98%. The results indicated that 10% of the respondents are close to the TE frontier, whereas about 6% are far from the TE frontier. The study observed that even the most efficient respondent was not optimal in the allocation of resource and need improvement to attain frontier TE.
Table 5. Frequency distribution of technical efficiency index
The mean TE score of rice farmers in the study area was 0.75. This implies that the rice farmers achieved on average 75% level of output in the study area. This shows that about 25% of output obtained by rice farmers is lost due to inefficiencies in production. Hence, an average of 25% TE is required by rice farmers to attain frontier output in the study area. The distribution of TE among rice farmers in this study collaborates the findings of Mabe et al. (Citation2018); Danso-Abbeam et al. (Citation2015); Ogundari (Citation2008); and Abdulai et al. (Citation2018).
4.5. RUE estimation
Table shows the estimates of RUE of fertiliser, weedicide, seed, and farm size. In this study, RUE of rice farmers was measured by the ratio of the MVP of each input used to their respective factor prices. The MVP is a measure for judging how resources are allocated. Inputs are said to be optimally allocated under pure competitive condition when there is no divergence between their MVP and their unit price. The RUE index of fertiliser was 0.496, which implies that fertiliser was overutilised by rice farmers in the study area. This means that decreasing the quantity of fertiliser used in production will increase rice output in the study area. This result is in consonance with Kadiri et al. (Citation2014) but contrary to Maikasuwa and Ala (Citation2013), Nimoh et al. (Citation2012). Ishiaku et al. (Citation2017), and Awunyo‑Vitor et al. (Citation2016).
Table 6. Resource-use-efficiency estimates
The RUE index of weedicide was −0.587. A negative index associated with weedicide shows that weedicide is being used to an extent that any increase in its use reduces rice output. This means that rice farmers should reduce the quantity of weedicide used in production to increase rice output in the study area. The study found a RUE index of 0.144 for seed, which connotes that seed was overutilised in production. This implies that rice farmers should reduce that quantity of seed used in production to increase rice output. This result also agrees with the finding of Danso-Abbeam et al. (Citation2015) and Kadiri et al. (Citation2014), while it contradicts the results of Awunyo‑Vitor et al. (Citation2016), Maikasuwa and Ala (Citation2013), and Nimoh et al. (Citation2012). On the contrary, farm size recorded a RUE index of 4.518, which implies that farm size was underutilised in the study area. Underutilisation of farm size shows that increasing rice farm size by farmers in the study area would reduce the level of rice output in production. The allocative efficiency of farm size shows that too little of land is being used by the farmers in the study area. This suggests that the farmers can incur more cost inland so as to be allocative efficient in production. This result conforms to the findings of Awunyo‑Vitor et al. (Citation2016), Maikasuwa and Ala (Citation2013), and Ishiaku et al. (Citation2017) but contradicts the result of Kadiri et al. (Citation2014).
5. Conclusions and recommendations
The aim of this study was to estimate RUE in the Tolon District in the Northern Region of Ghana. The finding of this study reveals that, apart from weedicide, farm size, quantity of weedicide, and fertiliser used have positive effects on output of rice in the study. However, apart from farm size, factors such as quantity of weedicide, fertiliser, and seed were overutilised in production. The study showed that TE varied widely among rice farmers in the study area, ranging from 11% to 98% with a mean of 75%. The mean level suggests that there exists more room to increase output by improving the allocative and TE. The factors that significantly affect technical inefficiency in rice production were age of farmers, extension access, household size, years of education, and access to credit. The study recommends a farm-level policy directed towards the stimulation of extension work through motivation to give the rural farm households the needed training on farm management to improve productivity. Participatory approaches involving all stakeholders should be adopted in the design and implementation of credit schemes to rice farmers to encourage its uptake.
6. Limitations and suggestions for future research
Majority of the respondents interviewed do not keep production records and only tried to remember information from their memories. The assumption that they remembered and gave accurate information is not entirely true. There was a language barrier between the respondents and the researchers which propelled them to involve interpreters during the data collection. This study suggests that future researchers should look at the impact of climate change and gender dichotomy of RUE in rice production in the Northern region of Ghana. This recommendation seeks to establish whether or not low participation of females in rice production is attributed to inefficiency in resource use in production. It will also establish whether or not climate change has a different impact on gender in rice production.
Additional information
Funding
Notes on contributors
Dominic Tasila Konja
Dominic Tasila Konja is a holder of Master of Philosophy degree in Agricultural Economics from University for Development Studies, Ghana. His main research interest includes adoption, agricultural productivity and frontier analyses, cost benefit analysis, profitability analysis, and consumer preference analysis.
Franklin N. Mabe
Franklin N. Mabe is a lecturer at the Department of Agricultural and Resource Economics, University for Development Studies, Ghana. Franklin has researched and published on various themes relating to adoption, efficiency and welfare economics.
Hamdiyah Alhassan
Hamdiyah Alhassan is a senior lecturer at the Department of Agricultural and Resource Economics, University for Development Studies, Ghana. Hamdiyah has researched and published on various themes relating to environmental and resource economics.
References
- Abdulai, S., Nkegbe, P. K., Donkoh, S. A. (2018). Assessing the technical efficiency of maize production in northern Ghana: The data envelopment analysis approach. Cogent Food and Agriculture, 4, 1512390. doi:10.1080/23311932.2018.1512390
- Adzawla, W., Fuseini, J., & Donkoh, S. A. (2013). Estimating technical efficiency of cotton production in Yendi Municipality, Northern Ghana. Journal of Agriculture and Sustainability, 4(1), Pp.115–140.
- Aigner, D., Lovell, C. A. K., & Schmidt, P. (1977). Formulation and estimation of stochastic production function models. Journal Econometrics, 6, 21–15. doi:10.1016/0304-4076(77)90052-5
- A-lhassan, S. (2012). Technical efficiency in smallholder paddy farms in Ghana: an analysis based on different farming systems and gender. Journal of Economics and Sustainable Development, 3(5), 91–106.
- Amaechina, E. C., & Eboh, E. C. (2016). Resource use efficiency in rice production in the lower Anambra irrigation project, Nigeria. Journal of Development and Agricultural Economics, 9(8), 234–242. August 2017. doi:10.5897/JDAE2016.0764. Article Number: B4F602465323 ISSN 2006-9774 http://www.academicjournals.org/JDAE
- Awunyo‑Vitor, D., Wongnaa, C. A., & Aidoo, R. (2016). Journal of Agriculture and Food Security. Resource Use Efficiency among Maize Farmers in Ghana, 5, 28. doi:10.1186/s40066-016-0076
- Battese, G. E. (1992). Frontier production functions and technical efficiency: A survey of Empirical Applications in Agricultural Economics. Agricultural Economics, 7, 185–208. doi:10.1016/0169-5150(92)90049-5
- Battese, G. E., & Broca, S. S. (1997). Functional forms of stochastic frontier production functions and models for technical inefficiency effects: A comparative study for wheat farmers in Pakistan. Journal of Productivity Analysis, 8, 395–414. doi:10.1023/A:1007736025686
- Battese, G. E., & Coelli, T. J. A. (1995). Model for technical inefficiency effects in a stochastic frontier production function for panel data. Empirical Economics, 20(2), 325–332. doi:10.1007/BF01205442
- Bempomaa, B., & De-Graft, A. H. (2014). Technical efficiency analysis of maize production: Evidence from Ghana. Applied Studies in Agribusiness and Commerce, APSTRACT, 8(3), 73–79.
- Coelli, T. J., Rao, D. S. P., O’Donnell, C. J., & Battese, G. E. (2005). An introduction to efficiency and productivity analysis. 2nd. New York, NY: Springer.
- Danso-Abbeam, G., Dahamani, A. M., & Bawa, G. A.-S. (2015). Resource-use-efficiency among smallholder groundnut farmers in Northern Region, Ghana. American Journal of Experimental Agriculture, 6(5), 290–304. Article no. AJEA.2015.087 ISSN: 2231-0606 SCIENCEDOMAIN international www.sciencedomain.org
- Donkoh, S. A., & Awuni, J. A. (2011). Adoption of farm management practices in lowland rice production in Northern Ghana. Journal of Agricultural and Biological Science, 2(4), 84–93.
- Donkoh, S. A., Ayambila, S., & Abdulai, S. (2013). Technical efficiency of rice production at the Tono irrigation scheme in Northern Ghana. American Journal of Experimental Agriculture, 3(1), 25–42. doi:10.9734/AJEA
- Esiobu, N. S., Nwosu, C. S., & Onubuogu, G. C. (2014). Economics of pineapple marketing in owerri municipal council area, Imo State, Nigeria. International Journal of Applied Research Technology, 3(5), 3–12.
- Evenson, R. E. (2001). Economic impacts of agricultural research and extension. Handbook of Agricultural Economics, 1, 573–628.
- Farrell, M. J. (1957). The measurement of productive efficiency. Journal of Royal Statistical Society, 3, 253–290. doi:10.2307/2343100
- Gautam, M. (2000). Agricultural extension: The Kenyan experience, an impact evaluation. Washington, D.C: The World Bank.
- Ghana Statistical Service (GSS). (2012). Ghana-Population and housing census-2010. Summary report of final result. doi:10.1094/PDIS-11-11-0999-PDN
- Ghana Statistical Service (GSS). (2014). Ghana living standard survey round 6 (GLSS 6). Accra: GSS.
- Ghana Statistical Service (GSS) (2018). Provisional 2017 annual gross domestic product. Statistics for Development and Progress, April 2018 Edition. Accra, Available at hhtp://www.statsghana.gov.gh
- Girei, A. A., Dire, B., Yuguda, R. M., & Salihu, M. (2014). Analysis of productivity and technical efficiency of cassava production in Ardo Kola and Gassol Local Government Areas of Taraba State, Nigeria. Agriculture and Fisheries, 3(1), 1–5. doi:10.11648/j.aff.20140301.11
- Institute of Statistical, Social and Economic (ISSER). (2014). State of the Ghanaian economy. In Institute of Statistical, Social and Economic Research. University of Ghana, Legon.
- Ishiaku, O. K., Haruna, U., Danwanka, H. A., & Suleiman, H. R. (2017). Resource use efficiency of fadama III small-scale rice farmers in Nasarawa State, Nigeria. International Journal of Agricultural Economics and Extension. ISSN: 2329-9797. Vol. 5 (4), pp. 284–294, April, 2017. Available online at. (), . . www.internationalscholarsjournals.org
- Kadiri, F. A., Eze, C. C., Orebiyi, J. S., & Onyeagocha, S. U. O. (2014). Resource-use and allocative efficiency of paddy rice production in Niger Delta Region of Nigeria. Global Journal of Agricultural Research, 2(4), 11–18. December 2014. Published by European Centre for Research Training and Development UK. Available at. www.eajournals.org
- Konja, D. T., Mabe, F. N., & Oteng-Frimpong, R. (2019). Profitability and profit efficiency of certified groundnut seed and conventional groundnut production in Northern Ghana: A comparative analysis. Cogent Economics & Finance, 7, 1631525. doi:10.1080/23322039.2019.1631525
- Korir, M. K., Serem, A. K., Sulo, T. K., & Kipsat, M. J. (2013). A stochastic frontier analysis of bambara groundnut production in Western Kenya. 18th International Farm Management Congress, Methven, Canterbury, New Zealand. 3:74–80.
- Mabe, F. N., Donkoh, S. A., & Al-hassan, S. (2018). Accounting for rice productivity heterogeneity in Ghana: The two-step stochastic metafrontier approach. International Journal of Agricultural and Biosystems Engineering, 12(8), 223–232. doi:10.1999/1307-6892/10009379
- Macauley, H., & Ramadjita, T. (2015). Cereal crops: Rice, maize, millet, sorghum, wheat. file:///C:/Users/user/Downloads/Documents/Cereal_Crops_Rice__Maize__Millet__Sorghum-Wheat.pdf
- Maikasuwa, M. A., & Ala, A. L. (2013). Determination of profitability and resource-use efficiency of yam production by women in Bosso local government area of Niger State, Nigeria. European Scientific Journal, June (2013), Edition. Vol. 9, No.16. 1857–7881 (Print) e - ISSN 1857-7431.
- Meeusen, W., & van Den Broeck, J. (1977). Efficiency estimation from Cobb–Douglas Production Functions with Composed Error. International Economic Review, 18(2), 435–443. doi:10.2307/2525757
- Ministry of Food and Agriculture (MoFA). (2011). Agriculture in Ghana: Facts and figures. In Accra: Ministry of food and agriculture, statistics, research and information directorate (MoFA-SRID).
- MoFA. (2016). Statistics, research and information directorate (SRID), Ministry of food and agriculture. March, 2016.
- MoFA (2017). Ministry of Food and Agricultural Progress Report 2017. file:///C:/Users/user/Downloads/Documents/MoFA%202017%20AGRICULTURAL%20PROGRESS%20REPORT_Final.PPMED.MoFA.pdf
- Nargis, F., & Lee, S. H. (2013). Efficiency analysis of Boro rice production in north-central region of Bangladesh. The Journal of Animal and Plant Sciences, 23(2), 527–533.
- Nimoh, F., Tham-Agyekum, E. K., & Nyarko, P. K. (2012). Resource-use efficiency in rice production: the case of kpong irrigation project in the Dangme West District of Ghana. International Journal of Agriculture and Forestry, 2(1), 35–40. doi:10.5923/j.ijaf.20120201.06
- Ogundari, K. (2008). Resource-productivity, allocative efficiency and determinants of technical efficiency of rain-fed rice farmers: A guide for food security policy in Nigeria. Agricultural Economics, 54(5), 224–233. doi:10.17221/AGRICECON
- Ohen, S. B., Ene, D. E., & Umeze, G. E. (2014). Resource use efficiency of cassava farmers in Akwa Ibom State, Nigeria. Journal of Biology, Agriculture and Healthcare, 4(2). ISSN 2224-3208 (Paper) ISSN 2225-093X(Online) http://www.iiste.org
- Okoye, B. C., Abass, A., Bachwenkizi, B., Asumugha, G., Alenkhe, B., Ranaivoson, R., … Ralimanana, I. (2016). Differentials in technical efficiency among smallholder cassava farmers in Central Madagascar: A Cobb Douglas stochastic frontier production approach. Cogent Economics & Finance, 4, 1143345. doi:10.1080/23322039.2016.1143345
- Oladele, O. I., Kolawole, A., & Wakatsuki, T. (2011). Land tenure, investment and adoption of Sawah rice production technology in Nigeria and Ghana: A qualitative approach. African Journal of Agricultural Research, 6(6), 1519–1524.
- Onubuogu, G. C., Esiobu, N. S., Nwosu, C. S., & Okereke, C. N. (2014). Resource use efficiency of smallholder cassava farmers in Owerri Agricultural zone, Imo State, Nigeria. Scholarly Journal of Agricultural Science, 4(6), 306–318. June, 2014 ISSN 2276-7118. Available online at. http://www.scholarly-journals.com/SJAS
- Ragasa, C., Dankyi, A. A., Acheampong, P., Nimo-Wiredu, A., Chapoto, A., Asamoah, M., & Tripp, A. (2013), Patterns of adoption of improved rice technologies in Ghana, Ghana Strategy Support Program and International Food policy research institute. Working Paper No.35, July 2013. Accra, Ghana.
- Rockström, J., Barron, J., & Fox, P. (2003). Water productivity in rain-fed agriculture: Challenges and opportunities for smallholder farmers in drought-prone tropical agro-ecosystems. In J. W. Kijne, D. Molden, & R. Barker (Eds.), Water productivity in agriculture: Limits and opportunities for improvement. Comprehensive Assessment of Water Management in Agriculture (Vol. 1, pp. 145–162). Wallingford, UK: CABI International.
- Roetter, R. P., Van Kuelen, H., Kuiper, M., Verhagen, J., & Van Laar, H. H. (2008). Science for agriculture and rural development in low-income countries. The Netherlands: Springer Publications.
- Rukuni, M. (1994). Evaluation of agricultural policy: 1880-1990. Harare, Zimbabwe: University of Zimbabwe Publications.
- Sanusi, S. M., Ogungbile, A. O., Yakasai, M. T., Ahmad, M. M., & Daneji, M. I. (2016). Optimization of resource use efficiency in small scale maize production in Niger State, Nigeria. Asian Journal of Science and Technology, 6(02), 1070–1075. February, 2015. Available Online at. http://www.journalajst.com
- Seck, P. A., Diagne, A., Mohanty, S., & Wopereis, M. C. S. (2012). Crops that feed the world 7: rice. Food Security, 4(1), 7–24. doi:10.1007/s12571-012-0168-1
- Sujan, M. H. K., Islam, F., Azad, M. J., & Rayhan, S. J. (2017). Financial profitability and resource use efficiency of boro rice cultivation in some selected area of Bangladesh. African Journal of Agricultural Research, 12(29), Pp.2404–2411. doi:10.5897/AJAR2017.12443
- United State Department of Agriculture (USDA). (2014). Foreign agricultural service. Grain and Feed Update Ghana.
- Wiredu, A. N., Gyasi, K. O., Marfo, K. A., Asuming-Brempong, S., Haleegoah, A., Asuming-Boakye,, & Nsiah, B. F. (2010). Impact of improved varieties on the yield of rice producing households in Ghana. Paper Prepared for the Second Africa Rice Congress, Bamako, Mali, 22-26 March 2010: Innovation and Partnerships to Realize Africa’s Rice Potential.
- Yiadom-Boakye, E., Owusu-Sekyere, E., Nkegbe, P. K., & Ohene-Yankyera, K. 2013. Gender, resource use and technical efficiency among rice farmers in the Ashanti Region, Ghana. Journal of Agricultural Economics and Development, 2(3), 102–110. ISSN 2327-3151 ©2013. Available online at http://www.academeresearchjournals.org/journal/jaed
- Zhu, J. (2003). Quantitative models for performance evaluation and benchmarking: Data envelopment analysis with spreadsheets and excel solver. Boston: Kluwer Academic Publishers.