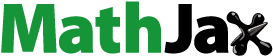
Abstract
In Ethiopia, the fishery sector plays an important role to improve food security, employment creation, income generation and poverty reduction. Despite the economic importance of fish farming at the household and national level, farm households have paid little attention to the sector in the study area. Consequently, this study was undertaken to examine the determinants of farm households’ participation in fish production. For this purpose, a cross-sectional survey with 120 households was conducted to collect quantitative primary data. Descriptive statistics, inferential statistics and binary logit model were used to analyze the data. The result indicated that farm households’ participation in fish production is determined by various socioeconomic and institutional factors. Age of the household head, educational status, household size, extension service and access to modern transportation service had a statistically significant effect on farm households’ participation in fish production. An attempt to increase farm households’ participation in fish production should give special attention to the existing heterogeneity in socioeconomic and institutional factors.
PUBLIC INTEREST STATEMENT
In Ethiopia, the fishery sector contributes significantly to the national economy through employment creation, poverty reduction and food security support. Although the country has huge fish potential, the fishery sector is still underexploited. Additionally, farm households have not fully reaped from fish farming which has huge potential to improve the living standards of their family. Likewise, the participation of farm households’ in fish production is poor. This needs research to answer the question why farm households’ participation in fish production is poor. Answers to the aforementioned question give information for policy makers and professionals to strengthen the participation of farm households in fish production. Therefore, this study provides the necessary information to responsible stakeholders to design and implement different strategies to promote the participation of farm households in fish production.
Competing interest
The authors declare no competing interests.
1. Introduction
Fish is an aquatic animal which serves as sources of food, nutrition, income and livelihood for millions of people in the world (G/Michael & Fantahun, Citation2019). Fish farming has quickly grown animal-based food production sector since the ancient civilization of Egypt and China (Olaoye et al., Citation2013; Tilahun, Alambo, & Getachew, Citation2016). Nowadays, it is practiced both in developed and developing countries (Miller, Citation2009). In developing countries, the livelihood of more than 500 million people are directly or indirectly tied with fish farming (Brander, Citation2010). In Africa, fish production, processing and marketing activities support the livelihood of more than 10 million people (Olaoye et al., Citation2013). As compared to the marine fishery, inland fisheries of Africa have 2.1 million tons of fish (FAO, Citation2004; Tilahun et al., Citation2016) which has become a major export item for Africa with an annual export value of $2.7 billion (Olaoye et al., Citation2013).
Ethiopia is a country with an area of 1,127,127 km2, water bodies cover approximately 7400 km2 (Cheffo, Teshome, & Tesfaye, Citation2015). In Ethiopia, water bodies are a home of diverse aquatic life including more than 180 fish species of which about 40 are endemic (Birhanu, Citation2015). Currently, the annual exploitable fish potential of Ethiopia is 51,481 tons (Chekol, Citation2013; Hirpo, Citation2017; Meko, Kebede, Hussein, & Tamiru, Citation2016) of which only 24% is exploited right now. According to FAO (Citation2008), fish farming has become an alternative to agriculture which depends on seasonal rainfall like Ethiopia. This indicated that fishery resources in Ethiopia have a vital role in poverty reduction, food insecurity and employment creation, yet the sector is not given due attention to any degree of comparable status (Lemma, Citation2012). Similarly, World Bank (Citation2012) stressed that the contribution of the fishery sector is less than 1% of a country’s Gross Domestic Product (GDP) though the sector has huge potential for the economic growth of the country. As discussed by Mwangi (Citation2008), the fishery sector contributes significantly to the national economy through employment generation, poverty reduction and food security support. In consequence, the livestock and fisheries sectors have given priorities in the government’s second Growth and Transformation Plan (GTPII) from 2016 to 2020 (World Bank, Citation2017). Consistent with the agricultural sector policy objectives, fishery is increasingly recognized as an alternative means of addressing the problem of food insecurity and poverty in Ethiopia. But fish production is underexploited with respect to its ability to achieve food security and poverty reduction (BAMGF, Citation2010). Similarly, fishing as a secondary or complementary activity can thus be indispensable for rural households both in terms of income source and food security (FAO, Citation2004). As the report of MoFED (Citation2010) revealed, the fishery sector provides regular employment for 21 thousand poor people and seasonal employment for others.
In Oromia region, fishery shares 18.5% of the country’s total fish production of which Gilgel Gibe river has a 0.8% share (Assefa, Citation2013). The Gelgel Gibe is located in the Jimma Zone of Oromia region, Ethiopia (Cheffo et al., Citation2015). The same source explained that the river has huge fish potential and is well known in Barbus intermediacy, Oreochromis niloticus and Labeo barbus fish species. According to Lemma (Citation2012), although there is some form of fisheries practiced in most freshwater bodies in Ethiopia, commercial fishery is concentrated at Lakes Tana, Chamo, Ziway, Abaya, Koka, Langano, Hawassa and Turkana. Similarly, Hirpo (Citation2017) underscored that 40% and 50.2% of fish supply to the major urban centers in Ethiopia are captured from the Rift Valley lakes and Lake Tana. This indicates that farm households who live in near the Gilgel Gibe river do not participate in fish production and marketing though fish marketing has given prior attention by the government of Ethiopia (Hirpo, Citation2017).
Despite the economic importance of fish farming at the household and national level, farm households have paid little attention to the sector in the study area (Hecht, John, Matthias, & Rohana, Citation2006). In addition to this, fish farmers have not fully reaped from the activity which has huge potential to improve the living standards of their family. Even though fishery gives many advantages to the economy of the country, currently the participation of farm households’ in fish production is poor. This needs research to answer the question why farm households do not participate in fish production. Answers to the aforementioned question give information for policy makers and professionals to strengthen the participation of farm households in fish production. The study area (Omo Nada Woreda) is located near the Gilgel Gibe hydroelectric dam which has huge fish potential and various fish species diversity, but farm households do not participate in fish production. Therefore, an extensive literature review was undertaken to evaluate the participation of farm households in fish production in Omo Nada woreda. However, studies regarding why farm households do not participate in fish production are nil. Consequently, this study was conducted to fill the above-mentioned gap which enables to design and implement different strategies to promote the participation of farm households in fish production. Hence, the objective of this study was to examine factors affecting farm households' participation in fish production in Omo Nada woreda.
2. Materials and methods
2.1. Description of the study area
This study was carried out in the Jimma Zone of Oromia region particularly in Omo Nada woreda. Omo Nada is one of the woredas in Jimma zone, Oromia region of Ethiopia. Geographically, it lies between 7°17ʹ to 7°49ʹ North latitudes and 37°00ʹ to 37°28ʹ East longitudes (Engdawork, Citation2015). It is located at 295 km far from Addis Ababa and 71 km from Jimma, Ethiopia. Omo Nada is bordered on the West by Dedo, on the North by Sokoru, on the South by Kersa and on the East by Tiro Afata (Engdawork, Citation2015).
The altitude of the study area ranges from 1000 to 3340 meter above sea level. It has three main rivers, namely: Gilgel Gibe, Nada Guda and Beyem. The land survey report shows that nearly 56.8% is arable, 25.2% pasture, 6.3% forest, and the remaining 11.7% is considered swampy and degraded land. The woreda is classified into dega (12%), woinadega (63%) and kolla (25%) agroclimatic zones. The average rainfall is 1467 mm (Endriyas, Belachew, & Megerssa, Citation2018). The minimum and maximum annual rainfall ranges from 1066 to 1200 mm with a mean annual temperature ranging from 18°C to 25°C (Engdawork, Citation2015). The total population of the woreda is 248,173 of whom 124,289 were men and 123,884 were women; 4.92% of its population were urban dwellers. Omo Nada has an estimated population density of 158.7 people per square kilometer, which is greater than the Zone average of 150.6 (CSA, Citation2007).
2.2. Sampling procedure and method of data collection
A multistage random sampling technique was applied to draw representative samples for this study. In the first step, Omo Nada woreda was selected purposively due to the high fish potential of the woreda. In the second step, two kebeles, namely: Haro Gibe and Wirtuyaddin were selected randomly. Following this, target households were stratified into participants and non-participants in fish production to capture their heterogeneity. Finally, a total of 120 sample households (60 households from participants and 60 from non-participants) were drawn using stratified random sampling followed by a simple random sampling technique.
To develop a draft survey questionnaire, checklists were prepared to conduct key informant interview and focus group discussions. A total of 20 key informant interviews and two focus group discussions were made. Then, the draft questionnaire was prepared. Moreover, a pretest survey was conducted to test data collection instruments, estimate the time required to finalize the interview and revise the questionnaire accordingly. For this purpose, 10 households were randomly selected for the pretest survey before the actual survey. Then, a semi-structured survey questionnaire was employed to produce quantitative cross-sectional primary data on demographic, socioeconomic, institutional variables and farm households' participation in fish production through face-to-face interview (Table ). Finally, well-trained enumerators who have good experience in the household survey were employed to gather the data required for this study.
Table 1. Definitions of working variables
2.3. Method of data analysis
Descriptive statistics, inferential statistics, and econometric model were used to analyze the data. The collected data were analyzed using SPSS version 22.0 and STATA 14.
Descriptive statistics: Descriptive statistics such as percentage, frequency, mean and standard deviation were employed.
Inferential statistics: T-test and chi-square test were employed to statistically measure the mean difference of continuous variables and the association of categorical variables with participation in fish production, respectively.
Econometric model: Households’ participation in fish production is the dependent variable of this study which takes 1 if the household is participant, 0 otherwise. Binary logit model was employed for this study. Mathematically, the model can be specified following (Cameron & Trivedi, Citation2005; Gujarati & Porter, Citation1999; Wooldridge, Citation2010):
where Pi represents the probability that the ith household participates in fish production and Zi is a linear function of independent variables (X) and is expressed as:
where β0 is the constant term, βi is coefficients of independent variables, Xi independent variables, and Ui is the error term. The βi tells us how the log-odds in favor of participants change as the independent variables change. The odds to be used can be defined as the ratio of the probability that a household is a participant in fish production (Pi) to the probability that he/she is not (1—Pi), i.e.
Finally, the model is specified as follows:
There is no direct way to explain the effect of a unit increase in an explanatory variable on the probability of participating in fish production keeping other variables constant. We can obtain an approximate answer by taking derivatives with respect to Xi, which of course makes sense for non-linear econometric models (Asrat, Belay, & Hamito, Citation2004). This result tells us by how much the dependent variable changes with respect to a small change in the independent variables. As a result, the effect of each significant independent variable on the probability of households’ participation in fish production is computed by keeping other variables constant, i.e.
Both literature review and focus group discussion were undertaken to identify potential independent variables which influence households’ participation in fish production. The effect of independent variables on the dependent variable were hypothesized based on the information obtained from a theoretical literature review of previous works (Birhanu, Citation2015; Cheffo et al., Citation2015; George, Olaoye, Akande, & Oghobase, Citation2010; Ofuoku, Olele, & Emah, Citation2008) and focus group discussions. The potential variables are listed in the table (Table ).
3. Results
3.1. Relationship between categorical variables and participation in fish production
About 86.7% of the respondents were male-headed, whereas the remaining 13.3% were female-headed households. Moreover, 47.5% of male-headed households and 3.3% of female-headed households were participated in fish production (Table ). The association between sex of the respondents and participation in fish production was statistically significant at p < 0.05 significance level. Among the respondents, 68.3% and 31.7% were literate and illiterate households, respectively. Table further revealed that 43.3% and 7.5% of participant households were literate and illiterate, respectively, whereas 24.2% and 25% of illiterate and literate household heads were not participated in fish production, respectively. Educational status and participation in fish production had a statistically significant association at p < 0.01 significance level.
Table 2. Descriptive statistics of categorical variables
The majority of the respondents (54.2%) received extension service, while 45.8% of the respondents did not receive extension service. Moreover, 39.2% and 11.7% of extension service users and non-users participated in fish production, while 15% and 34.2% of extension service users and non-users did not participate in fish production. The association between extension service and participation in fish production was statistically significant at p < 0.01 significance level. About 32.5% and 18.3% of participant households were credit users and non-users, respectively, whereas 20.8% and 28.3% of non-participant households were also credit users and non-users, respectively. Credit access and participation in fish production had a statistically significant association at p < 0.05 significance level.
The presence of surplus agricultural production is not an end to improve the living standard of the people. The issue of infrastructure, particularly access to modern transport services, serves as a backbone to transport production from surplus to deficit areas. Accordingly, 58.3% of the respondents’ access to modern transportation service to supply their product to the market, whereas 41.7% of the respondents did not access modern transportation service. Furthermore, 49.2% and 1.7% of participant households were users and non-users of transportation service. Conversely, 9.2% and 40% of non-participants were users and non-users of transportation service. The association between access to modern transportation service and participation in fish production was statistically significant at p < 0.01 significance level (Table ).
3.2. Relationship between continuous variables and participation in fish production
The age of the respondents ranges from 16 to 61 years with a mean and standard deviation of 36.9 and 12.4 years, respectively. The mean age of participant and non-participant households were 29 and 45 years, respectively. The mean age of the non-participant households were higher than the mean age of the whole observation. This implies that non-participant households are older than participant households. The mean age difference between participant and non-participant households was statistically significant at p < 0.01 significance level (Table ). The average household size of the total observation was 7 with a standard deviation of 2.5. Similarly, the average household size for participant and non-participant households were 8 and 7, respectively. The mean household size difference between participant and non-participant households was statistically significant at p < 0.05 significance level.
Table 3. Descriptive statistics of continuous variables
Distance from the lake was considered as one factor to participate in fish production. If the lake is far from the respondents’ home, it creates a burden for the participant households to transport harvested fish. The average distance of the lake for participant and non-participant households were 27.9 km and 39.9 km, respectively. The mean distance from the lake difference between participant and non-participant households was statistically significant at p < 0.05 significance level. This entails that the participation of the households decreases if their home is far from the lake.
Moreover, the chi-square value is significant at less than 1% significance level. This indicates that the overall significance of the model is good. The coefficients, statistical tests and marginal effects of each independent variable are presented under Table . The model result revealed that two variables were statistically significant at less than 1% significance level. These variables are the educational status of the household head and extension service, whereas age of the household head, household size and access to modern transportation service were statistically significant at less than 5% significance level (Table ).
Table 4. Result of the binary logit model
4. Discussion
In the study area, farm households' participation in fish production was influenced by socioeconomic and institutional factors. For example, the age of the household head had a negative and statistically significant effect on households’ participation in fish production. The probability of participating in fish production decreases by 4.6% for a unit increase in the age of the household head (Table ). This entails that as the age of the head gets older and older, his/her participation in fish production decreases. This is due to the fact that fish production and management of their product are tiresome for aged household heads. Additionally, these old household heads confront active labor force deficit to undertake farm activities besides fish production. Due to this reason, they fail to participate in fish production. Inline with the result of this study, George et al. (Citation2010) conclude that people in the active age are very much involved in fish hatchery enterprise, while the older people may not have enough capital and strength for fish farming.
As expected, educational status had a positive and statistically significant effect on households’ participation in fish production. The probability of participating in fish production increases by 74.4% if the head is literate. This implies that the participation of literate households in fish production is more than illiterate households. This is because literate heads have better knowledge about the role of fish production to diversify household income so as to achieve food security of his/her family than illiterate household heads. In addition to this, education is an important weapon to change the lives of smallholder farmers because educated household heads adopt important technologies and manipulate these technologies easily to improve their production and productivity which gives them more chance to engage in other alternative farm and non-farm activities. For example, Muchangi (Citation2016) examined the relationship between educational status and its influence on fish production and found that educational status had a significant influence on fish production at less than 5% significance level, consistent with the finding of this study.
The other important variable is the household size. Household size had a positive and statistically significant effect on households’ participation in fish production. The model result revealed that the probability of participating in fish production increases by 13.7% for a unit increase in household size. This relationship indicates that large household size increases the households’ participation in fish production. This is because having a large household size requires more food and income to satisfy their basic needs. In the study area, fish production is the dominant income and food source. As a result, the household head who has a large household size has participated in different fish production and processing activities to full fill the basic needs of his/her household members. The result of this study is consistent with previous studies (Birhanu, Citation2015).
We expect a positive relationship between extension service and households’ participation in fish production. As expected, they had a positive and statistically significant effect at less than 5% significance level. The probability of participating in fish production increases by 65.6% if the head gets an extension service. This indicates that extension service increases households’ participation in fish production because the extension agent serves as a bridge between farm households and technology innovators. They provide training to improve the technical skills of farm households to improve their fish production and productivity. This act triggers non-participants to engage in fish production. The report of Njagi, Njati, and Huka (Citation2013) indicated that fish farm profitability, productivity and fish farm-oriented programs were failed due to inadequate and poor extension service. This clearly reflects the role of extension service on fish farming. Extension service improves the technical skill of farmers to increase fish production by adopting different pond management practices.
Similarly, access to modern transportation service had a positive and statistically significant effect on households’ participation in fish production. The model result indicated that the probability of participating in fish production increases by 74.5% if the head gets access to modern transportation service. Modern transport improves the quality of fish product by minimizing the perishability of the product and facilitates the interaction between surplus and deficit geographical areas. This indicates that transportation service is a crucial factor to create a market for fish production which improves the living standards of the households. According to Kumolu-Johnson and Ndimele (Citation2011), fish is the most perishable food item especially in the tropical climates of developing countries. Therefore, modern transportation service is a viable solution to minimize the degree of perishability. Similarly, most of the fish catch from the lakes reach the market by traditional means of transportation which causes mechanical stress to fish products (Hirpo, Citation2017). This entails that modern transport service enables farm households to supply raw and processed fish in different areas where fish products have higher demand. This helps them to receive a better price for their product and increase their benefit from their product which leads to the improved living standard of their household members. The discussion by Asmah (Citation2008) reported that fish is one of the most perishable of human food item, which starts spoiling soon after death. The perishability nature of fish after death and lack of modern transport service heavily hamper the participation of farm households in fish production. Therefore, effective transportation service coupled with value addition activities minimizes the spoilage of fish after death. This entails that modern transport services increase farm households' participation in fish production.
5. Conclusion
This study examined factors affecting farm households' participation in fish production. The result indicated that male-headed households, literate households, extension service users, credit access users and modern transportation service users participated in fish production more than their counterpart. Additionally, the econometric model result indicated that the age of the household head, educational status, household size, extension service and access to modern transportation service had a statistically significant effect on farm households’ participation in fish production. The findings of this study indicate that an attempt to increase farm households’ participation in fish production should give special attention to the existing heterogeneity in socioeconomic and institutional factors. Moreover, the distance from the lake limits farm households’ participation in fish production. Therefore, the role of necessary facilities like road and transportation service should be recognized to increase farm households’ participation in fish production. The result also revealed that the participation of old-aged household heads was limited in fish production. Therefore, it is important to design age-based participation mechanism that combines old-aged and young household heads.
Additional information
Funding
Notes on contributors
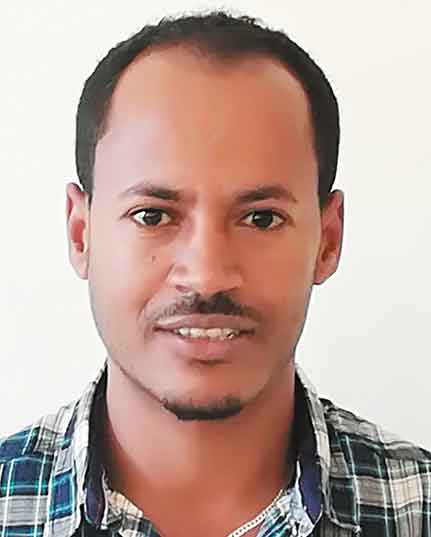
Birara Endalew
Birara Endalew joined Agriculture and Environmental Sciences College of Bahir Dar University in 2014 after he holds his bachelor degree in Agricultural Economics from Agriculture and Veterinary Medicine College of Jimma University, Ethiopia. After one-year work experience, he got in-country scholarship in Bahir Dar University. Now, he holds his master degree in Agricultural Economics on 20 December 2017. Now, he is engaged in teaching, research and community service. Specially, environmental resource valuation, food security, impact assessment, commercialization, value chain analysis, livelihood analysis, poverty analysis and technical efficiency are the author’s interest area of research.
Melese Zeleke
Mr. Melese Zeleke is lecturer at Debre Markos University.
Workneh Yenewa
Mr. Workneh Yenewa is an Assistant Researcher in Ethiopian Institute of Agricultural Research.
Zemen Ayalew
Dr. Zemen Ayalew is an Assistant Professor in Bahir Dar University. Food security, livelihood analysis, impact assessment and poverty analysis are the authors' interest area of research.
References
- Asmah, R. (2008). Development potential and financial viability of fish farming in Ghana. Scotland: Institute of Aquaculture University of Stirling. Retrieved from https://dspace.stir.ac.uk/bitstream/1893/461/1/PhD%20Document.pdf
- Asrat, P., Belay, K., & Hamito, D. (2004). Determinants of farmers’ willingness to pay for soil conservation practices in the southeastern highlands of Ethiopia. Land Degradation & Development, 15, 423–12. doi:10.1002/(ISSN)1099-145X
- Assefa, M. (2013). Assessment of fish products demand in some waterbodies of Oromia, Ethiopia. International Journal of Agricultural Sciences, 3, 628–632.
- BAMGF. (2010). Accelerating Ethiopian agriculture development for growth, food security, and equity. Bill and Melinda Gates Foundation report. Addis Ababa, Ethiopia. Retrieved from https://ethiopianagriculture.files.wordpress.com/2010/11/ethiopia-agriculture-diagnositc-integrated-report-july-20103.pdf
- Birhanu, W. (2015). Determinants of fish production in Lake Ziway. Ethiopia. St. Mary University. Retrieved from http://repository.smuc.edu.et/bitstream/123456789/2484/1/Wubeshet%20Birhanu.pdf
- Brander, K. (2010). Impacts of climate change on fisheries. Journal of Marine Systems, 79, 389–402. doi:10.1016/j.jmarsys.2008.12.015
- Cameron, A. C., & Trivedi, P. K. (2005). Microeconometrics: Methods and applications. New York, NY: United States of America by Cambridge University Press.
- Cheffo, A., Teshome, H., & Tesfaye, G. (2015). Opportunities and challenges of fish marketing at Gelgel Gibe Dam in Ethiopia. St.Mary University. Retrieved from http://197.156.93.91/bitstream/123456789/2668/1/Abebe%20Cheffo%20Revised%2015%20April%202016.pdf
- Chekol, A. (2013). Management and livelihood opportunity of Lake Tana Fishery. Ethiopia: the need for co management. Norwegian College of Fisheries Science, the Arctic University of Norway. Retrieved from https://munin.uit.no/bitstream/handle/10037/5916/thesis.pdf?sequence=1
- CSA. (2007). Population and housing census report. Addis Ababa: Central Statistical Agency, Ethiopia.
- Endriyas, M., Belachew, T., & Megerssa, B. (2018). Missed opportunities in family planning: Process evaluation of family planning program in Omo Nada district, Oromia region, Ethiopia. Contraception and Reproductive Medicine, 3, 1–9. doi:10.1186/s40834-018-0068-7
- Engdawork, A. (2015). Characterization and classification of the major agricultural soils in cascape intervention woredas in the central highlands of Oromia region. Ethiopia: CASCAPE–Addis Ababa University.
- FAO. (2004). The state of world fisheries and aquaculture. Rome, Italy: Food and Agriculture Organization of the United Nations.
- FAO. (2008). The State of World Fisheries and aquaculture. Rome, Italy: Food and Agriculture Organization of the United Nations.
- George, F., Olaoye, O., Akande, O., & Oghobase, R. (2010). Determinants of aquaculture fish seed production and development in Ogun State, Nigeria. Journal of Sustainable Development in Africa, 12, 22–34.
- Gujarati, D. N., & Porter, D. C. (1999). Essentials of econometrics. Singapore: Irwin/McGraw-Hill.
- Hecht, T., John, F. M., Matthias, H., & Rohana, P. S. (2006). Regional review on aquaculture development: Sub-Saharan Africa. Rome, Italy: Food and Agriculture Organization of the United Nations.
- Hirpo, L. A. (2017). Fisheries production system scenario in Ethiopia. International Journal of Fisheries and Aquatic Studies, 5, 79–84.
- Kumolu-Johnson, C., & Ndimele, P. (2011). A review on post-harvest losses in Artisanal fisheries of some African countries. Journal of Fisheries and Aquatic Science, 6, 365–378. doi:10.3923/jfas.2011.365.378
- Lemma, B. (2012). Report on the value chain assessment of the fishery sector in Ethiopia. Addis Ababa, Ethiopia: Food and Agriculture Organization, Sub-Regional Office for Eastern Africa.
- Meko, T., Kebede, A., Hussein, A., & Tamiru, Y. (2016). Review on opportunities and constraints of fishery in Ethiopia. International Journal of Poultry and Fisheries Sciences, 1, 1–8.
- Michael, A., & Fantahun, T. (2019). Assessment of the fishery, challenges and opportunities of Denbi reservoir in Bench Maji Zone, South Western part of Ethiopia. International Journal of Fisheries and Aquaculture, 11, 7–12. doi:10.5897/IJFA2018.0692
- Miller, J. W. (2009). Farm pond for water, fish and livelihood. Retrived from http://www.fao.org/3/i0528e/i0528e.pdf.
- MoFED. (2010). Ministry of Finance and Economic Development, poverty and food Security situation report, 2010. Addis Ababa, Ethiopia.
- Muchangi, C. T. (2016). Influence of farmer’s characteristics, agricultural extension and technology specific factors on adoption of organic farming technologies in Embu West Sub County, Embu, Kenya. PhD. Thesis, University of Nairobi, Embu, Kenya.
- Mwangi, M. (2008). Aquaculture in Kenya: Status, challenges and opportunities. Kenya: Directorate of Aquaculture Development.
- Njagi, K. A., Njati, I. C., & Huka, G. (2013). Factors affecting profitability of fish farming under economic stimulus programme in Tigania East District, Meru County, Kenya. IOSR Journal of Business and Management, 15, 25–36. doi:10.9790/487X-1532536
- Ofuoku, A., Olele, N., & Emah, G. (2008). Determinants of adoption of improved fish production technologies among fish farmers in Delta State, Nigeria. Journal of Agricultural Education and Extension, 14, 297–306. doi:10.1080/13892240802416186
- Olaoye, O., Ashley-Dejo, S., Fakoya, E., Ikeweinwe, N., Alegbeleye, W., Ashaolu, F., & Adelaja, O. (2013). Assessment of socio-economic analysis of fish farming in Oyo State, Nigeria. Global Journal of Science Frontier Research Agriculture and Veterinary, 13, 45–55.
- Tilahun, A., Alambo, A., & Getachew, A. (2016). Fish production constraints in Ethiopia: A review. World Journal of Fish and Marine Sciences, 8, 158–163.
- Wooldridge, J. M. (2010). Econometric analysis of cross section and panel data. Cambridge, Massachusetts London, England: The MIT press.
- World Bank. (2012). Hidden harvest: The global contribution of capture fisheries. Economic and sector work report no. 66469. Retrived from http://documents.worldbank.org/curated/en/515701468152718292/pdf/664690ESW0P1210120HiddenHarvest0web.pdf.
- World Bank. (2017). Livestock and fisheries sector development project. Retrieved from http://documents.worldbank.org/curated/en/455541540225547415/pdf/Disclosable-Version-of-the-ISR-Livestock-and-Fisheries-Sector-Development-Project-P159382-Sequence-No-02.pdf.