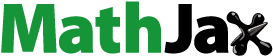
Abstract
Despite number of improved teff varieties with their production package (teff technologies, here after) are released/recommended for dryland areas, failure to integrate farmers’ desired traits and profitability analysis weakened their adoption rate. Scale-wide participatory evaluation and promotion of improved teff technologies was thus conducted to assess their profitability, farmer preferences and constraints in Northeast Amhara drylands. Zoble and Kuncho improved teff technologies were compared with the local teff production practice for three consecutive years on un-replicated farms using 270 farmers. All necessary data were collected and analyzed in descriptive statistics, cost-benefit ratio, weighted matrix and poisson regression model. Therefore, Zoble teff provided 1620 and 1280 kgha−1 average yield in Sekota and Lasta districts, respectively. Kuncho teff provided average yield of 1830 and 1420 kgha−1 in Lasta and Sekota districts. They also ensured 3.7 and 4.5 ETB return for 1 ETB investment on production costs in Sekota and Lasta districts, orderly. Moreover, Zoble and Kuncho teff were primary and secondary choices of farmers in Sekota, and vice-versa in Lasta. The regression result revealed that except land holding size of the household head, other significant factors (sex, family size, training and credit access) were positively influenced teff technology package application. Hence, Zoble and Kuncho teff technologies should further diffuse to Sekota and Lasta districts, respectively. Providing access to package components, eminence training and credit services is worthwhile to enhance farmers’ technology package application. Agricultural mechanization research also should take an initiative to design labor relaxing devices to ease the labor pressure imposed by large farm size.
PUBLIC INTEREST STATEMENT
Although teff production is an integral part of the mixed livelihood system in the dryland zones of Northeast Amhara, the sub-sector is defined by shortage of improved varieties and management practices. Therefore, to overcome these issues, numerous improved teff technologies were released and recommended for dryland areas simply based on breeders’ selection criteria. However, failure to integrate farmers’ preference traits and financial efficiency analysis on top of the agronomic achievement were main challenges that weaken the adoption rate of improved technologies. As a result, the current scale-wide evaluation and promotion of improved teff technologies was conducted to evaluate farmers’ preference traits, economic profitability and major constraints of different teff technologies in Northeast Amhara, Ethiopia; using participatory research approach.
Competing Interests
The authors declare no competing interests.
1. Introduction
Teff (Eragrostis tef), a cereal crop that belongs to the grass family Poaceae is endemic to Ethiopia and has been widely cultivated for centuries (Teklu & Tefera, Citation2005). It is the most important staple cereal crop in terms of production and consumption, which grows extensively under various climatic and soil conditions (Neela & Solomon, Citation2018). It is the most important economic crop cultivated by 43% of small holder farmers in Ethiopia, covering around 32% of the total annual acreage and 21% of the total grain production (Birrara, Citation2017). Teff is the most preferred crop in utilization not only for its higher milling efficiency and baking returns but also for its quality injera (pancake-like soft bread in Ethiopia) (Lacey & Llewellyn, Citation2005). Its straw has got several utilities; serving mainly as fodder for cattle, bedding material, mulch and local fuel source. Add to this, teff is an important source of cash income for farmers due to its higher prices from grain and biomass than other cereals (Hailu et al., Citation2003). Teff is also the leading crop in Amhara region due to its wider area coverage and production volume (Tesfaye et al., Citation2015). The region has 1.5 ton ha−1 average productivity which is by far better than the national average in similar production year (Ministry of Agriculture & Rural Development [MoARD], Citation2007). In north eastern Amhara region, where the study is undertaken, teff has got the first and second rank in its area coverage and production with average productivity of 1.3 and 1.4 t ha−1, respectively (Central Statistical Agency, Citation2017).
Ethiopian crop farming in general and the cereal subsector in particular face serious challenges. The most common challenges are lack of improved varieties, production inputs, improved management practices, soil fertility management as well as weed and pest management (Mihiretu et al., Citation2019). Despite the aforementioned importance and area coverage, teff productivity is much less at national, regional and local levels due to factors like lack of high yielding cultivars, poor management practices and low input utilization (Food and Agriculture Organization, Citation2016). Moreover, the erratic and uneven distribution of rainfall is becoming a threat to produce late maturing local teff varieties due to frequent moisture stress at flowering and grain filling stages thus leading to either lower yield or total crop failure Setotaw, Citation2011). Understanding these problems, Sekota Dry-land Agricultural Research Center has been pursuing several on-station trials to identify varieties with their production packages suiting to the agro-ecological contexts of its mandate areas. As a result, Kuncho and Zoble teff verities with their technology packages were found to be better and suiting to the study areas in terms of both adaptability and grain yield performance. Despite the efforts of science and technology which has been influencing the agricultural production system for decades, there are no as such promising changes in the life of target communities (Amanor, Citation1994). This is mainly because researches targeting on solving agricultural problems were designed and implemented on-station through researchers’ motivation, full management and follow up. Thus, the research outputs did not address farmers’ needs since the technologies’ effectiveness is measured merely in yield, that devoid farmer’ preferences, working circumstances and constraints.
Moreover, the trials were not in comparison to exiting local cultivars and production practices, as well as did not involve farmers and their preference parameters at different stages. Researchers thus lacked other most important biophysical and societal preference traits in varietal and technology selection. However, scientists believe that participatory experimentation is critical for sustainable technology promotion and transfer. Participation hence can be an effective technique in fostering awareness due to its direct experimental nature which is then helpful to discovery learning (Leeuwis, Citation2004). In recent scenario, studies show that participation helps smallholder farmers to perceive improved technologies from his/her point of view, in turn plays a vital role in shaping human practice (Leeuwis, Citation2004). Since the ultimate goal is to work on farmers’ condition, being open to all farmers will give room to extend the technologies in the extension system through social networks. Scale-wide participatory evaluation and promotion, thus helps to have extra considerations beyond biological traits.
This scale-wide participatory evaluation and promotion of improved teff technologies was thus conducted to assess and analyze the performance and profitability of improved teff technologies, the farmers’ technology preference and application constraints to the recommended package components in dryland areas of Northeast Amhara, Ethiopia.
In this study “improved teff technology” refers to using improved teff varieties with their recommended production package components (at ≥3× tilled farms, timely and row sowing, recommended fertilizer and seed rates, good weed and pest management).
2. Materials and methods
2.1. The study areas
The study was conducted in Sekota and Lasta districts of the northeast Amhara region (Figure ). Sekota district is found at an altitude of 2400 m above sea level, and has annual mean rainfall and temperature of 774.2 mm and 28.5° C, respectively. The dominant soil type of the district is loam and clay soil (Mihiretu et al., Citation2019aa). Lasta district on the other hand, located at altitude of 2400 m above sea level with the annual rainfall of 895.2 mm and average temperature of 26.2° C. The dominant soil type of the district is black sandy and loam (Woreda Office Agricultural Development [WoAD], Citation2018). The main crop types produced in the study areas are teff, wheat, faba bean and barley in descending order according to their economic importance and area coverage (Central Statistical Agency, Citation2017). Teff is produced once a year in the main rain season in relatively high and midland altitudes of the region (Tesfaye et al., Citation2015). In Sekota and Lasta districts, where the study is undertaken, teff has got first and second rank in its area coverage and production volume. It has also average productivity of 1.3 and 1.4 ton ha−1, respectively, in Sekota and Lasta districts (Central Statistical Agency, Citation2017).
2.2. Sampling and experimental procedure
In this study, two improved teff technologies (Kuncho and Zoble) with local production practice were compared and promoted in scale-wide participatory approach. Two-stage sampling technique was employed to select sample farmers. In the first stage, the north eastern Amhara region was selected to denote moisture deficit teff growing areas in North Ethiopia. In the second stage, Sekota and Lasta districts of the north eastern Amhara region were randomly selected for the study.
The sample size was determined using a formula provided by Yamane (Citation1967). The sample size for each district was determined by using proportionate sampling technique. In total, 270 farmers (90=per year and 30=per technology), who were willing to allocate 0.25 ha clustered farmland were selected for the experiment. The experiment was carried out for three consecutive production years (2015–2017), to reduce risks of seasonal variability and to raise farmers’ confidence on the technologies. Before launching the experiment, researchers organized operational platform to create awareness as well as share responsibilities among concerned stakeholders. Accordingly, main stakeholders identified and sign memorandum of understanding to part duties and responsibilities throughout the study periods (Table ). Cluster approach is preferred, because it helps to create competition among farmers in field management, pest and disease management and control. Moreover, it attracts eyes of neighbor farmers thereby inspire them to ask, observe and finally to accept the technologies (Porter, Citation1998). In order to create awareness, participant farmers were provided basic agronomic training on the improved technologies. Accordingly, the host farmers were applying the technologies as per the recommendation. Planting was in row at 15, 50 and 100 kg ha−1 seed, Urea and di ammonium phosphate (DAP) rates for improved technologies, whereas the local practice was without fertilizer at 25 kg ha−1 seed rate. Finally, extension activities like field days and diagnostic visits were held to create awareness about the technologies there by to benefit the farmers in the long run.
Table 1. Duties and responsibilities of stakeholders in technology evaluation and promotion
2.3. Method of data collection
Both quantitative and qualitative data necessary to the study were collected. The quantitative data such as grain and biomass yields were collected from sample farms using quadrant method. The profit data were then estimated from grain and biomass yields using farm gate prices of the harvesting season. Add to this, the production expenditures (total variable and fixed costs) were collected from sample farms and farmers via checklists. The total variable cost comprises of value of seeds, fertilizer, labor, pesticides among others; while the total fixed cost includes farmland rent, draft animal rent and depreciation on fixed farm tools and equipment such as plough, hoe, sickles and so on.
Furthermore, on top of identifying and counting teff technology package components utilized by sample households, their demographic, socioeconomic and institutional characteristics likely to determine the package application decision were collected using structured interview schedule. On the other hand, the qualitative data such as farmers’ reaction and preference to technologies was collected in focus group discussions (FGDs). Farmers therefore, probed and brainstormed to identify their main preference criteria in selecting best teff technologies under local context. Grain and biomass yield, vegetative performance, early maturity, seed color, disease and pest tolerance were identified as main preference parameters. Secondary data were also collected from different published and unpublished sources.
2.4. Method of data analysis
Descriptive statistics such as frequency, percentages, mean and standard deviation were used to analyze the quantitative data for the first objective. One way ANOVA was used to see if the mean yield of improved teff technologies were significantly different each other and from the local cultivar under existing practice. In addition, technological gap, extension gaps and technological index between the local and improved technologies were calculated using the formulas (1, 2, 3) (Mihiretu et al., Citation2019).
where: TG: technological gap, Ys: yield of improved technology, Yb: yield of the local practice, EG: extension gap, PY: potential yield of improved teff, TI: technological index
Technological gap is the difference between yield of improved teff technology and the local practice yield, however extension gap is the variance between the potential yield of improved teff and the yield of improved technology during the study periods. Technological gap is thus attained by using improved teff variety with its production package components such as optimum tilled farms, timely and row sowing, suggested fertilizer and seed rates, proper weed and pest management. Extension gap is the yield variation recorded due to technical application delinquency of farmers despite using improved varieties with their production package components. Potential yield is the yield record of improved teff technologies attained during technology releasing stage under full package application and thorough management of researchers. Therefore, Zoble and Kuncho improved teff technologies were recommended with the possible yield of 2070 and 2600 kg ha−1, respectively, for their respective recommendation domains.
The cost benefit analysis was also used to analyze the profitability of teff technologies across districts. Hence, the following economic variables were calculated to determine the profitability of each technology using the formulas (4, 5, 6 and 7) provided by Mihiretu et al. (Citation2019a).
Gross margin (GM) is the difference between total revenue (TR) earned and the total variable cost (TVC) incurred, but net margin (NM) is the difference between TR and total cost (TC). Total revenue is the total income realized on output produced, i.e. quantity sold multiplied by price per unit. Total variable cost is the cost that varies with changes in output, thus it is a function of output levels. It comprises the prices of seeds, fertilizer, labor, pesticides and transportation, while total fixed cost (TFC) is the cost that does not vary with outputs (land rent, draft animal rent, tools and equipment). Total cost is thus the entire expenditure for teff technologies or the sum of variable and fixed costs. The benefit cost ratio (BCR) is on the other hand, the total revenue divided by the total cost, hence when BCR is greater than 1, the business is said to be profitable.
The farmers’ preferences to teff technologies were analyzed in weighted ranking matrix. After ranking and weighting the identified parameters pair-wisely, weighted ranking matrix table was constructed. Farmers in each group then asked to compare technologies each other and give values. The values provided for each technology was counted to put scores. The scores were summed and the least sum was ranked as 1st. The rank was multiplied by the respective weight for each technology and the products were aggregated for final selection (least sum was ranked 1st) (Russell, Citation1997). Spearman’s rank correlation was used to see the degree of coincidence between farmers’ preference rank with actual value of measured attributes (Ferdous et al., Citation2016). The correlation coefficient is defined as:
where, d = difference in the ranks and n = number of phenomena ranked.
Predictors of farmers’ technology package application constraints were identified using Poisson regression model. This model is preferred because best used for modelling events where the outcomes are counts (discrete data with non-negative integer values) (Cameron, Citation2009; Dupont, Citation2002). Experience has shown that farmers are different in the amount and type of input use as a technology package. Therefore, counts of teff technology package components (viz., optimum tillage, timely sowing, row sowing, recommended fertilizer amount, proper weed and pest management) were the dependent variables since farmers may use the package components fully, by half or at lowest level. They were coded in integers, after summing the practiced component counts of each farmer from the total technology package expected to apply. The dependent variable is thus a count dependent variable measured in positive integers as 1, 2, 3, 4 and so on.
However, technology package application does not occur without influences from socioeconomic, geographical and institutional factors shaping the human-environment interaction (Azeb et al., Citation2017). In this study, farmers’ technology package application is also determined by predictor variables which are demographic, socioeconomic and institutional in nature (Tigabu & Gebeyehu, Citation2018).
These explanatory variables were selected on the basis of previous studies and summarized accordingly (Table ). Twelve explanatory variables were analyzed to identify their effects on the number of teff technology package components applied. Explanatory variables such as the household head’s sex, educational status, family size, farm experience, livestock holding, extension contact, participation in community affairs, training and credit access were hypothesized to affect the package application positively (Aynalem et al., Citation2018; Leggesse et al., Citation2018; Melesse, Citation2018). On the other hand, age and land holding status of the household head as well as distance from the nearest market center were assumed to influence technology package application adversely (Azeb et al., Citation2017; Tesfaye et al., Citation2015).
Table 2. Description of explanatory variables used in Poisson regression model
3. Results and discussion
3.1. Characteristics of sample farmers and farms
The average age of farmers in both districts was 42.5 years with farming experience of 28.5 years in average. Similarly, 14.5% of farmers were female headed but most farmers were engaged to marriage. The average family size was 5.3, indicating that most households had adequate labor for technology package application. Educational status is expected to determine farmers’ technology application, hence 56.5% of participants were illiterate (Table ). Besides, 99.2% of the participant farmers got training, but 16.8% of them agreed that the training was not sufficient for technology application as it was provided for short time. The productivity of crop technology directly linked to the agronomic status of farmlands under cultivation. The agronomic findings suggested that ‘three times tilling is an optimum level’ for teff technology package (Abate et al., Citation2017). Accordingly, results from this study indicated that 89.8% of farmers were tilling at optimum level. Those who tilled under the optimum level were due to limited access to draft animals at critical periods. Indeed, 98.4% of farmers were planting on the exact time and 88.6% of them had very good weed management.
Table 3. Characteristics of participant farmers in teff technology evaluation and promotion in Northeast Amhara region, n = 270
3.2. Performance and economic profitability of improved teff technologies
Yield is farmers’ major criterion in any crop technology adoption (Mihiretu et al., Citation2019a). Hence, the comparison between two improved teff technologies and one local cultivar under the existing practice was made and described below (Table ). An average grain yield of 1620 kg ha−1 was obtained from zoble improved teff technology in Sekota district. However, in Lasta district the highest yield was recorded from ‘kuncho’ improved teff technology with mean yield of 1830 kg ha−1. Therefore, ‘Zoble’ and ‘Kuncho’ improved teff technologies ensured 710 and 530 kg ha−1 yield increment over the local practice in Sekota and Lasta districts, respectively (p≤0.05). Using ‘Zoble’ and ‘Kuncho’ teff technologies thus had 78.0% and 40.8% yield advantage over the local practice in the districts, accordingly. As dry-land area, shortage of animal feed is farmers’ critical problem in the study districts hence the improved teff technologies were better in biomass yield than the local teff (Table ). Improved ‘Zoble’ and ‘Kuncho’ teff technologies thus had the biomass yield advantage of 24.8% and 30.1% over the local in Sekota and Lasta districts, respectively.
The extension gap of 450 and 770 kg ha−1 was recorded from Zoble and Kuncho improved teff technologies in Sekota and Lasta districts, respectively. This yield gap was recorded due to farmers’ technical delinquency in technology application despite using improved varieties with their production packages. This finding revealed that teff productivity problem could be overwhelmed through efficient extension service provision to farmers on top of providing technical support during technology package application. On the other hand, the technological gaps of 530 and 710 kg ha−1 in Sekota and Lasta districts, respectively, could be closed through the application of improved teff technologies as per the recommendation. Moreover, the technological indexes of 25.6% and 27.3% in Sekota and Lasta districts, respectively, provides an evidence as there are still rooms for teff productivity enhancement. The yield variability across treatments and districts is may be due to the technological and agro-ecological differences. Therefore, this finding agrees with the conclusions of Mihiretu et al. (Citation2019a) who states that the lower technological index describing the possibility of replicating the genetic yield potential of crop technologies in the real farm situation since the technological index postulates feasibility of technologies on the farmer’s field (Ferdous et al., Citation2016).
Given the farm gate price, benefit-cost ratio was computed on hectare basis hence farmers were able to generate the highest total revenue of 43188ETB from Zoble, followed by Kuncho and local teff technologies in Sekota district. However, in Lasta district, the highest total revenue was 51709.7ETB obtained from Kuncho improved teff technology (Table ). Except the local practices, there was no difference in costs among improved technologies within district. The cost of local practices across location was low due to lack of improved technology package utilization. The average net profit margin that farmers could make in Sekota and Lasta district was 31588.0 and 40304.7ETB/ha from Zoble and Kuncho improved technologies, respectively.
Therefore, farmers could earn an additional net income of 18068.4 and 12528 ETB/ha from the use of Zoble and Kuncho improved teff technologies over the local practice in Sekota district. However, using Kuncho improved teff technology in Lasta district could provide additional net income of 16059.3 ETB/ha. The benefit-cost ratio of Zoble and Kuncho improved teff technologies in Sekota and Lasta districts was 3.7 and 4.5 ETB/ha, respectively. In other words, farmers can generate 3.7 and 4.5 ETB benefit from Zoble and Kuncho technologies for 1 ETB investment on cost of production. Despite the benefit-cost ratio result shows that using all teff production practices is economically profitable, adopting the improved teff technologies was by far better than the local practice across locations.
This implies that with the context of geometric growth of human population and an arithmetic growth of agricultural production within the constant cultivable land, improving production through the use of improved technologies would be the best solution to improve food security concerns of the study areas.
Table 4. Mean grain and biomass yield of different teff technologies across districts
Table 5. Benefit-cost analysis of improved teff technologies and the local practice
3.3. Farmers’ preference and demand to improved teff technologies
The aim of participatory technology evaluation and promotion is not only maximizing yield but to improve actors’ involvement in selecting technologies that fulfill their preference for sustainable technology diffusion (Abate et al., Citation2017). Therefore, in both districts, farmers identified six common preference parameters to select the best teff technology. The parameters were weighted according to their importance to be used as comparison, then technology with greater percentage from the total was selected as primary choice. The overall weighted ranking matrix result shows that Zoble and Kuncho teff technologies were the first and second choices of farmers in Sekota district and vice versa in Lasta district (Table ). The study districts are agro-ecologically classified as dry-land and described by recurrent rainfall shortage; hence farmers ranked earliness as primary preference parameter. Among parameters, farmers preferred disease and pest tolerance before grain yield in Lasta district, because the area has a long history of shoot fly infestation. On the other hand, in Sekota district farmers are agro pastoral and require higher biomass for livestock feed thus it has got greater credit as parameter next to grain yield. This result is in harmony with the findings of Abate et al. (Citation2017), affirming that higher plant population is usually correlates with greater biomass yield (i.e. critical to the livestock production subsector).
Moreover, Spearman’s rank correlation to show the degree of coincidence between farmers’ preference rank and actual values rank of technologies for grain yield and biomass yield (Table ). Thus, the grain and biomass yield ranks were coincided by 50% and 75% as well as 50% and 25% in Sekota and Lasta district, respectively.
where, rs= correlation coefficient d = difference in the ranks assigned to the same phenomenon and n = number of phenomena ranked.
Table 6. Summary of farmers’ technology evaluation criteria and preference across districts
Table 7. Correlation between farmers’ preference rank and actual measured values rank
Table 8. Estimation of factors influencing teff technology package application, n = 270
3.4. Factors that determine improved teff technology package application
Among post-estimation tests that used to check the satisfaction of assumptions of poisson regression model, goodness-of-fit, heteroscedasticity, omitted variables and multicollinearity are vital and must be reported with the model outputs. Therefore, a significant likelihood ratio or non-significant test result (Table ) are indicators of a good fitting model. The test for heteroscedasticity suggests that errors are of the same variance hence the null hypothesis errors have constant variance is accepted. Likewise, the null hypothesis no omitted variable in the model is accepted since it has no omitted variable bias. The test of multicollinearity with mean variance inflation factor (VIF) of 1.6, suggests that no multicollinearity problem among independent variables (see details on Tables –A on the appendices). The poisson regression result thus presents that farmers were using 5.27 teff technology package components in average. To describe explicitly, 52.5%, 31.8% and 15.7% of farmers were using 4, 5 and 6 package components, respectively, out of six full package components. Factor variables assumed to influence teff technology package application are multifactorial, entailing demographic, socioeconomic and institutional features of the farmers (Table ). Based on empirical literature, 12 variables hypothesized to impact technology package application were examined in poisson regression model. As a result, according to the model output, five variables (sex, family size, land holding size, training and credit access of the household head) were statistically significant variables that influence teff technology package application. Except land holding size of the household head, the rest of factor variables were affecting the technology package application positively.
3.5. Sex of the household head
Being male of the household head, positively affects technology package application (p < 0.05), so that being male of the household head is associated with increasing teff technology package application by the multiple exponential of 0.016. In other words, the marginal effect result describes that male household heads are 8.4% likely to apply the teff technology package. This may be due to the fact that male farmers have better exposure and contact to extension service providers and information, while women are culturally assigned for domestic activities. This finding is thus in line with Kebede (Citation2013), affirming that male farmers are more likely to access information on technologies and pleased to take risks than their counterpart female farmers.
3.6. Family size of the household head
As expected, family size of the household (measured in adult equivalent) positively and significantly influenced the farmers’ decision to apply teff technology package components at 5% or lower significance level. Therefore, one more increase of the family member is associated with increasing teff technology package application by multiple exponential (0.013). The marginal effect result also shows that as the number of family size increases by one more person, the probability of farmer’s teff technology package application increases by 6.9%, all other factors held constant. This is may be because teff technology packages are unsurprisingly labor intensive and time consuming in nature. Therefore, farmers who have more family size have access to family labor, hence likely to produce more quantity of teff which in turn increases the probability of technology package application. This result is in agreement with the finding of Tazeze et al. (Citation2012), stating that households who have higher labor force are lucky to apply the full technology packages with an attempt to earn income and ease the food gap pressure imposed by larger family size.
3.7. Land holding size of the household head
It refers to the total hectare of land owned by farmers and was hypothesized to affect teff technology package application adversely and significantly. So that, the model result showed that size of land holding affected technology package application negatively at 10% or lower significance level. The poisson regression result thus depicts that one more hectare of land is associated with teff technology package application increasing by the multiple exponential (0.011). Accordingly, as farmers land holding increases by one hectare, the probability of teff technology package would decrease by 6%. The possible reason is that farmers may think of advantage from economic scale of production rather than land intensification. This result is in conflict with the findings of Burney (Citation2014), who expected that households with larger land are expected to work on their farm intensively rather than going for alternative livelihoods.
3.8. Training access of the household head
Access to training on improved teff technology package had also positive and significant effect on increasing technology package application decision at 10% or lower significance level. Accessing training on improved technologies is thus associated with increasing package application by multiple exponential (0.032). On the other hand, access to training on teff technology increases the farmers’ probability of package application by 17.5%. This suggests the dissemination of technology information via training and farm level education will increase the likelihood of teff technology package application. This finding is covenant with the result of Mihiretu et al. (Citation2019b), stating that training improves the probability of technology application and adaptation by increasing the knowledge and awareness levels of farmers.
3.9. Credit access of the household head
It is a dummy variable concerned with the effect of credit access on teff technology package application. Therefore, the model result depicts that farmer’s access to credit had a positive effect on the technology package application at 5% or lower significance level. This is because farmers who were accessing credit were solving their financial problem of purchasing package inputs such as fertilizer, peptides and improved seeds. The coefficient showed that farmers who accessed credit were likely to apply teff technology package by 2.9%. This result is in line with the finding of Efa et al. (Citation2016), who indicated that access to credit positively and significantly influences farmer’s teff technology package application.
4. Conclusion and recommendation
Findings of the current study confirmed that improved teff technologies provided higher grain and biomass yield than the local teff production practice in both study districts. As a result, Zoble and Kuncho improved teff technologies ensured 78% and 40.8% yield advantage over the local practice in Sekota and Lasta districts, respectively. Using Zoble and Kuncho improved teff technologies also conveyed an additional net income of 18068.4 and 16059.3ETB per hectare over the local practice in Sekota and Lasta districts, in the same order. The cost-benefit ratio outcome revealed that adopting these improved technologies could make 3.7 and 4.5ETB return for each 1ETB investment on the costs of production, accordingly. Likewise, the overall weighted ranking matrix shows that Zoble and Kuncho improved teff technologies were the primary choices of farmers in Sekota and Lasta districts, respectively.
Furthermore, the poisson regression model result presents that farmers were applying on average 5.24 components from the total (6) teff technology packages. Explicitly, 52.5%, 31.8% and 15.7% of farmers were applied 4, 5 and 6 package components, respectively. The empirical finding also shows that variables such sex, family size, training and credit access of the household head were affecting teff technology package application positively, at 10% and lower significant levels. On the other hand, land holding size of the household head, was influenced the technology package application negatively and significantly. This implies that an increase or decrease of these variables would cause an increase or decrease in the application of technology package components, correspondingly.
Therefore, Zoble and Kuncho improved teff technologies should further diffused to respective districts and other similar locations to enhance teff production and productivity. The empirical result, on the other hand, renders that household heads that are male in sex, ensured more family size, with training and credit access were applied the teff technology package components at different levels. As a result, supplying access to package components and agricultural inputs for female headed households on top of providing eminence training and credit services will create equity among farmers. This in turn will play a pivotal role in enhancing teff technology package application in the districts.
Nevertheless, higher land holding by the household head reduces teff technology package application, so that providing labor saving technologies is worthwhile to make use of the improved technology package components in the production system. In this regard, the agricultural mechanization research should take sizable duty of designing labor replacing apparatuses in order to ease the labor pressure imposed by large farmland size.
Acknowledgements
The authors’ are grateful to farmers and agricultural experts for their determination throughout the study processes.
Additional information
Funding
Notes on contributors
Ademe Mihiretu
Ademe Mihiretu is an Ethiopian citizen and graduate of Rural Development (MSc), Haramaya University (honours). Currently, He is a full time researcher of Socioeconomic and Agricultural extension in Sekota Dry-land Agricultural Research Center, Ethiopia. Ademe has published a significant number of research articles in reputable journals worldwide. He has also reviewed manuscripts invited from reputable publishers (Taylor & Francis, Springer, Sage), related to his field of specialization.
References
- Abate, B. E., Solomon, C. H., Tebkew, D. E., Kebebew, A. S., & Zerihun, T. A. (2017). Lead farmers approach in disseminating improved tef production technologies. Ethiopian Journal of Agricultural Sciences, 27(1), 25–19. https:// www. ajol.info/index. php/ejas/article/view/150333
- Amanor, K. S. (1994). Ecological knowledge and the regional economy: Environmental management in the Asesewa district of Ghana. Development and Change, 25(1), 4167. https://doi.org/10.1111/dech.1994.25.issue-1
- Aynalem, S., Nand, K., & Seema, S. (2018). Agricultural technology adoption and its determinants in ethiopia: a reviewed paper. Asia Pacific Journal of Research, 1(15), 2347–4793. https:// www.apjor.com
- Azeb, B., Tadele, M., & Latha, D. A. (2017). Determinants of smallholder farmers in teff market supply in Ambo district, West Shoa Zone of Oromia, Ethiopia. International Journal of Advanced Research in Management and Social Sciences, 6(2), 133–140. http:// www.garph.co.uk/
- Birrara, E. (2017). Teff production and marketing in Ethiopia. A Journal of Radix International Educational and Research Consortium, 6(4), 2250–3994. doi:10.7176/JESD/10-3-06
- Burney, J. (2014). Climate change adaptation strategies for smallholder farmers in the Brazilian Sertão’. Climatic Change, 126(1–2), 45–59. https://doi.org/10.1007/s10584-014-1186-0
- Cameron, A. C. (2009, March 28). Advances in count data regression talk for the applied statistics workshop, Southern California Chapter of the American Statistical Association, held at University of California - Los Angeles. http://cameron.econ.ucdavis.edu/racd/count.html
- Central Statistical Agency. (2017). Agricultural sample survey 2016/2017; report on area and production of crops. volume IV. The Ethiopian Statistical Agency annual bulletin.
- Dupont, W. D. (2002). Statistical modeling for biomedical researchers: A simple introduction to the analysis of complex data. Cambridge Press.
- Efa, G., Degye, G., Tinsae, D., & Tadesse, K. (2016). Determinants of market participation and intensity of marketed surplus of teff producers in Bacho and Dawo districts of Oromia State, Ethiopia. Journal of Agricultural Economics and Development, 5(2), 020–032. http:// academeresearchjournals. org/journals/jaed
- Ferdous, Z., Datta, A., Anal, A. K., Anwar, M., & Khan, M. R. (2016). Development of home garden model for year round production and consumption for improving resource-poor household food security in Bangladesh. NJAS - Wageningen Journal of Life Sciences, 78(1),103-110. https://doi.org/10.1016/j.njas.2016.05.006
- Food and Agriculture Organization. (2016). Database of agricultural production. FAO Statistical Databases (FAOSTAT). http://faostat.fao.org/default.aspx
- Hailu, T., Kebebew, A., Fufa, H., Tiruneh, K., & Tesfaye, T. (2003). Heritability and genetic advance in recombinant inbred lines of Teff (Eragrostis Teff). Euphatica, 131(1), 91–96. https://doi.org/10.1023/A:1023009720870
- Kebede, G. (2013). Spatial and temporal uncertainty of rainfall in arid and semi-arid areas of Ethiopia. Science Technology and Arts Research Journal, 2(4), 106–113. https://doi.org./10.4314/star.v2i4.19
- Lacey, T., & Llewellyn, C. (2005). Eragrostis Teff as a specialized niche crop. No. 42/2005. State of Western Australia. www.agric.wa.gov.au
- Leeuwis, C. (2004). Communication for rural innovation: Rethinking agricultural extension (3rd ed.). Blackwell.
- Leggesse, D., Michael, B., Adam, O., Hagos, K., & Girma, T. (2018). Duration analysis of technological adoption in Ethiopian agriculture. Journal of Agricultural Economics, 55(3), 613–631. https://doi.org/10.1111/j.1477-9552.2004.tb00117.x
- Melesse, B. (2018). A review on factors affecting adoption of agricultural new technologies in Ethiopia. Journal of Agricultural Science and Food Research, 9(3), 226. http://www.longdon.org/open-access/
- Mihiretu, A., Asefa, N., & Wubet, A. (2019). Participatory evaluation of sorghum technologies in the marginal dryland zones of Wag-lasta, Ethiopia. Cogent food and Agriculture,5 (1), 1671114. https:// doi.org/10.1080/23311932.2019.1671114.
- Mihiretu, A., Asresu, M., & Wubet, A. (2019a). Participatory assessment of lentil (Lens culinaris Medik.) production practices in marginal dry lands of Wag-lasta, Ethiopia. Archives of Agriculture and Environmental Science, 4(3), 288–294. https://doi.org/10.26832/24566632.2019.040305
- Mihiretu, A., Eric, N., & Lemma, T. (2019b). Determinants of adaptation choices to climate change in agro-pastoral dry lands of Northeastern Amhara, Ethiopia. Cogent Environmental Science, 5(1), 1636548. https://doi.org/10.1080/23311843.2019.1636548
- Ministry of Agriculture & Rural Development (MoARD), (2007). Crop Development Department, Crop Variety Register. Issue No. 10.
- Neela, S., & Solomon, W. (2018). Review on structural, nutritional and anti-nutritional composition of Teff (Eragrostis tef) in comparison with Quinoa (Chenopodium quinoa Willd.). Cogent Food & Agriculture, 4(1), 1546942. https://doi.org/10.1080/23311932.2018.1546942
- Porter, M. (1998). Clusters and the new economics of competition. Harvard Business Review megazine. http://hbr.org/product/clusters-and-the-new-economics-of-competition/an/98609-PDF-ENG
- Russell, T. (1997). Pair wise ranking made easy. In PLA notes No 28, Methodological complementary (pp. 25–27). International Institute of Environmental and Development (IIED), London.
- Setotaw, F. (2011). Technological change & economic viability in tef production. Proceeding of the Second International Workshop (pp 266–284), November 7-9, 2011. Debre Zeit, Ethiopia.
- Tazeze, A., Haji, J., & Ketema, M. (2012). Climate change adaptation strategies of smallholder farmers: The case of Babilie district, East Harerghe Zone of Oromia Regional State of Ethiopia. Journal of Economics and Sustainable Development, 3 (14), 2222–2855. http:// www.iiste.org
- Teklu, Y., & Tefera, H. (2005). Genetic improvement in grain yield potential and associated agronomic traits of tef (Eragrostis Tef). Euphytica, 141(3), 247–254. https://doi.org/10.1007/s10681-005-7094-7
- Tesfaye, W., Abdissa, T., & Berecha, G. (2015). Economic analysis of tef yield response to different sowing methods: Experience from Illuababora Zone, Ethiopia. Journal of Economics and Sustainable Development, 6(1), 56–60. Corpus ID: 56314781.
- Tigabu, D. G., & Gebeyehu, M. F. (2018). Agricultural extension service and technology adoption for food and nutrition security: Evidence from Ethiopia. FARA Research Report, 3(4), 30. doi: 10.22004/ag.econ.277332.
- Woreda Office Agricultural Development (WoAD). (2018). A working manual prepared by regional advisory experts. 121–126. Bahir Dar, Ethiopia.
- Yamane, T. (1967). Statistics: An introductory analysis (2nd ed.). Harper and Row.
Appendix A
Tests for goodness-of-fit
Table A1. Non-significant test results are indicators of a good fitting model
Appendix B
Tests for heteroscedasticity
The test for heteroscedasticity after OLS suggests that the errors are of the same variance. The null that the errors have constant variance is accepted.
Ho: constant variance
Variables: fitted values of techapp
Table A2. Breusch-pagan/Cook-Weisberg test for heteroscedasticity
Appendix C
Testes for omitted variables
The null that there is not omitted variable in the model is accepted suggesting that the model has no problem of omitted variable bias.
Table A3. Ramsey RESET test using powers of the fitted values of techapp Ho: model has no omitted variables
Appendix D
Tests for multicollinearity
The test for multicollinearity reported below suggests that there is no serious problem of multicollinearity among explanatory variables because the mean VIF is about 1.6.
Table A4. Multicollinearity test of explanatory variables