Abstract
Sorghum [Sorghum bicolor (L.) Moench]’s production and productivity is by far lower than its potential. Knowledge on the extent and pattern of genetic variability is prerequisite important for generating superior varieties of different objectives for sorghum improvement. Therefore, this research was conducted to quantify genetic variability, heritability, and genetic advance as percent of mean (GAM). A total of 258 advanced lines including a local cultivar “Deber” were evaluated in 2016/17 at Humera Agricultural Research Center, Northern Ethiopia. Alpha lattice design was used with two replications and three blocks were nested within a replication. Experimental results revealed significant difference for yield and yield attributing traits among genotypes. Higher range of genotypic coefficient of variation (GCV) (3.13–37.44) and phenotypic coefficient of variation (PCV) (5.11–38.08) values were observed. Yield per panicle (YPP) (97%) and harvest index (HI) (31%) were the highest and lowest broad sense heritable (H2)traits, respectively. Higher estimate of GAM observed for YPP (75.93%) followed by head weight (HW) (62.36%). Moreover, plant height (PH) (23.51, 83, and 44.22), YPP (37.44, 97, 75.93), HW (31.88, 89, 62.36), biomass (BM) (29.21, 74, 51.94), and grain yield (GY) (32.99, 78, 60.25) were traits with higher estimate of GCV, H2 (%) and GAM, respectively. This study showed wider genetic variability in the tested genotypes. Therefore, hybridization ad selection on these genotypes for a desired traits with high (H2) coupled with higher GCV and GAM will be effective to develop superior sorghum cultivars.
PUBLIC INTEREST STATEMENT
Technology generation in crop improvement for food security starts by the assessment of existence and magnitude of genetic variability in a given commodity that is very helpful for the breeder to improve crops. The improvement and access for improved varieties in sorghum is not yet much satisfactory as the crop has dual-purpose benefit (human food consumption and animal feed) and food security focused crop.
We estimate the genetic variability of 258 advanced lines of sorghum and found that these advanced lines were highly variable and heritable in their PH (cm), panicle length (PL) (cm), BM yield (kg), YPP (g) and GY (kg). Therefore, result implies improvement through selection breeding for improved variety generation is effective. The advanced lines of sorghum could also be used as an input for improved varieties generation in the future sorghum-breeding program.
Competing interests
The authors declare no competing interests.
1. Introduction
Sorghum [Sorghum bicolor (L.) Moench], is the fifth most important cereal crop in the world after wheat, rice maize, and barley (Ritter et al., Citation2007). It is food security focused crop mostly used as staple food for most of the Sub-Saharan Africa and South Asian countries (Bibi et al., Citation2010). It is one of the climates and drought resilient crop (Reddy et al., Citation2011). Although it is highly preferred to grow in the low lands where moisture is limiting, it has a very wider geographical adaptation than any other cereals, which covers almost all agro-ecologies in Ethiopia (Tesso et al., Citation2007). Most produced sorghum are globally used as animal feed, source of energy (breads and pasta) and source of industry (buskut preparations) (Mckevith, Citation2004) while locally consumed at household levels as human food, animal feed, source of industry (beer), fencing materials, preparation of other traditional foods and beverages like “Tella” and “Areke” as well as consuming as boiled and roasted sorghum (Legesse, Citation2015). This author also suggested its importance for injera making quality in Ethiopia is ranked second next to tef in which its grain is mostly used for local markets. However, the production and productivity of sorghum, nationally as well as globally is by far lower than its potential due to some biotic and abiotic stresses mainly striga and drought. The national average productivity is 2.3 tons/ha (CSA, Citation2016) while globally 4.89 ton/ha in United States (USDA, Citation2017). As Ethiopia is center of origin and diversity (Doggett, Citation1988); (De & Harlan, Citation1971) and Khoury et al., Citation2016) for sorghum, out of the 168,500 sorghum accessions conserved in different parts of the world (Billot et al., Citation2013), 21,932 sorghum accessions are from Ethiopia. Of which 9432 sorghum accessions conserved in the Ethiopian institute of biodiversity, 7000 in the US national gene bank system and 4500 accessions in the ICRISAT gene bank (Adugna, Citation2014). In addition, Sorghum has high genetic variability in Ethiopia, Sudan and Eritrea (Gebrekidan, Citation1982). Different scientists like (Tomar et al., Citation2012), (Amare et al., Citation2015) and (Yaqoob Muhammad, Citation2015) also discussed the presence of significant range of genetic variability among tested genotypes of sorghum. However, investigations on area production coverage in Ethiopia showed, more than 95% of sorghum production area were covered by the sorghum land races (Adugna, Citation2014) and the use of improved varieties is limited. This is implied that the Ethiopian breeding program and all the executed research activities in this area could not yet utilize effectively the available sorghum genetic variability for sorghum improvement and do not yet still improve the economically important stress in sorghum. (Rao & Patil, Citation1996) suggested that phenotypic selection according to desirable traits with higher heritability coupled with higher genetic advance (GA) is effective for the success of breeding to develop superior genotypes for sorghum improvement. Therefore, searching for additional genetic variability and maximum exploiting of the existing genetic variability for selection and development of superior and high yielding genotypes can overcome the low yielding sorghum. As (Godbharle et al., Citation2010)’s conclusion, quantifying the knowledge on the extent and pattern of genetic variability is prerequisite important to generate the superior varieties for different objectives of sorghum improvement, this research was conducted to quantify genetic variability, heritability and GA for the grain yield (GY) and yield components in the advanced lines of sorghum.
2. Materials and methods
2.1. Study area description
Field experiment was conducted at Humera Agricultural Research Center (HuARC), Tigray, northern Ethiopia in 2016/17 main growing season. It is located at 14°15ʹ N latitude and 36°37ʹ E longitude with an altitude of 609 m.a.s.l. The mean annual rainfall was 581.2 mm and temperature ranges from 17.5 °C in July to 42 °C in April. Most of the rainfall is concentrated during summer (June—September). The dominant soil type is black Vertisol and characterized by very deep (>150 cm) clay texture and (40-60%) clay content with low OM content (<2%). The location is characterized with its hot to warm semiarid plain of sorghum growing areas. Hot temperature, erratic and poor distribution of rainfall coupled with high evapo-transpiration makes the location vulnerable to terminal moisture stress.
2.2. Experimental materials description
258 advanced lines generated from 25 founders brought from all sorghum growing countries in the world, which is developed by Dr. Prof. Gebisa Ejeta in 1986 at Purdue University, USA, were evaluated with 1 local cultivar called “Deber.” These lines were advanced through self-pollination of single seed descent at the sixth generations. Then after exposed to performance evaluation of their genetic variability.
2.3. Field management and experimental design
Alpha lattice design with two replications and three blocks nested within replication were used. Single row planting of entries in 0.75, 0.20, 1, and 2 m spacing between rows, plants, block within replication and replications, respectively, were applied. Three seeds in 0.20 m difference were sown, followed by appropriate thinning at around née height. Experimental units received the same amount and rate of 100 kg/ha NPS and 50 kg/ha Urea fertilizers as per the recommendation for dry lowland areas (HuARC, 2009 unpublished).
2.4. Data collection
The major phonological and growth parameters yield and important yield components were recorded using the appropriate procedure. Plant and plot based data was collected. Plant-based data were collected from randomly selected and representative five plants in the plot. While the plot-based data were collected from the whole harvestable plot. Days to flowering (DF), Days to maturity (DM), Plant height (PH) (cm), Panicle length (PL) (cm), Panicle exertion (cm), Head weight (HW) (g), Yield per panicle (YPP) (g), Thousand seed weight (TSW) (g), Biomass (BM) yield (ton/ha) and GY (kg/ha) were some of the recorded quantitative data collected in the study.
2.5. Analysis of variance
Analysis of variance was computed according to the methods proposed by (Gomez & Gomez, Citation1984) using Genstat statistical software version 17.1 (Payne, Citation2009). It considers the block term as nested in the replication (Block/Rep). Best linear unbiased predictor (BLUP) means were estimated using multivariate mixed model (REML) spatial analysis considering the Block/Rep + treatment as random effect for special correction of the nearest block errors to avoid the biased estimate of variance components at 5% level of significance (Vargas et al., Citation2013). Tested genotypes were considered to be significant at (P ≤ 0.05) level of significance. Least significant difference [LSD (±)] and at (P ≤ 0.05) level of significance was used for mean comparison.
Phenotypic, genotypic and environmental variance components and their coefficients of variation were estimated based on the methods detailed in (Burton & Devane, Citation1953). Broad sense heritability (H2) and genetic advance as percent of mean (GAM) were also estimated according to the formula in (Allard, Citation1960).
3. Results and discussion
3.1. Analysis of variance
The Analysis of variance (Table ) for GY (kg/ha), BM (t/ha), days to flowering (DF)(days), DM (days), PH (cm), PL (cm), TSW (g), YPP (g), HW (g) and harvest index (HI) showed highly significant difference among the tested genotypes at (P < 0.01) level of significance. The result indicates, the tested genotypes were different in their potential to perform variable characteristics at the specified location. Similar highly significant different in genetic variability study of sorghum was reported by (Jain et al., Citation2010), (Tariq et al., Citation2012) and (Amare et al., Citation2015).
Table 1. ANOVA mean squares for 10 agronomic traits of sorghum advanced lines at Humera
GY (746.40–5004.56 kg/ha), BM (5.37–27.29 t/ha), PH (89.29–278.15 cm), YPP (16.04–163.17 g) and HW (27.45–190.54 g) showed wider range of mean performance (Table ). Exhibiting wider range of mean indicates that, the tested genotypes had experienced wider genetic and morphological variability. The result suggested that exploiting the wider genetic variability in the tested genotypes as source of breeding material for trait of interest improvement for different objectives could boost sorghum production and productivity. (Tariq et al., Citation2012) and (Abraha et al., Citation2015) in line with the current study had reported higher range of variability in PH.
3.2. Variance components
Genotypic variance (δ2 g), phenotypic variance (δ2p), environmental variance (δ2e), broad sense heritability (H2) genotypic coefficient of variation (GCV), phenotypic coefficient of variation (PCV) and GAM for yield and yield contributing traits of the sorghum advanced lines are presented in Table . Estimated variance components for the measured traits revealed that the PCV was higher in magnitude than the GCV, which implies that environmental effect influenced expression of the trait. The magnitude of GCV and PCV for the measured traits in the current study was in between a range of 3.13 and 5.11 for DM to 37.44 and 38.08 for YPP, respectively. It indicates the presence of substantial broad based genetic variability.
Table 2. Variance estimation of 10 agronomic traits in the sorghum advanced lines
According to (Deshmukh et al., Citation1986), GY, YPP, HW, BM and PH had higher GCV and PCV value that ranges in between 23.51 and 38.08. The result indicated that tested genotypes had greater and effective possibility of sorghum improvement if these traits are considered during selection and hybridization. (Amare et al., Citation2015), (Abraha et al., Citation2015) and (Ranjith et al., Citation2017) reported similar higher GCV and PCV value for GY, BM and PH of sorghum.
Moreover, moderate genotypic and PCV ranging from 13.69 to 17.34 were recorded for PL, TSW and HI indicating acceptable improvement could be obtained through selection of the above traits. On the contrast, DF and DM had recorded low GCV and PCV. This is to mean that, DF and DM contributes low magnitude of heritable genetic (additive) factor to the next generation, which indicates no need of investment to improve these traits aiming for sorghum improvement. (Ranjith et al., Citation2017) and (Chavan et al., Citation2011) in agreement with the current study reported low GCV and PCV value for DF.
3.3. Heritability (H2)
The result of broad sense heritability estimation (Table ) revealed that the highest heritability in broad sense (0.97) was obtained for YPP and the lowest (0.31) recorded fromHI. According to (SINGH, Citation2001) classification, PH (0.83),.TSW (0.89), HW (0.89) and YPP (0.97) were classified under very high heritability. The heritability estimation is indicating the presence of positive response of sorghum improvement through selection of these traits because of their higher heritability. High heritability estimate for PH, YPP and GY in lined with the current study were reported by (Ranjith et al., Citation2017; Al-Tabbal & Al-Fraihat, Citation2011; Seetharam & Ganesamurthy, Citation2013; Dhutmal et al., Citation2014). Perhaps, (Johnson et al., Citation1955) and (Udeh & Ogbu, Citation2011) suggested that, “only high broad sense heritability doesn’t always provide high prediction of genetic gain to ensure effective selection for improvement rather higher heritability coupled with higher estimate of GCV and GAM.” This suggestion implies that, these traits to be effective for selection for ensuring GY improvement in sorghum; they have to be supported with the estimate of higher GCV and GAM. Furthermore, GY (0.78), BM (0.74), DF (0.78) and PL (0.71) also classified under moderately high heritability while DM (0.37) and HI (0.31) recorded low broad sense heritability. Its implication is making the effectiveness of selection breeding (breeding success) difficult due to the contribution of non-additive genetic effect to the phenotypic expression of traits. Similar low heritability was reported by (Chavan et al., Citation2010) for DM in sorghum.
3.4. Genetic advance as percent of mean (GAM)
The GAM was estimated (Table ) and ranged from 3.95% for DM to 75.93% for YPP. According to Johnson et al. (Citation1955), YPP (75.93), GY (60.25), HW (62.36), BM (51.94), PH (44.22), TSW (26.62), PL (25.32) and HI (22.08) were classified under traits with high GAM. This showed most of the traits measured were under higher control of additive genetic effect while the influence of non-additive effect was minimal. The result indicated that planning to improve sorghum through selection of the above traits is suitable breeding procedure because of its higher estimate of GAM. For the traits PH, PL, GY and YPP in sorghum, similar higher estimate of GAM were reported by (Ranjith et al., Citation2017). Only two traits namely DF (15.76) and DM (3.95) were classified under moderate and low GAM, respectively.
As far as the use of traits as base population for effective selection breeding according to (Johnson et al., Citation1955) and (Udeh & Ogbu, Citation2011) is important, in the current study, the PH (23.51, 83, 44.22), YPP (37.44, 97, 75.93), HW (31.88, 89, 62.36), BM (29.21, 74, 51.94) and GY (32.99, 78, 60.25) were traits with higher estimate of GCV, broad sense heritability in percent (H2) and GAM, respectively. The result indicates these traits were controlled with additive genetic effect and had heritable variability in the tested genotypes. (Shinde et al., Citation2010) and (Sabiel et al., Citation2016) reported high heritability coupled with high GAM in sorghum genetic variability study.
4. Summary and conclusion
Tested genotypes in the current study showed statistically high significant difference at (P < 0.01) level of significance revealing presence of substantial amount of genetic variability. It confirms a positive response for the effectiveness of selection based on the traits with high and medium PCV and GCV values for trait of interest improvement. As heritability estimation provides prediction of expected progress for progeny/traits expression through selection, PH (0.83), TSW (0.89), HW (0.89) and YPP (0.97) are the higher heritable traits. In addition, PH (83, 23.51, 44.22), YPP (97, 37.44, 75.93), HW (89, 31.88, 62.36), BM (74, 29.21, 51.94) and GY (78, 32.99, 60.25) were the traits with higher estimate of broad sense heritability in percent (H2) coupled with higher estimate of GCV and GAM, respectively. Therefore, these traits are effective for selection breeding to be used as base population due to their higher heritable characteristics coupled with higher estimate of GCV and GAM in the study.
Acknowledgements
The authors would like to acknowledge Tigray Agricultural Research Institute (TARI), Humera Center (HuARC), Haramaya University, Integrated Striga control (ISC) project for their financial support and facilitating other logistics in the accomplishment of this research work. The author’s special thanks also goes to Yemane Tsehaye (PhD), Megan Fenton and all Humera Agricultural Research Center (HuARC) staff for their special help in data analysis and data handling.
Disclosure statement
The authors declare that they have no conflicts of interest.
Additional information
Funding
Notes on contributors
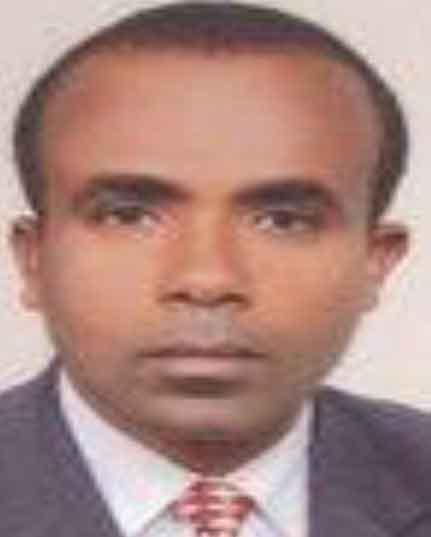
Gebremedhn Gebregergs
Mr. Gebremedhn Gebregergs has been an associate researcher in cereal crops in Tigray Agricultural Research Institute (TARI), Humera center for the past 5 years. Currently, he is working with sorghum breeding and coordinating the sorghum breeding program in the institute.
References
- Abraha, T., Githiri, S. M., Kasili, R., Araia, W., & Nyende, A. B. (2015). Genetic variation among sorghum (Sorghum bicolor L. Moench) landraces from eritrea under post-flowering drought stress conditions. American Journal of Plant Sciences, 6(9), 1410. https://doi.org/10.4236/ajps.2015.69141
- Adugna, A. (2014). Analysis of in situ diversity and population structure in Ethiopian cultivated Sorghum bicolor (L.) landraces using phenotypic traits and SSR markers. SpringerPlus, 3(1), 212. https://doi.org/10.1186/2193-1801-3-212
- Allard, R. (1960). Principies of plant breeding John Wiley. John Wiley and Sons. Inc.
- Al-Tabbal, J. A., & Al-Fraihat, A. H. (2011). Genetic variation, heritability, phenotypic and genotypic correlation studies for yield and yield components in promising barley genotypes. Journal of Agricultural Science, 4(3), 193. https://doi.org/10.5539/jas.v4n3p193
- Amare, K., Zeleke, H., & Bultosa, G. (2015). Variability for yield, yield related traits and association among traits of sorghum (Sorghum Bicolor (L.) Moench) varieties in Wollo, Ethiopia. Journal of Plant Breeding and Crop Science, 7(5), 125–9. doi: 10.5897/JPBCS2014.0469.
- Bibi, A., Sadaqat, H. A., Akram, H. M., & Mohammed, M. I. (2010). Physiological markers for screening sorghum (Sorghum bicolor) germplasm under water stress condition. International Journal of Agriculture and Biology, 12(3), 451–455. http://www.fspublishers.org.
- Billot, C., Ramu, P., Bouchet, S., Chantereau, J., Deu, M., Gardes, L., Noyer, J.-L., Rami, J.-F., Rivallan, R., & LI, Y. (2013). Massive sorghum collection genotyped with SSR markers to enhance use of global genetic resources. PloS One, 8(4), e59714. https://doi.org/10.1371/journal.pone.0059714
- Burton, G. W., & Devane, E. (1953). Estimating heritability in tall fescue (Festuca Arundinacea) from replicated clonal material 1. Agronomy Journal, 45(10), 478–481. https://doi.org/10.2134/agronj1953.00021962004500100005x
- Chavan, S., Mahajan, R., & Fatak, S. U. (2010). Genetic variability studies in sorghum. Karnataka Journal of Agricultural Sciences, 23(2), 322–323. http://eprints.icrisat.ac.in/id/eprint/57.
- Chavan, S., Mahajan, R., & Fatak, S. U. (2011). Correlation and path analysis studies in sorghum. Crop Research, 42(1), 2. doi: 10.25081/jsa.2017.v1i0.31No.
- CSA 2016. Report on area and production of major crops. The federal democratic republic of Ethiopia Central statistical agency agricultural sample survey.
- De, W. E. T., & Harlan, J. (1971). The origin and domestication ofSorghum bicolor. Economic Botany, 25(2), 128–135. https://doi.org/10.1007/BF02860074
- Deshmukh, S., Basu, M., & Reddy, P. (1986). Genetic variability, character association and path coefficients of quantitative traits in Virginia bunch varieties of groundnut. Indian Journal of Agricultural Sciences, 56(12), 816-821. https://agris.fao.org
- Dhutmal, R., Mehetre, S., More, A., Kalpande, H., Mundhe, A., & Sayyad Abubakkar, A. (2014). Variabity parameters in Rabi sorghum (sorghum bicolor L. Moench) drought tolerant genotypes. The Bio-scan, 9(4), 1455–1458. www.theecoscan.in.
- DOGGETT, H. (1988). Sorghum (2nd ed.). Longman.
- Gebrekidan, B. 1982. Utilization of accession in sorghum improvement. Sorghum in the eighties. Proceedings of the international symposium on sorghum, ICRISAT, Patancheru.
- Godbharle, A., More, A., & Ambekar, S. (2010). Genetic variability and correlation studies in elite ‘B’and ‘R’lines in Kharif sorghum. Electronic Journal of Plant Breeding, 1(4) 989–993. https://pdfs.semanticscholar.org.
- Gomez, K. A., & Gomez, A. A. (1984). Statistical procedures for agricultural research. John Wiley and Sons Inc. publisher of award-winning journals.
- Jain, S., Elangovan, M., & PATEL, N. (2010). Correlation and path coefficient analysis for agronomical traits in forage sorghum [Sorghum bicolor (L.) Moench]. Indian Journal of Plant Genetic Resources, 23(1), 15. https://scholar.google.co.uk.
- Johnson, H. W., Robinson, H., & Comstock, R. (1955). Estimates of genetic and environmental variability in soybeans. Agronomy Journal, 47(7), 314–318. https://doi.org/10.2134/agronj1955.00021962004700070009x
- Khoury, C. K., Achicanoy, H. A., Bjorkman, A. D., Navarro-Racines, C., Guarino, L., Flores-Palacios, X., Engels, J. M. M., Wiersema, J. H., Dempewolf, H., Sotelo, S., Ramirez-villegas, J., Castaneda-alvarez, N. P., Fowler, C., Jarvis, A., Rieseberg, L. O. R. E. N. H., & Struik, P. C. (2016). Origins of food crops connect countries worldwide. Proceedings of the Royal Society B: Biological Sciences. B, 283(1832), 0792. https://doi.org/10.1089/rspb.2016.0792
- Legesse, H. G. (2015). On-farm evaluation of sorghum [Sorghum bicolor (L.) Moench] varieties under tie ridge and NP fertilizer at Mekeredi, moisture stress area of Amaro, Southern Ethiopia. Journal of Agricultural and Biological Sciences, 2(2), 37–41. http://www.publicscienceframework.org/journal/absj.
- Mckevith, B. (2004). Nutritional aspects of cereals. Nutrition Bulletin, 29(2), 111–142. https://doi.org/10.1111/j.1467-3010.2004.00418.x
- Payne, R. W. (2009). GenStat. Wiley Interdisciplinary Reviews. Computational Statistics, 1(2), 255–258. https://doi.org/10.1002/wics.32
- Ranjith, P., Ghorade, R., Kalpande, V., & Dange, A. (2017). Genetic variability, heritability and genetic advance for grain yield and yield components in sorghum. International Journal of Farm Sciences, 7(2), 90–93. http://www.bepls.com.
- Rao, M., & Patil, S. (1996). Variability and correlation studies in F2 population of kharif x rabi crosses of sorghum. Karnataka Journal of Agricultural Sciences, 9(1), 78–84.
- Reddy, B., Ashok Kumar, A., Ramesh, S., & Sanjana Reddy, P. (2011). Breeding sorghum for coping with climate change. In Y. SS, B. Redden, H. JL, & H. Lotze-Campen (Eds.), Crop adaptation to climate change (pp. 326–339). John Wiley & Sons Inc.
- Ritter, K. B., Mcintyre, C. L., Godwin, I. D., Jordan, D. R., & Chapman, S. C. (2007). An assessment of the genetic relationship between sweet and grain sorghums, within Sorghum bicolor ssp. bicolor (L.) Moench, using AFLP markers. Euphytica, 157(1–2), 161–176. https://doi.org/10.1007/s10681-007-9408-4
- Sabiel, S. A., Noureldin, I., Baloch, S. K., Baloch, S. U., & Bashir, W. (2016). Genetic variability and estimates of heritability in sorghum (Sorghum bicolor L.) genotypes grown in a semiarid zone of Sudan. Archives of Agronomy and Soil Science, 62(1), 139–145. https://doi.org/10.1080/03650340.2015.1039522
- Seetharam, K., & Ganesamurthy, K. (2013). Research note characterization of sorghum genotypes for yield and other agronomic traits through genetic variability and diversity analysis. Electronic Journal of Plant Breeding, 4(1), 1073–1079. http://www.indianjournals.com.
- Shinde, D., Biradar, B., Salimath, P., Kamatar, M., Hundekar, A., & Deshpande, S. (2010). Studies on genetic variability among the derived lines of B x B, B x R and R x R crosses for yield attributing traits in rabi sorghum (Sorghum bicolor (L.) MOENCH). Electronic Journal of Plant Breeding, 1(4), 695–705. https://www.researchgate.net.
- SINGH, B. D. (2001). Plant Breeding principles and methods. Kalyani Publishers.
- Tariq, A. S., Akram, Z., Shabbir, G., Gulfraz, M., Khan, K. S., Iqbal, M. S., & Mahmood, T. (2012). Character association and inheritance studies of different sorghum genotypes for fodder yield and quality under irrigated and rainfed conditions. African Journal of Biotechnology, 11(38), 9189–9195. doi: 10.5897/AJB11.2561.
- Tesso, T., Gutema, Z., Deressa, A., & Ejeta, G. (2007). An integrated Striga management option offers effective control of Striga in Ethiopia. Integrating New Technologies for Striga Control: Towards Ending the Witch-Hunt, 199-212. https://doi.org/10.1142/9789812771506_0015
- Tomar, S. S., Sivakumar, S., & Ganesamurthy, K. (2012). Research note genetic variability and heritability studies for different quantitative traits in sweet sorghum [Sorghum bicolor (L.) Moench] genotypes. Electronic Journal of Plant Breeding, 3(2), 806–810. https://www.researchgate.net/publication/286249872.
- Udeh, I., & Ogbu, C. (2011). Principal component analysis of body measurements in three strains of broiler chicken. Science World Journal, 6(2), 11–14. http://www.vuzv.sk/slju/14_2/3_Egena.pdf.
- USDA. (2017). World agricultural production. Foreign agricultural service. Circular series WAP. United States Department of Agriculture.
- Vargas, M., Combs, E., Alvarado, G., Atlin, G., Mathews, K., & Crossa, J. (2013). META: A suite of SAS programs to analyze multienvironment breeding trials. Agronomy Journal, 105(1), 11–19. https://doi.org/10.2134/agronj2012.0016
- Yaqoob Muhammad, N. H. A. A. R. (2015). Genetic variability and heritability analysis for yield and morphological traits in Sorghum (Sorghum Bicolor (L.) Moench) genotypes. Journal of Agricultural Research, 53(3). https://apply.jar.punjab.gov.pk.