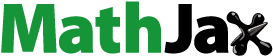
Abstract
Cotton is a very important crop that consists of traits with different associationship due to genetic and environmental factors. In order to determine the degree of association between seed cotton yield and important traits, a study was done using an RCBD experiment with ten genotypes. Seed cotton yield, GOT, lint yield, boll weight, bolls per plant, seed weight, plant height, fibre length, elongation, fineness and strength data were collected and analysed. Genotypic and phenotypic correlation analysis was done in Meta R. Estimation of direct and indirect effects was done using path analysis in Microsoft Excel. Analysis of variance revealed significant differences for boll weight, seed weight, GOT and plant height. Seed cotton yield was correlated with lint yield (r = 0.71***), fibre elongation (r = 0.54***), bolls per plant (0.27***), seed weight (r = 0.22***), strength (r = 0.21***) and fineness (r = 0.13*) at genotypic level. Ginning outturn was correlated with lint yield (r = 0.70***), elongation (r = 0.60***) and strength (r = 0.50***). Boll weight was correlated with seed weight (r = 0.56***) whilst plant height was highly associated with fibre strength (r = 0.58***). The adjusted R Square (0.98), low standard error (0.12) and low residual effect (R = 0.01) in regression analysis indicated that variability of seed cotton yield was explained by the causal variables. Lint yield had the highest direct effect on seed cotton yield (r = 1.055). Traits that could be used for indirect selection were bolls per plant (r = 0.006), seed weight (0.022) and or plant height (0.012). Gin outturn (0.737) had the highest indirect contribution to seed cotton yield through lint yield, followed by strength (0.012) through plant height, seed weight (0.011) through boll weight, fibre fineness (0.010) through boll weight. It was therefore concluded that selection of high yielding cotton genotypes could emphasize more on lint yield, boll weights, plant height and bolls per plant for better-performing lines. Gin outturn and fibre strength could be used indirectly to improve seed cotton yield through other traits.
PUBLIC INTEREST STATEMENT
Cotton variety development is a complex and rigorous activity due to the presence of many negatively correlated traits of economic importance that exist within the plant. It is therefore imperative that the traits are studied and appreciated so as to facilitate creation of data base for informed decisions during selections of best varieties.
Competing interests
The authors declare no competing interests
1. Introduction
Cotton (Gossypium hirsutum L.) is one of the most important industrial crops in the world (Farias et al., Citation2016a). It is used for both fibre and feed production. It accounts for a third of the global trade, with the major exporters being the United States, Uzbekistan, and India, and the major importers being China and Southeast Asian countries. In Zimbabwe, it is the third most important cash crop that is exploited much for the benefit all levels of the cotton value chain (AMA, Citation2018). It contributes significantly to the gross domestic product of the economy and as such has received a lot of attention in funding and research. This has greatly resulted in improvement of local varieties on yield and other associated traits (Riaz et al., Citation2019). However, the relationship that exists amongst the traits are greatly influenced by genetic constitution and the environment and it needs clarification under existing conditions through exploration of genotypic and phenotypic correlations (A.M. Khan et al., Citation2017).
Estimates of genotypic and phenotypic correlations among characters are useful in planning and evaluating breeding programmes. Knowledge of the correlation that exists between important characteristics facilitates the selection of genotypes with high field and fibre performance in cotton (Shabbir et al., Citation2016). The genetic correlation value offers a measure of the genetic inter-relationship between characteristics and may explain the degree of relationship between characters genetically, rather than phenotypically. As the phenotypic variance of a trait can be partitioned into environmental and genetic components, the covariance between the two traits can also be partitioned into environmental and genetic components (Ahmad et al., Citation2016). The overall effect of this is that, selection of cotton genotypes based on one trait may result in gross changes in the genetic constitution influencing the expression of other important agronomic traits (Farias et al., Citation2016b). The relative importance of direct and indirect effects of the traits on seed cotton yield are not considered in the correlations thus causing the changes (Lynch & Walsh, Citation1998).
Wright (Citation1921), proposed the path analysis which provided the understanding of trait association, through partitioning of the correlation coefficients in direct and indirect effects on the trait of interest which in the case of cotton is the seed cotton yield. The causal variables are first standardised to obtain the estimates of these effects using the regression equations. A few studies for cotton using fibre yield as the primary dependent variable have been done using this method for breeding purposes (Iqbal et al., Citation2003; Tyagi et al., Citation1998). The objective of the study was to establish the relationship between seed cotton yield and polygenic traits and determine the relative importance of direct and indirect effects each trait have on seed cotton yield.
2. Materials and methods
2.1. Description of the study sites
The field evaluations were carried out at seven locations that are representative of the cotton growing areas in Zimbabwe namely Kadoma (29° 53ʹ east), Matikwa (32° 14ʹeast), Shamva (31°71ʹeast), Kuwirirana (30°48ʹeast), Muzarabani (31° 00ʹ east), Wozhele (30°14ʹeast) and Chitekete (28° 56ʹ east) across the country. The site selection was based on representation of all the major cotton growing areas within the middleveld cotton production areas of the country making the locations random effects.
3. Plant materials
The experiment consisted of 10 cotton genotypes developed at the Cotton Research Institute of Zimbabwe and these were made up of three commercial check varieties and seven experimental lines. The trials were conducted for two seasons from 2015/16 to 2016/17. The genotypes used were five check varieties CRI MS 1, CRI MS 2 and SZ 9314 while the experimental lines 912–05-1, 932–05-3, GN96(b)-05-8, SO-99-9, 917–05-7, 938–05-3, TN96-05-9.
4. Experimental layout and design
The trials were laid out in randomized complete block designs (RCBD) with three 3 replications. The gross plot sizes used were 36 m2 and net plot sizes were 16 m2 where the data was collected. The recommended agronomic practices at each location were applied as for raising a successful crop as recommended in the Cotton handbook of 1998.
5. Data collection and data analysis
Data was collected on seed cotton yield, boll weight, ginning outturn (GOT), plant height, bolls per plant and fibre data collected on fibre strength, fibre length, fibre elongation and micronaire (fibre fineness). Analysis of variance was carried out for these traits using GenStat 18th version. Correlations of both types (genetic and phenotypic) were calculated using Meta R software version 6.0 from seasonal means using analysis of variance and covariance procedures proposed by Al-Jibouri et al. (Citation1958) and Falconer and Mackay (Citation1996) as follows:
where rG = genotypic correlation coefficient,COVG(A,B) = (Mg-Me)/r genetic covariance between variables A and B,VG(A) = (Mg-Me)/r genotypic variance for variable A,VG(B) = (Mg-Me)/r genotypic variance for variable B,Mg and Me = mean squares of treatments (genotypes) and error, respectively,r = number of replications
Where rP = phenotypic correlation coefficient, COVP (AB) = phenotypic covariance between variables A and B = COVG (A,B) + Ve(A,B),VP (A) = VG (A) + Ve (A) phenotypic variance for variable A,VP (B) = VG (B) + Ve (B) phenotypic variance for variable B,Ve = Me/r error variance,r = number of replications.
Mean scores of the various traits were standardized since the units of measurements of the traits were different following the expression x = (x-m)/sd, where x = score, m = group mean, sd = group standard deviation. Regression analysis was then carried out using Microsoft Excel 2007 to determine the partial regression coefficients (also known as the direct path coefficients). These were then multiplied with the correlation coefficients to determine the indirect path coefficients (Akintunde, Citation2012). Seed cotton yield was considered as a dependent variable and the rest of the traits were considered as independent variables. Statistical significance of the correlation coefficients for the different trait associations were determined using Microsoft Excel 2007.
6. Results and discussion
The analysis of variance for the mean values of the 10 genotypes revealed highly significant differences (P < 0.001) for boll weight and seed weight, significant differences (P < 0.05) for gin outturn percentage and plant height as shown on the mean squares of the different traits in Table . According to Salahuddin et al. (Citation2010), this provides enough evidence of for the significant genetic variability of the traits among the genotypes, which have further influenced on other traits associated with them.
Table 1. Mean square and CV for seed cotton yield and associated traits
6.1. Significance levels: * = 0.05, ** = 0.01, *** = 0.001
Table shows the results of genotypic correlation analysis of seed cotton yield and the associated traits. More traits revealed high positive correlation at genotypic level more than at phenotypic level which indicated the high inherent association of the traits in the study (Table ). This was also noted in studies done on soyabeans (Glycine max L.) by Agedew in 2006, sorghum (Sorghum bicolor L.) by Wassihun in 2006 and coriander (Coriandrum sativum L.) by Beemnet et al. in 2013.
Table 2. Genotypic correlation matrix of seed cotton yield and 10 associated traits
Seed cotton yield was significantly and highly positive correlated with lint yield (r = 0.71***) and fibre elongation (r = 0.54***) at genotypic level. Other positive correlation at genotypic level which were significant existed between seed cotton yield and bolls per plant (0.27***), seed weight (r = 0.22***), fibre strength (r = 0.21***) and fibre fineness (r = 0.13*) with negative correlation being observed with plant height (r = −0.52***). A strong and significant genotypic relationship was observed among GOT and lint yield (r = 0.70***), fibre elongation (r = 0.60***) and fibre strength (r = 0.50***). Some positive and significant relationship also existed between the GOT and boll weight (r = 0.24***), plant height (r = 0.30***), fibre length (r = 0.11**) and micronaire (r = 0.40***). Boll weight genotypically correlated with seed weight (r = 0.56***) whilst plant height was highly associated genotypically with fibre strength (r = 0.58***). Elongation was also correlated genotypically with strength (r = 0.55***) whilst strength with micronaire (r = 0.71***). Khalid et al. (Citation2018) similarly reported positive and significant correlation seed cotton yield with plant height, number of bolls per plant and boll weight. In another study, Salahuddin et al. (Citation2010) also had similar results of high correlation between seed cotton yield and boll numbers per plant, plant height and boll weight. Hence, selection of cotton plants having these distinguishing traits will facilitate cotton seed yield improvement.
At phenotypic level, there were high positive and significant (P < 0.001) correlation between seed cotton yield and lint yield (r = 0.93) whilst positive and significant (P < 0.001) correlation existed between seed cotton yield and boll weight (r = 0.37) and plant height (r = 0.31) as shown in Table . Seed weight was significantly and positively correlated with boll weight (r = 0.61***), elongation with fibre length (r = 0.54***), fibre strength with fibre length (r = 0.72***), fibre micronaire with fibre length (r = 0.63***), fibre micronaire with fibre elongation (r = 0.56***) and fibre micronaire with fibre strength (r = 0.73***). In traits that exhibited higher phenotypic correlation values than the genetic correlation values, apparent association amongst the two traits would not be only due to genes but due to favourable influence of the environment and where values were zero or insignificant then the two traits would be independent (Hussain et al., Citation2010).
Table 3. Phenotypic correlation matrix of seed cotton yield and 10 associated traits
The positive association of seed weight with boll weight, plant height and bolls per plant will facilitate simultaneous improvement through selection for the traits as the later are associated with seed cotton yield. However, simultaneous improvement of seed weight and seed cotton yield or plant height and seed cotton yield is very difficult due to the negative association of the pair of traits. An independent selection should be done for the improvement of such traits.
In this study, the regression analysis results of seed cotton yield against the associated traits as presented in Table , revealed high values of adjusted R Square (0.98), low standard error value (0.12) and low residual effect (R = 0.01). This indicated that most of the variability of seed cotton yield was explained by the causal variables in the study. The P value for the intercept was significantly less than alpha (P < 0.05) implying that slope of the regression line was significantly different from zero and the null hypothesis of no effect of the causal variables on seed cotton yield rejected. As noted by Zaiontz (Citation2015), this indicated that regression model was a significant good fit. The P values of the all the coefficients of the traits except for gin outturn, lint yield, boll weight and seed weight were greater than 0.05 suggesting that these four traits significantly contributed to the observed variation in seed cotton yield
Table 4. Regression analysis of seed cotton yield and associated traits
The results of direct and indirect correlation coefficients regressed with seed cotton yield and are presented in Table . Lint yield had the highest direct effect on seed cotton yield (r = 1.055) which implied that lint yield could be used as marker for direct selection. However, since this is a trait that can be determined after harvest, traits in the field that can be used for indirect selection could be bolls per plant (r = 0.006), seed weight (0.022) and or plant height (0.012) as they have direct effect values that are greater than the correlation coefficients (r = 0.00, r = −0.005 and r = 0.004, respectively) with seed cotton yield. Doggett (Citation1988) reported that the height of the plant influences the number of nodes produced, which equates with the number of leaves produced thereby increasing the photosynthetic capacity, and hence increased yield. The indirect correlation coefficients between seed cotton yield and elongation (r = 0.000) and the direct effect (r = 0.002) implied that the observed phenotypic correlation coefficient explained the true associationship (Akatwijuka et al., Citation2019). Gin outturn percentage, boll weight, fibre elongation and fibre fineness had positive genetic correlations but with negligible to positive direct effects, indicated that the indirect effects caused the observed correlation.
Table 5. Estimates of genotypic direct (bold and diagonal) and indirect effects of individual traits to seed cotton yield via other 11 independent traits of 10 genotypes tested over two seasons of 2015/16 and 2016/17 at various sites
The path coefficient analysis of indirect and direct effects of the associated traits with seed cotton yield revealed that gin outturn (0.737) had the highest indirect contribution to seed cotton yield through lint yield, followed by strength (0.012) through plant height, seed weight (0.011) through boll weight, fibre fineness (0.010) through boll weight indicating the importance of these traits to plant height, boll weight and seed cotton yield. These need to be carefully considered simultaneously when selecting for yield improvement in cotton.
SC = seed cotton yield (kg/ha), G.O.T = gin outturn, LY = lint yield (kg/ha), BW = boll weight (g), BP = Bolls per plant, 100 swt = 100 seed weight (g), PH = plant height (cm), UHML = upper half mean length (inches), EL% = elongation percentage, STR = strength (g/tex), MIC = micronaire
7. Conclusion
The study concluded that for selecting high yielding cotton genotypes for the middleveld areas of Zimbabwe, more emphasis should be given to genotypes that produce more lint yield, boll weights, average plant height and high number of bolls per plant for better performing lines as they were closely associated. This was the order of importance in terms of association of the traits contributing to seed cotton improvement. However, seed cotton yield improvement greatly affected seed weight and similarly high seed weights were associated with low number of bolls per plant. It was also concluded that the greater number of bolls per plant, greatly reduced the boll weight in cotton so a balance have to be reached to achieve optimum yields. Fibre elongation, strength and fineness were linked to seed cotton yield genetically and was concluded that these could play positive roles as selection criteria to improve seed cotton yield. It was also concluded that gin outturn and fibre strength could be used indirectly to improve seed cotton yield through other traits such as plant height and boll weight.
Additional information
Funding
Notes on contributors
B. Chapepa
Chapepa Blessing is a plant breeder working at Cotton Research Institute of Zimbabwe as a cotton breeder. His research areas are developing, implementation analyzing data and information dissemination of the national cotton breeding program in the Department of Research and Specialist Services of the Ministry of Agriculture in the country. Other research interests include crop modelling using remote sensing technology and molecular tool applications.
Marco Mare is also plant breeder at Cotton Research Institute and is involved in developing, implementation analyzing data and information dissemination of the national cotton breeding program.
Washington Mubvekeri is the head of Cotton Research Institute and is responsible for all cotton research programs in the country.
Dr. Dumisani Kutywayo is the director of research in the Department of Research and Specialist Services and plays a critical role in ensuring quality research outputs.
References
- Ahmad, S., Fiaz, S., Riaz, A., Bashir, I., & Zeb, A. (2016). Correlation analysis of morphological and fiber quality traits in upland cotton (Gossypium hirsutum L. International Journal of Biosciences, 9(4), 200–11. http://dx.doi.org/10.12692/ijb/9.4.200-208
- Akatwijuka, R., Rubaihayo, P. R., & Odong, T. L. (2019). Correlations and path analysis of yield traits in sorghum grown in southwestern highlands of Uganda. African Crop Science Journal, 27(3), 437–444. https://doi.org/10.4314/acsj.v27i3.8
- Akintunde, A. (2012). Path analysis step by step using Excel. Journal of Technical Science and Technologies, 1(1), 9–15. https://dx.doi.org/10.4314/acsj.v27i3.8
- Al-Jibouri H. A., Miller, P. A., & Robinson, H. F. (1958). Genotypic and environmental variances and covariances in an upland cotton cross of interspecific origin. Agronomy Journal, 50, 633– 636. https://doi.org/10.2134/agronj1958.00021962005000100020x
- AMA. 2018. Agricultural marketing authority report. Zimbabwe.
- Baloch, M. J. (2004). Genetic variability and heritability estimates of some polygenic traits in upland cotton. Pakistan Journal of Scientific and Industrial Research, 42(6), 451–454. https://inis.iaea.org/search/search.aspx?orig_q=RN:36079332
- Batool, S., Khan, N. U., Makhdoom, K., Bibi, Z., Hassan, G., Marwat, K. B., Farhatullah, F., Mohammad, R., & Khan, I. A. (2010). Heritability and genetic potential of upland cotton genotypes for morpho-yield traits. Pakistan Journal of Botany, 42(2), 1057–1064. https://www.researchgate.net/publication/236853671_Heritability_and_genetic_potential_of_upland_cotton_genotypes_for_morpho-yield_traits
- Doggett, H. (1988). Sorghum (2nd ed.). Longman, UK.
- Falconer, D. S., & Mackay, T. F. C. (1996). Introduction to quantitative genetics (4th ed.). Essex, UK: Longman.
- Farias, F. J. C., Carvalho, L. P., Silva Filho, J. L., & Teodoro, P. E. (2016a). Biplot analysis of phenotypic stability in upland cotton genotypes in Mato Grosso. Genetics and Molecular Research, 15(2), gmr.15038009. https://doi.org/10.4238/gmr.15028009
- Farias, F. J. C., Carvalho, L. P., Silva Filho, J. L., & Teodoro, P. E. (2016b). Correlations and path analysis among agronomic and technological traits of upland cotton. Genetics and Molecular Research, 15(3), gmr.15038239. https://doi.org/10.4238/gmr.15038239
- Hussain, K., Khan, I. A., Sadaqat, H. A., & Amjad, M. (2010). Genotypic and phenotypic correlation analysis of fibre and fiber quality determining traits in upland cotton (Gossypium hirsutum). International Journal of Agriculture and Biology, 12(3), 348–352. http://www.fspublishers.org
- Iqbal, M., Chang, M. A., Iqbal, M. Z., & Hassan, M. (2003). Correlation and path coefficient analysis of earliness and agronomic characters of upland cotton in Multan. Journal of Agronomy, 2(3), 160–168. https://doi.org/10.3923/ja.2003.160.168
- Khalid, M. A., Malik, T. W., Fatima, N., Shakeel, A., Karim, I., Arfan, M., Merrium, S., & Khanum, P. (2018). Correlation of economic traits in upland cotton. ACTA Scientific Agriculture, 2(10), 59–62. https://actascientific.com/ASAG/pdf/ASAG-02-0196.pdf
- Khan, A. M., Fiaz, S., Bashir, I., Ali, S., Afzal, M., Kettener, K., Mahmood, N., & Manzoor, M. (2017). Estimation of genetic effects controlling different plant traits in cotton (Gossypium hirsutum L.) under clcuv epidemic condition. Cercetari Agronomice in Moldova, 50(1), 47–56. https://doi.org/10.1515/cerce-2017-0004
- Khan, N. U., Abro, H. K., Kumbhar, M. B., Hassan, G., & Mahmood, G. (2000). Study of heterosis in upland cotton-II. Morphology and yield traits. The Pak. Cottons, 44(1), 13–23. https://www.scirp.org/(S(oyulxb452alnt1aej1nfow45))/reference/ReferencesPapers.aspx?ReferenceID=2152403
- Khan, N. U., Hassan, G., Kumbhar, M. B., Marwat, K. B., Khan, M. A., Parveen, A., Aiman, U., & Saeed, M. (2009b). Combining ability analysis to identify suitable parents for heterosis in seed cotton yield, its components and lint % in upland cotton. Industrial Crops and Products, 29(1), 108–115. https://doi.org/10.1016/j.indcrop.2008.04.009
- Larik, A. S., Malik, S. I., Kakar, A. A., & Naz, M. A. (2000). Assessment of heritability and genetic advance for yield components in G. hirsutum. Science Khyber, 13, 39–44. https://www.researchgate.net/publication/287823603_Assessment_of_heritability_and_genetic_advance_for_yield_components_in_G_hirsutum
- Lynch, M., & Walsh, B. (1998). Genetics and analysis of quantitative traits. Sunderland, MA: Sinauer Associates. http://www.invemar.org.co/redcostera1/invemar/docs/RinconLiterario/2011/febrero/AG_8.pdf
- Riaz, B., Saeed, A., Fiaz, S., & Adeel, R. (2019). Genetic diversity among Bt Cotton (Gossypium hirsutum L.) germplasm assessed through morphological and within-boll yield attributes. Journal of Animal and Plant Sciences, 29(1), 226–231. https://www.researchgate.net/publication/330926411
- Salahuddin, S., Abro, S., Rehman, A., & Iqbal, K. (2010). Correlation analysis of seed cotton yield with some quantitative traits in upland cotton (Gossypium hirsutum L.). Pakistan Journal of Botany, 42(6), 3799–3805. https://www.researchgate.net/publication/266492609
- Shabbir, R. H., Bashir, Q. A., Shakeel, A., Khan, M. M., Farooq, J., Fiaz, S., Ijaz, B., & Noor, M. A. (2016). Genetic divergence assessment in upland cotton (Gossypium hirsutum L.) using various statistical tools. Journal of Global Innovations in Agricultural and Social Sciences, 4(2), 62–69. https://doi.org/10.22194/JGIASS/4.2.744
- Soomro, Z. A., Larik, A. S., Kumbhar, M. B., Khan, N. U., & Panhwar, N. A. (2008). Correlation and path analysis in hybrid cotton. SABRAO Journal Breeding and Genetics, 40(1), 49–56. https://www.researchgate.net/publication/289246089
- Tyagi, A. P., Mor, B. R., & Singh, D. P. (1998). Path analysis in upland cotton (Gossypium hirsutum L. Indian Journal of Agricultural Research, 22(1), 137–142.
- Wright, S. (1921). Correlation and causation. Journal of Agricultural Research, 20(7), 557–585. https://pdfs.semanticscholar.org/90bd/1ea6df6cf7ff14c392818751270b93d53d4e.pdf
- Zaiontz, C. A. 2015. Testing the significance of the slope of regression line. Real Statistics Resource Pack Software. Release 4.3. www.real-statistics.com