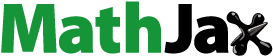
Abstract
Even though poverty has decreased in recent years, it is still a challenge in Ethiopia especially in rural areas the rate of decrease is slower than the counterpart. Most rural livelihoods in the study area depend on rain-fed agriculture and their exposure to poverty and its risk factors not well documented. So, to fill this gap this study examined the extent and the major determinants of poverty at different levels of expenditure quantiles in the rural household of Bahir dar zuria district, Ethiopia. This study used the FGT index and quantile regression model. The determinants of rural household poverty were total family size, total land ownership, distance to market, non-off-farm income, and bad health status of the household head. It was found that the total family size and bad health status of the household head can increase the poverty of the rural household which is lower the standard of living of rural households. While the non-off-farm income can decrease the poverty of the rural household. Thus, to reduce the poverty of the rural household; government policies and programs should give more emphasis on family planning, the necessity of health insurance, and improving the service of health institutions to bring overall household welfare development. Moreover, it promotes rural households to participate in off-farm and non-farm livelihood activities beyond agriculture to diversify their employment.
PUBLIC INTEREST STATEMENT
Even though the government, international community, and NGOs have been trying to reduce poverty and disparity. Still, rural poverty has not declined so much due to ineffective and insufficient social protection of pro-poor government programs. Hence, this study examined the extent and determinants of rural household poverty based on consumption expenditure in Bahir dar zuria district, Ethiopia. This paper used the FGT index and quantile regression model. So far, especially for rural livelihoods vulnerability of returning to poverty remains high due to various risks and shocks. Risk factors and shocks require enhancing smallholders’ access to insurance schemes provision of credit facilities promoting off-farm activities and helping farmers use improved technologies. Therefore, this paper contributed to the empirical literature by examining the contributions of different factors to the poverty of rural households at different levels of expenditure per capita.
1. Introduction
Poverty reduction and improving household wellbeing is an important issue for developing countries on their goals of development policies and strategies. Ethiopia is a low-income country ranking 164th out of 187 countries (World Bank, Citation2017). After decades of low-income levels and sluggish economic growth, since 2004 Ethiopia has seen growth at a higher rate than most African countries. In 2017, Ethiopia overtook Kenya as East Africa’s largest economy (IMF, Citation2017). Real GDP growth averaged 10.9 percent annually (8.0 percent per capita) in 2004 to 2014, Ethiopia is the 11th poorest country in the world by income per person, and home to Sub-Saharan Africa’s second most populous with a population size of about 90 million in 2015/16 with 2.5 percent growth per annum (FDRE, Citation2018), the vast majority (84%) of which are rural dwellers and subsistence farmers, the poorest 40 percent tend to be even more likely to live in rural areas and engage in agriculture (World Bank, Citation2016).
In the effort to reduce poverty and achieve economic growth, the country has been implementing a series of poverty-focused development strategies. One of which is agricultural growth and investments, since Agriculture accounts for most jobs, about 40 percent of output and exports and contributing 37 percent of GDP in 2015/16. In Ethiopia, each 1 percent growth in Gross Domestic Product (GDP) resulted in a 0.15 percent reduction in poverty. For every 1 percent in agricultural output; poverty was reduced by 0.9 percent. Hence, agriculture is an important driver of economic growth and rapid poverty reduction in Ethiopia, but female farmers benefit less from this because they are less productive than their male counterparts (World Bank, Citation2016). Economic growth has also driven by the growth in services sectors, contributing 47 percent of GDP in 2015/16. So far the performance of the industrial sector during the First Growth and Transformation Plan (GTP I) from 2010/2011 to 2014/2015 indicated that the sector has not been able to achieve the hoped-for changes in terms of the structure of the economy as it grapples with multiple challenges (UNDP, Citation2018).
However, despite impressive economic growth, the expansion of social services and a substantial decrease in poverty from 46% of the population living below the national poverty line in 1995, 44% in 2000, 30% in 2011, and also under 24% in 2015/16. While poverty has decreased remarkably in general, poverty is still a challenge in Ethiopia as over 22 million people are living below the national poverty line, with 80 percent in rural areas. The rate of decrease in recent years is slower in rural areas as compared to urban areas. For rural Ethiopia, according to 1995 to 2015/16 household consumption expenditure survey report, based on the national poverty line, the proportion of poor people decreased from 48 percent to 26 percent. A significant number of children in rural areas remain locked in a vicious circle of poverty and vulnerability to environmental and socio-economic shocks (Ethiopia Poverty Fact Sheet, Citation2018). Programs targeting poverty should mainly focus on risk factors and shocks that swing households in and out of poverty such as drought, conflict, price fluctuations, flooding, illness and death of household members, underemployment, and the like (Foster, Citation2007; Gunther & Harttgen, Citation2009). These shocks and risks can lead to a substantial loss of consumption, income, or wealth of households (Dercon et al., Citation2005; Gillis et al., Citation2001). In 2016, the most common shock reported was a food shortage, and crop failure probably resulted from El Nino drove drought that hit many parts of Ethiopia. And also, in 2009 and 2011, almost all households were exposed to at least one economic shock, probably due to persistent monthly food price rises for all items. The random variation observed in the distribution shocks across major regional states as well as survey rounds. While the Southern region and Amhara regions are the worst hit by drought, food, and input price rise in the three surveys round, Tigray and Oromia are relatively affected by those shocks. These indicate the high exposure of households to various shocks in rural Ethiopia and hence are at risk of falling into poverty in the future (CSA, Citation2012; FDRE, Citation2018; Sisay, Citation2018). High prices and good weather ensured that the adoption of modern inputs brought high returns and poverty reduction for those well connected to markets. Yet, the vulnerability of returning to poverty remains high, especially for rural livelihoods due to various risks and shocks attributed to their dependence on rain-fed agriculture (Hill & Porter, Citation2017; World Bank, Citation2016). Risk factors and shocks require enhancing smallholders’ access to insurance schemes, provision of credit facilities, promoting off-farm activities, and helping farmers use improved technologies such as drought-resistant seed varieties, irrigation facilities, and so on.
In Ethiopia, while more farmers may benefit from urban spillover effects on agricultural prices and access to modern inputs due to their proximity to secondary towns, the size of the benefits they realize may be smaller (Vandercasteelen et al., Citation2018). Moreover, rural migrants are more likely to participate in the non-farm sector of secondary towns (Christiaensen et al., Citation2016). While rural towns are not well developed to handle the growing rural population and social assistance are not big enough to provide employment. Moreover, rural finances have expanded, but cannot still meet people’s demand in rural areas. Land tenure system limiting rural-urban migration, if a farmer permanently migrated to urban areas his/her land will be taken (Woldehanna, Citation2019).
The 2015 UNDP Human Development Report, Ethiopia’s human development index (HDI) value was 0.448 putting it in the low human development category and ranked 174th position out of 188 countries. The HDI value increased from 0.283 in 2000 to 0.448 in 2015 indicated a 58.2 percent increase over the past 15 years. Between 1990 and 2015, life expectancy at birth increased by 17.5 years to 64.6 years, mean years of schooling by 1.1 years to 2.6 years, expected years of schooling by 5.3 years to 8.4 years, and national income per capita by 134.7 percent to 1523 USD (2011 PPP$). But Ethiopia remains among the set of low human development countries around the world and has seen little change relative to others due to sluggish performance in education, particularly in mean years of schooling. The multidimensional poverty index which identifies multiple overlapping deprivations in health, education, and standard of living computed based on the 2011 household consumption expenditure survey. Indicated that 88.2 percent of the population is multidimensionally poor. Consumption inequality has shown a considerable increase from 0.298 in 2010/11 to 0.328 in 2015/16. The rise in inequality was also seen in both urban (from 0.37 to 0.38) and rural (from 0.27 to 0.28) areas of Ethiopia. As a result of a very equal consumption distribution in rural areas, inequality showed a modest increase though it is still low by international standards and even compared with sub-Saharan African countries (FDRE, Citation2018; UNDP, Citation2018).
According to the Central Statistical Agency (CSA), in 2008 the average Ethiopian farmer holds 1.2 hectares of land, with 55.13% of them holding less than 1.0 hectare. The income sources of the majority of rural households are primarily dependent on smallholder agriculture in the form of crop and livestock production. About 90% of rural households in Oromia, 85% in Amhara; 83% in Tigray, and significant proportions in southern nation, nationalities and peoples (SNNP) engaged in solely in agriculture. Amhara region also constitutes 40% of the livestock population of the country (Sisay, Citation2018). In 2017, the highest multidimensional poverty (59%) was in Amhara and Oromia regions, while SNNP (57.4%) is the second. The relatively lower poverty incidence is in Tigray (53.7), and others (50.6%) regions (University of Oxford, Citation2017).
The regional distribution of total poverty in Ethiopia in 2015/16, poverty incidence is the highest in Tigray (27%) followed by Beneshangul Gumuz (26.5%), and Amhara (26.1%), while poverty estimates are lowest in Harari (7.1 percent) followed by Dire Dawa (15.4 percent) and Addis Ababa (16.8 percent). In terms of food poverty, the highest poverty is also observed in Tigray (32.9%), followed by Amhara (31.3%) and Afar (28.3 percent). The lowest food poverty is found again in Harari (6.3%), followed by Dire Dawa (12.2%) and Gambela (17.2%). Overall, compared to the previous survey years, the difference in poverty among the regional states in 2015/16 has narrowed substantially. Moreover, absolute poverty is much lower than food poverty in all regions. The region with the largest number of poor people in Oromia accounted for above one-third of all Ethiopian living in poverty in 2004/05 (actually 36%). Large numbers of poor people are also found in Amhara (5.3 million) and SNNP (3.1 million) in 2015/16. In general, despite the relative improvement in the past twenty years, the poverty level in Ethiopia is still unacceptably high (Federal Democratic Republic of Ethiopia: FDRE, Citation2018).
Amhara regional state is located in the northwestern and north-central parts of Ethiopia. With a population of 21.1 million in 2017, Amhara is the second most populous region making up 22.4 percent of the Ethiopian population. Nearly 84 percent of the population resides in rural areas and are engaged in agriculture. Agriculture remains the dominant economic sector than the services and industry sectors in the region. Poverty is pervasive in the Amhara region, and the rate is higher than the national average. In 2011, the Amhara region had a child poverty rate of 34 percent. Based on the 2016 household income and consumption expenditure survey (HICES), about 26.1 percent of the populations in the region were below the nationally defined poverty line compared to 23.5 percent for the entire country. Poverty in rural areas is more prevalent (28.8 percent) than in urban areas (11.6 percent). However, the level of poverty in the region has significantly declined from 30.5 percent in 2010/11 to 26.1 percent in 2016. The food poverty situation in the region is also critical. The region has the highest percentage of people living in food poverty in Ethiopia, 31.3 percent in 2016. According to the 2016 EDHS, childhood malnutrition (stunting) in the region is among the highest in the country that is 46 percent in Amhara while 38 percent in the whole country (UNICEF, Ethiopia, Citation2018).
As the economy rising in Ethiopia, food consumption growing more rapidly than nonfood consumption. Nationally, food consumption as a share of total consumption has fallen from 60 percent to 56 percent between 1995/96 and 2004/5 and 52 percent in 2010/11, but this has again slightly risen to 55 percent in 2015/16. Between 2005 and 2016, while consumption levels were increasing overall, the bottom 15 percent in rural areas did not experience real consumption growth. The severity of rural poverty was higher in 2016 than in 2005, implying the existence of a segment of the rural population that has yet to benefit from Ethiopia’s development (FDRE, Citation2017).
Even though the government, the international community, and non-governmental organizations (NGOs) have been trying to reduce poverty and disparity in the distribution of the benefits of economic growth across geographic areas and social groups. Still, rural poverty has not declined so much due to ineffective and insufficient social protection of pro-poor government programs. Most of the earlier studies are focused on poverty dynamics and inequalities among the poor at the national level (Ethiopia). Identifying the determinants of poverty in the rural household helps to improve the welfare of rural Ethiopians and can lead to the development of poverty reduction policies and strategies. Hence, the purpose of this study was to examine the extent and the major determinants of poverty at different levels of expenditure quantiles of the population in the rural household of Bahir dar zuria district, northwestern Amhara region, Ethiopia.
This paper is organized as follows. Section 2 describes the empirical literature review and hypothesis development. Section 3 introduces the description of the study area, data, and sample. Section 4 explores the method of data analysis. Section 5 presents empirical results and discussion. Finally, section 6 presents the conclusion.
2. Empirical literature review and hypothesis development
Based on the previous empirical studies, the following factors were reviewed and hypothesized to influence the poverty of rural households as follows.
2.1. Landholding size and poverty
The total landowner of the household is measured in timad that is one timad represents 0.25 hectare. It is one of the basic assets of the household. Empirical research carried out by Shibru et al. (Citation2013) found that the farmland size had negative impacts on the likelihood of a household being poor in the Dembel district of Ethiopia. For rural households in Mozambique, an asset such as farm size ownership is positively related to the wellbeing of households (Kassie et al., Citation2014). The land is found to be associated with lower poverty levels (Bersisa & Heshmati, Citation2016). Therefore, households having more land own are expected to positively influence the welfare of rural households.
2.2. Age of Head of the Household and poverty
Age of the household head is measured in years and a study conducted in Ethiopia by Kebede and Sharma (Citation2014) shows that the age of household head is negatively correlated with the probability of being poor. Hence, the age of the household head is expected to be positively and negatively associated with the welfare of rural households.
2.3. Gender of Head of the Household and poverty
The gender of the household head is a categorical variable that is zero represents females and one otherwise. It is known that most of the Ethiopian rural households are male-headed except the male household head dies or loses functionality due to old age. Workneh (Citation2008) argues that cultural and societal norms in rural areas often create considerable negative impacts on the nutritional status of women and children, making them vulnerable social groups. The household head being female is positively correlated with the probability of being poor (Kebede & Sharma, Citation2014; Teka et al., Citation2019; Tsehay & Bauer, Citation2012). This reflects the low level of empowerment of females to valuable assets (like land) in rural Ethiopia. Female farm managers in Ethiopia are 23 percent less productive than their male counterparts. They have less time to spend on farm work and farm less land, more of which is rented (World Bank, Citation2015). Female-headed households, especially in rural areas of Ethiopia are likely to have lower consumption (FDRE, Citation2018). Later, this study hypothesis that gender of the household head is expected to be negatively correlated with the poverty of rural household.
2.4. Education and poverty
The educational level of the household head is also a categorical variable equaling; zero if the household had no education, one if the household had primary education and two otherwise. Year of schooling of the household head is measured in years of schooling of the household head. Education has contributed to poverty reduction and increasing the welfare of the poor (World Bank, Citation2016). Education has a clear and positive correlation with consumption, in both urban and rural areas. Even completing informal education shows significant increases in consumption, showing that investment in adult education may also pay returns in Ethiopia (FDRE, Citation2018). Literacy and schooling are important indicators of the quality of life in their own right. That is the literacy was found to be negative and significant, which means that literate household heads are less likely to be poor than are illiterate households. This means that education might increase earning potential and improve the occupational and geographic mobility of labour (Kebede & Sharma, Citation2014; Teka et al., Citation2019; World Bank Institute, Citation2005). Hence, education is hypothesized to have a positive impact on the welfare of rural households.
2.5. Access to credit and poverty
Access to credit is the household received credit from different formal and informal sectors during the last 12 months. It is categorizing as zero represents not and one otherwise. The previous study shows that credit is positively associated with the welfare of households (Teka et al., Citation2019; Tsehay & Bauer, Citation2012). Access to and utilization of credit is facilitated to increase or diversify household incomes and outflow from poverty. A research done by Kassie et al. (Citation2014) found that possession of assets such as access to credit was found to be positively related to the well-being of sample households in Malawi. So, access to credit is expected to be positively associated with the welfare of rural households.
2.6. Family size and poverty
The total family size of the household affects the welfare of rural households. Households with larger family sizes are more likely to be poor (Bersisa & Heshmati, Citation2016; Kebede & Sharma, Citation2014). Therefore, family size effect will be expected positive when a household has a large household size this implies more economically active household members (less dependency ratio) and negative otherwise.
2.7. Non-off-farm income and poverty
Non/off-farm income is the amount of income in birr obtained from off-farm and non-farm livelihood activities during the last 12 months. It is the most important factor in explaining consumption and poverty. Using selected agro-pastoral households of Ethiopia, it is remarked that the participation in non-farm opportunities had notable impacts on the likelihood of a household being poor in Dembel district (Shibru et al., Citation2013). For rural households in Mozambique, engagement in off-farm is positively related to the well-being of households (Kassie et al., Citation2014). Hence, non/off-farm income is expected to positively affect the welfare of rural households.
2.8. Health status and poverty
The health condition of the household head is a categorical variable that is zero represents an excellent, one represents good and two represents bad. It is noted that the health status of the household head is correlated with poverty and household living standards. The household which has poor health can lower the productivity of household members and contribute to poverty. Health has contributed to poverty reduction and increasing the welfare of the poor (World Bank, Citation2016). As a result, health status is hypothesized to hurt rural households’ welfare.
2.9. Distance to market and poverty
Distance to the district market from a residence in minutes of walking time is a community level characteristic. It was found to be negative. In other words, the nearer the household is to marketplaces and relatively large towns, the better the access to markets and to public services, as well as to private service providers, and hence the lower the chance of falling into poverty (Bersisa & Heshmati, Citation2016; Teka et al., Citation2019). The remoteness of rural areas that lower the price farmers get for their goods and raise the price they pay for purchases, due to high transport costs are responsible for generating food insecurity among the poor and contributes to poverty (World Bank Institute, Citation2005). Besides distance to the city may also affect the costs of inputs of farmers. While the nominal prices may be the same for all cooperatives, the transaction costs of obtaining fertilizer and improved seeds are much higher in remote areas (Minten et al., Citation2013; Vandercasteelen et al., Citation2018). Poorer agricultural households are more remote and they are less likely to use agricultural inputs (Minten et al., Citation2015) and are less likely to see poverty reduction from the gains in agricultural growth that are made (Hill & Tsehaye, Citation2014). Poverty is much higher in remote areas, and in the periphery and lowland, often agro-pastoral, areas of Ethiopia (Shibru et al., Citation2013; World Bank, Citation2016). Finally, distance to market is hypothesized to reduce the risk of rural households falling into poverty.
3. Description of study area, data and sample
This study was conducted in Zenzelima kebele in Bahir dar zuria district, Amhara region, Ethiopia. It consists of three villages namely Michael, Sesa beret, and Gedro villages (CSA, Citation2007). There are 1997 households in Zenzelima kebele with a total population of 10,614, of whom 5233(49.3%) are male and the remaining 5321(50.7%) are female. It has about 4535 hectares of cultivated land, of which 1939 hectare is covered by annual crop and 345 hectares is covered by irrigation. The ecology of this kebele is favourable for teff, maize, finger millet, and khat. The production system is both livestock and crop production.
In this study to determine the sample size simple random sampling with proportion techniques was used. The technique is expressed as follows:
where n is the number of household sampled; N is a total number of household in the kebele, P is the probability of the household being poor, P = 0.5; 1-P is the probability of the household being non-poor; d is a margin of error, and Z is valued at 5% level of significance. Therefore, the sample size is calculated as
Moreover, the Zenzelima kebele comprises three villages, so take sampled households from these villages proportionally to make the sample more representatives.
These 350 households are selected by a simple random sampling technique and out of these 158, 97, and 115 households selected from Gedro, Michael, and Sesa beret village, respectively (Table ). Thus, a total of 350 rural household heads were randomly interviewed from April to May in 2019 using a structured questionnaire out of 1997 target household heads. All values of total household food and non-food expenditure were converted into year 2018/9 Ethiopian Birr.
Table 1. The Villages and Number of Sample Taken
4. Methods of data analysis
To examine the factors influencing the poverty of rural households at different quantiles of the consumption expenditure per capita was estimated using quantile regression.
5. Quantile regression model
In linear regression analysis, estimate the conditional mean of the consumption expenditure given a set of explanatory variables using the ordinary least square (OLS) method. The estimated coefficients in the regression mean to represent the average change in the consumption expenditure associated with a change in the related explanatory variable. While the quantile regression model as developed by Koenker and Bassett (Citation1978) is used to estimate poverty determinants in different points of the expenditure distribution. It has the advantage of allowing parameter variation across quantiles of the consumption expenditure distribution. The estimated coefficients of the quantile regression are not sensitive to outliers of the dependent variable than OLS, and the quantile estimator is more efficient than linear regression when errors are not normally distributed i.e., it is a robust method (Buchinsky, Citation1998; Cameron & Trivedi, Citation2005). Bootstrapped techniques were used to compute the standard errors to be more efficient and consistent estimates (Koenker & Hallock, Citation2001). It also enables researchers to pre-define any positions of the distribution according to their specific inquiries (Hao & Naiman, Citation2007).
In this study, to examine the determinants of poverty at different expenditure quantile of rural households using quantile regression model is presented below;
Where is the natural logarithm of per capita expenditure per year of
household and,
indicates
a vector of characteristics of the
household.
are unknown parameters for quantile
and
represents the disturbance term and for a random response variable Y with probability distribution function F(y) = Pr(Y ≤ y), the θth quantile of Y is defined as the inverse function Q(θ) = inf{y: F(y)≥θ}, where 0 < θ < 1, the quantile regression for a given quantile
is obtained from the minimization of the objective function F over
For θ = 0.5, the quantile regression is reduced to the median regression. This study fitted a simultaneous quantile regression model divided at ϴ = 10, 15, 50, 60, and 80 quantile levels, with standard errors estimated by bootstrapping with 100 replications (Table ). To examine the conditional distribution of expenditure per capita (natural log) as a function of explanatory variables in rural households in Ethiopia at the mean and various other points (levels) of the expenditure per capita distribution in 2018/19. The linear regression model was estimated and presented in Table for the comparison of these results. Finally, as suggested by Koenker and Machado (Citation1999) for quantile regression analysis the goodness-of-fit is tested.
Table 2. Summary Statistics for Continuous Variables
Table 3. Frequency Distribution of Categorical Variables
Table 4. Total Poverty Measures by Village and Gender Using the Sampled Household
Table 5. Food Poverty Measures by Village and Gender Using the Sampled Household
Table 6. Simultaneous Quantile Regression and Linear Regression Results
6. Empirical results and discussion
6.1. Descriptive results
This part of the study presents descriptive results use data containing 350 households in three selected villages of Bahir dar zuria district. Table shows the descriptive statistics on the household and community characteristics of farmers for sampled data. The expenditure variable is an average yearly expenditure per household size. The average yearly expenditure per household size for 2018/19 is 12,542.56 birr. Yearly expenditure per capita ranges from 1198.4 birrs to 734,962 birr, which represents the broad range of cross-sectional expenditure groups.
It can be observed from Table that the average total family size of the rural household was 5.3. Adult equivalent family size is also 3.8. Education is measured as the total number of years of schooling. The average years of schooling of rural farm household head in the study area is 2.1 years. Age is measured in years. The average age of the household heads was around 45 years. The dependency ratio is calculated as the ratio of the number of family members not in the labour force (children aged 0 to 14 years plus old-aged greater than 64 years) to those in the labour force in the household. A high dependency ratio will be associated with greater poverty. The average dependency ratio of the sampled household is 0.6. The average total landowner for the rural household head is 5.6 timad (1.4 hectares). Distance to the district market from residence is an important factor in analyzing poverty. So, the average distance to the district market from a residence in minutes of walking time is 44.17.
The result of the descriptive analysis also reveals that the village is a dummy variable, Gedro is the largest village in the study area and it accounts for 45.14 percent of the sampled population lives in this area. Table shows that the majority (84%) of rural household heads in the sample are male. The marital status of the household head is a categorical variable that zero represents single, one represents married, two represents divorced and three represents widowed. Married household heads comprise 92% of the sampled household. Sixty-six percent of the household head has no education and 24.29 percent of household heads have primary education. This shows that still, education access to adults remained low. During the last 12 months, 47.7 percent of the rural household in sampled villages received credit service from formal and informal sources. Moreover, most health condition of the household head is good (56%) and excellent (39.14%).
The major source of rural household income can be classified into the farm, off-farm, and non-farm sources. Farm income is earnings from crops grown in farmers’ fields, animal production, rents of farm durables, and by-products of crops and animals. Off-farm income is wages received by working on other farms either in the farmer’s village or in neighbouring villages. Non-farm income sources include all income from wages earned from business and other non-farm activities, pensions, and remittances. For this study considered non-farming occupation represents non-farm plus off-farm income activities. Hence, rural households were participated in different types of employment activities to generate income. So, the major source of livelihoods (occupation) of the rural household head in Bahir dar zuria district is farming (crop, livestock, and its products) which is 88.86 percent while the remaining is non-farming. The non-farming in this area includes seedling business, petty trade, handicrafts, selling of local beverages, building construction (carpenter, plasterer), security guard, daily labourer, religious leader, and others (Table ). Also, as shown in Table , from different sources of rural household income the highest yearly average income source was obtained from the sale of crops. Thus, the mean yearly income of households from; sale of crops (36233.09 birrs), sale of livestock and its products (11644.25 birrs), and non-farm plus off-farm livelihood activities (19789.84 birrs). These are the main sources of livelihood contributing to the yearly income of rural households.
6.2. Measuring poverty
Poverty is defined as pronounced deprivation in well-being (World Bank, Citation2000), where well-being can be measured in different approaches. The approaches used for this study of wellbeing, poverty is measured by comparing an individual’s income or consumption with some defined threshold below which they are considered to be poor (World Bank Institute, Citation2005).
For quantitative analysis of poverty, the most common approach to measure rural household welfare in developing countries like Ethiopia is based on household consumption expenditure approach than income. For the reason that consumption can be a better indicator of lifetime welfare than income since it remains fairly stable and households may be more able or willing to recall what they have spent rather than what they earned (World Bank Institute, Citation2005). Moreover, households had few incentives to under-report (Deaton, Citation1997). Hence, consumption expenditure was used in this study to assess poverty. Consumption includes both goods and services that are purchased and those provided from one’s production. The real per capita consumption expenditure is obtained by dividing consumption expenditure by family size (Deaton & Zaidi, Citation2002). Then, total household expenditure per capita is the measure of welfare assigned to each member of the household. But, consumption has to be adjusted for differences in the calorie requirement of different household members (age, gender). This adjustment can be made by deflating household consumption by an adult equivalent scale that depends on the nutritional requirement of each family member (Dercon & Krishnan, Citation2000).
6.3. Constructing the poverty line
To identify the poor households first set a poverty line. So, the cost of basic need method is the most acceptable approach of poverty measurement to construct the poverty line. In measuring poverty, food constitutes three-quarters of the spending of poor households and then it represents the very basic needs of the household (World Bank Institute, Citation2005). Therefore, it should be included in the poverty line. For the cost of a basic need method, the following steps were used to calculate the food poverty line. First, select the food items commonly consumed by the majority of the poor households, 17 food items and its calorie content (Table ) were identified based on the Ethiopian nutrition and health research institute: EHNRI (Citation2000). Second, each food item in the bundle of goods is weighted with the appropriate unit of measurement (number, kilogram, and litre). Third, each unit of the food items consumed by a household in a week is divided by the corresponding number of adult equivalent (Table ) members of the household to get the number of kilograms each adult gets in a week. Then, calculate the kilocalorie content of the food consumed in kilograms for each adult gets in a week. Fourth, sum all food in kilocalorie per adult equivalent consumed in a week to get the weekly requirement and divide by seven days to compute the daily calorie intake per adult equivalent for each household. Lastly, assuming 2200 kcal per adult equivalent to being the minimum calorie required per adult equivalent per day in Ethiopia (MOFED, Citation2012). Therefore, this study estimated the cost of meeting this food energy requirement. The food poverty line is determined 101.28 birrs per adult equivalent per week (Table ). Then a specific allowance for the nonfood goods consistent with the spending pattern of the poor is added to the food poverty line. To account for the non-food expenditure, the food poverty line is divided by the food share of the poorest 25 percent of the population. Then, this food poverty line is divided by the food share of the poorest twenty-five percent of the population (0.621) to arrive at the absolute poverty line. So, the absolute poverty line is determined by 163.09 birrs per adult equivalent per week. Thus, households whose weekly per adult consumption falls below 163.09 birrs are considered to be poor (in poverty).
6.4. Poverty indices
This study measured household poverty in rural Ethiopia using the consumption expenditure method. After the poor have been identified, the most commonly used poverty indices such as headcount index; the poverty gap, and the squared poverty gap were computed. These three measures of poverty can be thought of as being generated by a family of measures proposed by Foster et al. (Citation1984), also called the FGT (Foster-Greer-Thorbecke) measure. It can be defined as:
Where n is the sample size, denotes the number of the poor,
denotes the consumption per adult of household i, z is the poverty line, the parameter α is a measure of the sensitivity of the index to poverty. The poverty gap for individual i is given by
(with
). When the parameter α = 0, the measure is the headcount index (
) is the proportion of the population that is counted as poor when α = 1, the poverty gap index (
) is shown the extent to which individuals fall below the poverty line and computes that as a percentage of the poverty line (Foster et al., Citation1984), and when α = 2, the poverty severity (squared poverty gap) index (
) is used to address the problem of inequality among the poor and it reflects the severity of poverty (World Bank Institute, Citation2005).
This paper examines the extent of poverty across the rural villages of the Bahir dar zuria district. Hence, the poverty indices were calculated using the total poverty line 163.09 birr per adult equivalent per week. Accordingly, aiming to assess how many people are poor, but also how poor they are. Table gives the headcount, poverty gap, and poverty severity index for the overall sample of households of all the villages combined and for Gedro, Sesa beret, and Mikael villages, respectively. Using a consumption-based measure of poverty; the estimates show that 19.43 percent of the sample households are poor, living with poverty. The poor households are 4.7 percent (7.67 birrs) below the poverty line with a poverty severity index of.018. As it can compare the incidence of poverty was highest in Gedro village (31.01%) followed by Michael (17.24%), which is located in Bahir dar zuria district, Ethiopia. Relative to these three villages, Sesa beret had the lowest proportion (3.81 percent) of poor people. It is also noted that both the poverty gap and severity index was lowest in Sesa beret relative to the rest of the villages whereas higher in Gedro. Hence, Gedro is the most vulnerable village whereas Sesa beret is a relatively best village in Bahir dar zuria district of Ethiopia. It also presents poverty indices by the gender of the household head. It shows that relative poverty is more severe for male-headed households. The results show that 20.07% of the male-headed households were poor as compared to 16.07% headcount poverty for female-headed households.
A popular measure of inequality is the Gini coefficient, which can be calculated from the household income, expenditure, or any welfare indicating data after sorting the observations. The inequality varies from zero to one, with zero showing that income is equally shared and distributed. When it approaches one, income is skewed to specific groups, with an unfair distribution. The distribution of total household income and expenditure, income per capita and spending per adult equivalent show the disparities across villages surveyed and also between the poor and the non-poor rural households (World Bank Institute, Citation2005).
Thus, Table also shows the results for the Gini coefficient for consumption expenditure and indicated that the extent of income inequality measures among villages of rural households is 37.99 percent. This overall Gini index is an indication of the existence of income variation among households. Specifically, the highest income inequality (52.26%) is observed in Sesa beret compared to households relative to other villages. The Gini index for Sesa beret more than fifty percent which is shown as an unfair distribution of income. These results show that the inequality was higher than the result of the previous studies by FDRE (Citation2018) showed that income inequality measured by the Gini coefficient of consumption expenditure using 2015/16 data in rural Ethiopia is 28.4. Moreover, lower-income inequality was also found for female-headed households (0.3541) as compared to the counterpart.
Moreover, assess the poverty status of the rural household by using the food poverty line that is 101.28 birr. Table showed that 18.85 percent of the respondents were found to be living below the poverty line. And also with a poverty gap index of 4.99 percent and a poverty severity index of 2.19 percent. The incidence of poverty can be compared among selected villages of Bahir dar zuria district; the highest level of poverty (29.75%) is noted in Gedro village, with a poverty gap index of .0866 and a poverty severity index .0406. In this village, almost 30 percent of respondents are living with a level of food consumption below the minimum calorie intake required to sustain life.
As shown in Table , the lower level of poverty is observed in female-headed households than the counterpart. So, it had 16.07 percent of the rural households are poor, 3.24 percent of them were living below the poverty line. The poverty severity index was 0.019. Hence, these female-headed households need 3.28 birr per adult equivalent to reach the minimum required calorie to sustain life. Consider the poverty gap index; the amount of money required to female-headed households is 1.77 birrs less than the population income shortfall. This outcome might be due to the support of the government, NGOs, the surrounding people, and relatives to female-headed households. On the other hand, the male-headed household has the highest percentage of poor furthermore has a larger poverty gap and high poverty severity index. They need 5.39 birr per week for each adult equivalent to reach the poverty line.
6.5. Determinants of poverty in rural households
The regression result presented here is very important for identifying the main cause of poverty in different levels of consumption expenditure of a rural household is very crucial to overcome the root causes by designing policy interventions. Therefore, this study used the quantile regression model.
7. Quantile regression results
It is based on the behaviour of different consumer groups. Households were classified into different categories using consumption expenditure per capita. So, households in the sample are divided into five groups (quintiles) based on their yearly consumption expenditure per capita values. Table shows the estimated results of the quantile regressions on the determinants of consumption expenditure per capita (poverty) for rural households. Quantile regression analysis is the kind of analysis that attempts to explain the level of expenditure per capita as a function of a variety of explanatory variables. It also estimates how closely each independent variable is related to the various conditional quantiles of expenditure per capita, holding all other influences constant. Therefore, develop more detailed and accurate information from the expenditure on all different levels of expenditure groups. Estimate using five different quantile levels, 10%, 15%, 50%, 60%, and 80% is reported. For comparison purposes, report the results of both quantile regression and OLS regression estimates of the expenditure per capita. Table reports linear regression results and quantile regression results for 10th, 15th, 50th, 60th, and 80th quantiles. All quantile estimates are qualitatively similar to the OLS results, and the median regression results are very close to the OLS estimates. A comparison of the OLS estimates and those from the quantile regression shows that the estimated coefficients retained their signs but their magnitude differed across quantiles.
As evidenced in Table , it can be observed that the goodness of fit of the quantile regression at each of the five expenditure quantiles was assessed using pseudo-R-square by Koenker and Machado (Citation1999). The values of pseudo-R-square at 10th, 15th, 50th, 60th, and 80th quantiles, together with the value of the measure of goodness-of-fit for the OLS (R-squared) are shown in the last row of Table ; where the value of pseudo-R-square increases with the quantile being increased.
In the quantile regression result, the interpretation of a statistically significant variable namely positively associated with household expenditure per capita decreases the poverty of the rural household whereas negatively associated with the household expenditure per capita increases the poverty of the rural household. The findings remarked that the total family size of the household has a statistically significant negative effect at all five quantiles of the expenditure distribution of rural households as well as in OLS. Besides, the coefficient decreases with increasing the quantiles from −0.1527 to −0.1560 for 0.1 and 0.15 quantiles, respectively. Hence, the total family size of the rural household can increase the poverty of the rural household. This implies that the household having more economically inactive members (large family size) means that generating less income of the household and hence raising the risk of rural households falling into poverty. This result supports previous studies by Tsehay and Bauer (Citation2012). But this study not consistent with Teka et al. (Citation2019).
Table shows that the total land ownership of the household is statistically significant at most of the quantiles of the distribution while not significant for OLS. The total landowner of the household head is positively associated with the household expenditure per capita but is only significant at 50th, 60, and 80th percentile. The coefficients increase from 0.021 to 0.028 with increases from 50th to 60th percentiles. Thus, total land ownership is an important factor that decreases the poverty of rural households. This study in line with previous studies in Ethiopia by Tsehay and Bauer (Citation2012) which confirmed that the size of cultivated land increases the welfare of households positively indicating that land is a crucial asset for mixed farming smallholders. Previous studies have also reported similar results as Kassie et al. (Citation2014) in Zambia and Malawi, an increase in the proportion of farm area allocated to maize and continuous use of improved maize varieties were found to strongly and positively influence the wellbeing of rural households. Moreover, this result is supported by the previous studies done by Kebede and Sharma (Citation2014); Bigsten and Shimeles (Citation2003).
The result also showed that distance to the district market is positively associated with household expenditure per capita but significant only at the 80th percentile. As a result, the distance to the district market can decrease the poverty of rural households, which improves the living standard of rural households. This result is also confirmed by Dercon et al. (Citation2007) that road access has contributed to poverty reduction. Reduced distance to the nearest small town by about 12 kilometers also brings down the probability of being chronically poor by about 38 percent. Better roads in the localities make it easier for households to access local market towns which are in turn linked to larger urban centres. As smaller cities are more closely located to the rural hinterland, the production and marketing linkages for agricultural products could be stronger because of lower transportation costs and stronger local ties (Richards et al., 2016).
The estimates of simultaneous quantile regression in Table indicated that non-off-farm income of the household is positively associated with household expenditure per capita and it is significant at 10th, 15th, 50th, 60, and 80th quantiles in addition to OLS. Therefore, non-off-farm income can decrease the poverty of the rural household that means improve the living standard of the rural household. This finding is consistent with author Tsehay and Bauer (Citation2012) that are engaged in off-farm activities correlate positively with the welfare of households. According to the World Development Report (Citation2008), off-farm labour in agriculture and the rural non-farm incomes can enhance the potential of farming as a pathway out of poverty.
The estimated result in Table shows that the health status of the household head is a categorical variable; the excellent health status of the household head is the reference group for comparison with other health status categories. It can be observed that the household head that has bad health status is negatively associated with household expenditure per capita in the 50th quantile (−0.2457, p-value<.1) as compared to the excellent health status of the household head. So, the bad health status of the rural household head can increase the poverty of the rural household. This is unlike the FDRE (Citation2018). But, the study by Dercon et al. (Citation2005) using a multi-shock data also confirmed that droughts and illness shocks occurring in the five years before the survey reduce total per capita consumption.
8. Conclusions
This study examines the extent and determinants of rural household poverty based on consumption expenditure in Bahir dar zuria district, Ethiopia. This paper presents a quantile regression model used to find out which households and community characteristics have an impact on poverty. Quantile regression analysis was used to develop a more detailed analysis of how individual variables affect different expenditure groups differently while OLS analysis that describes only average statistics.
The poverty estimates were based on consumption expenditure as a measure of household welfare using the Foster-Greer-Thorbecke (FGT) measure; about 19.43 percent of rural households were poor. There was a high incidence of poverty in Gedro (31.01 percent) relative to the rest villages of the rural household. The income inequality measured by the Gini coefficient of consumption expenditure was 37.99 percent and indicated that the existence of income variation among rural households. Especially, the highest income inequality is observed in Sesa beret compared to rural households relative to other villages. This study includes different characteristics of rural household poverty. From the summary of the results, the major determinants of rural household poverty in Bahir dar zuria district were total family size, total land ownership, distance to district market, non-off-farm income, and bad health status of the household head.
It concluded that the total family size and bad health status of the household head can increase the poverty of the rural household which is lower the standard of living of rural households. Similarly, for rural households having additional family size the likelihood of being poor was increased. While the distance to the district market and non-off-farm income can decrease the poverty of the rural household. Also, it showed that the total land ownership of the household can decrease the poverty of the rural household. It means that an increase in land owned can reduce the likelihood that the rural household is being poor in Bahir dar zuria district, Ethiopia. This paper contributes to the empirical literature by examining the contributions of different factors to the poverty of rural households at different levels of expenditure per capita. Thus, to reduce the poverty of the rural household, government policies and programs should give more emphasis on family planning, the necessity of health insurance, and improving the service of health institutions to bring overall household welfare development in rural areas. Moreover, it promotes rural households to participate in off-farm and non-farm livelihood activities beyond agriculture to diversify their employment.
Competing Interests
The author declares no competing interests.
Additional information
Funding
Notes on contributors
Anteneh Mulugeta Eyasu
Anteneh Mulugeta Eyasu is M.Sc. in Applied Statistics at Hawassa University, Ethiopia. His research interest includes econometric analysis of; socio-economic issues, crop production, poverty, gender, climate change, corporate social responsibility, and marketing.
References
- Bersisa, M., & Heshmati, A. (2016). Poverty and wellbeing in East Africa a multi-faceted economic approach. Springer International Publishing Switzerland. http://doi.org/10.1007/978-3-319-30981-1_1
- Bigsten, A., & Shimeles, A. (2003, May 30-31). The dynamics of poverty in ethiopia. Paper prepared for a WIDER Conference on Inequality, Poverty and Human Well-being, Helsinki, Finland.
- Buchinsky, M. (1998). Recent advances in quantile regression models: A practical guideline for empirical research. Journal of Human Resources, 33(1), 88–20. https://doi.org/10.2307/146316
- Cameron, A. C., & Trivedi, P. K. (2005). Microeconometrics: Methods and applications. Cambridge University Press.
- Christiaensen, L., De Weerdt, J., & Kanbur, R. (2016). Urbanization and poverty reduction: The role of secondary towns in Tanzania (No. 18). Universities Antwerp. Institute of Development Policy and Management (IOB).
- CSA. (2007). Population and housing census report. Addis Ababa, Ethiopia: Office of the Population Census Commission.
- CSA. (2012) . Country and regional level consumer price indices. Addis Ababa: Central Statistical Agency.
- Deaton, A. (1997). The analysis of household surveys: A micro econometric approach to development policy. Johns Hopkins University Press for the World Bank.
- Deaton, A., & Zaidi, S. (2002). Guidelines for constructing consumption aggregates for welfare analysis. Living standards measurement study working paper, 135, 104. xi, Washington, D.C.: The World Bank.
- Dercon, S., Hoddinott, J., & Woldehanna, T. (2005). Consumption and shocks in 15 Ethiopian Villages, 1999-2004. Journal of African Economies, 14(4), 559–585. https://doi.org/10.1093/jae/eji022
- Dercon, S., Hoddinott, J., & Woldehanna, T. (2007). Growth and poverty in rural Ethiopia: Evidence from 15 communities 1994-2004. Chronic Poverty Research Centre.
- Dercon, S., & Krishnan, P. (2000). Vulnerability, seasonality, and poverty in Ethiopia. Journal of Development Studies, 36(6), 25–53. https://doi.org/10.1080/00220380008422653
- EHNRI. (2000). Food composition table for use in Ethiopia. Ethiopian Health and Nutrition Research Institute.
- Ethiopia Poverty Fact Sheet. (2018). Poverty and intergenerational change: Preliminary findings from the 2016 young lives survey (round 5). Young Lives Ethiopia.
- FDRE. (2017). Ethiopia’s progress towards eradicating poverty: An interim report on 2015/16 poverty analysis study. National Planning Commission.
- FDRE. (2018). Poverty and economic growth in Ethiopia 1995/96-2015/16. Planning and Development Commission.
- Foster, J., Greer, J., & Thorbecke, E. (1984). A class of decomposable poverty measures. Econometrica, 52(3), 761–766. https://doi.org/10.2307/1913475
- Foster, J. E. (2007). A class of chronic poverty measures. Working Paper No. 07-W01. Department of Economics, Vanderbilt University, Nashville, USA.
- Gillis, M., Shoup, C., & Sicat, G. P. (2001). World development report 2000/2001, attacking poverty. the World Bank.
- Gunther, I., & Harttgen, K. (2009). Estimating households vulnerability to idiosyncratic and covariate shocks: A novel method applied in Madagascar. World Development, 37(7), 1222–1234. https://doi.org/10.1016/j.worlddev.2008.11.006
- Hao, L., & Naiman, D. Q. (2007). Quantile regression. Sage Publications.
- Hill, R., & Porter, C. (2017). Vulnerability to drought and food price shocks: Evidence from Ethiopia. World Development, 96(2017), 65–77. https://doi.org/10.1016/j.worlddev.2017.02.025
- Hill, R., & Tsehaye, E. (2014). Growth, safety nets, and poverty: Assessing progress in Ethiopia from 1996 to 2011. Background Paper prepared for the Ethiopia Poverty Assessment.
- IMF. (2017). World GDP per capita ranking. 2017-data-and-charts-forecast http://ethiopia.opendataforafrica.org/sijweyg/world-gdp-per-capita-ranking-
- Kassie, G. T., Abate, T., Langyintuo, A., & Maleni, D. (2014). Poverty in maize growing rural communities of southern Africa, development studies research. An Open Access Journal, 1(1), 311–323. http://doi.org/10.1080/21665095.2014.969844
- Kebede, T., & Sharma, M. K. (2014). The determinant of poverty in Ethiopia. Ethiopian Journal of Economics, XXIII(1), 113–130.
- Koenker, R., & Bassett, J. G. (1978). Regression quantiles. Econometrica. Journal of the Econometric Society, 46(1), 33–50. https://doi.org/10.2307/1913643
- Koenker, R., & Hallock, K. (2001). Quantile regression. Journal of Economic Perspectives, 15(4), 143–156. https://doi.org/10.1257/jep.15.4.143
- Koenker, R., & Machado, J. A. (1999). The goodness of fit and related inference processes for quantile regression. Journal of the American Statistical Association, 94(448), 1296–1310. https://doi.org/10.1080/01621459.1999.10473882
- Minten, B., Koru, B., & Stifel, D. (2013). The last mile(s) in modern input distribution: Pricing, profitability, and adoption. Agricultural Economics, 44(6), 629–646. https://doi.org/10.1111/agec.12078
- Minten, B., Tamru, S., Engida, E., & Kuma, T. (2015). Transforming staple food value chains in Africa: The case of teff in Ethiopia. The Journal of Development Studies, 52(5), 627–645. https://doi.org/10.1080/00220388.2015.1087509
- MOFED. (2012) . Ethiopia’s progress towards eradicating poverty: An interim report on poverty analysis study (2010/11). Development Planning and Research Directorate Ministry of Finance and Economic Development.
- Shibru, T., Muktar, J., Haji, M., & Yohannes, M. W. (2013). Dimensions and determinants of agro-pastoral households’ poverty in dembel district of somali regional state. Ethiopia. Journal of Economics and Sustainable Development, 4(15), 2013.
- Sisay, B. (2018). Poverty, vulnerability, and resilience in rural Ethiopia: Forward-looking empirical analyses for more effective policy interventions [Ph.D. Thesis]. Addis Ababa University.
- Teka, A. M., Woldu, G. T., & Fre, Z. (2019). Status and determinants of poverty and income inequality in pastoral and agro-pastoral communities. Household-based Evidence from Afar Regional State, Ethiopia, World Development Perspectives, 15(2019), 100123. https://doi.org/10.1016/j.wdp.2019.100123
- Tsehay, A. S., & Bauer, S. (2012). Poverty and vulnerability dynamics: Empirical evidence from smallholders in the Northern Highlands of Ethiopia. Quarterly Journal of International Agriculture, 51(4), 301–332.
- UNDP. (2018) . Ethiopia national human development report. Industrialization with a Human Face.
- UNICEF, Ethiopia. (2018). Amhara regional state 2007/08 – 2015/16. Budget brief.
- University of Oxford. (2017). Ethiopia country briefing: Multidimensional poverty index data bank.
- Vandercasteelen, J., Beyene, S. T., Minten, B., & Swinnen, J. (2018). Big cities, small towns, and poor farmers: Evidence from Ethiopia. World Development, 106(2018), 393–406. https://doi.org/10.1016/j.worlddev.2018.03.006
- Woldehanna, T. (2019). Complexities and dynamics of rural poverty in Ethiopia: 1996-2016. Department of Economics, Addis Ababa University.
- Workneh, N. (2008). Food security and productive safety net program in Ethiopia. In T. Assefa (Ed.), Digest of Ethiopia’s national policies, strategies, and programs (pp. 1–22). Forum for Social Studies.
- World Bank. (2000). World development report 2000/2001: Attacking poverty. Oxford University Press.
- World Bank. (2015). Ethiopia poverty assessment overview. Poverty Global Practice Africa Region.
- World Bank. (2016). Priorities for ending extreme poverty and promoting shared prosperity, systematic country diagnostic (Report No: 100592-ET). Washington, DC.
- World Bank. (2017). Ethiopia GDP per capita PPP https://tradingeconomics.com/ethiopia/gdp-per-capita-ppp
- World Bank Institute. (2005). Introduction to poverty analysis. Poverty Manual, All, J. H. Revision of August 8. World Bank.
- World Development Report. (2008). Rural households and their pathways out of poverty. World Bank.
Appendix
Table A1.Food Items Used to Estimate the Food Poverty Line
Table A2. Nutrition (calorie) based Equivalence Scale