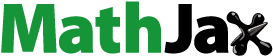
Abstract
The study examined how farmers’ technological choice affects technical efficiency (TE) in small-scale maize production in northern Ghana. A random sample of 340 respondents took part in the study. A binary probit modeling was applied to analyse adoption decision, while double bootstrap data envelopment analysis was relied upon to evaluate TE Truncated regression was used to assess the sources of inefficiency. The results indicated a bias-corrected TE of 57% under variable returns to scale assumption and 52% when constant returns to scale was assumed. Accounting for potential endogeneity of the adoption variable, the findings indicated that technical efficiency increased with technology adoption. Technical efficiency improved with herd size but decreased with educational attainment, number of household members, access to extension, frequency of weeding, and size of cultivated land. The study, therefore, recommends that making improved seeds available and affordable to small-scale producers and training farmers on effective weed management could be potential measures to enhance the farm-level technical efficiency.
PUBLIC INTEREST STATEMENT
Low agricultural productivity is a major concern in smallholder agriculture because of the important role agriculture plays in rural livelihoods and socio-economic development in developing countries. A major factor contributing to low agricultural productivity is low adoption of improved technologies including improved planting materials. Despite efforts by research institutions to develop and disseminate improved technologies to smallholders, adoption of these technologies has been low in many developing countries. This study, therefore, assessed the factors influencing adoption of improved maize seeds and the impact of adoption on technical efficiency of smallholder farmers in Ghana. The study adopted a non-parametric efficiency approach. The results indicated a positive and significant effect of improved variety adoption on technical efficiency. Hence, there is the need to encourage farmers to adopt improved varieties and follow recommended practices to optimize farm output. Improving farmers’ access to input subsidy is required to promote technical efficiency of maize production.
Competing interests
Authors declare there are no competing interests regarding this research.
1. Introduction
Agricultural growth through higher productivity is a topical issues that has gained global attention for the past decades because of its influence on food and nutrition security (FNS) and long-term expansion of the economy (Collier & Dercon, Citation2014). Typically, developed countries have been able to enhance productivity at the farm level by adopting improved technologies and access to productivity-enhancing resources such as credit, land, and markets. Improved agrarian technologies adopted include improved crop varieties, weed, insect pest management, farm equipment, and conservation agriculture (Asfaw et al., Citation2012; Reardon et al., Citation2009; Sheahan & Barrett, Citation2017). According to Lee (Citation2005), the adoption of agricultural technologies has the potential to increase farm yields, increase farmers’ income, and ensure food security. Despite the benefits of improved agricultural technologies, adoption is low in several less-developed countries (Abebe et al., Citation2013; Jayne & Rashid, Citation2013; Sheahan & Barrett, Citation2017), including Ghana.
Ghana is an agrarian economy, with the agricultural sector providing jobs to about 71% of rural dwellers (Ghana Statistical Service, Citation2014a). In most rural communities, where agricultural production is the predominant livelihood, poverty, and food insecurity are rife (Ghana Statistical Service, Citation2014b). Agricultural development is therefore pivotal to eradicate poverty and enhance economic development. Indeed, promoting agricultural productivity can improve the welfare of rural farm households through higher income (Asfaw et al., Citation2012). Evidence suggests that the uptake of improved planting materials improves yield and thus household welfare as well as food and nutrition security (Donkor et al., Citation2016; Sheahan & Barrett, Citation2017).
The government of Ghana has put in place initiatives to improve the productivity of smallholder farming. The Food and Agriculture Sector Development Policy (FASDEP II) was initiated in 2007 to enhance productivity. Similarly, the Ghana Shared Growth and Development Agenda (GSGDA 2010–2013) and Medium-Term Agriculture Sector Investment Plan (METASIP 2010–2015) have all acknowledged the importance of boosting agricultural productivity of staple crops by providing farmers access to improved technologies and other productive resources such as credit and irrigation facilities. The most recent of the comprehensive strategies set up by the government is the Planting for Food and Jobs (PFJ) programme increase the production and yield of maize and other crops such as rice, soybeans, sorghum, and vegetables through the provision of subsidized inputs such as fertilizer, improved planting materials, and extension advice (MoFA (Ministry of Food and Agriculture), Citation2017).
In spite of these efforts, the type of seed use by farmers remains a major challenges affecting farm productivity. For instance, farmers’ uptake of improved maize varieties (IMVs) is still below expectation, with production at the subsistence level. In Ghana, there are several seed types available and used by farmers. These include certified inbreeds seeds, hybrid seeds, and farmers’ recycled seeds or what is tagged as ordinary seeds. In the context of this study, IMVs are those certified inbreed seeds and hybrid seeds released and introduced to farmers by national research bodies such as Savanna Agricultural Research Institute (SARI) and the Crops Research Institute (CRI). These are open pollinated varieties and quality protein maize with higher yielding potential (usually 3.5–5.5 tons/ha) and early maturing (75–110 days). Other features include drought, pests and disease tolerance, resistant to lodging and striga infestation, among others. Although most of the IMVs released can be cultivated across almost all the agro-ecologies in Ghana, some are most suited to the Sudan and Guinea savannah, which constitute the northern part of Ghana. Some of the varieties most suited and hence, are mostly used by farmers in northern Ghana, including the study area include Dodzi, CSIR-Abontem, Sanzal-sima, Ewu-boyul, Wang-dataa, Bihilifa, Tigli, and Obaatanpa, among others. It is expected that farmers adopt these varieties to increase farm productivity, and subsequently improved their livelihoods.
However, low adoption of these IMVs by smallholders has contributed to farm-level inefficiencies. Thus, efforts geared towards identifying the drivers of IMV adoption and its effect on production efficiencies are critical in enhancing the productivity of farmers. This is because the judicious use of productive resources required to enhance smallholder production systems, thus promoting economic growth. This study, therefore, aimed at investigating the influence of adopting IMVs on farm efficiencies in the Tolon district, Ghana.
Some empirical investigations have examined the influence of adopting modern technologies on farmers’ technical efficiency (TE) levels. Principal among such studies that are similar to this study are Asante et al. (Citation2019), Azumah et al. (Citation2019), Rahman and Norton (Citation2019), and Abdulai et al. (Citation2018). The point of departure from this study is that Asante et al. (Citation2019) employed the metafrontier model and found the adoption of fertilizer to increase maize farmers’ technical efficiency level in Ghana. Azumah et al. (Citation2019) applied stochastic frontier analysis (SFA) and reported that the adoption of an irrigation system enhanced the technical efficiency level of Ghanaian rice producers. Rahman and Norton (Citation2019) also used the SFA and found that the decision to adopt integrated pest management (IPM) did not influence the technical efficiency level of Bangladeshi eggplant growers. Nkegbe (Citation2018) used the double bootstrap data envelopment analysis (DEA) approach to estimate the impact of agricultural credit on smallholders’ TE in northern Ghana. The study was a pioneering work in terms of the estimation of technology adoption within a non-parametric technical efficiency framework in relation to Ghanaian agriculture. The study, however, did not account for endogeneity of the credit variable. This study extends the literature further by evaluating the impact of IMV adoption on the technical efficiency of maize farmers within a non-parametric framework, specifically the data envelopment analysis. Also, we accounted for endogeneity of the adoption variable by including the predicted adoption scores rather than the observed adoption variable in the estimation.
2. Literature review
TE is attained when an economic agent is able to apply minimum quantities of production factors to realize a given output quantity (i.e., input-oriented), or for an economic agent to obtain feasible maximum output using a given set of resources (i.e., output-oriented) (Coelli et al., Citation2002). The ability to produce efficiently is important because most smallholders are resource-constrained and depend largely on agriculture for their livelihoods. According to Singh et al. (Citation2002), a smallholder farmer is a producer who cultivates an average of 2 ha. Improving the TE of production will improve the level of productivity thus enhancing farm income and household food security. Estimating the level of efficiency provides insight into the resource-use efficiency of farmers, while evaluating the sources of inefficiency gives insight into the measures necessary to reduce inefficiency in production. Theoretically, the two main approaches for TE estimation are stochastic frontier analysis (SFA) and data envelopment analysis (DEA). The SFA model is parametric in nature, accommodates noise in the data, and based on defining a functional form and a model for the source of inefficiency (Kumbhakar et al., Citation2009). DEA on the contrary, is non-parametric and requires no a priori assumption of the relationship between inputs and outputs. The DEA approach is however deterministic in nature and does not require a model identifying the sources of inefficiency.
Motivated by these arguments, various empirical studies have been conducted using the input-oriented DEA technique to evaluate the efficiency of firms or economic agents. Balcombe et al. (Citation2008) applied the DEA double bootstrap technique and found a mean technical efficiency of 64% (at constant returns to scale, CRS) and 59% (at variable returns to scale, VRS) for rice farmers in Bangladesh. They observed that access to credit, extension, and higher education increased farmers’ technical efficiency, while age decreased efficiency. Using the DEA double bootstrap approach, Wouterse (Citation2010) found that continental migration increased the TE of Burkinabe farmers. Also, Mitra and Yunus (Citation2018) applied the DEA double bootstrap procedure and observed the mean TE of tomato producers in Bangladesh to be 83%. The study revealed that efficiency increased with education, and training but decreased with age. These studies, and many others, indicate that farmers are not making efficient use of their scarce resources as revealed by the presence of inefficiency in production. Furthermore, the studies reviewed highlight several sources of inefficiency in production, including farm and farmer characteristics, socio-economic and institutional factors. Our choice of variables for evaluating the factors affecting technical efficiency of Ghanaian smallholders was informed by previous studies and a priori expectation.
In terms of empirical studies on the impact of adoption of agricultural technologies on the TE level of farmers, different econometric techniques, crop types, and agricultural technologies have been used with conflicting results. For example, Benedetti et al. (Citation2019), based on stochastic frontier analysis (SFA), found that the adoption of a fertigation system increased the level of TE among Italian farmers. In a similar study, Rahman and Norton (Citation2019) applied SFA and found no significant influence of adoption of integrated pest management (IPM) on the TE level of eggplant growers in Bangladesh. Using the meta-frontier model, Asante et al. (Citation2019) found that the adoption of fertilizer increased the technical efficiency of maize farmers in Ghana. Azumah et al. (Citation2019) employed the SFA correcting for sample selection bias and found the adoption of irrigation system to increase the technical efficiency level of Ghanaian rice producers. These studies highlight different approaches used in estimating the impact of technology adoption on TE. Most of the studies reviewed are aligned to the SFA tradition. In this study, we apply the DEA methodology to assess the effect of technology adoption on TE of smallholder farmers in the context of a developing country.
Although there are existing studies on technology adoption by smallholder farmers in Ghana, the results are conflicting and relate to different technologies and crops. Importantly, there is the need for scientists to regularly update their research findings because the factors affecting technical efficiency are specific to time, geographic location and crop type (Donkoh, Citation2011).
3. Methodology
3.1. Study area and data
Maize is Ghana’s major staple food crop and the most significant source of food security (Wongnaa & Awunyo-Vitor, Citation2018). Also, maize production is an essential source of income in areas where it is cultivated and has featured significantly in most national public documents because it has the potential to enhance food security (MoFA (Ministry of Food and Agriculture), Citation2017). Despite these roles of maize, Ghana has experienced average shortfalls in domestic maize supplies. This is primarily due to low productivity, as yields remain low around 1.99 metric tonnes per hectare for Ghanaian farmers in 2016 instead of an achievable yield level of 5.5 metric tonnes per hectare (MoFA (Ministry of Food and Agriculture), Citation2016).
The study was conducted in the Tolon district (Figure ), which is located in the Northern region of Ghana. Tolon is a rural district, with the majority of the people engaged in crop farming (97.5% of households), predominately as small-scale producers (Ghana Statistical Service, Citation2014c). Maize is widely cultivated by small-scale farmers in the district, which per the 2010 national population census, had 72,990 inhabitants (Ghana Statistical Service, Citation2014c). Located in the dry savanna zone, the district experiences one rainy season, from April/May, and ends in October/November. Average daily temperatures are very high (reaching over 40 degrees Celsius during the peak). Crop production depends mainly on natural rainfall which does not exceed 1,200 mm per annum.
Cross-sectional data were obtained from 340 smallholder maize farmers from 11 communities in the district for the 2018 farming season. Multistage sampling was used for data collection. Stage one involved selection of the Tolon district as a major maize cultivating districts in the Northern Region. This was followed by the random selection of 11 communities in the district. In each community, 30–35 maize producers were randomly selected, based mainly on geographical spread of the communities. Without an existing sampling frame, the sample size was determined using the 2010 Population Census estimate of the district’s population. Cochran’s procedure for sample size determination indicated that a sample size of 340 is representative of the population engaged in farming. The respondents were interviewed using face-to-face interviews using semi-structured questionnaires. The majority of the respondents were interviewed in the local dialect with the assistance of interpreters. The information collected from respondents covered input and output quantities, farm-specific variables, socioeconomic, demographic, and institutional factors.
3.2. Technology adoption model
Following Khonje et al. (Citation2015), farm households’ choice of IMVs is analysed under the random utility theory. It posits that rational economic agents (i.e. farm households) will choose to plant IMVs if the utility derived from adoption () exceeds the utility from non-adoption (
). However, the utilities are unobservable and the maximum benefit derived from adoption (
) is an unobservable variable related to farmers’ socio-economic factors, as presented in EquationEquation (1)
(1)
(1) :
where is binary and equals 1 if farmers adopt IMVs and 0 otherwise,
represents unknown parameters,
represents farmer/farm-specific and institutional factors,
is the error term that has a normal distribution. Thus, the likelihood to adopt is presented as
where F is the cumulative distribution function for . Estimation of Equationequation (2)
(2)
(2) is done by employing the probit model (Wooldridge, Citation2010). The model to estimate the probability of observing a farmer adopting IMVs is expressed in EquationEquation (3)
(3)
(3) :
Empirically, the probit model used to estimate adoption is represented as follows:
where Xi represents the factors influencing adoption, and ui is a random error term. The explanatory variables include farmer’s age and its quadratic term, educational level, household size, extension contact, degree of specialization in maize production, soil fertility status, access to credit, farmer group membership, herd size, and participation in off-farm work. After obtaining the probit model estimates, the predicted probability of adoption of IMV was estimated and used as an independent variable in the efficiency model to control for endogeneity.
3.3. DEA model
The study relies on DEA methodology to evaluate technical efficiency and how it is influenced by technology adoption. Unlike the parametric stochastic production frontier approach, the DEA approach does not assume a priori functional form for the input–output relationships and distributional assumptions of the inefficiency term (Farrell, Citation1957; Fraser & Cordina, Citation1999). Specifically, the DEA input-oriented approach was used because maize farmers do not have much control over the level of their output as compared to their inputs. Following Farrell (Citation1957), the input-oriented model for individual farm unit or DMU is calculated by using the following model:
;
and
is a scalar indicating technical efficiency scores of the jth maize farmer or DMUs in the sample, λj represents a vector of weight of each firm that is not situated on the efficient frontier, and
is an
input matrix, representing the quantity of use of the ith input on the jth maize farm.
is the quantity of output on the jth maize farm. For this specification, TEi ≤ 1, where a value of 1 indicates that the jth farmer is fully efficient.
The above specification denotes constant returns to scale (CRS), which is ideal given that all farms are operating at their optimum scale. The assumption of CRS is therefore regarded as restrictive, which can be relaxed by including additional constraints. Additional constraint of ,
or
to EquationEquation (5)
(5)
(5) enables technical efficiency to be estimated under assumptions of variable, decreasing or increasing returns to scale.
The maximum efficiency is 1; hence, is the proportion by which producers can potentially reduce input utilization to attain the same production. Truncated regression is subsequently applied to estimate the factors influencing farmers’ efficiency, where the response variable is the estimated TE score. A notable feature of the Simar and Wilson DEA approach that we employ in this study is the double bootstrap, which provides bias-corrected TE scores. According to Simar and Wilson (Citation2007), this procedure, unlike the traditional censored regression, corrects for serial correlation between the efficiency scores. The truncated regression model is expressed as
where is the DEA scores that have been corrected for bias,
with right truncation at 1-
, γ represents unknown parameters, and
denotes farmer/farm-specific and socioeconomic factors hypothesized to affect technical efficiency. The explanatory variables in the vector Zi include the predicted adoption score, farmer’s age and its quadratic term, household size, educational level, degree of specialization in maize cultivation, engagement in off-farm work, farmer group membership, size of herd, farm size and frequency of weeding. These explanatory variables were adopted from previous studies (Asante et al., Citation2019; Azumah et al., Citation2019; Kassie et al., Citation2013; Khonje et al., Citation2015; Konja et al., Citation2019). To address potential endogeneity of the adoption variable, the predicted adoption score was appended as an independent variable in the efficiency model. For this study, 2000 bootstrap replications were used. The data were analysed using Stata version 15.
A common method in efficiency analysis is to use a Tobit model to assess the factors influencing efficiency due to the censored nature of the TE scores. Such an approach has however been criticised because it lacks a well-defined data-generating process. Also, there are serious serial correlations between the estimated TE scores that make conventional approaches to statistical inference invalid. Simar and Wilson (Citation2007), therefore suggested truncated regression as a better approach for the second-stage analysis.
The double bootstrap approach employs a data-generation process that seeks to address the challenges associated with estimating EquationEquations (5)(5)
(5) and (Equation6
(6)
(6) ). The approach follows the following steps: (i) estimate each farm’s efficiency scores using EquationEquation (5)
(5)
(5) ; (ii) Estimate the truncated regression using EquationEquation (6)
(6)
(6) ; (iii) calculate a set of bootstrap estimates; (iv) calculate each farms’ TE score adjusted for bias; (v) the TE scores adjusted for bias serves as the response variable in a truncated regression to estimate the factors affecting TE (vi) conduct a series of bootstrapping to provide bootstrap estimates; and (vii) calculate new confidence intervals based on the bootstrap estimates.
The description, unit of measurement, and expected signs of the explanatory variables in the probit and truncated regression models are shown in Table .
Table 1. Description and expected signs of the explanatory variables in the models
4. Results and discussion
4.1. Characteristics of the respondents
Table presents the summary statistics of the sampled farmers. Adopters had significantly higher maize output and used higher input quantities (labour, fertilizer, and agrochemicals) in production than non-adopters. As expected, the number of visits from extension agents was higher for adopters. On average, farmers received one extension visit during the farming season, which is very low. On the other hand, adopters allocated less of their total agricultural land to maize production. In other words, adopters had a lower degree of specialization in maize production in the study area. Furthermore, adopters were older and had more years of formal education. Adopters also had smaller household sizes and were more likely to participate in off-farm work. The respondents had on average 10 household members.
Table 2. Characteristics of respondents
The respondents had an average farm size of 3.8 acres and a mean maize output of 1253 kg. Furthermore, 42% of the respondents adopted IMVs, while 46.5% participated in a farmer group organization. The adopters of IMVs have a significantly higher average age (40 years) than non-adopters (37 years). The average age of both groups implies that most of the sampled farmers are within their active and productive years. Adopters had more years of formal education and fewer members per household relative to non-adopters. Also, a significantly higher proportion of adopters engaged in off-farm income-generating activities while the respondents had approximately one extension contact during the cropping season, with a minimum of zero and a maximum of five. The respondents also owned an average of two cattle and allocated 52% of the total agricultural land to maize cultivation. Access to agricultural credit did not differ between adopters and non-adopters. On average, the respondents weeded their farms twice during the cropping season, while 54% of the respondents perceived their soils to be fertile.
4.2. Determinants of improved maize variety adoption
Table presents the results of the adoption model. The association between the age of the respondents and adoption was positive and significant at 1%. Older farmers, who are premised to be more experienced in production, are therefore more likely to adopt IMVs. A unit increase in age increases the likelihood of adoption by 0.043. However, the quadratic term of the age variable indicates that adoption increases at a decreasing rate. In other words, beyond a certain threshold, adoption begins to decline with age. The positive effect of age on adoption of IMV agrees with Anang (Citation2019) and Danso-Abbeam et al. (Citation2017) in their studies in northern Ghana but disagrees with that of Islam et al. (Citation2012) in their study in Bangladesh.
Table 3. Determinants of improved maize variety adoption
Farmers’ educational level had a positive correlation with adoption, implying that additional years of formal education positively influenced farmers’ decision to cultivate IMV. An additional year of education has the likelihood to enhance adoption by 0.017. This result resonates with other empirical studies (see Ahmed, Citation2015; Anang, Citation2019; Deepa et al., Citation2015; Ghimire et al., Citation2015) on the adoption of agrarian technology, which points to a positive significant influence of formal education on farm technology adoption. On the other hand, farmers who allocated a higher proportion of their farmland to maize production were less likely to adopt improved varieties at 5% significance level. A unit increase in the proportion of land allotted to maize cultivation decreases the probability of adoption by 0.365. As farmers allocate a greater proportion of their farmland to maize cultivation, the cost of adoption is expected to rise, which may deter cash-constrained farmers from adopting IMVs.
Supply-driven factors like extension services and farmer-based organizations (FBOs) are critical avenues for information dissemination on productivity-enhancing inputs such as IMV. Smallholders’ access to extension services enhanced technology adoption at 1% level, which confirms the findings of other studies (Kassie et al., Citation2015; Kijima & Sserunkuuma, Citation2013). The probability of adoption is 0.093 higher for farmers with access to extension than for their neighbours without extension access. However, being a member of a farmers’ group was associated with a lower likelihood of adopting improved varieties, while farmers who perceived their soils to be fertile had a higher likelihood of IMV adoption. FBO membership decreased the probability of adoption by 0.13, while the probability of adoption was 0.137 higher for farmers who perceived their soils to be fertile relative to those who perceived their soils to be poor.
4.3. Results of the technical efficiency analysis
The bias-corrected efficiency estimates of the sampled rice farms under both CRS and VRS assumptions are presented in Table . Under CRS, TE ranged between 0.1 and 0.91, with a mean score of 0.52. With regard to farm efficiency under VRS, the original scores ranged between 0.21 and 1, with an average score of 0.72, while the estimated scores after bias correction ranged between 0.16 and 0.95, with a mean score of 0.57.
Table 4. Distribution of technical efficiency scores
The findings are comparable to other studies. For example, Kirimov (Citation2013) estimated non-bias and bias-corrected efficiency scores of 67% and 59%, respectively, for potato farms in Uzbekistan. However, our results are lower than those obtained from Abatania et al. (Citation2012), where non-bias and bias-corrected TE scores of 86% and 77% were estimated for farms in northern Ghana. The results further reveal that 39% have TE scores not exceeding 50%, while 61% have efficiency scores exceeding 50%. Besides, 23.5% of the respondents had TE levels exceeding 70%. The results indicate that there is a large scope to enhance the TE of the respondents through improvement in resource allocation at the farm level.
4.4. Determinants of technical efficiency
Farmers’ managerial style and practices guide their less efficient counterparts in production; hence, the need to identify the drivers of inefficiency in production. The factors influencing TE are presented in Table . Although the results from both CRS and VRS are presented, the focus of the discussion will be on the result under the VRS assumption. This is because agriculture faces several external environmental and policy-driven conditions. Thus, it is not tenable to assume CRS for many farmers. The study followed Farrell’s (Citation1957) input-oriented approach in estimating the TE of the respondents. Using this procedure, a positive coefficient of the explanatory variables corresponds to an increase in technical efficiency, and vice versa. To account for endogeneity of IMV adoption, the predicted adoption score rather than the actual (observed) value of adoption was used as the explanatory variable in the model.
Table 5. Truncated regression estimates of the determinants of technical efficiency φ
The results indicate that adoption enhances technical efficiency. This result is supported by the findings of other authors such as Owusu (Citation2016) who reported a 6%—8% increase in technical efficiency as a result of IMV adoption by Ghanaian smallholders. Similarly, Ahmed et al. (Citation2017) observed that adopters of IMV in Ethiopia were 4.2% more technically efficient than non-adopters. Obayelu et al. (Citation2016) also noted that Nigerian smallholders who adopted improved protein maize recorded higher technical efficiency than those who did not adopt.
Furthermore, years of formal education and farmers’ technical efficiency were inversely related at 1% significance level, suggesting that being less formally educated enhanced farmers’ technical efficiency. The result is at variance with a priori expectation but consistent with that of Anang et al. (Citation2016) on rice production in northern Ghana. Similarly, household size and technical efficiency were inversely related at 10% significance level. The implication is that households with fewer members are more technically efficient, possibly because, an increase in household size puts pressure on the scarce cash resources available to the farm household. Moreover, the larger the size of the family, the larger the dependency ratio, which could lead to this finding. The result agrees with Mango et al. (Citation2015), who found a negative association between the number of household members and technical efficiency of maize production in Zimbabwe.
Also, technical efficiency decreased with maize acreage at 1% significance level. This suggests that the scale of operations is crucial in production. Respondents with more extensive farmlands may be operating beyond their scope (decreasing returns to scale), which might lead to inefficient management of productive resources. Similar results have been observed by Etim and Udoh (Citation2014) and Tien and Thong (Citation2014) among crop farmers in Nigeria and Vietnam, respectively. However, the finding of Mango et al. (Citation2015) is contrary to this study.
Contrary to a priori expectation, contact with agricultural extension agents had a negative influence on TE at 10% level. As emphasized by many empirical studies (Abdulai et al., Citation2017; Danso-Abbeam et al., Citation2018; Evenson, Citation2001; Gautam, Citation2000), agricultural extension service is a critical avenue for disseminating information on production technologies, which is expected to enhance productivity. On average, farmers received only one (1) extension visit during the cropping season, which could account for the negative influence of extension services on farmers’ efficiency.
The results further indicate a positive influence of herd size on technical efficiency at 1% significance level. Hence, it is commendable to encourage smallholder farmers to keep livestock. The result agrees with a priori expectation because draught animals (especially cattle) are useful in smallholder farming systems where they are used in land preparation and carting of farm inputs, and provide manure for soil fertility improvement. Lastly, weeding frequency was negatively correlated with farmers’ efficiency. The general recommendation for maize producers is to weed their farms twice. Hence, farmers who carried out more than two weeding activities used more resources than required, reducing their level of efficiency.
5. Conclusion and recommendations
In this study, we have estimated the influence of IMV on the TE of sampled farms in the Tolon District, Ghana. It was observed from the probit analysis that the probability of adoption of IMV is shaped by the combined effects of age, educational attainment, extension contact, membership of farmer group, and perception of soil fertility status. The results from the DEA analysis showed a mean bias-corrected TE score of 57% under the VRS assumption. The result suggests that the output level could be increased by 43% without additional input requirements. The second-stage truncated regression estimates indicated that adoption of IMV improved the efficiency of the sampled farms in the study area. This suggests that adoption of IMV has been translated into improving the efficiency of maize production by the sampled farmers.
From a policy perspective, this study has two insightful findings that can serve as an empirical basis for the design and implementation of farm-level programmes. First, the fact that farmers’ observed output lag behind their potential suggests that improvement in technical and managerial skills among these farmers is critical to boost the productivity of maize farms. Second, farmers should be encouraged to plant improved varieties to enhance their output and productivity levels. This could be achieved by ensuring that farmers have access to subsidized fertilizer and improved maize seeds. Finally, farmers need training on effective weed management to reduce the frequency of weeding.
Abbreviations
CRS | = | Constant returns to scale |
DEA | = | Data envelopment analysis |
DMU | = | Decision-making unit |
FASDEP | = | Food and Agriculture Sector Development Policy |
FNS | = | Food and nutrition security |
GSGDA | = | Ghana Shared Growth and Development Agenda |
IMV | = | Improved maize variety |
MoFA | = | Ministry of Food and Agriculture |
SFA | = | Stochastic frontier analysis |
TE | = | Technical efficiency |
VRS | = | Variable returns to scale |
Acknowledgements
The authors are grateful to the farmers who participated in the study especially for their cooperation and willingness to provide information to the enumerators during the field survey.
Additional information
Funding
Notes on contributors
Benjamin Tetteh Anang
Benjamin Tetteh Anang holds a PhD in agricultural economics and is a senior lecturer at the Faculty of Agriculture, University for Development Studies in Ghana. His research focuses on efficiency and productivity analysis, access to financial services, and impact evaluation studies.
Hamdiyah Alhassan
Hamdiyah Alhassan (PhD) is an environmental and resource economist and a senior lecturer at the Department of Agricultural and Resource Economics, University for Development Studies in Ghana. She has published dozens of peer-reviewed academic papers with her research interest including efficiency and productivity analysis, environmental evaluation studies, climate change adaptation and mitigation and food security.
Gideon Danso-Abbeam
Gideon Danso-Abbeam (PhD) is an applied economist and a lecturer at the Faculty of Agribusiness and Applied Economics, University for Development Studies, Ghana. His research covers agrarian technology adoption, efficiency measurement, gender development, and impact evaluation of farm households. He has published many articles in international peer-reviewed journals.
References
- Abatania, N. L., Hailu, A., & Mugera, A. W. (2012). Analysis of farm household technical efficiency in Northern Ghana using bootstrap DEA (No. 423-2016-27040)
- Abdulai, S., Nkegbe, P. K., & Donkoh, S. A. (2017). Assessing economic efficiency of maize production in Northern Ghana. Ghana Journal of Development Studies, 14(1), 123–16. https://doi.org/10.4314/gjds.v14i1.7
- Abdulai, S., Zakariah, A., & Donkoh, S. A. (2018). Adoption of rice cultivation technologies and its effect on technical efficiency in Sagnarigu District of Ghana. Cogent Food and Agriculture, 4(1), 1424296. https://doi.org/10.1080/23311932.2018.1424296
- Abebe, G. K., Bijman, J., Pascucci, S., & Omta, O. (2013). Adoption of improved potato varieties in Ethiopia: The role of agricultural knowledge and innovation system and smallholder farmers’ quality assessment. Agricultural Systems, 122, 22–32. https://doi.org/10.1016/j.agsy.2013.07.008
- Ahmed, M. H. (2015). Adoption of multiple agricultural technologies in maize production of the Central Rift Valley of Ethiopia. Studies in Agricultural Economics, 117(3), 162–168. https://doi.org/10.7896/.2063-0476
- Ahmed, M. H., Geleta, K. M., Tazeze, A., & Andualem, E. (2017). The impact of improved maize varieties on farm productivity and wellbeing: Evidence from the East Hararghe Zone of Ethiopia. Development Studies Research, 4(1), 9–21. https://doi.org/10.1080/21665095.2017.1400393
- Anang, B. T. (2019). Are adopters of improved rice varieties more productive than non-adopters? Empirical evidence from northern Ghana. Ghana Journal of Development Studies, 16(1), 92–107. https://doi.org/10.4314/gjds.v16i1.5
- Anang, B. T., Bäckman, S., & Sipiläinen, T. (2016). Agricultural microcredit and technical efficiency: The case of smallholder rice farmers in Northern Ghana. Journal of Agriculture and Rural Development in the Tropics and Subtropics, 117(2), 189–202. http://nbn-resolving.de/urn:nbn:de:hebis:34-2016061350415
- Asante, B. O., Temoso, O., Addai, K. N., & Villano, R. A. (2019). Evaluating productivity gaps in maize production across different agroecological zones in Ghana. Agricultural Systems, 176, 102650. https://doi.org/10.1016/j.agsy.2019.102650
- Asfaw, S., Kassie, M., Simtowe, F., & Lipper, L. (2012). Poverty reduction effects of agricultural technology adoption: A micro- evidence from rural Tanzania. Journal of Development Studies, 48(9), 1288–1305. https://doi.org/10.1080/00220388.2012.671475
- Azumah, S. B., Donkoh, S. A., & Awuni, J. A. (2019). Correcting for sample selection in stochastic frontier analysis: Insights from rice farmers in Northern Ghana. Agricultural and Food Economics, 7(1), 9. https://doi.org/10.1186/s40100-019-0130-z
- Balcombe, K., Fraser, I., Latruffe, L., Rahman, M., & Smith, L. (2008). An application of the DEA double bootstrap to examine sources of efficiency in Bangladesh rice farming. Applied Economics, 40(15), 1919–1925. https://doi.org/10.1080/00036840600905282
- Benedetti, I., Branca, G., & Zucaro, R. (2019). Evaluating input use efficiency in agriculture through a stochastic frontier production: An application on a case study in Apulia (Italy). Journal of Cleaner Production, 236, 117609. https://doi.org/10.1016/j.jclepro.2019.117609
- Coelli, T. J., Rahman, S., & Thirtle, C. (2002). Technical, allocative, cost and scale efficiencies in Bangladesh rice production: A non-parametric approach. Journal of Agricultural Economics, 53(3), 607–626. https://doi.org/10.1111/j.1477-9552.2002.tb00040.x
- Collier, P., & Dercon, S. (2014). African agriculture in 50 years: Smallholders in a rapidly changing world? World Development, 63, 92–101. https://doi.org/10.1016/j.worlddev.2013.10.001
- Danso-Abbeam, G., Bosiako, J. A., Ehiakpor, D. S., & Mabe, F. N. (2017). Adoption of improved maize variety among farm households in the northern region of Ghana. Cogent Economics and Finance, 5(1), 1416896. https://doi.org/10.1080/23322039.2017.1416896
- Danso-Abbeam, G., Ehiakpor, D. S., & Aidoo, R. (2018). Agricultural extension and its effects on farm productivity and income: Insight from Northern Ghana. Agriculture and Food Security, 7(1), 1–10. https://doi.org/10.1186/s40066-018-0225-x
- Deepa, R., Bandyopadhyay, A. K., & Mandal, M. (2015). Factors related to adoption of maize production technology in Cooch Behar district of West Bengal. Agriculture, 5(2), 775–777.
- Donkoh, S. A. (2011). Technology adoption and efficiency in Ghanaian agriculture: A microeconomic study. USA LAMBERT Academic Publishing.
- Donkor, E., Owusu-Sekyere, E., Owusu, V., & Jordaan, H. (2016). Impact of row-planting adoption on productivity of rice farming in northern Ghana. Review of Agricultural and Applied Economics, 19(2), 19–28. https://doi.org/10.15414/raae.2016.19.02.19-28
- Etim, N. A. A., & Udoh, E. J. (2014). Identifying sources of efficiency among resource poor indigenous vegetable farmers in Uyo, Nigeria. International Journal of Food and Agricultural Economics, 2, 33–39.
- Evenson, R. (2001). Economic impacts of agricultural research and extension. In B. Gardner & G. Rausser (Eds.), Handbook of agricultural economics (Vol. 1, pp. 573–628). Elsevier Science.
- Farrell, M. J. (1957). The measurement of productive efficiency. Journal of the Royal Statistical Society. Series A (General), 120(3), 253–290. https://doi.org/10.2307/2343100
- Fraser, I., & Cordina, D. (1999). An application of data envelopment analysis to irrigated dairy farms in northern Victoria, Australia. Agricultural Systems, 59(3), 267–282. https://doi.org/10.1016/S0308-521X(99)00009-8
- Gautam, M. (2000). Agricultural extension: The Kenyan experience, an impact evaluation. The World Bank.
- Ghana Statistical Service. (2014a). Ghana Living Standards Survey Round 6 (GLSS6). GSS.
- Ghana Statistical Service. (2014b). GLSS 6 poverty profile in Ghana: 2005–2013. www.statsghana.gov.gh/docfiles/glss6/GLSS6_Poverty%20Profile%20in%20Ghana.pdf
- Ghana Statistical Service. (2014c). 2010 population and housing census (District analytical report). http://www2.statsghana.gov.gh/docfiles/2010_District_Report/Northern/TOLON.pdf
- Ghimire, R., Wen-chi, H., & Shrestha, R. B. (2015). Factors affecting adoption of improved rice varieties among rural farm households in Central Nepal. Rice Science, 22(1), 35–43. https://doi.org/10.1016/j.rsci.2015.05.006
- Islam, K. Z., Sumelius, J., & Backman, S. (2012). Do differences in technical efficiency explain the adoption rate of HYV rice: Evidence from Bangladesh. Agricultural Economics Review, 13(1), 93–104.
- Jayne, T. S., & Rashid, S. (2013). Input subsidy programs in sub-Saharan Africa: A synthesis of recent evidence. Agricultural Economics, 44(6), 547–562. https://doi.org/10.1111/agec.12073
- Karimov, A. (2013). Productive Efficiency of Potato and Melon Growing Farms in Uzbekistan: A Two Stage Double Bootstrap Data Envelopment Analysis. Agriculture, 3(3), 503–515. doi:10.3390/agriculture3030503
- Kassie, M., Jaleta, M., Shiferaw, B., Mmbando, F., & Mekuria, M. (2013). Adoption of interrelated sustainable agricultural practices in smallholder systems: Evidence from rural Tanzania. Technological Forecasting and Social Change, 80(3), 525–540. https://doi.org/10.1016/j.techfore.2012.08.007
- Kassie, M., Teklewold, H., Jaleta, M., Marenya, P., & Erenstein, O. (2015). Understanding the adoption portfolio of sustainable intensification practices in eastern and southern Africa. Land Use Policy, 42, 400–411. https://doi.org/10.1016/j.landusepol.2014.08.016
- Khonje, M., Manda, J., Alene, A. D., & Kassie, M. (2015). Analysis of adoption and impacts of improved maize varieties in Eastern Zambia. World Development, 66, 695–706. https://doi.org/10.1016/j.worlddev.2014.09.008
- Kijima, Y., & Sserunkuuma, D. (2013). The adoption of NERICA rice varieties at the initial stage of the diffusion process in Uganda. African Journal of Agricultural and Resource Economics, 8(1), 45–56.
- Konja, D. T., Mabe, F. N., & Alhassan, H. (2019). Technical and resource-use-efficiency among smallholder rice farmers in Northern Ghana. Cogent Food and Agriculture, 5, 1651473.
- Kumbhakar, S., Tsionas, E., & Sipiläinen, T. (2009). Joint estimation of technology choice and technical efficiency: An application to organic and conventional dairy farming. Journal of Productivity Analysis, 31(3), 151–161.
- Lee, D. R. (2005). Agricultural sustainability and technology adoption: Issues and policies for developing countries. American Journal of Agricultural Economics, 87(5), 1325–1334. https://doi.org/10.1111/j.1467-8276.2005.00826.x
- Mango, N., Makate, C., Hanyani-Mlambo, B., Siziba, S., & Lundy, M. (2015). A stochastic frontier analysis of technical efficiency in smallholder maize production in Zimbabwe: The post-fast-track land reform outlook. Cogent Economics and Finance, 3(1), 1117189. https://doi.org/10.1080/23322039.2015.1117189
- Mitra, S., & Yunus, M. (2018). Determinants of tomato farmers’ efficiency in the Mymensingh district of Bangladesh. Data envelopment analysis approach. Journal of Bangladesh Agricultural University, 16(1), 93–97. https://doi.org/10.3329/jbau.v16i1.36487
- MoFA (Ministry of Food and Agriculture). (2016, October). Agriculture in Ghana. Facts and Figures 2015. Statistics, Research and Information Directorate (SRID); Accra.
- MoFA (Ministry of Food and Agriculture). (2017). Planting for food and jobs, strategic plan for implementation (2017-2020). Republic of Ghana.
- Nkegbe, P. K. (2018). Credit access and technical efficiency of smallholder farmers in Northern Ghana: Double bootstrap DEA approach. Agricultural Finance Review, 78(5), 626–639. https://doi.org/10.1108/AFR-03-2018-0018
- Obayelu, A. E., Moncho, C. M. D., & Diai, C. C. (2016). Technical efficiency of production of quality protein maize between adopters and non-adopters, and the determinants in Oyo State, Nigeria. Review of Agricultural and Applied Economics, 19(2), 29–38. https://doi.org/10.15414/raae/2016.19.02.29-38
- Owusu, V. (2016). Technical efficiency of technology adoption by maize farmers in three agro-ecological zones of Ghana”. Review of Agricultural and Applied Economics, 19(2), 39–50. https://doi.org/10.15414/raae/2016.19.02.39-50
- Rahman, S. M., & Norton, G. W. (2019). Farm-level impacts of eggplant integrated pest management: A stochastic frontier production function approach. International Journal of Vegetable Science, 25(6), 590–600. https://doi.org/10.1080/19315260.2019.1566188
- Reardon, T., Barrett, C. B., Berdegué, J. A., & Swinnen, J. F. (2009). Agrifood industry transformation and small farmers in developing countries. World Development, 37(11), 1717–1727. https://doi.org/10.1016/j.worlddev.2008.08.023
- Sheahan, M., & Barrett, C. B. (2017). Ten striking facts about agricultural input use in Sub-Saharan Africa. Food Policy, 67, 12–25. https://doi.org/10.1016/j.foodpol.2016.09.010
- Simar, L., & Wilson, P. W. (2007). Estimation and inference in two-stage, semi-parametric models of production processes. Journal of Econometrics, 136(1), 31–64. https://doi.org/10.1016/j.jeconom.2005.07.009
- Singh, R. B., Kumar, P., & Woodhead, T. (2002). Smallholder farmers in India: Food security and agricultural policy (RAP Publication number 2002/03).
- Tien, N. V., & Thong, P. L. (2014). Analyzing economic efficiency of the lotus farms in Dong Thap Province (in Vietnamese). Scientific Journal of Can Tho University, 30, 120–128.
- Wongnaa, C. A., & Awunyo-Vitor, D. (2018). Achieving sustainable development goals on no poverty and zero hunger: Does technical efficiency of Ghana’s maize farmers matter? Agriculture and Food Security, 7(1), 1. https://doi.org/10.1186/s40066-018-0223-z
- Wooldridge, J. M. (2010). Econometric analysis of cross section and panel data (2nd ed.). MIT Press.
- Wouterse, F. (2010). Migration and technical efficiency in cereal production: Evidence from Burkina Faso. Agricultural Economics, 41(5), 385–395. https://doi.org/10.1111/j.1574-0862.2010.00452.x