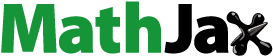
Abstract
Technological change in agriculture in land-scarce developing countries is required for land use intensification to feed the growing population. The purpose of this study is to analyze factors affecting adoption decision of improved wheat variety and its effect on households’ welfare in a semi-arid economy of Tigrai, northern Ethiopia. First, a correlated random effect double hurdle model with a control function approach is used to analyze determinants of improved wheat adoption decision and extent of adoption. Second, fixed effect instrumental variable method is used to assess the impact of area under improved wheat cultivation on households’ welfare. Double hurdle model results indicate that access to improved wheat, landholding, male adult and access to information have strong and positive effect on adoption decision and extent of adoption of improved wheat. The impact analysis shows that extent of improved wheat adoption has strong and positive effect on households’ income, consumption, and food security. Moreover, the gender and wealth disaggregation analysis show that extent of improved wheat adoption has a positive and significant effect on welfare of male and female-headed, land poor and land rich households. Thus, adoption of improved wheat is a pro-poor strategy and increasing access to the technology appears a promising pathway for rural development in a land scarce and semi-arid economy like Ethiopia.
Keywords:
Public interest statement
Economic growth and poverty reduction are entirely manifested by the improvement of societal welfare and this depends on increasing in the productivity of available resources i.e., land, labor,and capital. In areas of land scarcity and high population pressure, increasing agricultural production through expanding cultivable land size (extensification) is not viable strategy, in steady using more inputs and technologies per unit of land (intensification) becomes notable option. This approach is effectively implemented by several Asian countries in 1970s and was dubbed the “green revolution”, which ensures higher agricultural production and market. This study suggests that promoting access to improved wheat variety inspired smallholders to adopt the improved technology and certainly increased farm level productivity and food security at household level in a semi -arid and land scarce developing country. This is also a strategic choice for the Ethiopian government, where thorough economic reforms and promoting extension programs are quite necessitating.
1. Introduction
The continuous increase in population pressure in developing countries is associated with declining per capita cultivable land and it becomes a great challenge to agricultural research and development (Matuschke et al., Citation2007). Almost half of the population in Sub-Saharan Africa (SSA) lived in abject poverty and its growth rate is double to that of the global average (AFDB, Citation2012). Two third of Africa’s poor reside in rural areas where agriculture is the primary means of livelihood, but the sector unable to ensure self-reliance in food security at national and household level (IBID).In Africa, although areas under crop cultivation increase, crop productivity has not grown in the desirable manner (AFDB,Citation2012). Similar feature is also observed in Mexico that in 38 years from 1962 to 2000, wheat area cultivated increased by about 80%, while wheat yield increased only by 25 % (Lobell et al., Citation2005). The low yield rate compared to the area under wheat crop in Mexico is the result of traditional agricultural practices, mainly using local wheat varieties.
This study builds on farmers’ behavior of technology adoption in risk and land-scarce economy that smallholders are “poor but efficient” (Schultz, Citation1964). Adoption of agricultural technology is a dynamic process and this could be true when smallholders operate in a decent environment and a sufficient amount of time is given to learn about the new technology. This infers that for the first time, when the new agricultural technology is introduced, some farmers adopt it instantly, some farmers required considerable time and promotional effort until they have adopted, and some farmers become reluctant to learn and to use the technology. Farmers in developing countries face resource constraints and imperfect input and output markets, improving the efficiency of the existing production system through increasing cultivable land size cannot be a cost-effective strategy. This is especially relevant in Sub-Saharan African (SSA) where food security is acute and new agricultural investment is severely conditioned by liquidity constraint. The hypothesis of this study states that adoption of improved agricultural technology (wheat in this case) enhanced land use intensification and positively contributes to welfare of smallholders in a semi-arid economy of Ethiopia.
There have been quite different types of adoption studies on improved agricultural technologies and the subsequent welfare impacts in Ethiopia and elsewhere. For instance, the study of Shiferaw et al. (Citation2014); Workineh et al. (Citation2020); Tesfaye et al. (Citation2016); Aklilu et al. (Citation2022); Abbas & Chandio, (Citation2018) assess adoption determinants and welfare impact of improved wheat varieties in Ethiopia and in Pakistan. Despite these empirical evidence on the subject matter, there are some conceptual and methodological gaps in the available studies. The study of Tsegaye and Bekelle (Citation2012); Tesfaye et al. (Citation2016); Aklilu et al. (Citation2022) use cross-sectional data and assessed adoption determinants and impact of improved wheat adoption on farmers’ welfare in central and southern Ethiopia. They found adopters have cultivated part of their land with improved wheat and gained higher households’ income, insured food security and consumption expenditure compared to non-adopters. However, these studies face some short comings. First, their study focuses on the agricultural potential areas of Ethiopia where food insecurity is not serious issue and their study area lacks universality to represent areas with low agricultural potentials and acute food insecurity. Second, technology adoption is dynamic process and farmers’ adoption behavior varies over time and imperative to update the information based on the current technologies being adopted by other farmers. Thus, the use of cross-sectional data flops to provide insights into how farmers learn and spread the improved technologies over time among smallholders.
The study of Aklilu et al., Citation2022) exhaustively reviewed adoption process of improved wheat by Ethiopian farmers. Their finding revealed that access to credit, farmers’ perception of technology adoption and organizational membership show significant and positive effect on adoption of improved wheat variety. But, this study has suffered from estimation and identification strategy issues. First, control variables like access to credit and organizational membership are potential endogenous regressors and dearth of strict exogeneity and create biased estimates. Second, farmers’ perception on adoption of improved wheat variety is typically subjective behavior. This implies that famers’ attitude is attributed to unobservable individual heterogeneity and varies with the level of literacy, social interaction, proxy to information, access to service, and risk taking. Uncontrolled to the unobservable individual heterogeneity is potentially suspected to create variation in farmers’ technology adoption decisions and leads to biased estimates.
Tesfaye et al. (Citation2016) on the other hand, using Propensity Score Matching (PSM), analyze the impact of improved wheat adoption on farm level productivity and income of Ethiopian farmers. Results depicted that improved wheat adopters gain better farm level productivity and higher households’ income compared to their counterpart non-adopters. While this study faces methodological pitfall. Intuitively, propensity score matching is suffering from the issue of self-selection bias and lacks to make robust impact results. Of course, Average Treatment Effect (ATE) is considered as a remedy for the drawback of PSM, assuming that farm level productivity and household income of adopters can reasonably be approximated to farm level productivity and household income of non-adopters before the survey period. Whereas, ATE is not vigorous remedy, as ATE using PSM procedure is not possible, instead technology adoption usually influenced by farmers’ self-selection or sometimes through program placement. In addition, their study uses variables like participation in off-farm activities and access to institutional credit services as a control variable in the impact estimation model. Still, these variables are potential endogenous regressors and naturally unlikely to bring strong impact difference among the adopter groups. The study skips to take any remedy to fix such estimation challenges and considered as its key defect. The current study analyzes the determinants and impact of improved wheat adoption on households’ welfare in developing countries through stepping up the above mentioned empirical limitations.
This paper explores the recent efforts in prompting land use intensification strategy in a semi-arid and land scarce economy, with the goal of informing important policy questions in Ethiopia. (a) What factors influence adoption decisions (demand side) and access (supply side) to the improved wheat variety? (b)What has been the impact of adoption of improved wheat variety on households’ welfare measured by log of per capita income, per capita consumption expenditure, per capita off farm income and probability of food secured? (c) To what extent adoption of improved wheat variety contributes to welfare difference across male and female headed households? (d) Did adoption of improved wheat variety affect households’ welfare depending on initial wealth status?
To answer these questions, the paper uses three rounds of household survey panel data (2005/06, 2009/10, and 2014/15) production seasons and meticulous estimation methods via addressing some potential endogeneity and individual unobservable heterogeneity concerns. First, adoption decision of improved wheat variety is estimated using a Correlated Random Effect Double Hurdle(CRE-DH) model with a control function approach to fix the endogeneity issue associated with access to improved wheat variety (Cameron & Trivedi, Citation2010). Second, the impact of improved wheat adoption on households’ welfare is estimated using household Fixed Effect Instrumental Variable (FE-IV) model to fix for potential correlation between covariates and time in-variant unobserved heterogeneity (Wooldridge, Citation2010).
The contribution of this study is three fold: First, it provides new insight into the development efforts on the adoption of improved agricultural technologies (improved wheat) in a land scarce, semi-arid, and food insecurity is widely applicable in Tigrai, Ethiopia. Second, the current study provides new intuition into factors affecting the likelihood of adoption and intensity of adoption for improved wheat variety through the learning process that have not been long recognized in developing countries. Third, the paper discusses policy implications for how to integrate and reap the benefits from the promotion of improved wheat adoption, given its importance for farm level productivity, food security, employment, and market participation in a populated economy. To the knowledge of the researcher, this is the first study in its kind on adoption of improved wheat technology and its welfare impact using long and rich farm household panel data in Africa, particularly in Ethiopia.
The paper is organized as follows. Section 2 deals with wheat production, the development of improved wheat adoption and its economic importance in Ethiopia. Section 3 presents review of the related empirical literature. Description of study area, study design and data are presented in section 4. Estimation methods and strategy issues are discussed in section 5. Results and discussions are presented in section 6; and finally brief concluding draws out implications for technology adoption and its welfare impact in semi-arid environments in section 7.
2. Background: Wheat production and its importance in Ethiopia
Wheat is one of the important cereal crops grown in the mid and high land of Ethiopia and consumed in cultural and processed techniques (Aklilu et al., Citation2022). Ethiopia is the second wheat producer in SSA next to South Africa and wheat ranks the fourth crop in terms of total area cultivation and production after teff, maize and sorghum (Kotu et al., Citation2000). The wheat sub sector takes high attention and strategic choice for the Ethiopian government that enhances to achieve the first target of Millennium Development Goal(MDG-1) that aims reducing the proportion of people lived less than one dollar a day by half in 2015 (Maya et al., Citation2013). One approach of achieving this goal is through promoting the application of improved agricultural technologies among the smallholders. The total area under wheat cultivation in Ethiopia accounts 1.6 million hectares and produced 3.9 million tons in the 2013/ 2014 production season (see Kelemu, Citation2017 for the details). Using the 2012/13 production season survey data, Kelemu (Citation2017) found that domestically produced wheat is used for food consumption (58%), for market and seed preserved for the next production season (19%), and 23% for industrial purpose. The Ethiopian government has promoted rural extension services to achieve food security at household level. As a result, wheat production share has reached in Oromia (57.4) %; in Amhara (27%); in South Nations and Nationals and People (8.7%); and in Tigrai (6.2%) from the respective region’s cultivated land size (Aklilu et al., Citation2022).
Despite the potential and its importance in Ethiopia, the country still remains the major wheat importer. According to the Ethiopian Grain Trade Enterprise, in 2016 the country has imported about 1.05 million tons of wheat, of which 750 thousand tons is imported from Russia and Argentina and the remaining 300 thousand tons of wheat is from external food aid (Aklilu et al., Citation2022). This indicates that yet, the self-food sufficiency of the country remains a big concern and much effort is considerably demanding.
Institutions such as agribusiness sectors, smallholder farmers and producer cooperatives are actively participating in cultivating improved wheat in mid and high lands of Ethiopia (Tesfaye et al., Citation2016). High yield is one attribute for adoption of improved wheat variety and significantly contributes to Ethiopia economy (Aklilu et al., Citation2022; Kelemu, Citation2017). For instance, (i) Due to high population pressure, per capita landholding in mid and high land of the country is declined over time, and the application of improved wheat variety enhanced land use intensification and gained better farm level productivity. (ii) Higher and more stable improved wheat variety has great impact on household food security, consumption, and nutrition. (iii) Higher wheat production improves farmers’ market participation through selling improved quality and quantity of wheat product and generating better market surplus. (iv) Due to better precipitation in mid and highland agro ecologies, demand for inorganic fertilizer is relatively high. Thus, adoption of improved wheat variety in the mid and highland agro ecologies creates a crowding-in effect to use inorganic fertilizer and created further improvement in farm level productivity.
Another benefit of improved wheat plantation in the high land of Ethiopia is its rust resistance. About 68% of the study region is a semi-arid high land agro ecology and local wheat is significantly affected by crop diseases “leaf rust” (Puccinia striiformis) and “stem rust” (P. graminis; Kotu et al., Citation2000). This reduces not only productivity but also the quality of the wheat crop. Cognizant to its importance in a semi-arid region, growing improved wheat variety maximizes yield and maintains crop quality. Farm households also demand improved wheat variety not only from its high crop yield but also it generates significant amount of by-product (residuals) for animal fodder (Aklilu et al., Citation2022).
3. Review of empirical literature: technology adoption and household welfare
Economic growth and poverty reduction is commonly manifested by the advancement of societal welfare. Literally, economic growth and poverty reduction depend on increasing in the productivity of available resources (land, labor and capital). In areas of land scarcity and high population pressure, increasing agricultural production through increasing cultivable land size (extensification) is not viable strategy, pretty using more inputs and technologies per unit of land (intensification) becomes better option (G. M Singh et al., Citation2022; Tsegaye & Bekelle, Citation2012; Xiaomin et al., Citation2022).. This option is effectively implemented by several Asian countries in 1970s and was dubbed the “green revolution” (Nick, Citation2021).
There have been extensive works on adoption of improved agricultural technologies and benefits derived from adoption in developing countries. This section reviews the relevant empirical studies. The study of Tesfaye et al. (Citation2016) using Propensity Score Matching (PSM) assessed adoption decision of improved wheat technology and its impact on farm level productivity and households’ income in Arisi zone of Ethiopia. Their finding reveals that sex of headship and live stock ownership influence positively and significantly intake decision of improved wheat variety, while human literacy proxy by educational level favors dis adoption of the technology. Moreover, adopters of the technology have captured higher farm level productivity and household income, on average compared to non-adopters. Literally, the study argues that PSM enables to match the adopters with non-adopters with similar propensity score values as a proxy to a randomized experiment and reducing self selection bias. More specifically, they assumed homogenous behavior of sampled households prior to the survey period in terms of technology adoption and benefit packages drawn from adoption decisions. However, the study faces some methodological pitfall. First, technology adoption is a dynamic process and farmers’ adoption behavior expected to be inconsistent. This implies that before the survey period, farmers could be dis adopters, while they could adopt the technology during the survey period, and vise vis. Thus, PSM does not give a warranty that before the survey period, all farmers practice the same adoption behavior on the new technology. Second, their study disregards to make a clear differential welfare effect of improved wheat adoption between male and female headed households and hard to interpret the result. Likewise, headship literacy status is negatively correlated with adoption of improved wheat in the study site. This finding is inconsistent to most similar empirical works in developing countries (Abbas & Chandio, Citation2018; Aklilu et al., Citation2022; Christine & Christopher, Citation2006; Makaiko et al., Citation2015; Simone et al., Citation2017). Moreover, the study does not justify why such like correlation is prevailed.
Using cross sectional data and binary discrete choice model, the studies of Tsegaye and Bekelle (Citation2012); Makaiko et al. (Citation2015) analyze adoption decision and impact of improved wheat and improved maize varieties on household welfare in Ethiopia and Zambia, respectively. Consistently, both studies found that adoption of the improved farm technologies significantly increases farmers’ food consumption, crop income and food security. In the meantime, these studies remark similar methodological defects. They used static model that has adopted the technology at a particular point in time and adoption process is yet not completed and create biased estimates. This is due to that fact static model ignores the dynamic effect and the inability to control the unobserved household heterogeneity effect. In addition, static model does not account changes over time for the farmers’ ability to improve their technical efficiency in growing and marketing the crop.
Simone et al. (Citation2017) used three rounds of panel data and analyzed the impact of improved Chickpea adoption on smallholders’ income and poverty reduction in Ethiopia. The study has demonstrated the conceptual and methodological issues in a clear manner. Specifically, the study addressed the importance of access to the improved technology (supply side) on the adoption decision of the technology (demand side) and take appropriate remedies to fix the problem of self selection bias in adoption decision. Moreover, endogeneity issues in the causal effect of improved Chickpea technology on households’ income and poverty reduction is handled using an instrumental variable analysis. The finding reveals that adoption of improved technology significantly increases households’ income and poverty reduction at household level. Despite the rigorous finding and importance of the study in a land scarce developing country, the study pledges an identification strategy issue. First, off farm income is potentially endogenous variable and using it as a regressor creates biased adoption and impact results. Second, in a panel data set, endowment variables like households’ assets adjust themselves over time and inhibited strict exogeneity in the adoption and impact specifications, so that robustness of estimated result is highly mistrusted .
The review of the related literature for this study disclosed that there are no comprehensive studies that have been systematically examined in terms of methodology, theory, data, and relevance on adoption and welfare impact of improved agricultural technologies in a changing environment .To fill this knowledge gap, this study attempts to assess empirically adoption of improved wheat technology and its causal effect on the wide range of households’ welfare variables using long and rich observational data in land scarce and semi-arid economy of Ethiopia.
4. Study area, data, and descriptive statistics
4.1. Description of the study area
The study area is Tigrai regional state located in the northern part of Ethiopia where mixed agriculture (farming and livestock production) is the main livelihood of the rural society. The major products growing in the region are cereals, and wheat is one of the most commonly planted crop. Though the region is semi-arid and agriculture is operated in rain-fed and a traditional system, it contributes 38.7% of the Regional Gross Domestic Product (RGDP) (BoFED Tigray, Citation2014). Livestock production is one means of livelihood in the rural society and used as an input for farming activities, households’ food stuff, and market. Population growth rate of the study region reaches 2.5 % per year and an average population density is 327 persons/km2 which is slightly higher than the national average (BPF, Citation2014). The rainy season of the region is mid of June to mid of September (Tigray, Citation2014). Due to weather variability and changing environment, the rain-fed agriculture is severely constrained to meet the food requirement of the region’s population.
Arable land of the study region accounts 1.03 million hectare where 83 % of this area was covered with cereals in the 2013/14 production season(Tigray, Citation2014). About 68% of the region’s agro ecology is mid and high lands where wheat crop is dominantly growing (Doss et al., Citation2003; Kotu et al., Citation2000). Improved wheat variety is distributed by cooperatives and bureau of regional agriculture. Limited access and higher technology price, imperfect input and out market, imperfect information and pervasive transaction costs appeared to have major constraints of improved wheat adoption in the study sites. The study covers five-zones, which is 80% of the region’s area namely, Southern, South eastern, Eastern, Central, and North western.
4.2. Data
The data used in this paper come from a balanced panel of 274 farm households surveyed in 2005/06, 2009/10, and 2014/15 production seasons in rural Tigrai, northern Ethiopia. All households in the sample set are rural households and farming is the main economic activity. Based on the baseline survey design in 1998/99 as described in Hagos and Holden (Citation2003), two-stage stratified random sampling technique was applied. In the first stage, communities were stratified based on variations in agricultural potential, access to irrigation and market, population density and agro ecology variation. In the second stage, enumeration areas (Kebelles) were randomly sampled within each stratum, and a random sample of households were selected for a detailed interview. The data collection process is managed by the collaboration between Norwegian University of Life Sciences, Norway and Mekelle University, Ethiopia.
The survey incorporated detailed questions on household compositions and characteristics, farm characteristics, market, access to services, endowments, and technology adoption variables. The household composition and characteristics captured information like as household head’s gender, age, and the number of active labor force, and headship literacy status. The gender distribution of households’ head helps to control on most farming decisions such as technology adoption, farm investment and market participation. The human productive element of the household head (young, male and literate) labor force presents the ability to undertake the laborious task in farming activities, apply their effort to exploit the benefits drawn from technology adoption and market opportunities.
The survey also captured welfare data in a standard measurement like per capita crop income, per capita total income, per capita consumption expenditure, per capita off-farm income, and the probability of food secured households. The survey also captured information related to by-product income (residuals used as a fodder for animals). This welfare variable is expected to influence smallholders to make technology adoption decision. The household survey was supplemented by village level, farm level and access to service variables. Some of these variables are: farm size owned and cultivated, farm distance, land quality, distance to market and distance to all-weather roads, access to information, access to irrigation, access to transport services, agro-ecological zones (proxy to rainfall and rainfall variability), output prices, input prices, administrative locations, and year dummy. The land and non-land resource endowments backed up farmers’ adoption decision in an imperfect input and out markets. Irrigation program reduces risk of technology adopters as they suspect that the new technology may not feasible in a semi-arid agriculture with erratic weather condition. Access to information empowers farmer’s awareness about the attributes of the new technology and motivated to adopt. Administrative locations help to capture spatial heterogeneity among the sampled communities. The output price and input price are computed using community-level median values. These values help to fix the tricky associated with the outlier values of average prices when computing monetary values of agricultural products and inputs. The rainfall intensity and rainfall variability data are captured at community level from daily satellite record of the African Rainfall Climatology Version 2 (ARC2) precipitation estimates.
4.3. Descriptive statistics
The mean and distribution of the outcome variables (adoption and intensity of wheat varieties) are presented in Table . Adoption rate is measured in terms of the average households planting improved wheat variety over the survey periods. Although access to the improved wheat variety increases over the survey periods (Table ), adoption rate of the technology slightly decreased from 13.8 % in 2005/06 to 13.1% in 2009/10, but significantly increased to 17.1 % in 2014/15 production season. Intuitively, adoption is a dynamic process and some farmers adopt in the first survey period while dis adopt in the next period and vice versa. Another argument for lower rate of the technology adoption decision could be from farmers’ low sympathetic on the attributes of the improved wheat variety. For instance, in the first planting period, farmers plant the improved wheat variety through purchasing from the seed distributors or from open market and transport directly to the farm gates. After harvesting (four to five months’ post plantation), a small portion of the improved wheat product is preserved for next year cultivation period. In the next planting period, framers use the genetically improved wheat harvested in the previous production period by transporting from home to the farm get. But they do not consider the variety as improved and reported as local variety. Adoption rate of any type of wheat variety shows a linear increment over the survey periods and on average, 46 % of farm households used local variety wheat, indicates the dominance of local wheat in the study region.
Table 1. Access and adoption rate of improved wheat variety (mean value)
Average landholding size of farmer household in the study region is about one hectare (Table ) which is similar to the country average (Gebremedhin et al., Citation2009). Table also disclosed that on average, area used for any type of wheat variety increases from 0.47 hectare in 2005/06 to 0.55 hectare in 2009/10 and this has been significantly increased to 1.0 hectare in 2014/15 cropping period. Area under improved wheat variety increases from 0.36 hectare in 2005/06 to 0.47 hectare in 2009/10 and this has been further increased to 0.55 hectare in 2014/15 cropping season. The intuition is that though adoption rate of the improved wheat technology fluctuates across the survey periods (Table ), intensity of adoption shows a linear increment. This might be due to the allocation of substantial farm size for the improved technology by the already adopters.
Table 2. Landholding size and area under improved wheat (mean value)
4.4. Who plants improved wheat in Tigrai?
Table presents the t-test and chi-square comparison of the mean key household-level characteristics of improved wheat adopters and non-adopters. Results show that improved wheat adopter households are distinguished from non-adopters in terms of households’ endowment such as farm size, number of farms owned, and family labor. Households with more family size are more likely to adopt the improved wheat variety compared to households with small family size, and the difference is statistically significant at the 5% in 2005/06 and at the 1% in 2009/10 and 2014/15. This suggests that adoption of improved agricultural technology has food security implication, and larger family households are expected to deploy more labor to cultivate the improved wheat variety compared to households with few family members. Due to credit market imperfection, resource endowments in developing countries make a differential effect on technology adoption decision among smallholders. Table shows a positive and monotonic relationship between improved wheat adoption and size of landholding and number of farms owned. This shows that wealthier farmers are more likely to adopt the improved wheat variety compared to poor farmers at least in two of the three survey periods, and the difference is statistically significant at the 5 % level. Similar findings were reported in Ethiopia (Tsegaye & Bekelle, Citation2012; Workineh et al., Citation2020); in Pakistan (Abbas & Chandio, Citation2018).
Table 3. Socio-economic characteristics of adopters and non adopters(mean value)
Farmers accessed to irrigation program are more likely to adopt the improved wheat variety in 2014/15 cropping season compared to their counterpart non-irrigated households and the difference is statistically significant at the 1 % level. The intuition is that irrigated farmers are interested to adopt precipitation friendly and yield enhancing agricultural technology like inorganic fertilizer, in turn positively influence adoption of improved wheat variety.
Table presents the comparison of the mean value of welfare indicators among the improved wheat adopter categories. The data show that adopter households are benefited more from improved wheat plantation compared to non-adopter in all of the survey periods. As a result, improved wheat adopters captured higher total crop income, per capita consumption expenditure, and wheat income compared to their counterpart non-adopters and the difference is statistically significant at the 1% level. This indicates that in land scarce and populated economy, land use intensification through applying improved agricultural technologies becomes an essential option of welfare maximization and poverty reduction at household level. There is insignificant difference in total income among the adopter categories, although adopters earn more total income than non-adopters. This may be due to income variations generated from other off-farm activities for non-adopter households.
Table 4. Welfare measures by adopter and non adopter of improved wheat (mean value)
5. Empirical methods
The paper focuses on two points: (1) analyze adoption and adoption intensity of improved wheat variety in semi-arid of Tigrai; and (2) analyze the welfare impact of improved wheat adoption. There are some challenging issues in estimating the models of improved wheat adoption and welfare effects. This is particularly how the unobserved individual heterogeneity effect and potential endogeneity of some control variables are addressed.
5.1. Estimation models
In the absence of price clearing mechanism, and input and output markets imperfections, smallholder farmers in developing countries make non-separable decisions between consumption and production functions (Singh et al., Citation1986). For instance, lack of or missing credit market leads to evidently raising some input costs by the shadow price of the credit service (Sadoulet & De Janvry, Citation1995). Subsequently, smallholders’ technology adoption decision depends on a vector of endowments. For a given level of resource constraints, adoption model distinguishes among farmers and expressed in an extended form as follow:
Selection of covariates in Equationequation (1)(1)
(1) is based on adoption theory (Feder et al., Citation1985) and their functional relation is expressed as follows. Q*/ Q refers to likelihood of adoption and adoption intensity of improved wheat, respectively. The intensity of adoption is expressed in terms of area under improved wheat growing measured in hectare. As discussed in Table , higher percentage of smallholders in the study area did not adopt the improved wheat, implying that adoption intensity of the technology is censored at observation with zero values. Thus, a corner solution framework model is an appropriate option for formulating the intensity of improved wheat specification. P refers to a vector of input and output prices. Higher technology price expects to discourage farmers’ adoption decision. The market value of the welfare variables in the impact estimation models gives only the nominal value (current market value). As the study deploys long panel data, computing the real (inflation-adjusted) value over the three survey periods become profoundly important. To do this, first, the market value of welfare variables captured in each survey period is deflated by the 2009/10 price, considering this year as a base year Consumer Price Index (CPI). This is because the year 2009/10 prevails stable average market price of agricultural products. Second, the real market value of the welfare variables in each survey period is converted to USD using the respective year’s exchange rate. H refers to household demographic variables like family size and household head’s characteristics expressed in terms of age, gender, and literacy status. The human capital element proxy by young and literate heads is expected to influence positively the probability of adoption and the area cultivated under improved wheat compared to aged and illiterate heads. The intuition is that literate and young headed households are expected to be efficient, avail themselves to updated technology and market information, and captured higher benefit packages from adoption compared to illiterate and elder headed households. The variable L refers to the households’ resource endowment expressed in terms of livestock ownership, own farm size and other wealth indicators. Wealthier farmers are more likely to adopt the improved wheat technology compared to the poor farmers. The implication is that in the imperfect input and output markets, wealthier households can fix the liquidity constraints and potentially motivated to adopt the improved technology compared to their counterpart poor households.
A refers to access to the improved wheat technology in the study sites. This is a dummy variable with the value of one if farmers are accessible to the technology and, zero otherwise. It is anticipated that farmers accessed to the technology are more likely to adopt the technology and dedicate to plant relatively large farm size under improved wheat. The vector V included in the model is to account for: (1) farm characteristics such as soil quality expected to affect the rightness of improved wheat seed for the farm and area used to grow the technology; and (2) climate conditions, proxy by rainfall and rainfall variation during the cropping seasons. Y refers to the year dummy that explains the variation of adoption and degree of adoption of the technology over the survey periods, compared to the base year (2005/06). C is the unobservable heterogeneity effect that is time-invariant variable and expected to affect technology adoption decision. ϵ is the unobservable effect of the model assuming zero mean and constant variance and cov(Xit,ϵt= 0). α is a vector of parameters to be estimated “i” and “t” are individual and time identifiers, respectively.
EquationEquation (1)(1)
(1) can be estimated using the tobit model framework, as tobit is popular for two-stage estimation (i.e., the probability and intensity of adoption) assuming ϵit is normally distributed, i.e., ϵit|Xit,~ Normal (0,δ2; (Tobin, Citation1958). However, in a panel data set, tobit model has two potential limitations. First, due to supply side constraints, the technology is not randomly distributed among farm households. This implies that the probability of a positive value (y > 0) and the actual value (y = 0) are not jointly estimated. Second, tobit model deals with restricted estimation approach and a given variable explains equally the probability and intensity of adoption decisions. An alternative to tobit model, a corner-solution of dependent variable, Double Hurdle (DH) model is applied (Burke, Citation2009). Double Hurdle model has a flexible feature compared to tobit and enables to estimate adoption and intensity of adoption in a separate equation. The first equation of the double hurdle model deals with factors affecting technology adoption decision (probit model), and the second equation of the double huddle model deals with factors affecting adoption intensity of the technology via truncated normal regression.
Next, the welfare impact of improved wheat adoption is analyzed. The outcome variables include the value of wheat crop production, consumption expenditure, off-farm income, total household income and the probability of food secured. Food security is defined as adequate and certain to get nutrition and safe food or capable to acquire satisfactory food in a socially acceptable way (Titus & Adetokunbo, Citation2007). Households are grouped into food secured and food insecured using the Food Security Index (FSI). Food security index is computed by dividing the per capita food consumption expenditure by two-third of the average per capita food consumption expenditure of all households. A household with per capita food expenditure falls below two-third of the mean per capita food expenditure is characterized as food in secured. On the other hand, a household with per capita food expenditure falls above the two-third of mean per capita food expenditure is denoted as food secured (Titus & Adetokunbo, Citation2007). Dummy variable is constructed with value of one for the food secured households, zero otherwise. The welfare variables area specified by W and modeled as follows:
Where W’ is a vector of welfare variables as described above. The welfare model specification in Equationequation (2)(2)
(2) is estimated when Q is treated as the extent of area under the improved wheat cultivation. L refers to households’ endowment. H includes household head and household characteristics. The other variables specified in Equationequation (2)
(2)
(2) are many of the same variables in Equationequation (1)
(1)
(1) . EquationEquation (2)
(2)
(2) is estimate using fixed effect estimator.
5.2. Estimation strategies
5.2.1. Controlling for unobserved heterogeneity (Ci)
When estimating a panel data model, the issue of unobservable heterogeneity effect should be addressed. This is to mean that adoption decision of improved wheat variety varies across farmers even if access to the improved wheat variety is random. For instance, a farmer with better farming skill, management ability, risk taker, access to market and information can adopt the technology and capture better benefits compared to a farmer with low farming skill, little managerial ability, risk averse, and limited market and technology information. This is observed to the individual farmer but not observed by the researcher. The intuition is that the unobservable heterogeneity effect may be correlated with other control variables and possibly leads to bias the adoption and impact estimations, that is, cov(Ci, it ≠ 0), in Equationequation (1)
(1)
(1) and in Equationequation (2)
(2)
(2) above.
i represents the vector of control variables.
If the time-invariant unobservable heterogeneity is not correlated with any of the other covariates, cov(Ci, = 0), the unobservable heterogeneity effect is part of the random error term and the adoption and welfare models are estimated as a random effect model. But, the randomness of the unobservable heterogeneity effect cannot have guaranteed on its orthogonality and uncorrelated with the other covariates in the adoption and welfare estimation. On the other hand, the Fixed Effect (FE) model is expected to fix the unobservable heterogeneity effect through the demeaning process for the linear welfare models Equationequation (2)
(2)
(2) , while FE is in-appropriate for nonlinear adoption models (Equationequation 1
(1)
(1) ) due to the incidental parameter problem (Wooldridge, Citation2010). For non-linear panel data models, the Correlated Random Effect (CRE) that is the work of Mundlak (Citation1978) and Chamberlain (Citation1982) is applied. This model relaxes the strict exogeneity assumption by allowing the relation between Ci, and X’it and removes the unobservable individual heterogeneity effect from the technology adoption model. In the CRE specification, the unobservable individual heterogeneity effect is expressed as a function of the mean value of all time-varying control variables.
When is the mean value of the explanatory variables which is the same for individual households in the panel data set, while differs across households (cross-sectional unit) and (Wooldridge, Citation2010). Plug Equationequation (3)
(3)
(3) into adoption Equationequation (1)
(1)
(1) and formulate full Mundlak-Chamberlain adoption equation by combining
as follows:
Note that Equationequation (4)(4)
(4) is simply a form of a non-linear model when the dependent variable is binary as well as censored. Then, correlated random effect double hurdle model (CRE craggit) is used to estimate the improved wheat adoption decision.
5.2.2. Controlling for Endogeneity (i)
Despite the elimination of the time-invariant variable using the Mundlak-Chamberlain specification yet, there is a possibility that access to the improved technology could be endogenous in the adoption Equationequation (1)(1)
(1) due to potential correlation with leftover unobservable time-varying heterogeneity. This is manifested by the significant number of farm households were non-adopter and the improved wheat adoption is nonrandom (Table ). Thus, access variable in the above Equationequation (1)
(1)
(1) is possibly correlated with the error term cov(Ait, ϵit ≠ 0). The possible way of solving the endogeneity issue associated with unobservable time-varying heterogeneity in adoption Equationequation (1)
(1)
(1) is using instrumental variable method. However, instrumental variable method is appropriate for linear outcome models. Instead, for non-linear models, a control function approach is used on the similar kinds of identification conditions (Wooldridge, Citation2010).
Following Smith and Blundell (Citation1986) approach, for controlling and testing the endogeneity problem in a corner solution model, there are two-step procedure. First, estimate the reduced form access to the improved wheat variable Equationequation (5)(5)
(5) below using correlated random effect probit model and capture the residual (i.e., the difference between the observed and its predicted value, i.e.,
). The reduced form access to the improved wheat equation is presented as a linear function of the exogenous variables including the instrument (Zit). Second, include the predicted value of the access variable (
) in the adoption Equationequation (6)
(6)
(6) along with the endogenous access to the improved wheat variable.
A is dummy variable where a given farmer is accessed to the improved wheat variety or not(1/0). Z is an instrument or exclusion restriction variable expected to influence access variable in Equationequation (5)(5)
(5) but not directly affect the adoption Equationequation (6)
(6)
(6) . This variable exhibits strict exogeneity and time variant.
is a vector of control variables that influence access to the improved wheat variety. Y is a year dummy to capture access and adoption difference over time compared to the base year.
is the mean value of all the time variant control variables in a panel data set.
The control function approach in the adoption model requires exclusion restriction variables or instruments (Zi) in Equationequation (5)(5)
(5) . The exclusion variables have to be uncorrelated with the error term in Equationequation (6)
(6)
(6) , cov(Zit
0), but correlated with the endogenous variable in Equationequation (5)
(5)
(5) ; cov(Zit,
≠ 0). The error term generated from the correlated random effect probit model (
:) is then included as a regressor in Equationequation (6)
(6)
(6) . The statistical significance of the residual provides a test for endogeneity of access to the improved wheat variable. The control function approach requires exclusion restriction(s) as discussed above. In this case, proportion of households residing at the mid and high land agro-ecologies was served as an instrument. The causal mechanism states that as households accessed to the improved technology increased, the likelihood of up taking the technology is expected to increase. Statistical validity test has been done by including the instrument in the adoption equation in one specification. If the instrument was insignificant in the adoption model but significant in the access model, and if the error term (the difference between the observed and predicted value of access variable) was significant in the technology adoption model. Then, endogeneity is an issue and was corrected for with the control function.
On the other hand, the impact estimation procedure is not free from the endogeneity issue. This is due to the variable of interest (area under improved wheat) in the impact estimation model is potentially endogenous variable. This implies that not all farm households used their farms for improved wheat plantation. Then, area under improved wheat plantation will be correlated with the error term in the welfare Equationequation (2)(2)
(2) cov(Qit,
≠ 0). To address this, a three-step estimation procedure is applied. First, estimate area under improved wheat cultivation using a censored tobit model. Second, captured the predicted value of area under improved wheat. Third, estimate linear Fixed Effect Instrumental Variables (FE-IV) for the linear welfare variables and Fixed Effect Linear Probability Instrumental Variables (FELP-IV) model for nonlinear welfare variable (i.e., probability of food secured). In this case, the predicted value of area under improved wheat variety serve as instrument for observed values. The merit of this procedure is more efficient compared to the standard 2SLS when the endogenous regressor is a corner response or censored variable. The exclusion restriction variable in this model is satisfied by the farm characteristics which are not involved in the welfare outcome equations. Tests have done whether farm characteristics affect directly the welfare variables, but results do not show this after controlling for area under improved wheat.
6. Results and discussions
6.1. Improved wheat adoption
The balanced panel data used for the analysis comprise 822 farm households and the summary statistics of the key variables is presented in Table . On average, 25 % of the sampled households are headed by female, implies male headed households are dominant in the study communities which is expected in developing countries, male patriarchal family (Darfour & Rosentrater, Citation2022). This is depicted by on average; the annual growth rate of male headed household is higher than their counter part female headed households by 15 %. Family size of average household is about five persons relatively low compared to other developing countries for instance, nine in Pakistan (Abbas & Chandio, Citation2018); seven in Zambia (Makaiko et al., Citation2015). Age is proxy of human capital and almost all household heads exist in the productive age range (15–65) years. Smallholders are risk averse and used the new technology with skeptical. This is because, the new technology may not be friendly with a changing environment and to minimize the crop loss, farmers would like to use the local wheat. This might be one reason that high percentage of farm households in the study communities dis-adopt the technology (see, Table for the details).
Table 5. Descriptive statistics of variables used in the econometric analysis by survey period (Mean value)
Table shows the results of the Correlated Random Effect Double Hurdle model of improved wheat adoption. Results from Hurdle 1 present factors affecting adoption decision of the technology, while results from Hurdle 2 present factors affecting intensity of technology adoption. The residual from the first stage access to improved wheat variable is included in the adoption equations along with the observed access variable. This helps to test and control for the endogeneity of access variable on adoption of improved wheat technology. Standard errors are estimated using the bootstrap method to account for the two-stage estimation in this control function procedure. Significant coefficient of the residual in Hurdle 1 indicates that improved wheat technology is potentially endogenous variable as expected and the problem of endogeneity is fixed using the control function approach. Estimation results of the first stage CRE probit estimation of access to improved wheat variety is presented in Appendix Table .
Table 6. Improved wheat adoption decision. Double Hurdle model using the Correlated Random Effect (Access to improved wheat treated as endogenous)
Access to improved wheat variety has positive but insignificant effect on improved wheat adoption decision (Hurdle 1), while positive and significant effect on the extent of improved wheat adoption (Hurdle 2) at the 1% level. The result suggests that access to the technology enabled smallholders to allocate relatively substantial land size by those who already adopt the improved technology. This provokes to the Ethiopian government and other developmental agencies that their concern should be promoting access to the improved wheat varieties among the smallholders, so that over time diffusion rate of the technology will be increased. The finding of this study is quite consistent to other similar studies in Ethiopia that access to the improved Chickpea variety significantly and positively contributed to smallholders’ Chickpea adoption decision(Simone et al., Citation2017).
Price of improved wheat affects negatively and significantly technology adoption decision. The marginal effect shows that as price per kilogram of improved wheat increases by one dollar, the probability of improved wheat adoption decreases by 13 % at the 1 % level. The result is consistent with the finding of Bezu et al. (Citation2014) in Malawi that higher maize technology price deters significantly improved maize adoption decision. Households with large number of male adults positively affect adoption of improved wheat. The result shows that for an extra one unit of adult male, other things constant and the likelihood of improved wheat adoption increases by 2.3% at the 5 % level. This is in line with the expectation that adult labor is more robust and less sensitive to risk than any other resource endowments and thus, qualifies to self-reliance to adopt the new technology in a changing environment. The probability and intensity of improved wheat adoption increases with land holding as expected. The marginal effect shows that, for an increase of own land by one hectare on average, the probability of adoption and area under the improved wheat have increased by 8.5% and 6.4 % at the 1 % level, respectively. The implication is that, first wealthier farmers enable to fix the liquidity constraints and financed the higher technology price, in turn show positive demand to the new technology. Second, wealthier farmers can reduce the risk of crop loss by cultivating diversified crop varieties and substantial farm size can allocate for the new technology compared to farmers with limited farm size. Similar findings are prevailed in developing countries where land poor farmers face resource constraints and regret to adopt the new technology (Bezu et al., Citation2014, Ogada et al., Citation2014)
The probability of improved wheat adoption is positively and significantly associated with access to information. The marginal effect describes that households accessed to information adopt the improved wheat by 8.5 % higher than households without access to information. The intuition is that access to information proxy by ownership of communication and media devices (mobile, television and radio) are expected to acquire updated and price information pertaining to the new technology. This is specifically attributed to mobile telephone enhances farmers’ communication with individuals such as model farmers, agricultural extension workers, and traders. This in turn helps to increase farmers’ awareness about the feature of the technology from those who might have prior information.
6.2. Household welfare effect of improved wheat adoption
Household level Fixed Effect model is used to assess the impact of improved wheat adoption on households’ welfare. Here again, standard errors are estimated using the bootstrap method to account for the Fixed Effect Instrumental Variable estimation procedure. The welfare variables included per capita income, per capita consumption expenditure, per capita wheat income, per capita off-farm income and probability of food secured.
Table presents results from the fixed-effects models. The findings show that area under improved wheat is positively and significantly correlated with households’ per capita income, per capita consumption expenditure, per capita wheat income, and the probability of food secured. After controlling other factors, the fixed effect estimates demonstrate that a 1% increases in area under improved wheat leads to an increase in per capita income by 0.32 %, in per capita consumption expenditure by 0.19 %, in per capita wheat income by 2.26 %, and the probability of food secured by 13.7 % at the 1 % level, respectively. This is an encouraging result given landholding in high lands of Ethiopia is very small and fragmented, land-use intensification approach through adoption of modern agricultural technology is a promising strategy to increase crop production and poverty reduction. Other studies in developing countries also found a similar positive impact of adopting improved agricultural technology on households’ income in Ethiopia (Gebremedhin et al., Citation2009); in India (Matuschke et al., Citation2007); in Mexico and Nepal (La Rovere et al., Citation2008); in Malawi (Bezu et al., Citation2014); in Zambia (Makaiko et al., Citation2015).
Table 7. Welfare effect of improved wheat adoption (Fixed Effect models)
Farm households’ income includes crop and crop by-products (residuals), livestock production, and from off-farm activities. The off-farm income is generated from activities other than farming related activities. The per capita wheat income is the sum total of local and improved wheat varieties.
The fixed effect result also demonstrates that adoption of improved wheat has a positive but insignificant correlation with per capita off-farm income. This was intended to show whether improved wheat adoption has a crowding in or crowding out effect with activities out of farming. The intuition is that technology adoption expected to demand more labor and perhaps family labor was probable invest on improved wheat plantation at the expense of participating in off- farm activities. However, result does not support this prior expectation. This may be due to the availability of excess labor in rural Ethiopia and the labor absorbing potential of adopting the improved technology is below the expectation, and the excess family labor exits from farming and join to the off farm income generating activities. Thus, adoption of improved wheat variety has a crowding in effect to off farm activities, but with weak economic link.
Other significant covariates of the fixed effect estimation include family labor, land holdings, number of oxen and non-ox-livestock units. Households with an extra one unit of labor force are positively associated with the probability of food secured household. This implies that the availability of an active labor force is an important component of farming and enables to keep the food requirement of the household. Households with an extra one unit of ox are correlated negatively and significantly with per capita off-farm income. This is quite intuitive. Given the importance of ox in farming (labor intensive activity), household with extra number of oxen expected to cultivate substantial land size and grow the improved technology and obtain higher farm income at the expense of off farm income. On the contrary, a household with an extra one unit of non-ox livestock unit is correlated positively and significantly with per capita off-farm income. The possible justification could be, non -ox livestock are mostly back animals such as donkey, mule, and camel are important tools in transporting commodities in the rural areas, perhaps generate higher off-farm income by renting out of these resources.
6.3. Benefit comparison of improved wheat adoption: A disaggregate analysis
In this section, disaggregate welfare models of per capita wheat income and probability of food secured households are analyzed. This is aimed to compare the impact of technology adoption on the above welfare variables between male-headed and female-headed households, and between land poor and land rich households. Fixed effect models are used to estimate the impact.
The fixed effect estimation results of total wheat income and probability of food secured for male-headed and female-headed households are reported on Table . Results revealed that a percentage increase in the area under improved wheat is associated with increases in the per capita wheat income for male and female -headed households by about 2.4 % and 2% at the 1 % level, respectively. Similarly, a percentage increase in the area under improved wheat is associated with increase the probability of food secured household by 0.21% for male headed households, at the 1% level and by 0.35% for female headed households, but insignificant. This study verifies the impact of improved wheat adoption on wheat income and poverty reduction is not gender sensitive and no evidence of household-head gender difference in the effect of improved wheat adoption decision. It seems that all households have a potential to capture the same benefits from the use of improved wheat.
Table 8. Comparison of wheat income and probability of food secured for male- and female-headed households(Fixed Effect Models)
Results from estimation of wheat income and food security disaggregated by a households’ wealth status are reported in Table . Wealth in this case refers to the farm size owned by the households. Farmers are grouped in to five quantiles. Households fall in the first quantile are relatively poor, while households fall in the fifth quantile are relatively rich. Table presents the quantile fixed effect model results. Results indicate that area under improved wheat cultivation is positively and significantly correlated with wheat income of both quantiles. Keeping other variable’s effect unchanged, a percentage increase in the area under improved wheat is associated with increase in the per capita wheat income of household by 2.30 %, 1.67 %, 2.84%, 3.14% and 3.50 % in the first to the fifth quantile, at the 1 % level, respectively. This indicates that although there is landholding size variation among rural households, both groups (the land rich and land-poor households) are benefited from adoption of the technology and one can argue that on average, all Ethiopian farmers are benefited from adoption of the improved wheat variety. But wealthier households are likely to benefit more in terms of wheat income and the probability of food secured from cultivating improved wheat varieties. This indicates that wealthier households, who can allocate substantial farm size, can afford technology price and neutralize the risk associated with the technology.
Table 9. Comparison of Quantile Fixed Effects model estimations of wheat income (log form)
7. Conclusions and policy implications
This article uses household balanced panel data of 274 farm households for the period 2005/06–2014/15 from the semi-arid Tigrai region in northern Ethiopia to assess adoption of improved agricultural technology and systematically evaluate its impact on welfare of smallholder farmers. In particular, the study has assessed the effect of access to improved wheat variety, landholding, family labor, population pressure, and access to information, access to irrigation on the likelihood and intensity of adoption of the improved wheat technology and show positive effect. Overall, the study finds low adoption rate and relatively small land size is planted with the improved wheat.
The ex-post welfare impacts of improved wheat adoption are estimated using fixed effect models, and results revealed that area under improved wheat is positively correlated with households’ per capita income, per capita consumption expenditure, wheat income and food security. In addition, the finding of this study shows that both poor and better off households are benefited from adoption of improved wheat variety. This indicates the importance of wheat technology for poor families by ensuring higher income and uplifting rural households out of poverty.
This study provides new evidence to the existing literature and other countries. First, the study includes consistent data collection methods over time, use long-run satellite rainfall and rainfall variability data, which is not common in developing countries. The intuition is weather station in countries like Ethiopia (in developing countries in general) is set to cover wider geographical areas and thus their use as climate shock variables at micro level could be less meaningful in this case. This study avoids the reservation on the reliability of using rainfall data collected from “nearest” weather station. Second, the survey covers various agro ecologies and having data from wider areas with substantial variation in population pressure, market access, access to irrigation, access to infrastructure and gives robust and comprehensive findings. Third, the positive effect of improved wheat variety adoption on households’ welfare is an encouraging result, especially in view of the finding that female-headed households are equal likely to use the improved wheat technology with male headed households. The results in this study taken together and lend evidence that the use of improved wheat technology is good for improving the welfare of the poor and women farmers in a land scarce and populated areas.
This paper builds on Schultz (Citation1964) that risk-averse farmer adoption behavior appears to be correlated to local conditions, human capital, endowment, and production risk. Thus, results of this study have some policy implications.
First, there is a need for increase diffusion efforts for improved wheat variety in less-dense population areas suitable for wheat production. Farmers in these areas are significantly less likely to adopt improved wheat, and this study finds it likely that improved diffusion efforts could increase the adoption in these areas.
Second, higher technology price discourages smallholders’ adoption decision. This partly attributed to financial constraints to afford technology price in a risk environment. Thus, there is a need for access to rural credit market, so that smallholders enable to fix the liquidity constraints and encouraged to adopt the improved technology.
Third, technology adoption is dynamic and learning process. Results reveal that better information dissemination concerning the new technology enhances technology adoption decision among smallholders. Reducing transaction costs through implementing cost effective agricultural policies such as provision of mobile telephone based extension services that helps to enhance access to and bridge the technology gap can significantly contribute toward improved food security in the rural areas.
The study is demanding and results are comprehensive and intuitive, but to some extent it accommodates few limitations. First, the survey does not have detailed data on access to extension services that may have affected the technology diffusion processes among smallholders via learning by doing. It is known that extension programs to stimulate the adoption of agricultural technologies have been part of the Ethiopian government’s policies since the mid-1990s. Second, the study reserves to assess the effect of other yield enhancing technologies like inorganic fertilizer on adoption decision of the improved wheat variety. It leaves these issues for future studies. Third, the study region is mid and high land and local wheat crop is affected by rust. Growing improved wheat variety resists to “ leaf rust”(Puccinia striiformis) and “stem rust” (P. graminis) during maturity period. This study is less likely to notice information about farmers’ adoption decision is also based on the advantage of its rust resistance. Given the complexity and seriousness of the issues, much more research is needed within this area.
Acknowledgements
Data collection has been funded by NORAD through the NOMA and NORHED programs, especially the “Climate-Smart Natural Resource Management and Policy” (CLISNARP) collaborative research and capacity-building program between the School of Economics and Business at Norwegian University of Life Sciences, Mekelle University, Ethiopia, and LUANAR University in Malawi.
Disclosure statement
No potential conflict of interest was reported by the author(s).
Additional information
Funding
Notes on contributors
Menasbo Gebru Tesfay
Menasbo Gebru Tesfay completed his First and Second Degree in Economics, from Mekelle University, Ethiopia. He studied PhD in Economics at Norwegian University of Life Sciences, Norway. He has been teaching economics courses to undergraduate and post graduate students at the Department of Economics, Mekelle University, for more than 10 years. He has published more than 12 articles at national and international journals. His research interests are on land use policies, land markets, technology adoption, agricultural marketing, welfare analysis and program evaluations.
References
- Abbas, A., & Chandio, Y. J. (2018). Factors influencing the adoption of improved wheat varieties by rural households in Pakistan. Agric Food, 3(3), 216–26. https://doi.org/10.3934/adgrfood.2018.3.216
- Aklilu, A., Messeret, L., & Abera, A. (2022). Adoption of improved wheat production technology in Gorech district, Ethiopia. Agric Food Sec, 11(3). https://doi.org/10.1186/s40066-021-343-4
- Bezu, S., Kassie, G. T., Shiferaw, B., & Ricker-Gilbert, J. (2014). Impact of improved maize adoption on welfare of farm households in Malawi: A panel data analysis. World Dev, 59, 120–131. https://doi.org/10.1016/j.worlddev.2014.01.023
- BPF (Cartographer). (2014). Bureau of Planning and Finance, Atlas of Tigrai regional state. Tigrai regional state.
- Burke, W. J. (2009). Fitting and interpreting Cragg’s tobit alternative using Stata. Stata J, 9(4), 584. https://doi.org/10.1177/1536867X0900900405
- Cameron, A. C., & Trivedi, P. K. (2010). Microeconometrics using Stata (Revised) ed.). Cambridge university press.
- Chamberlain, G. (1982). Multivariate regression models for panel data. J Economet, 18(1), 5–46. https://doi.org/10.1016/0304-4076(82)90094-X.
- Christine, M. M., & Christopher, B. B. (2006). The complex dynamics of smallholder technology adoption: The case of SRI in Madagascar. Agric Econ, 35(2006), 373–388. https://doi.org/10.1111/j.1574-0862.2006.00169.x
- Darfour, B., & Rosentrater, K. A. (2022). Pre harvest and post-harvest farmers expreicnes and practis in five maize growing regions in Ghana. Front Nutr, 9, 725815. https://doi.org/10.5389/frnt.2022.72515
- Doss, C. R., Mwangi, W, Verkuijl, H, & De Groote, H (2003). Adoption of maize and wheat technologies in Eastern Africa: A synthesis of the findings of 22 case studies: CIMMYT. https://repository.cimmyt.org/xmlui/bitstream/handle/10883/1037/76070.pdf. Accessed June 12, 2019
- AFDB. (2012). Malawi interim country strategy paper 2011–2012. African Development Bank.
- Feder, G., Just, R. E., & Zilberman, D. (1985). Adoption of agricultural innovations in developing countries: A survey. Available at 0013-0079/85/3302-0025 USD01.00. Econ Dev Cult Chag, 332(2), 255–298. https://doi.org/10.1086/451461
- Gebremedhin, B., Jaleta, M., & Hoekstra, D. (2009). Smallholders, institutional services, and commercial transformation in Ethiopia. Agric Econ, 40(s1), 773–787. https://doi.org/10.1111/j.1574-0862.2009.00414.x
- Hagos, F., & Holden, S. (2003). Rural household poverty dynamics in northern Ethiopia 1997-2000: Analysis of determinants of poverty. Paper presented at the Chronic Poverty Research Centre International Conference, Manchester. Accessed September 2016, available at http://www.chronicpoverty.org/uploads/publication_files/CP_2003_Hagos_Holden.pdf.
- Kelemu, K. (2017). Determinants of farmers access to information about improved wheat varieties: case of farmers in major wheat growing regions of Ethiopia. Int J R Agric Sci, 4(1). Accessed July 27, 2019. https://www.researchgate.net/profile/Kaleb_Kelemu/publication/316644343
- Kotu, B. H., Verkuijl, H., Mwangi, W., & Tanner, D. (2000). Adoption of improved wheat technologies in Adaba and Dodola Woredas of the Bale Highlands, Ethiopia: CIMMYT. https://pdfs.semanticscholar.org/2a4a/eaa6da19f93987675df16cadbd74d279a797.pdf. Accessed July 2, 2019
- La Rovere, R., Mathema, S. B., Dixon, J., Aquino-Mercado, P., Gurung, K. J., & Hodson, D. (2008). Economic and livelihood impacts of maize research in hill regions in Mexico and Nepal: Including a method for collecting and analyzing spatial data using Google Earth. CIMMYT.
- Lobell, D. B., Ortiz-Monasterio, J. I., Asner, G. P., Matson, P. A., Naylor, R. L., & Falcon, W. P. (2005). Analysis of wheat yield and climatic trends in Mexico. Field Cr Res, 94(23), 250–256. https://doi.org/10.1016/j.fcr.2005.01.007
- Makaiko, K., Julius, M., Arega, D. A., & Menela, K. (2015). Analysis of adoption and imapct of improved maize varieties in Eastern Zambia. World Dev, 66, 609–706. http://dx.org/10.1016/j.wporlddev.2014.09.008
- Matuschke, I., Mishra, R. R., & Qaim, M. (2007). Adoption and impact of hybrid wheat in India. World Dev, 35(8), 1422–1435. https://doi.org/10.1016/j.worlddev.2007.04.005
- Maya, F., Brett, D., & Nelson, S. (2013). Limitations of the millennium development Goals: A literature review. Global Public Health, 8(1:), 1109–1122. https://doi.org/10.1080/17441692.2013.845676
- Mundlak, Y. (1978). On the Pooling of Time Series and Cross Section Data. Econmetrica: J Econom Soc, 69–85. https://doi.org/10.2307/1913646
- Nick, W. (2021). Making the Green Revolution greener: Agricultural adaptation and climate change. https://www.theigc.org/blog/making-the-green-revolution-greener-agricultural-adaptation-and-climate-change
- Ogada, M., Germano, J., & Diana, M, M. (2014). Farm technology adoption in Kenya: A simultaneous estimation of inorganic fertilizer and improved maize variety adoption decisions. Agric Food Econ, 2(1), 12. https://doi.org/10.1186/s40100-014-0012-3
- Sadoulet, E., & De Janvry, A. (1995). Quantitative Development Policy Analysis. Exercise Solutions: Johns Hopkins University Press. http://caoye.lzu.edu.cn/upload/news/N20141106111536.pdf. Accesssed Feb 4, 2019.
- Schultz, T. W. (1964). Transforming Traditional Agriculture.Yale. University Press.
- Shiferaw, B., Kassie, M., Jaleta, M., & Yirga, C. (2014). Adoption of improved wheat varieties and impacts on household food security in Ethiopia. Food Pol, 44(272–284), 272–284. https://doi.org/10.1016/j.foodpol.2013.09.012
- Simone, V., Bernard, G. M., Kai, M., & Jeffrey, D. M. (2017). Welfare impacts of improved chickpea adoption: A pathway for rural development in Ethiopia. Food Pol, 66(2017), 50–61. http://dx.doi.org/10.1016/j.foodpol.2016.11.007
- Singh, I., Squire, L., & Strauss, J. (1986). Agricultural household models: Extensions, applications, and policy. Johns Hopkins University Press.
- Singh, G. M., Xu, J., Schaefer, D., Ray, W. Z., Wang, Z., & Zhang, F. (2022). Maize diversity for full armyworm resistance in a warming world. Crop Sci, 62(1), 1–19. https://doi.org/10.1002/csc2.20649
- Smith, R. J., & Blundell, R. W. (1986). An exogeneity test for a simultaneous equation Tobit model with an application to labor supply. Econometrica: J Econom Soc, 54(3), 679–685. https://doi.org/10.2307/1911314
- Tesfaye, S., Bedada, B., & Mesay, Y. (2016). Impact of improved wheat technology adoption on productivity and income in Ethiopia. Afric Cro Sc J, 24(1), 127–135. https://doi.org/10.4314/acsj.V24i1.14S
- Tigray, B. (2014). Bureau of Finance and Economic Development, Five Years (2010/11-2014/15) Growth & Transformation Plan.
- Titus, B., & Adetokunbo, G. (2007). An analysis of food security situation among Nigerian urban households: Evidence from Lagos State, Nigeria. J Cen Eur Agric, 8(3), 397–406. Accessed May 2, 2019. https://hrcak.srce.hr/19609
- Tobin, J. (1958). Estimation of relationships for limited dependent variables. Econometrica, 26(1), 24–36. https://doi.org/10.2307/1907382
- Tsegaye, M., & Bekelle, H. (2012). Impact of Adoption of Improved Wheat Technology on Households’ Food consumption in Southern Ethiopia. Selected poster presentation at the International Association of Agricultural Economists (IAAE)Triennial Conference Fozdolguacu. Brazil, 18-24 August, 2012.
- Wooldridge, J. M. (2010). Econometric analysis of cross section and panel data: MIT press. https://mitpress.mit.edu/books/econometric-analysis-cross-section-and-panel-data-sec. Accessed August 3, 2019
- Workineh, A., Tayech, L., & Ehite, H. K. (2020). Agricultural technology adoption and impact on smallholder farmers’ welfare in Ethiopia. Afric J Agric Res, 15(3), 431–445. https://doi.org/10.5897/AJAR2019.1402
- Xiaomin, L., Karsten, S., Yajuan, L., & Jima Reiner, D. (2022).Farm). size, farmers’ perception and chemical fertilizer over use in grain production: Evidence from maize farmers in northern China. J Env Man, 325(Part A), 116347. https://doi.org/10.1016/j.jenvman2022.6347
Table A1.
Correlated Random Effect probit model of access to improved wheat technology