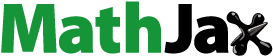
Abstract
Maize is a major staple produced by most peasant farmers in Ghana, amidst climate variabilities that potentially thwart the attainment of global sustainable development goals (SDGs), specifically SDG −2 of zero hunger. Ordinarily, one expects the extant literature to be replete on a nexus between climate variability adaptation strategies and maize yields. Ironically, there appears to be scant information on the expected nexus in Ghana’s coastal areas. The dual questions about what adaptation strategies significantly affect maize yield, and the extent (magnitude) to which climate variability strategies affect maize yield beg answering. Inspired by these research questions, the objective of this article is to examine the effect of climate variability adaptation strategies on maize yield. This study relies on a cross-sectional data covering 197 smallholder maize farmers in the Cape Coast Metropolitan Assembly of Ghana’s Central Region. The study is deeply rooted in a quantitative approach employing multiple linear regression and a treatment effect model (inverse probability weighted regression adjustment—IPWRA). Our findings reveal that adaptation strategies correlate with maize yields. Specifically, estimates from the IPWRA show that irrigation and changes in planting dates positively correlate with maize yields. The implication is that these adaptation strategies improve maize yields. Smallholder farmers are encouraged to adopt effective climate variability adaptation strategies to minimize the adverse risks associated with climate variability. The government of Ghana’s initiative for arid regions, dubbed as the “one village one dam” initiative can be upscaled to southern Ghana to ensure sustainable agricultural development.
1. Introduction
Most countries south of the Sahara have been cited to be highly vulnerable to the adverse effects of climate variability because such countries major economic activities are anchored on agriculture, and largely dependent on the climate, which continues to be unpredictable given the high variations (Ankrah et al., Citation2023; Anum et al., Citation2022). However, dependence on natural climatic factors has been shown to be detrimental to crop yields. For instance, erratic rainfall and droughts constitute major climate factors that cause food insecurities in Ethiopia (Conway & Schipper, Citation2011; Demeke et al., Citation2011). Bajguz and Hayat (Citation2009) showed that increased temperatures accounted for about half (50%) of yield loss. Climate variability directly impacts crop yield and phenology (Duncan et al., Citation2015; Ruane et al., Citation2013). Variations in rainfall and temperature may potentially lead to dry spells and a reduction in crop maturity periods. The literature (Lobell & Gourdji, Citation2012; Lombardozzi et al., Citation2015) establishes that temperature increase reduces crop yields, increases crop respiratory cycles, photosynthetic activities, and damage plant cells. Despite the adverse effects of climate variability on crop yields, there continues to be a high reliance by smallholder farmers on the natural climate for crop production. Fewer farmers practice irrigation, as indicated by Okyere et al. (Citation2022), and similarly, fewer smallholder farmers subscribe to agricultural insurance as an effective mitigation measure to minimize the potential risks of depending solely on the natural climate in Ghana (Ankrah et al., Citation2021).
We first set the tone by differentiating between climate variability and climate change. Christensen et al. (Citation2007) defined climate variability as changes that occur in the average climate variables such as temperature, sunlight, rainfall, humidity etc., covering temporal and spatial scales that extend beyond individual weather events. Whereas climate change refers to changes in climatic variables that extend over a prolonged period often 10 years and above, due to natural occurrences and human activities (Anum et al., Citation2022; IPCC, Citation2018). The distinction between the two definitions lie in the fact that the climate variability accumulates over a prolonged time to yield climate change. This article is concerned with climate variability adaptation strategies and focuses on only smallholder farmers short-term climate adaptation strategies.
This article focuses on climate variability and maize yield in Ghana for the following reasons. First, Ghana forms part of the countries identified to be less resilient to climate variability effects due to the country’s high dependence on agriculture. For instance, Derkyi et al. (Citation2018) argued that smallholder farmers continue to struggle with variations in climate in Ghana. Kabo-Bah et al. (Citation2016) indicated that Ghana is already contending with the adverse effects of climate extremes, with longer, hotter days during the dry seasons and more floods during the rainy seasons. Nkrumah et al. (Citation2014) earlier confirmed rising temperatures in various parts of Ghana. Second, Ankrah et al. (Citation2021) argued that cereals generally constitute major staples in Africa. Maize is an essential staple in Ghana consumed by different social classes (i.e., the rich and poor) with additional use in feed composition for livestock (Ankrah et al., Citation2023). Thirdly, due to population increase and dietary changes, demand for grains such as maize is predicted to triple in SSA by 2050 (Kikoyo & Nobert, Citation2016). Smallholder farmers continuous production of cereals using current production practices is in doubt. Islam et al. (Citation2016) predicted that by 2050, maize yields will be 25% lower due to climate variability globally. Tripathi et al. (Citation2016) indicated that relative to rice and wheat, maize yield remains adversely affected by climate variability. The related literature clearly establishes that climate variability influences maize yields, hence climate variability adaptation strategies remain critical in the quest to minimize the adverse effects, especially in the context of limited crop insurance. This article, therefore, addresses the research questions: What climate variability adaptation strategies affect maize yield? And to what extent do specific climate variability adaptation strategies affect maize yield and the magnitude thereof?
Effective climate variability strategies are crucial in the fight against climate variability in reducing the adverse effects. In this regard, numerous adaptation strategies exist that include using hybrid seeds, drought-tolerant varieties, delayed planting, irrigation, indigenous knowledge adaptations, etc. Rao et al. (Citation2022) showed that delayed planting of crops minimizes maize yield loss in India. The literature (Kassie et al., Citation2015; Shrestha, Citation2014) shows that delayed sowing of crops avoids drought and heat stress at the juvenile stages of plant growth. Tingem and Rivington (Citation2009) indicated that delayed sowing of maize by 60 days led to optimum maize yields in Cameroun. Deb et al. (Citation2015) indicated that a delayed planting could lead to 5–22.5% increase in maize yield in India. Tachie-Obeng et al. (Citation2013) indicated that a delayed planting time of 6 months increases crop yield by 8.2% in northern Ghana. Additionally, the authors showed that improved maize varieties increased maize yields. This finding on improved crop varieties as a climate variability strategy to increase maize yields is supported in Ghana (Antwi-Agyei et al., Citation2014), Cameroun (Tingem & Rivington, Citation2009), South America (Travasso et al., Citation2006), and Uganda (Epule et al., Citation2018). Generally, the literature (Lv et al., Citation2020; Saddique et al., Citation2020) establishes that delayed sowing time improves crop yield, and supplementary irrigation improves maize yield. Additionally, increased fertilizer application (Asrat & Simane, Citation2018; Rao et al., Citation2022), soil and moisture conservation practices (Kikoyo & Nobert, Citation2016) improve maize yield. Kikoyo and Nobert (Citation2016) indicated that improved soil fertility, as a single climate variability strategy, had less effect on crop yields when rains are low. Conspicuously, missing in the adaptation pieces of literature are copious information on how climate variability adaptation strategies affect maize yields in Ghana, despite the country’s vulnerability to climate variability. Studies examining climate variability adaptation strategies on maize yields have been scant even though maize is an important staple. Rather, most studies (Antwi-Agyei et al., Citation2012; Atiah et al., Citation2022) examine how changes in climatic variables affect maize yields. For instance, Cudjoe et al. (Citation2021) examined how changes in temperature and rainfall affected maize yield in southern Ghana.
Our study’s novelty lies in the focus on how climate variability adaptation strategies influence maize yields, distinct from the replete literatures that have overly examined the quantitative assessment of climatic (Baffour-Ata et al., Citation2021, Citation2023; Cudjoe et al., Citation2021; Srivastava et al., Citation2018) or non-climatic variables (Aidoo et al., Citation2021; Ankrah et al., Citation2023; Anum et al., Citation2022; Asante et al., Citation2021; Okyere et al., Citation2022) effect on maize yields in Ghana. The climate variability literature in Ghana, appears scant relative to the climate change literature, and thus there is a need for sustained research to ascertain how climate variability adaptation strategies affect maize yield in Ghana.
We use Ghana as a case in point to illuminate the more comprehensive policy implications for sub—Saharan Africa (SSA), given that maize is a major staple that contributes to the dietary needs and income of farmers. Indeed, the concern about the scarce literature on the nexus between the effect of climate variability adaptation strategies on cereal crop yields has recently been echoed by Atiah et al. (Citation2022). We draw inspiration from the recent concern by contributing to showing what climate variability adaptation strategies work and what does not work effectively in improving maize yields in southern Ghana. We note that what works in another country, might not necessarily work in Ghana, given that even within Ghana, specific adaptation strategies do not work throughout the country but remain bound to specific geographies amidst variabilities over time. Specifically, we show that irrigation and staggering planting dates (early or late planting) positively correlate with maize yields. The implication is that adequate financial investments are encouraged to be channelled into formalizing peasant informal irrigation schemes, as well as encourage the increased adoption of changes in planting dates (early or late planting) to increase maize yields. Generally, the limited climate variability adaptation literature in Ghana, appear overly concentrated on northern Ghana (Bawayelaazaa Nyuor et al., Citation2016; Ndamani & Watanabe, Citation2014), which obviously faces the extremes of the weather variables (rainfall, sunshine, relative humidity etc) coupled with the geographical space unimodal rainfall pattern. For instance, Baffour-Ata et al. (Citation2021) examined climate variability effects on staples in northern Ghana. We, however, argue that southern Ghana also deserves attention because it contributes significantly to the food staple production and equally stands at the mercy of weather variations. Hence, the need to contribute to the unbalanced literature on climate variability and cereal crop yield nexus, which systematically remains skewed towards northern Ghana, and by extension, most northern spaces in other countries in South-Saharan Africa (SSA). Finally, our study adds to the estimation rigour by using a doubly robust treatment effect—the inverse probability weighted regression adjustment (IPWRA)- which remains rarely used in the climate variability and crop yield nexus.
The article unfolds as follows in terms of structure: next is an overview of the methodology that foregrounds the study. The penultimate section presents the results and undertakes a discussion of the results within the established literature. We finally conclude and proffer policy recommendations worthy of consideration.
2. Materials and methods
2.1. Study area
The study was undertaken in Ghana’s Central Region, specifically, the Cape Coast Metropolitan Assembly (See Figure ). The study site was selected due to consistently available data obtained from the Statistics Research and Information Department (SRID), and the Ministry of Food and Agriculture (MoFA). The data showed low maize yields. We found missing consistent data on maize yields spanning several years, hence we restricted our information to available data. We found that the low yields in the study area were partly due to climate variability, therefore the need to investigate what pertains. The information on the factors responsible for low maize yields was based on available extant literature and exploratory study with officials in the Department of Agriculture in the metropolitan assembly. The Ghana Meteorological Agency (GMeT) provided reliable data on weather variables (sunshine, rainfall, and temperature) from the year under consideration (Ankrah et al., Citation2023).
Figure 1. Map of Cape Coast Metropolitan Assembly showing study areas.
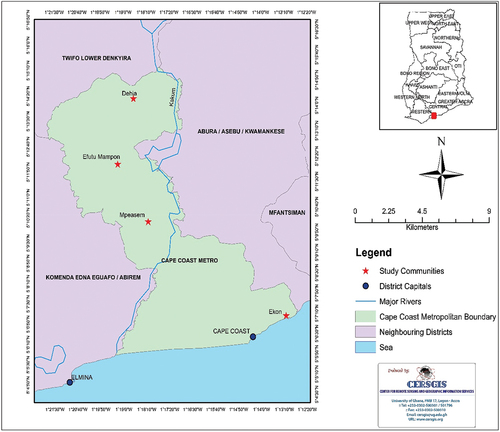
The Cape Coast Metropolis has an area of 122 square kilometers in total. It lies within longitude 15W and latitude
06N. It is bounded on the west by Komenda Edina Eguafo or Abrem Municipality, Abura Asebu Kwamankese District by the east, Twifo Hemang Lower Denkyira District by the north, and the Gulf of Guinea by the south. The total population as of 2010 stood at 118,106. Males were less (57,365) than females (60,741). The majority (60%) of farmers, and fishermen are found in agricultural-related activities (Ghana Statistical Service, Citation2010). The active agricultural workforce stands at 28,000, with few (0.3%) large scale/commercial farmers and the majority (99.7%) smallholder farmers. About 8,000 ha of potential arable lands exist, but only 3,500 Ha is under cultivation (Ankrah et al., Citation2023).
The secondary forest is the main vegetation interspersed with thickets and shrubs. A coastline of 13 km long exists in the study area. Relative humidity ranges between 60% to 80%. While temperatures lie between C to
C. Two main seasons exists i.e., the rainy season, which peaks in May, June and October. Typical annual rainfall precipitation falls between 90 cm to 110 cm along the coast, while the villages witness precipitation within the range of 110 cm to 160 cm. The dry periods start in November and February (Ankrah et al., Citation2023; Ghana Statistical Service, Citation2010).
2.2. Study population
Babbie (Citation2020) defines a population as the total aggregate respondents. In our study, the population includes all maize farmers in the study site.
2.3. Sampling and sample size
Sample size defines individual subsets drawn from a population. Taking inspiration from Yamane (Citation1967) formula for determining sample size, we obtained a minimum sample size of 394 based on the formula below:
n represents the sample size, where N represents for the population size and e represents the precision level. The study area had a population size of 28,000, therefore based on a 95% confidence level, a degree of variability (p = 0.5) and a precision level (e = 5%). We obtained a minimum sample size of: =
=
Based on the formula, we obtained a sample size of n = 394 farmers. However, due to the start of Covid-19, it became a challenge to interview all the farmers based on the fear of contracting the COVID-19 virus although all the required safety protocols were adhered to. Additionally, limited time did not permit the researchers to stay longer to collect data. Thus, the expected sample size was divided into two yielding a sample of 197 (See Ankrah et al., Citation2023).
In the simple random sampling approach, every individual has an equal and likely chance of being selected from the population (Creswell & Creswell, Citation2017). Thus, this sampling approach permits a more representative sample and reduces selection bias. Except for Ekon community where only 47 farmers were selected based on the unavailability of three respondents, fifty (50) farmers were selected from the other communities (See Ankrah et al., Citation2023).
2.4. Development of data collection instrument
The research objective of our study guided the design of the data collection instrument. A structured questionnaire comprising closed and open-ended questions was used to elicit primary information on smallholder maize outputs, and adaptation strategies. The questionnaire was first pre-tested for reliability and validity of data. Cohen et al. (Citation2017) defined validity as the level of accuracy that is applied to the research process in obtaining accurate and consistent measurements. The researchers pre-tested the questionnaires in the Agona West Municipal Assembly, Central Region. This involved a sample of 20 farmers. The responses helped amend the final questionnaire to accommodate questions that the researchers never thought of. It is worthy to note that the study area selected for the pre-test was totally different from the actual study area for the study. Therefore, the pre-test community did not in any way affect the responses obtained in the final selected study area.
2.5. Data collection
The questionnaires were administered in-person through interviewing the selected respondents in four communities (Dahia, Efutu Mampon, Mpeasem, and Ekon). The selection of the four communities was undertaken randomly from the list of maize growing communities obtained from the Department of Agriculture in the Cape Coast Municipal Assembly. The researchers adhered strictly to the COVID-19 protocols including washing hands, wearing masks, the use of hand sanitizers, and social distancing. However, few respondents that could not be reached, were interviewed through their mobile phones. The data collection commenced in May, and eventually came to an end in July, 2021. The administration of the questionnaires was done in the local language of the respondents (i.e., the Fante language). But the responses to the questionnaires were captured in the English language by a trained enumerator who translated the questions into the local language and vice versa. Typically, a questionnaire administered lasted for about 35 minutes.
2.6. Research design
This study falls within the positivist paradigm that relies solely on quantitative method of enquiry. The study used cross-sectional survey involving 197 maize farmers through questionnaire administration. The use of questionnaire permits the analysis of knowledge, determination of relationships among variables quantitively, opinions, trends, and attitudes (Creswell & Creswell, Citation2017). Additionally, cross-sectional data permit researchers to collect descriptive information (socio-demographic characteristics, farm level characteristics), and information that relates variables. For example, the effect of climate adaptation strategies on maize yields). Questionnaires permit the collection of varied and rich data from a sampled population (Ankrah et al., Citation2023; Leavy, Citation2017).
2.7. Data analysis and processing
This study examines the effects of climate variability adaptation strategies (CVAS) on maize yield in Ghana’s Central Region. In addressing the research objective, the study employs two empirical models: (1) multiple linear regression (MLR) and (2) inverse probability weighting regression adjustment (IPWRA). The MLR model is specified as follows:
where represents total quantity of maize produced per hectare for farmer
,
is the constant term,
are the estimated coefficients
are the Kth independent variables (including the various CVAS), and
is the error term assumed to be randomly distributed. (Please see the independent variable used in Table ). Similarly, the study uses EquationEquation (1)
(1)
(1) to estimate the effect of the intensity of CVAS adoption on maize yield. Here, instead of relying on the individual CVAS, which are measured as dummy variables, we used the number of CVAS adopted by the maize farmers. Lastly, addressing the potentially upward biased estimates from the MLR models, the study employed the IPWRA model-a treatment estimation strategy with the so-called doubly robust properties (Cattaneo, Citation2010; Wooldridge, Citation2010). Therefore, this model addresses the potential self-selection bias and heterogeneity in the adoption of CVAS. For instance, the adoption of CVAS is not random, since farmers may adopt based on observed and non-observed factors. Therefore, failure to account for this potential source of self-selection could lead to upward biased estimates. Interestingly, this model has been applied by various studies and it has generated robust evidence on the effect of the adoption of various agricultural technologies (see for instance (Drukker, Citation2016; Manda et al., Citation2018; Tambo & Mockshell, Citation2018)., Probit model is used to specify the treatment model while ordinary least squares are used to estimate the outcome model. We also report robust standard errors for all the regressions. Finally, we estimate the average effect on the treated (ATT) using Stata version 14.2.
Table 1. Independent variables used
3. Results and discussion
3.1. Descriptive statistics
3.1.1. Respondents socioeconomic characteristics
Table below presents the results of the socioeconomic characteristics mainly the sex, educational level, and marital status. The socio-demographic characteristics provide context for understanding the interpretation of the results.
Table 2. Farmers demographic characteristics
Table shows that majority (85%) of the farmers interviewed were males. Male domination in agriculture generally permeates at the national level in Ghana, it is therefore not surprising that males dominate in the agricultural activities in the study area. The Ghana Statistical Service (Citation2020) supports the finding on male dominance in agriculture. Other recent studies (Ankrah et al., Citation2022; Anum et al., Citation2022; Kwapong et al., Citation2021) support the male dominance in agriculture in Ghana. The male dominance is probably due to the labourious nature of agriculture, which generally disadvantages women (Antwi & Antwi-Agyei, Citation2023; Dzanku & Tsikata, Citation2022; Ogisi & Begho, Citation2023; Quisumbing et al., Citation2014; Tufa et al., Citation2022).
Table shows that approximate 8% of respondents are widowed. We found respondents level of education falling into six categories: no formal education, primary/basic education (primary and Junior High School -JHS), senior high education (Senior High School (SHS), and tertiary. Majority (67%) of the farmers had no formal education. For the limited farmers with formal education, the highest level appeared to be basic school (21%), followed by SHS (5%). Only 4% and 3% had tertiary and technical/vocational education respectively (Ankrah et al., Citation2023). This low level of farmers education has been upheld by other studies (Awunyo-Vitor et al., Citation2016; Kwapong et al., Citation2021).
Table above presents the additional socio-economic characteristics of the farmers. This includes income, farm size, maize yield, and farming experience. The average maize farmers income was estimated to be GH¢ 2,693 (US$ 456).Footnote1 The minimum and maximum farmers income was estimated to be GH¢ 960 (US$163) and GH¢ 8400 (US$ 1424) respectively. Farmers fell within an average farming experience of 13 years, an average farm size of 1.08 hectares, and a minimum farm size of 0.4 hectares. The farmers background shows an appreciable level of experience engaged in farming activities. The higher the farming experience, farmers tend to better adapt to climate variability including the use of indigenous coping strategies (Ankrah et al., Citation2022, Citation2023; Makondo & Thomas, Citation2018). The average maize yield of the farmers was 1.12 tonnes. The farm size of 1 hectare point to the scale of operation of the farmers involved in the study, to be mostly smallholder farmers. Generally, the evidence (Afranaa Kwapong & Ankrah, Citation2023; Ghana Statistical Service, Citation2010, Citation2020; Kwapong et al., Citation2021) shows dominance of smallholder farmers in the agricultural space in Ghana.
3.1.2. Estimates from multiple linear regression
First, we refer to Appendix 1 on a test performed to check multicollinearity issues that can render our estimates biased for the multiple linear regression model. The result shows acceptable values of the variance inflation factor with a mean value of 1.22. In fact, the result reveals a very low mean variance inflation factor implying no issues of multicollinearity. In addition, we performed a heteroskedasticity test using the Breusch-Pagan/Cook-Weisberg test for heteroskedasticity. The null hypothesis was that our regressors have constant variance (homoscedasticity), while the alternative hypothesis stated otherwise. The result (chi-square 7.06 and P-value 0.008) reveals that the null hypothesis should be rejected. In view of that, we estimated our model using the robust standard errors to correct for any issues of heteroskedasticity.
Table below presents the result of the multiple linear regression. The p-value of the F-statistic shows that the model is jointly significant at 1%, 5% and 10% significance levels. We present two models where the first column examines the intensity of adaptation strategies, as well as factors that influence adaptation strategies. The second column examines the individual climate variability adaptation strategies. We first discuss the results of the individual adaptation strategies in the second column. Overall, five climate variability adaptation strategies remained statistically significant: agricultural diversification, agroforestry, drought-resistant varieties, appropriate tillage methods, and irrigation. Agricultural diversification, tillage methods are statistically significant at 5% significance level. While agroforestry, drought-resistant varieties, and irrigation are statistically significant at 5% significance level. We take turns to discuss the adaptation strategies in the ensuing paragraphs. Our results show that adopting climate variability adaptation strategies such as agricultural diversification, appropriate tillage methods, and irrigation positively influences maize yield. This means that smallholders use of these adaptation strategies positively affects maize yields. On the other hand, agroforestry practices and planting drought-resistant varieties as a climate variability adaptation strategy had an inverse relationship on the maize yield obtained in the study area. Other climate variability adaptation strategies (fertilizer application, shifting cultivation, pests and disease control etc.) were not statistically significant, implying that the adoption of such climate variability adaptation strategies does not significantly affect maize yield in the Cape Coast Metropolitan Assembly, holding all other things equal.
Table 3. Summary statistics of continuous variables
Agricultural diversification can be defined as increasing the portfolio of crops that a farmer cultivates, thus a shift from monoculture, towards multiple crops and livestocks with the intention to meet competitive market demands and improving upon farm profits in order to reduce vulnerability from losses from just a single farm enterprise (Asante et al., Citation2018; Bellon et al., Citation2020). Agricultural diversification had a positive relationship with the quantity of maize produced. Agricultural diversification has been widely recommended as a means of avoiding total crop loss in the event of a disaster. Indeed, Dumenu and Obeng (Citation2016) indicated that agricultural diversification constituted a prominent climate variability adaptation strategy employed by smallholder farmers in Ghana. Crop diversification or livestock diversification helps mitigate the shocks of climate variability impacts. Mixed cropping is a common practice among farmers, often farmers cultivate maize, cassava, plantain, cowpeas, and other vegetables in their farming practices. Mixed farming which includes the rearing of livestock and the cultivation of crops (Low et al., Citation2023; Mekuria & Mekonnen, Citation2018). Generally mixed farming provides a good incentive for minimizing climate variability effects on agricultural households. Kosoe and Ahmed (Citation2022) supported the finding that crop diversification improves crop yield among cash crop farmers. Diversification increases income generation and hence leads to improvements in farmers’ livelihood outcomes. Akinnagbe and Irohibe (Citation2014) indicated that crop diversification is insurance against rainfall variability. On the contrary, Kumodzie (Citation2018), found an inverse relationship between crop diversification and rice output in the Hohoe Municipality of Ghana. Our results, however showed that, agricultural diversification is a useful climate variability adaptation strategy that positively influences maize yield. Indeed, Baffour-Ata et al. (Citation2021) recommended agricultural diversification as a useful climate variability adaptation strategy based on their study in Ghana (See Table ).
Agroforestry practice had a negative association with maize yield. This implies that adopting agroforestry as an adaptation strategy had negative returns on maize yield. Intercropping maize with the planting of trees becomes non-beneficial when the tree form canopies, which reduce sunlight intensity required for crop development, and competition for soil nutrients which potentially leads to low crop yields for maize (Gao et al., Citation2013; Raza et al., Citation2020). For instance, tree planting may reduce the space available for maize cultivation and therefore, can decrease maize yield relative to when all the land is dedicated to the cultivation of maize. Food crops tend to perform better when intercrop with other food crops and not trees. For instance, the intercropping of maize and legumes tend to enrich the soil and translate into higher maize yields. In other words, farmers who use their farms mainly to cultivate maize (monocropping) may have the opportunity to experience an increase in maize yield. This is because farm inputs such as fertilizer, disease and pest control are dedicated solely for maize production. Contrary to this assertion, Akinnagbe and Irohibe (Citation2014) reported that agroforestry practices increase organic matter in the soil, which serves as a source of soil nutrients and increases crop productivity. Indeed, there are tree crops that help fix nitrogen in the soil and help improve soil fertility. Tree crops can generally be intercropped with food crops, including maize, when the canopy is not properly formed and closed. This happens typically during the formative years (1–5 years). It is important to note that our study site falls within the coastal area, hence the agroecology does not support tree crop production. Our study provides novel evidence to the already existing evidence on how agroforestry inversely affects maize yields in the global south (See Table ).
Table 4. Multiple linear regression estimation of CVAS on maize yield
Tillage methods positively influence maize yield in the study area. The tillage methods used in the study site are manual labour-intensive methods. This includes weeding with a hoe and cutlass, sowing maize with cutlass in making holes in the soil and covering with the hands or feet. Tillage methods among smallholder farmers often tend to be manual and less sophisticated. The cost involved in hiring tractors to plough, harrow, and till the farmlands appear expensive for smallholder farmers. Fortunately for smallholder farmers in southern Ghana, their farmlands do not require ploughing and harrowing before planting. This contrasts with northern Ghana, where most farmers require ploughing and harrowing of the farmlands before planting given the nature of their lands. Medium and commercial farmers however employ mechanization on their farms. Our result varies with Liu et al. (Citation2020), who reported that no-tillage negatively affects or reduces crop yield. The reason could be attributed to the fact that tillage practices generally improve soil conditions and, as a result, increase crop yield. Tillage improves soil aeration, loosens the soil structure to allow for soil moisture conservation, and improves microbial activities (de Freitas & Landers, Citation2014; Peng et al., Citation2023; Telles et al., Citation2018). Specifically, tillage methods are very useful in the instance of droughts, and high temperatures in trapping water into the soil to be readily made available for plant use. Our study contributes to the climate variability literature on how tillage methods contribute positively to climate variability adaptation strategies.
Table shows that planting drought-resistant varieties does not positively affect maize yield. The study site is not an arid site that requires planting drought-resistant varieties to address challenges associated with droughts. Therefore, smallholder farmers who plant drought-resistant varieties do not get positive returns. This implies that it is not worthwhile to invest money into the purchase and planting of drought-resistant maize varieties. Even though drought stress can lead to yield losses of between 10–25% in drought-prone areas (Fisher et al., Citation2015). Indeed, Fisher et al. (Citation2015) confirmed that planting drought-tolerant maize varieties positively improves yields in Sub-Saharan Africa. Other literature (Ochieng et al., Citation2016, Citation2017; Traore et al., Citation2015) shows a positive effect of planting drought resistant varieties.
Irrigation as a climate variability adaptation strategy proved to inure positively to maize yields in the study area. Very few farmers practice the use of irrigation as an adaptation strategy. The few who do use simple irrigation techniques such as the use of barrels in storing water on-farm and not the use of sophisticated and capital-intensive irrigation techniques. Our finding confirms other findings (Araya et al., Citation2017; Burke & Lobell, Citation2010; Fisher et al., Citation2015; Moradi et al., Citation2013) that indicated that irrigation as a climate variability adaptation strategy increases yields and profit margins, with an additional advantage of allowing flexibility in planting dates and the maize varieties that can be planted. Irrigation is a very common adaptation strategy, mainly in the arid regions of SSA. For instance, irrigation is very common in Burkina Faso where irrigation canals have been constructed since the 1970s (Fisher et al., Citation2015).
Collectively, the estimate in the first column shows that the intensity of climate variability adaptation strategies (CVAS) is significant for the 13 different adaptation strategies (See Table ). This implies that adopting higher adoption climate variability adaptation strategies improves maize yields. The policy implication is to encourage more adaptations of individual climate variability adaptation strategies to improve maize yields in southern Ghana. The result show that the intensity of CVAS is influenced by farming experience, and sex, while the second column confirms the covariates and adds on religion. The number of years that an individual farmer has been engaged in farming gives ample experience for the farmer to make an informed decision about the number of CVAS to adopt. This is because the farmer might have tried and tested some CVAS over time and settled on what works and what does not work. Indeed, the extant literature (Awunyo-Vitor et al., Citation2016; Danso-Abbeam et al., Citation2017; Mensah-Bonsu et al., Citation2017) establishes that farming experience positively influences adoption of maize technologies in Ghana. The sex of a farmer whether male or female influences the number of CVAS to adopt. Indeed, the related studies (Adams et al., Citation2021; Anang et al., Citation2020; Quaye et al., Citation2022) show that the sex of farmers affects the adoption of maize technologies in Ghana. Male farmers are seen to be more risk-loving, while female farmers are risk-averse and hence might be hesitant to adopt more CVAS all together (Love et al., Citation2014; Mbah et al., Citation2023).
3.2. Estimates of treatment effects of climate variability adaptation strategies on maize yield in southern Ghana
3.2.1. Estimates from inverse probability weighting regression adjustment (IPWRA)
Before proceeding to the discussion of the results from the IPWRA estimates, we present the model diagnostics. The overlap plot (Figure ) shows considerable overlap in the density of the propensity score for adopters and non-adopters of the CVAS, even though the result is skewed to the right. Similarly, our Chi-squared overidentification test shows no statistically significant results (p-value of 0.862) for the balancing using 12 covariates included as the control variables. Lastly, the standardized differences and variances approach zero and one, respectively, after the weighting of the data using the IPWRA model.
Table shows the more robust estimate of the adoption of CVAS on maize yields, hence we concentrate primarily on the results generated from the IPWRA. The results obtained from the PWRA partly confirm the results obtained from the multiple regression analysis carried out earlier (See Table ). Table shows that the adoption of irrigation, and early/late planting positively improve maize yield in this study context. This implies that farmers who adjust their planting periods to align with the changing climate patterns are more likely to reduce climate variability and subsequently obtain higher yields. Growing maize in Ghana, is mostly dependent on the onset of the rains. Given that most farmers have small lands and are less sophisticated in their farming systems. The sowing of maize seeds is typically timed to align with the onset of the rains. Therefore, delayed rains imply sowing late, whereas and early rains, imply sowing early.
Table 5. Treatment effects estimation of CVAS on maize yield
Our result supports the finding of Tachie-Obeng et al. (Citation2013), who found that early or delayed planting improved maize yields. The literature (Kassie et al., Citation2015; Shrestha, Citation2014) shows that delayed sowing prevents the crops from drought stress and heat during the early formative stages. Lv et al. (Citation2020) and Saddique et al. (Citation2020) supported the finding that delaying planting dates positively correlated with crop yields. The adjustment of planting date appears well documented in the related literature, but with sparing evidence in the climate variability and maize yields nexus literature. Our findings, therefore, improve understanding on the climate variability adaptation literature in Ghana, particularly for maize which is a staple food crop.
We found out that closely associated with reasons why farmers, plant early or late has to do with the onset of the rains. In improving resilience to climate variability, irrigation appears to be a useful adaptation strategy that positively improves maize yields in Ghana. As discussed earlier under the multiple regression analysis, we observe that few farmers practice irrigation as an adaptation strategy, however, among the few who do, they resort to simple irrigation techniques such as storage of water in barrels, planting in marshy areas, or on riverbanks, harvesting rainwater into containers and using for irrigation in complementing the natural rains. This helps to improve farmers resilience to climate variability. Our finding remains upheld in the literature (Araya et al., Citation2017; Burke & Lobell, Citation2010; Fisher et al., Citation2015; Moradi et al., Citation2013) that shows that irrigation increases yields and farmers profit margins, with a complementary advantage of allowing flexibility in staggering planting dates. Irrigation has been a common adaptation strategy mainly in arid regions, but our findings show that adverse climate variability is opening up irrigation as a climate variability adaptation strategy in non-arid regions (See Table ).
4. Conclusion and policy recommendations
Climate variabilities remain pervasive in the global world. However, farmers in sub-Saharan Africa (SSA) are disproportionately affected. Maize is an essential staple in SSA, and its production is mainly contingent on the onset of natural rainfall, which by default introduces uncertainties in production. The smallholder farmer’s ability to adapt to the adverse effects of climate variability is not in doubt, but the accounts on adaptation strategies that remain effective, has been largely anecdotal. Therefore, this study assessed the effect of adopting climate variability strategies (CVAS) on maize farmers yield in southern Ghana’s Cape Coast Metropolitan Assembly. The findings showed that some climate variability adaptation strategies (irrigation and early or late planting) positively correlated with maize yields. Irrigation positively correlated with maize yields, implying that adopting irrigation increases maize yields. We find that even though irrigation appears well established as a climate variability strategy in arid regions, the use of irrigation in non-arid regions among maize farmers is also gaining traction. Additionally, we found that changes in planting dates (early or late planting) positively affected maize yields implying that sustained agricultural extension and advisory services that target changes in planting dates can go a long way to enhance maize yields in southern Ghana.
Our findings showed that borrowing funds, planting in rows, applying indigenous knowledge, shifting cultivation, agroforestry, and agricultural diversification, among others, do not statistically influence maize yields. The Ghana Irrigation Development Authority (GIDA) under the Ministry of Food and Agriculture (MoFA) is encouraged to institutionalize the informal irrigation schemes practiced by farmers to ensure all year production of maize. Perhaps, this is an opportunity to strengthen the “one village one dam” (1V1D) initiative of the government of Ghana to cover non-arid areas such as southern Ghana to increase maize production. Agricultural extension and advisory services are encouraged to promote and sustain increased interest in the changes to planting dates (early or late planting) as a useful climate variability adaptation strategy in minimizing the adverse effects of climate variability.
Authors Contribution
All the authors were involved in the conceptualization, draft, data analysis, interpretation, and approval of the manuscript.
Availability of data and materials
Research data and materials are available upon request. Competing Interest The authors disclose that they have no known financial interests or personal relationships that could have appeared to influence the work reported in this paper.
Consent for publication
We had approval to anonymize respondents’ information and publish the contents of the research. All authors read and agreed to the published version of the manuscript.
Ethics approval and consent to participate
The authors declare that respondents consented to participate in the interview. The purpose of the research was explained to the respondents before they granted explicit concern to participate in the interview. Approved consent was received from the participants of the study.
Study limitation
The study could have relied on a time series climate data to see how maize yield responds to climate variability, but this was not done due to data limitation. The authors, however, employed a treatment effect estimation model to improve the estimates generated.
Acknowledgments
We acknowledge the smallholder farmers in the Cape Coast Municipal Assembly who provided information for the research. We are grateful to the Municipal Director of Agriculture at the Cape Coast Municipal Assembly and the Agricultural Extension Agents (AEAs) who helped with the data collection.
Disclosure statement
No potential conflict of interest was reported by the author(s).
Additional information
Funding
Notes on contributors
Daniel Ankrah
Daniel Adu Ankrah Daniel is a Senior Lecturer and Head, Department of Agricultural Extension, University of Ghana. Daniel is a Development Sociologist, with research interests that intersect climate variability and change, agricultural food systems, agricultural innovation studies, gender, agricultural extension, and digital financial inclusion.
Charles Okyere
Charles Okyere Charles is a Senior Lecturer and an Agricultural Economists with the Department of Agricultural Economics and Agribusiness, University of Ghana. His research interests cut across climate variability and change, impact evaluations, experimental and quasi-experimental economics, and nutrition.
Jojo Mensah
Jojo Mensah Jojo is an Agricultural Extensionist. He recently completed his Master’s degree in Agricultural Extension from the Department of Agricultural Extension, University of Ghana. He has strong research interests in climate variability and change, food security, agricultural extension and advisory services.
Emmanuel Okata
Emmanuel Okata Emmanuel is a graduate student with the Department of Agricultural and Resource Economics, University of Saskatchewan, Saskatoon, Canada, studying Agricultural Economics. Emmanuel obtained a bachelor’s degree in Agricultural Economics from the University of Ghana. He has research interest in food security, data mining, climate variability and change.
Notes
1. 1US$ to an average exchange rate of GHC 5.9 in 2021.
References
- Acharjee, T. K., van Halsema, G., Ludwig, F., Hellegers, P., & Supit, I. (2019). Shifting planting date of boro rice as a climate change adaptation strategy to reduce water use. Agricultural Systems, 168, 131–23. https://doi.org/10.1016/j.agsy.2018.11.006
- Adams, A., Jumpah, E. T., & Caesar, L. D. (2021). The nexuses between technology adoption and socioeconomic changes among farmers in Ghana. Technological Forecasting and Social Change, 173, 121133. https://doi.org/10.1016/j.techfore.2021.121133
- Adavi, Z., Moradi, R., Saeidnejad, A. H., Tadayon, M. R., & Mansouri, H. (2018). Assessment of potato response to climate change and adaptation strategies. Scientia Horticulturae, 228, 91–102. https://doi.org/10.1016/j.scienta.2017.10.017
- Afranaa Kwapong, N., & Ankrah, D. A. (2023). Understanding innovation process within an interactive social network: Empirical insights from maize innovations in southern Ghana. Cogent Social Sciences, 9(1), 2167390. https://doi.org/10.1080/23311886.2023.2167390
- Aguilera, E., Lassaletta, L., Gattinger, A., & Gimeno, B. S. (2013). Managing soil carbon for climate change mitigation and adaptation in Mediterranean cropping systems: A meta-analysis. Agriculture, Ecosystems & Environment, 168, 25–36. https://doi.org/10.1016/j.agee.2013.02.003
- Aidoo, D. C., Boateng, S. D., Freeman, C. K., & Anaglo, J. N. (2021). The effect of smallholder maize farmers’ perceptions of climate change on their adaptation strategies: The case of two agro-ecological zones in Ghana. Heliyon, 7(11), e08307. https://doi.org/10.1016/j.heliyon.2021.e08307
- Akinnagbe, O. M., & Irohibe, I. J. (2014). Agricultural adaptation strategies to climate change impacts in Africa: A review. Bangladesh Journal of Agricultural Research, 39(3), 407–418. https://doi.org/10.3329/bjar.v39i3.21984
- Anang, B. T., Bäckman, S., & Sipiläinen, T. (2020). Adoption and income effects of agricultural extension in northern Ghana. Scientific African, 7, e00219. https://doi.org/10.1016/j.sciaf.2019.e00219
- Anderson, R., Bayer, P. E., & Edwards, D. (2020). Climate change and the need for agricultural adaptation. Current Opinion in Plant Biology, 56, 197–202. https://doi.org/10.1016/j.pbi.2019.12.006
- Ankrah, D. A., Agyei-Holmes, A., & Boakye, A. A. (2021). Ghana’s rice value chain resilience in the context of COVID-19. Social Sciences & Humanities Open, 4(1), 100210. https://doi.org/10.1016/j.ssaho.2021.100210
- Ankrah, D. A., Anum, R., Anaglo, J. N., & Boateng, S. D. (2023). Influence of sustainable livelihood capital on climate variability adaptation strategies. Environmental and Sustainability Indicators, 18, 100233. https://doi.org/10.1016/j.indic.2023.100233
- Ankrah, D. A., Kwapong, N. A., & Boateng, S. D. (2022). Indigenous knowledge and science-based predictors reliability and its implication for climate adaptation in Ghana. African Journal of Science, Technology, Innovation & Development, 1–13. https://doi.org/10.1080/20421338.2021.1923394
- Ankrah, D. A., Kwapong, N. A., Eghan, D., Adarkwah, F., & Boateng-Gyambiby, D. (2021). Agricultural insurance access and acceptability: Examining the case of smallholder farmers in Ghana. Agriculture & Food Security, 10(1), 1–14. https://doi.org/10.1186/s40066-021-00292-y
- Ankrah, D. A., Kwapong, N. A., Manteaw, S. A., & Agyarko-Fosuhene, F. (2023). Sustainable cereal production: A spatial analytical approach using the Ghana living standards survey. Heliyon, 9(7), e17831. https://doi.org/10.1016/j.heliyon.2023.e17831
- Ankrah, D. A., Mensah, J., Anaglo, J. N., & Boateng, S. D. (2023). Climate variability indicators-scientific data versus farmers perception; evidence from southern Ghana. Cogent Food & Agriculture, 9(1), 2148323. https://doi.org/10.1080/23311932.2022.2148323
- Ankrah, D. A., Okyere, C. Y., Mensah, J., & Anaglo, J. N. (2023). Choice and intensity of climate variability adaptation strategies: Evidence from maize farmers in southern Ghana. Geographical Review, 1–25. https://doi.org/10.1080/00167428.2023.2212390
- Ankrah, D. A., Tsikata, D., & Dzanku, F. M. (2022). Agricultural commercialization and gender mainstreaming in decentralized Ghana: The politics of business. In Agricultural commercialization, gender equality and the right to food (pp. 148–165). Routledge. https://doi.org/10.4324/9781003202004-10
- Antwi-Agyei, P., Fraser, E. D., Dougill, A. J., Stringer, L. C., & Simelton, E. (2012). Mapping the vulnerability of crop production to drought in Ghana using rainfall, yield and socioeconomic data. Applied Geography, 32(2), 324–334. https://doi.org/10.1016/j.apgeog.2011.06.010
- Antwi-Agyei, P., Stringer, L. C., & Dougill, A. J. (2014). Livelihood adaptations to climate variability: Insights from farming households in Ghana. Regional Environmental Change, 14(4), 1615–1626. https://doi.org/10.1007/s10113-014-0597-9
- Antwi, K., & Antwi-Agyei, P. (2023). Intra-gendered perceptions and adoption of climate-smart agriculture: Evidence from smallholder farmers in the Upper East Region of Ghana. Environmental Challenges, 12, 100736. https://doi.org/10.1016/j.envc.2023.100736
- Anum, R., Ankrah, D. A., & Anaglo, J. N. (2022). Influence of demographic characteristics and social network on peri-urban smallholder farmers adaptation strategies-evidence from southern Ghana. Cogent Food & Agriculture, 8(1), 2130969. https://doi.org/10.1080/23311932.2022.2130969
- Araya, A., Kisekka, I., Lin, X., Prasad, P. V., Gowda, P., Rice, C., & Andales, A. (2017). Evaluating the impact of future climate change on irrigated maize production in Kansas. Climate Risk Management, 17, 139–154. https://doi.org/10.1016/j.crm.2017.08.001
- Asante, F., Guodaar, L., & Arimiyaw, S. (2021). Climate change and variability awareness and livelihood adaptive strategies among smallholder farmers in semi-arid northern Ghana. Environmental Development, 39, 100629. https://doi.org/10.1016/j.envdev.2021.100629
- Asante, B. O., Villano, R. A., Patrick, I. W., & Battese, G. E. (2018). Determinants of farm diversification in integrated crop–livestock farming systems in Ghana. Renewable Agriculture and Food Systems, 33(2), 131–149. https://doi.org/10.1017/S1742170516000545
- Asare-Nuamah, P., Botchway, E., & Onumah, J. A. (2019). Helping the helpless: Contribution of rural extension services to smallholder farmers’ climate change adaptive capacity and adaptation in rural Ghana. International Journal of Rural Management, 15(2), 244–268. https://doi.org/10.1177/0973005219876211
- Ashby, J. A., Kristjanson, P. M., Thornton, P. K., Campbell, B. M., Vermeulen, S. J., & Wollenberg, E. K. (2012). CCAFS gender strategy (p. 29). CGIAR Research Program on Climate Change, Agriculture and Food Security (CCAFS).
- Asrat, P., & Simane, B. (2018). Farmers’ perception of climate change and adaptation strategies in the Dabus watershed, North-West Ethiopia. Ecological Processes, 7(1), 1–13. https://doi.org/10.1186/s13717-018-0118-8
- Atiah, W. A., Amekudzi, L. K., Akum, R. A., Quansah, E., Antwi‐Agyei, P., & Danuor, S. K. (2022). Climate variability and impacts on maize (Zea mays) yield in Ghana, West Africa. Quarterly Journal of the Royal Meteorological Society, 148(742), 185–198. https://doi.org/10.1002/qj.4199
- Atinkut, B., & Mebrat, A. (2016). Determinants of farmers choice of adaptation to climate variability in Dera woreda, south Gondar zone, Ethiopia. Environmental Systems Research, 5(1), 1–8. https://doi.org/10.1186/s40068-015-0046-x
- Awunyo-Vitor, D., Wongnaa, C. A., & Aidoo, R. (2016). Resource use efficiency among maize farmers in Ghana. Agriculture & Food Security, 5(1), 1–10. https://doi.org/10.1186/s40066-016-0076-2
- Ayanlade, A., Radeny, M., & Morton, J. F. (2017). Comparing smallholder farmers’ perception of climate change with meteorological data: A case study from southwestern Nigeria. Weather and Climate Extremes, 15, 24–33. https://doi.org/10.1016/j.wace.2016.12.001
- Babbie, E. R. (2020). The practice of social research. Cengage learning.
- Baffour-Ata, F., Antwi-Agyei, P., Nkiaka, E., Dougill, A. J., Anning, A. K., & Kwakye, S. O. (2021). Effect of climate variability on yields of selected staple food crops in northern Ghana. Journal of Agriculture and Food Research, 6, 100205. https://doi.org/10.1016/j.jafr.2021.100205
- Baffour-Ata, F., Tabi, J. S., Sangber-Dery, A., Etu-Mantey, E. E., & Asamoah, D. K. (2023). Effect of rainfall and temperature variability on maize yield in the Asante Akim North District, Ghana. Current Research in Environmental Sustainability, 5, 100222. https://doi.org/10.1016/j.crsust.2023.100222
- Bajguz, A., & Hayat, S. (2009). Effects of brassinosteroids on the plant responses to environmental stresses. Plant Physiology and Biochemistry, 47(1), 1–8. https://doi.org/10.1016/j.plaphy.2008.10.002
- Bawayelaazaa Nyuor, A., Donkor, E., Aidoo, R., Saaka Buah, S., Naab, J. B., Nutsugah, S. K., Bayala, J., & Zougmoré, R. (2016). Economic impacts of climate change on cereal production: Implications for sustainable agriculture in Northern Ghana. Sustainability, 8(8), 724. https://doi.org/10.3390/su8080724
- Bellon, M. R., Kotu, B. H., Azzarri, C., & Caracciolo, F. (2020). To diversify or not to diversify, that is the question. Pursuing agricultural development for smallholder farmers in marginal areas of Ghana. World Development, 125, 104682. https://doi.org/10.1016/j.worlddev.2019.104682
- Burke, M., & Lobell, D. (2010). Food security and adaptation to climate change: What do we know? Climate Change and Food Security: Adapting Agriculture to a Warmer World, 133–153. http://link.springer.com/book/10.1007%2F978-90-481-2953-9
- Campbell, B. M., Vermeulen, S. J., Aggarwal, P. K., Corner-Dolloff, C., Girvetz, E., Loboguerrero, A. M., Ramirez-Villegas, J., Rosenstock, T., Sebastian, L., & Thornton, P. K. (2016). Reducing risks to food security from climate change. Global Food Security, 11, 34–43. https://doi.org/10.1016/j.gfs.2016.06.002
- Cattaneo, M. D. (2010). Efficient semiparametric estimation of multi-valued treatment effects under ignorability. Journal of Econometrics, 155(2), 138–154. https://doi.org/10.1016/j.jeconom.2009.09.023
- Choudhury, B. U., Nengzouzam, G., & Islam, A. (2022). Runoff and soil erosion in the integrated farming systems based on micro-watersheds under projected climate change scenarios and adaptation strategies in the eastern Himalayan mountain ecosystem (India). Journal of Environmental Management, 309, 114667. https://doi.org/10.1016/j.jenvman.2022.114667
- Christensen, J. H., Hewitson, B., Busuioc, A., Chen, A., Gao, X., Held, R., Jones, R., Kolli, R. K., Kwon, W., & Laprise, R. (2007). Regional climate projections: Climate change: The physical science basis. Contribution of Working group I to the Fourth Assessment Report of the IPCC.
- Cohen, L., Manion, L., & Morrison, K. (2017). Validity and reliability. In Research methods in education (pp. 245–284). Routledge. https://doi.org/10.4324/9781315456539-14
- Conway, D., & Schipper, E. L. F. (2011). Adaptation to climate change in Africa: Challenges and opportunities identified from Ethiopia. Global Environmental Change, 21(1), 227–237. https://doi.org/10.1016/j.gloenvcha.2010.07.013
- Creswell, J. W., & Creswell, J. D. (2017). Research design: Qualitative, quantitative, and mixed methods approaches. Sage publications.
- Cudjoe, G. P., Antwi-Agyei, P., & Gyampoh, B. A. (2021). The effect of climate variability on maize production in the Ejura-Sekyedumase Municipality, Ghana. Climate, 9(10), 145. https://doi.org/10.3390/cli9100145
- Danso-Abbeam, G., Bosiako, J. A., Ehiakpor, D. S., Mabe, F. N., & Aye, G. (2017). Adoption of improved maize variety among farm households in the northern region of Ghana. Cogent Economics & Finance, 5(1), 1416896. https://doi.org/10.1080/23322039.2017.1416896
- Deb, P., Shrestha, S., & Babel, M. S. (2015). Forecasting climate change impacts and evaluation of adaptation options for maize cropping in the hilly terrain of Himalayas: Sikkim, India. Theoretical and Applied Climatology, 121(3–4), 649–667. https://doi.org/10.1007/s00704-014-1262-4
- de Freitas, P. L., & Landers, J. N. (2014). The transformation of agriculture in Brazil through development and adoption of zero tillage conservation agriculture. International Soil & Water Conservation Research, 2(1), 35–46. https://doi.org/10.1016/S2095-6339(15)30012-5
- Delgado, J. A., Mosquera, V. H. B., Alwang, J. R., Villacis-Aveiga, A., Ayala, Y. E. C., Neer, D., Monar, C., & López, L. O. E. (2021). Potential use of cover crops for soil and water conservation, nutrient management, and climate change adaptation across the tropics. Advances in Agronomy, 165, 175–247. https://doi.org/10.1016/bs.agron.2020.09.003
- Demeke, A. B., Keil, A., & Zeller, M. (2011). Using panel data to estimate the effect of rainfall shocks on smallholders food security and vulnerability in rural Ethiopia. Climatic Change, 108(1), 185–206. https://doi.org/10.1007/s10584-010-9994-3
- Denkyirah, E. K., Okoffo, E. D., Adu, D. T., Aziz, A. A., Ofori, A., & Denkyirah, E. K. (2016). Modeling Ghanaian cocoa farmers’ decision to use pesticide and frequency of application: The case of Brong Ahafo Region. SpringerPlus, 5(1), 1–17. https://doi.org/10.1186/s40064-016-2779-z
- Denkyirah, E. K., Okoffo, E. D., Adu, D. T., Bosompem, O. A., & Yildiz, F. (2017). What are the drivers of cocoa farmers’ choice of climate change adaptation strategies in Ghana? Cogent Food & Agriculture, 3(1), 1334296. https://doi.org/10.1080/23311932.2017.1334296
- Derkyi, M., Adiku, S. G., Nelson, V., Dovie, B. D., Codjoe, S., & Awuah, E. (2018). Smallholder farmers’ perception of climatic and socio-economic factors influencing livelihoods in the transition zone of Ghana. AAS Open Research, 1, 7. https://doi.org/10.12688/aasopenres.12839.1
- Diallo, A., Donkor, E., & Owusu, V. (2020). Climate change adaptation strategies, productivity and sustainable food security in southern Mali. Climatic Change, 159(3), 309–327. https://doi.org/10.1007/s10584-020-02684-8
- Drukker, D. M. (2016). Estimating treatment effects from observational data using teffects, stteffects, and eteffects. UK Stata Users Group meeting, https://fmwww.bc.edu/repec/usug2016/drukker_uksug16.pdf
- Dumenu, W. K., & Obeng, E. A. (2016). Climate change and rural communities in Ghana: Social vulnerability, impacts, adaptations and policy implications. Environmental Science & Policy, 55, 208–217. https://doi.org/10.1016/j.envsci.2015.10.010
- Duncan, J. M., Dash, J., & Atkinson, P. M. (2015). Elucidating the impact of temperature variability and extremes on cereal croplands through remote sensing. Global Change Biology, 21(4), 1541–1551. https://doi.org/10.1111/gcb.12660
- Dzanku, F. M., & Tsikata, D. (2022). Implications of socioeconomic change for agrarian land and labour relations in rural Ghana. Journal of Rural Studies, 94, 385–398. https://doi.org/10.1016/j.jrurstud.2022.07.010
- Enimu, S., & Onome, G. (2018). Determinants of climate change adaptation strategies among farm households in Delta State, Nigeria. Curr Inves Agri Curr Res, 5(3). CIACR. MS. ID. 000213615. In (Vol. 8, pp. 61-68): UPINE PUBLISHERS Open Access L Current Investigations in Agriculture and …. https://doi.org/10.32474/CIACR.2018.05.000213.
- Epule, T. E., Ford, J. D., Lwasa, S., Nabaasa, B., & Buyinza, A. (2018). The determinants of crop yields in Uganda: What is the role of climatic and non-climatic factors? Agriculture & Food Security, 7(1), 1–17. https://doi.org/10.1186/s40066-018-0159-3
- Fagariba, C. J., Song, S., & Soule Baoro, S. K. G. (2018). Climate change adaptation strategies and constraints in northern Ghana: Evidence of farmers in Sissala West District. Sustainability, 10(5), 1484. https://doi.org/10.3390/su10051484
- Fisher, M., Abate, T., Lunduka, R. W., Asnake, W., Alemayehu, Y., & Madulu, R. B. (2015). Drought tolerant maize for farmer adaptation to drought in sub-Saharan Africa: Determinants of adoption in Eastern and southern Africa. Climatic Change, 133(2), 283–299. https://doi.org/10.1007/s10584-015-1459-2
- Gao, L., Xu, H., Bi, H., Xi, W., Bao, B., Wang, X., Bi, C., Chang, Y., & Niedz, R. P. (2013). Intercropping competition between apple trees and crops in agroforestry systems on the Loess Plateau of China. PloS One, 8(7), e70739. https://doi.org/10.1371/journal.pone.0070739
- Gebru, G. W., Ichoku, H. E., & Phil-Eze, P. O. (2020). Determinants of smallholder farmers’ adoption of adaptation strategies to climate change in Eastern Tigray National Regional State of Ethiopia. Heliyon, 6(7), e04356. https://doi.org/10.1016/j.heliyon.2020.e04356
- Getachew, F., Bayabil, H. K., Hoogenboom, G., Teshome, F. T., & Zewdu, E. (2021). Irrigation and shifting planting date as climate change adaptation strategies for sorghum. Agricultural Water Management, 255, 106988. https://doi.org/10.1016/j.agwat.2021.106988
- Ghana Statistical Service. (2010). 2010 population and housing census.
- Ghana Statistical Service. (2020). Ghana Census of Agriculture-National Report. G. S. Service. https://ejournal.poltektegal.ac.id/index.php/siklus/article/view/298%0A
- Guodaar, L. (2015). Effects of climate variability on tomato crop production in the Offinso North District of Ashanti region. Kwame Nkrumah University of Science and Technology].
- Holzkämper, A., Klein, T., Seppelt, R., & Fuhrer, J. (2015). Assessing the propagation of uncertainties in multi-objective optimization for agro-ecosystem adaptation to climate change. Environmental Modelling & Software, 66, 27–35. https://doi.org/10.1016/j.envsoft.2014.12.012
- Hosen, N., Nakamura, H., & Hamzah, A. (2020). Adaptation to climate change: Does traditional ecological knowledge hold the key? Sustainability, 12(2), 676. https://doi.org/10.3390/su12020676
- IPCC. (2018). Global warming. Nature, 291(5813). https://doi.org/10.1038/291285a0
- Islam, S., Cenacchi, N., Sulser, T. B., Gbegbelegbe, S., Hareau, G., Kleinwechter, U., Mason D’Croz, D., Nedumaran, S., Robertson, R., Robinson, S., & Wiebe, K. (2016). Structural approaches to modeling the impact of climate change and adaptation technologies on crop yields and food security. Global Food Security, 10, 63–70. https://doi.org/10.1016/j.gfs.2016.08.003
- Kabo-Bah, A. T., Diji, C., Nokoe, K., Mulugetta, Y., Obeng-Ofori, D., & Akpoti, K. (2016). Multiyear rainfall and temperature trends in the Volta river basin and their potential impact on hydropower generation in Ghana. Climate, 4(4), 49. https://doi.org/10.3390/cli4040049
- Kassie, B. T., Asseng, S., Rotter, R. P., Hengsdijk, H., Ruane, A. C., & Van Ittersum, M. K. (2015). Exploring climate change impacts and adaptation options for maize production in the Central Rift Valley of Ethiopia using different climate change scenarios and crop models. Climatic Change, 129(1), 145–158. https://doi.org/10.1007/s10584-014-1322-x
- Kato, E., Ringler, C., Yesuf, M., & Bryan, E. (2011). Soil and water conservation technologies: A buffer against production risk in the face of climate change? Insights from the Nile basin in Ethiopia. Agricultural Economics, 42(5), 593–604. https://doi.org/10.1111/j.1574-0862.2011.00539.x
- Kgosikoma, K. R., Lekota, P. C., & Kgosikoma, O. E. (2018). Agro-pastoralists’ determinants of adaptation to climate change. International Journal of Climate Change Strategies and Management, 10(3), 488–500. https://doi.org/10.1108/IJCCSM-02-2017-0039
- Kikoyo, D. A., & Nobert, J. (2016). Assessment of impact of climate change and adaptation strategies on maize production in Uganda. Physics and Chemistry of the Earth, Parts A/B/C, 93, 37–45. https://doi.org/10.1016/j.pce.2015.09.005
- Kosoe, E. A., & Ahmed, A. (2022). Climate change adaptation strategies of cocoa farmers in the Wassa East District: Implications for climate services in Ghana. Climate Services, 26, 100289. https://doi.org/10.1016/j.cliser.2022.100289
- Kumodzie, E. D. (2018). Effects of climate change adaptation strategies on production efficiency of smallholder rice farmers in the Hohoe Municipality. University of Ghana].
- Kwapong, N. A., Ankrah, D. A., Anaglo, J. N., & Vukey, E. Y. (2021). Determinants of scale of farm operation in the eastern region of Ghana. Agriculture & Food Security, 10(1), 1–11. https://doi.org/10.1186/s40066-021-00309-6
- Leavy, P. (2017). Research design: Quantitative, qualitative, mixed methods, arts-based, and community-based participatory research approaches. Guilford Publications.
- Le Dang, H., Li, E., Bruwer, J., & Nuberg, I. (2014). Farmers’ perceptions of climate variability and barriers to adaptation: Lessons learned from an exploratory study in Vietnam. Mitigation and Adaptation Strategies for Global Change, 19, 531–548. https://doi.org/10.1007/s11027-012-9447-6
- Limantol, A. M., Keith, B. E., Azabre, B. A., & Lennartz, B. (2016). Farmers’ perception and adaptation practice to climate variability and change: A case study of the Vea catchment in Ghana. SpringerPlus, 5(1), 1–38. https://doi.org/10.1186/s40064-016-2433-9
- Liu, L., Basso, B., & Wang, X. (2020). Impacts of climate variability and adaptation strategies on crop yields and soil organic carbon in the US Midwest. PloS One, 15(1), e0225433. https://doi.org/10.1371/journal.pone.0225433
- Lobell, D. B., & Gourdji, S. M. (2012). The influence of climate change on global crop productivity. Plant Physiology, 160(4), 1686–1697. https://doi.org/10.1104/pp.112.208298
- Lombardozzi, D. L., Bonan, G. B., Smith, N. G., Dukes, J. S., & Fisher, R. A. (2015). Temperature acclimation of photosynthesis and respiration: A key uncertainty in the carbon cycle‐climate feedback. Geophysical Research Letters, 42(20), 8624–8631. https://doi.org/10.1002/2015GL065934
- Love, A., Magnan, N., & Colson, G. J. (2014). Male and female risk preferences and maize technology adoption in Kenya.
- Low, G., Dalhaus, T., & Meuwissen, M. P. (2023). Mixed farming and agroforestry systems: A systematic review on value chain implications. Agricultural Systems, 206, 103606. https://doi.org/10.1016/j.agsy.2023.103606
- Lv, Z., Li, F., & Lu, G. (2020). Adjusting sowing date and cultivar shift improve maize adaption to climate change in China. Mitigation and Adaptation Strategies for Global Change, 25(1), 87–106. https://doi.org/10.1007/s11027-019-09861-w
- Makondo, C. C., & Thomas, D. S. (2018). Climate change adaptation: Linking indigenous knowledge with Western science for effective adaptation. Environmental Science & Policy, 88, 83–91. https://doi.org/10.1016/j.envsci.2018.06.014
- Manda, J., Gardebroek, C., Kuntashula, E., & Alene, A. D. (2018). Impact of improved maize varieties on food security in Eastern Zambia: A doubly robust analysis. Review of Development Economics, 22(4), 1709–1728. https://doi.org/10.1111/rode.12516
- Marie, M., Yirga, F., Haile, M., & Tquabo, F. (2020). Farmers’ choices and factors affecting adoption of climate change adaptation strategies: Evidence from northwestern Ethiopia. Heliyon, 6(4), e03867. https://doi.org/10.1016/j.heliyon.2020.e03867
- Martey, E., & Kuwornu, J. K. (2021). Perceptions of climate variability and soil fertility management choices among smallholder farmers in northern Ghana. Ecological Economics, 180, 106870. https://doi.org/10.1016/j.ecolecon.2020.106870
- Mbah, L. T., Molua, E. L., Bomdzele, E., & Egwu, B. M. (2023). Farmers’ response to maize production risks in Cameroon: An application of the criticality risk matrix model. Heliyon, 9(4), e15124. https://doi.org/10.1016/j.heliyon.2023.e15124
- Mekuria, W., & Mekonnen, K. (2018). Determinants of crop–livestock diversification in the mixed farming systems: Evidence from central highlands of Ethiopia. Agriculture & Food Security, 7(1), 1–15. https://doi.org/10.1186/s40066-018-0212-2
- Mensah-Bonsu, A., Sarpong, D. B., Al-Hassan, R., Asuming-Brempong, S., Egyir, I. S., Kuwornu, J. K., & Osei-Asare, Y. B. (2017). Intensity of and factors affecting land and water management practices among smallholder maize farmers in Ghana. African Journal of Agricultural and Resource Economics, 12(311–2017–735), 142–157. https://doi.org/10.22004/ag.econ.258607
- Moradi, R., Koocheki, A., Nassiri Mahallati, M., & Mansoori, H. (2013). Adaptation strategies for maize cultivation under climate change in Iran: Irrigation and planting date management. Mitigation and Adaptation Strategies for Global Change, 18(2), 265–284. https://doi.org/10.1007/s11027-012-9410-6
- Mukherjee, M., & Schwabe, K. (2015). Irrigated agricultural adaptation to water and climate variability: The economic value of a water portfolio. American Journal of Agricultural Economics, 97(3), 809–832. https://doi.org/10.1093/ajae/aau101
- Mulwa, C., Marenya, P., & Kassie, M. (2017). Response to climate risks among smallholder farmers in Malawi: A multivariate probit assessment of the role of information, household demographics, and farm characteristics. Climate Risk Management, 16, 208–221. https://doi.org/10.1016/j.crm.2017.01.002
- Mwinkom, F. X., Damnyag, L., Abugre, S., & Alhassan, S. I. (2021). Factors influencing climate change adaptation strategies in North-Western Ghana: Evidence of farmers in the Black Volta basin in Upper west region. SN Applied Sciences, 3(5), 1–20. https://doi.org/10.1007/s42452-021-04503-w
- Ndamani, F., & Watanabe, T. (2014). Rainfall variability and crop production in northern Ghana: The case of Lawra District.
- Nendel, C., Kersebaum, K. C., Mirschel, W., & Wenkel, K.-O. (2014). Testing farm management options as climate change adaptation strategies using the MONICA model. The European Journal of Agronomy, 52, 47–56. https://doi.org/10.1016/j.eja.2012.09.005
- Nkrumah, F., Klutse, N. A. B., Adukpo, D. C., Owusu, K., & Quagraine, K. A. (2014). Rainfall variability over Ghana: Model versus rain gauge observation. http://hdl.handle.net/123456789/6277
- Ochieng, J., Kirimi, L., & Makau, J. (2017). Adapting to climate variability and change in rural Kenya: Farmer perceptions, strategies and climate trends. Natural Resources Forum, 41(4), 195–208. https://doi.org/10.1111/1477-8947.12111
- Ochieng, J., Kirimi, L., & Mathenge, M. (2016). Effects of climate variability and change on agricultural production: The case of small scale farmers in Kenya. NJAS-Wageningen Journal of Life Sciences, 77(1), 71–78. https://doi.org/10.1016/j.njas.2016.03.005
- Ogisi, O. D., & Begho, T. (2023). Adoption of climate-smart agricultural practices in sub-Saharan Africa: A review of the progress, barriers, gender differences and recommendations. Farming System, 1(2), 100019. https://doi.org/10.1016/j.farsys.2023.100019
- Ojo, T., & Baiyegunhi, L. (2020). Determinants of climate change adaptation strategies and its impact on the net farm income of rice farmers in south-west Nigeria. Land Use Policy, 95, 103946. https://doi.org/10.1016/j.landusepol.2019.04.007
- Okyere, C. Y., Ankrah, D. A., & Ahene‐Codjoe, A. A. (2022). Intra-household health effects of irrigation in southern Ghana. Irrigation and Drainage, 71(1), 268–285. https://doi.org/10.1002/ird.2630
- Peng, Q., Liu, B., Hu, Y., Wang, A., Guo, Q., Yin, B., Cao, Q., & He, L. (2023). The role of conventional tillage in agricultural soil erosion. Agriculture, Ecosystems & Environment, 348, 108407. https://doi.org/10.1016/j.agee.2023.108407
- Porter, J. R., Challinor, A. J., Henriksen, C. B., Howden, S. M., Martre, P., & Smith, P. (2019). Invited review: Intergovernmental panel on climate change, agriculture, and food—A case of shifting cultivation and history. Global Change Biology, 25(8), 2518–2529. https://doi.org/10.1111/gcb.14700
- Powlson, D. S., Stirling, C. M., Jat, M. L., Gerard, B. G., Palm, C. A., Sanchez, P. A., & Cassman, K. G. (2014). Limited potential of no-till agriculture for climate change mitigation. Nature Climate Change, 4(8), 678–683. https://doi.org/10.1038/nclimate2292
- Quaye, W., Onumah, J. A., Boimah, M., & Mohammed, A. (2022). Gender dimension of technology adoption: The case of technologies transferred in Ghana. Development in Practice, 32(4), 434–447. https://doi.org/10.1080/09614524.2021.2000588
- Quisumbing, A. R., Meinzen-Dick, R., Raney, T. L., Croppenstedt, A., Behrman, J. A., & Peterman, A. (2014). Gender in agriculture. Springer, 102072, 444. https://doi.org/10.1007/978-94-017-8616-4
- Rao, A. S., Chandran, M. S., Bal, S. K., Pramod, V., Sandeep, V., Manikandan, N., Raju, B., Prabhakar, M., Islam, A., & Kumar, S. N. (2022). Evaluating area-specific adaptation strategies in rainfed maize under future climates in India. Science of the Total Environment, 836, 155511. https://doi.org/10.1016/j.scitotenv.2022.155511
- Raza, M. A., Feng, L. Y., van der Werf, W., Iqbal, N., Khan, I., Khan, A., Din, A. M. U., Naeem, M., Meraj, T. A., & Hassan, M. J. (2020). Optimum strip width increases dry matter, nutrient accumulation, and seed yield of intercrops under the relay intercropping system. Food and Energy Security, 9(2), e199. https://doi.org/10.1002/fes3.199
- Roco, L., Engler, A., Bravo-Ureta, B. E., & Jara-Rojas, R. (2015). Farmers’ perception of climate change in Mediterranean Chile. Regional Environmental Change, 15(5), 867–879. https://doi.org/10.1007/s10113-014-0669-x
- Ruane, A. C., Cecil, L. D., Horton, R. M., Gordón, R., McCollum, R., Brown, D., Killough, B., Goldberg, R., Greeley, A. P., & Rosenzweig, C. (2013). Climate change impact uncertainties for maize in Panama: Farm information, climate projections, and yield sensitivities. Agricultural and Forest Meteorology, 170, 132–145. https://doi.org/10.1016/j.agrformet.2011.10.015
- Saddique, Q., Cai, H., Xu, J., Ajaz, A., He, J., Yu, Q., Wang, Y., Chen, H., Khan, M. I., & Liu, D. L. (2020). Analyzing adaptation strategies for maize production under future climate change in Guanzhong Plain, China. Mitigation and Adaptation Strategies for Global Change, 25(8), 1523–1543. https://doi.org/10.1007/s11027-020-09935-0
- Sadiq, M. A., Kuwornu, J. K., Al-Hassan, R. M., & Alhassan, S. I. (2019). Assessing maize farmers’ adaptation strategies to climate change and variability in Ghana. Agriculture, 9(5), 90. https://doi.org/10.3390/agriculture9050090
- Shrestha, S. (2014). Adaptation strategies for rice cultivation under climate change in Central Vietnam. In Climate change impacts and adaptation in water resources and water use sectors (pp. 93–119). Springer. https://doi.org/10.1007/978-3-319-09746-6_6
- Singh, P., Nedumaran, S., Ntare, B., Boote, K. J., Singh, N. P., Srinivas, K., & Bantilan, M. (2014). Potential benefits of drought and heat tolerance in groundnut for adaptation to climate change in India and west Africa. Mitigation and Adaptation Strategies for Global Change, 19(5), 509–529. https://doi.org/10.1007/s11027-012-9446-7
- Srivastava, A. K., Mboh, C. M., Zhao, G., Gaiser, T., & Ewert, F. (2018). Climate change impact under alternate realizations of climate scenarios on maize yield and biomass in Ghana. Agricultural Systems, 159, 157–174. https://doi.org/10.1016/j.agsy.2017.03.011
- Tachie-Obeng, E., Akponikpe, P., & Adiku, S. (2013). Considering effective adaptation options to impacts of climate change for maize production in Ghana. Environmental Development, 5, 131–145. https://doi.org/10.1016/j.envdev.2012.11.008
- Tambo, J. A. (2016). Adaptation and resilience to climate change and variability in north-east Ghana. International Journal of Disaster Risk Reduction, 17, 85–94. https://doi.org/10.1016/j.ijdrr.2016.04.005
- Tambo, J. A., & Mockshell, J. (2018). Differential impacts of conservation agriculture technology options on household income in sub-Saharan Africa. Ecological Economics, 151, 95–105. https://doi.org/10.1016/j.ecolecon.2018.05.005
- Teixeira, E. I., de Ruiter, J., Ausseil, A.-G., Daigneault, A., Johnstone, P., Holmes, A., Tait, A., & Ewert, F. (2018). Adapting crop rotations to climate change in regional impact modelling assessments. Science of the Total Environment, 616, 785–795. https://doi.org/10.1016/j.scitotenv.2017.10.247
- Telles, T. S., Reydon, B. P., & Maia, A. G. (2018). Effects of no-tillage on agricultural land values in Brazil. Land Use Policy, 76, 124–129. https://doi.org/10.1016/j.landusepol.2018.04.053
- Thinda, K., Ogundeji, A., Belle, J., & Ojo, T. (2020). Understanding the adoption of climate change adaptation strategies among smallholder farmers: Evidence from land reform beneficiaries in South Africa. Land Use Policy, 99, 104858. https://doi.org/10.1016/j.landusepol.2020.104858
- Tingem, M., & Rivington, M. (2009). Adaptation for crop agriculture to climate change in Cameroon: Turning on the heat. Mitigation and Adaptation Strategies for Global Change, 14(2), 153–168. https://doi.org/10.1007/s11027-008-9156-3
- Tofa, A. I., Kamara, A. Y., Babaji, B. A., Akinseye, F. M., & Bebeley, J. F. (2021). Assessing the use of a drought-tolerant variety as adaptation strategy for maize production under climate change in the savannas of Nigeria. Scientific Reports, 11(1), 8983. https://doi.org/10.1038/s41598-021-88277-6
- Traore, B., Van Wijk, M. T., Descheemaeker, K., Corbeels, M., Rufino, M. C., & Giller, K. E. (2015). Climate variability and change in southern Mali: Learning from farmer perceptions and on-farm trials. Experimental Agriculture, 51(4), 615–634. https://doi.org/10.1017/S0014479714000507
- Travasso, M. I., Magrin, G. O., Baethgen, W. E., Castaño, J. P., Rodriguez, G. R., Pires, J. L., Gimenez, A., Cunha, G., & Fernandes, M. (2006). Adaptation measures for maize and soybean in south eastern South America. AIACC.
- Tripathi, A., Tripathi, D. K., Chauhan, D., Kumar, N., & Singh, G. (2016). Paradigms of climate change impacts on some major food sources of the world: A review on current knowledge and future prospects. Agriculture, Ecosystems & Environment, 216, 356–373. https://doi.org/10.1016/j.agee.2015.09.034
- Tufa, A. H., Alene, A. D., Cole, S. M., Manda, J., Feleke, S., Abdoulaye, T., Chikoye, D., & Manyong, V. (2022). Gender differences in technology adoption and agricultural productivity: Evidence from Malawi. World Development, 159, 106027. https://doi.org/10.1016/j.worlddev.2022.106027
- Upadhaya, K., Barik, S., Kharbhih, V. M., Nongbri, G., Debnath, G., Gupta, A., & Ojha, A. (2020). Traditional bun shifting cultivation practice in Meghalaya, Northeast India. Energy, Ecology and Environment, 5(1), 34–46. https://doi.org/10.1007/s40974-019-00144-3
- Vicuna, S., Alvarez, P., Melo, O., Dale, L., & Meza, F. (2014). Irrigation infrastructure development in the Limarí basin in Central Chile: Implications for adaptation to climate variability and climate change. Water International, 39(5), 620–634. https://doi.org/10.1080/02508060.2014.945068
- Wooldridge, J. M. (2010). Econometric analysis of cross section and panel data. MIT press.
- Wossen, T., Berger, T., Swamikannu, N., & Ramilan, T. (2014). Climate variability, consumption risk and poverty in semi-arid northern Ghana: Adaptation options for poor farm households. Environmental Development, 12, 2–15. https://doi.org/10.1016/j.envdev.2014.07.003
- Woznicki, S. A., Nejadhashemi, A. P., & Parsinejad, M. (2015). Climate change and irrigation demand: Uncertainty and adaptation. Journal of Hydrology: Regional Studies, 3, 247–264. https://doi.org/10.1016/j.ejrh.2014.12.003
- Yamane, T. (1967). Elementary sampling theory (1th ed.). Prentice Hall Inc.
- Yaro, J. A. (2013). The perception of and adaptation to climate variability/change in Ghana by small-scale and commercial farmers. Regional Environmental Change, 13(6), 1259–1272. https://doi.org/10.1007/s10113-013-0443-5
- Yaro, J. A., Teye, J., & Bawakyillenuo, S. (2015). Local institutions and adaptive capacity to climate change/variability in the Northern Savannah of Ghana. Climate and Development, 7(3), 235–245. https://doi.org/10.1080/17565529.2014.951018