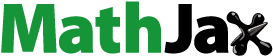
Abstract
Studying the inventory management literature regarding the models with controllable lead time, many researchers have assumed the random demand follows the normal distribution. However, in practice, it is observed that an accurate demand distribution is often skewed to the right for many items and fitting the normal distribution to the random demand may cause a great financial loss for an inventory/production system. Hence, the motivation of this study is to design a mathematical model where the demand follows the log-normal distribution. Also in order to expand upon previous research concerning the random available capacity, we assume that the random capacity follows a gamma-type distribution to cover a wide range of distribution shapes. Moreover, we consider the ordering cost is a deterministic variable and it is reduced by an extra investment. Also, to find an optimal policy of the proposed probabilistic mathematical model, a solution algorithm is established and a numerical example is proposed showing that utilizing the proposed model rather than the standard continuous-review model with the normal demand may reduce the total expected cost more than 20%.
PUBLIC INTEREST STATEMENT
In order to help inventory managers to make correct and accurate decisions for their systems in stochastic environments, a continuous-review mathematical inventory model is proposed for simulating an inventory system where uncertain demand and available capacity patterns are the right-skewed types. It is also considered that the lead time and ordering cost are the controllable variables. The results validate that savings due to utilizing the proposed method might be more than 20% of using standard continuous-review inventory model wherein the demand follows the normal distribution.
1. Introduction
There has been growing interest in utilizing the probabilistic continuous-review (Q,r) inventory model with various assumptions and limitations for simulating real systems from researchers in the area of inventory control and management (Lee & Nahmias, Citation1993; Hariga, Citation2010). One of the significant assumptions for the model is about the distributional information of the lead time demand. Regarding this issue, Hadley and Whithin (Citation1963) proposed an algorithmic procedure for the normally distributed lead time demand. Bagchi and Hayya (Citation1984) developed an inventory problem with stochastic lead time demand where the distribution of stochastic lead time is the Erlang and the random demand is normally-distributed. Mohebbi and Posner (Citation1998) studied an inventory model in which the demand follows the Poisson distribution. They also assumed that the lead time follows the hyperexponential and the Erlang distributions. Tadikamalla (Citation1978) showed that the approximation of the Weibull distribution to the lead time demand when the true distribution of the demand is right skewed can be justifiable. Burgin (Citation1975) proposed an inventory model in which the lead time demand follows the gamma distribution. Tadikamalla (Citation1979), in another study, compared several distributions for approximating the lead time demand (i.e., the normal, the logistic, the gamma, the log-normal and the Weibull) in a lot-size reorder point inventory model and he stated if the distribution of the demand during lead time is right-skewed, the normal and the logistic approximations to the lead time demand are inadequate and the log-normal, gamma and Weibull approximations are versatile and adequate. These three distributions are very similar to each other, while their means and variances are the same (Liittschwager, Citation1965; Tadikamalla, Citation1984). Also, these distributions provide accurate approximations to the lead time demand. However, the log-normal is an applicable candidate since the log-normal distribution can cover a wide range of the right-skewed distribution shapes and its cumulative distribution function can be obtained from a standard normal table and therefore the calculation regarding the reorder level is easier (Tadikamalla, Citation1979). Cobb, Rumí, and Salmerón (Citation2013) proposed an inventory problem wherein the demand per unit time follows the log-normal distribution and the lead time is a probabilistic variable, but the distribution of the demand during lead time is unknown. They developed a procedure based on the mixtures of truncated exponentials (MTE) function to simulate the distribution of the demand during lead time. Halkos, Kevork, and Tziourtzioumis (Citation2014) proposed an efficient procedure for a continuous-review inventory problem to find the optimal reorder point and order quantity providing the global minimum value when the demand during lead time follows the normal and log-normal distributions. Wanke, Ewbank, Leiva, and Rojas (Citation2016) presented a model in which the probabilistic demand and lead time are both follow the triangular distribution. Rojas (Citation2017) proposed a continuous-review inventory model where the demand per unit of time follows a triangular distribution for new products with limited historical data. Kouki, Zied Babai, Jemai, and Minner (Citation2018) proposed a continuous-review full-lost sale base stock inventory model in which uncertain demand follows the compound Poisson distribution.
Many inventory models have assumed that the shortage is allowable. There exist two main categories of the allowable shortage models in the inventory management literature. For the first group, the assumption is that all customers will wait up to receiving of the next order quantity which is called the full backordering case. For the second group, all customers relinquish the systems during the shortage situations which is called lost sale case. But, often in practice, some customers want to wait until receiving the next order quantity while others prefer to relinquish the system. For this condition, the partial backordering mathematical model for inventory systems is considered. The first solution to such model is derived by Montgomery, Bazaraa, and Keswani (Citation1973) and then many authors have expanded this in their studies (e.g., Jauhari, Citation2014; Ouyang, Yeh, & Wu, Citation1996; Chang, Ouyang, Wu, & Ho, Citation2006; Gholami-Qadikolaei, Mirzazadeh, & Tavakkoli-Moghaddam, Citation2015; Braglia, Castellano, & Song, Citation2017; Kumar & Kumar, Citation2016).
In the classical production/inventory models, the parameters such as the setup/ordering cost and the lead time, are assumed to be constant and fixed, and therefore they are not controllable variables. However, often in practice, the lead time can be shortened with an added cost, and hence the parameter is a controllable variable. Reducing the lead time leads to lower safety stock, reduce the loss caused by stockout, increase the service level to the customer, and gain the competitive advantages in business. Liao and Shyu (Citation1991) were first to introduce the concept of variable lead time in an inventory model. They considered the order quantity in their proposed model as a predetermined constant. They divided the lead time into its components and assumed that each component can be reduced to its minimum duration with a crashing cost. Finally, they showed that controlling the lead time may reduce the total expected cost. Hariga and Ben-Daya (Citation1999) studied an inventory model with reducible lead time when the distribution of the demand during lead time is unknown. Based on Moon and Gallego (Citation1994)’s findings, they utilized the minimax distribution-free procedure in order to solve the model in the most unfavorable situation. Pan, Hsiao, and Lee (Citation2002) presented an inventory model with the lead time reduction strategy and they considered the lead time crashing cost as a function of both order quantity and reduced lead time. They also expanded the previous solution algorithms regarding lead time reduction models to solve their model. Chandra and Grabis (Citation2008) assumed lead time as a function of procurement costs. Tahami, Mirzazadeh, Arshadi-Khamseh, and Gholami-Qadikolaei (Citation2016) proposed an integrated inventory model with controllable lead time under inflationary condition. Also, setup/ordering cost reduction has been recognized as an effective way to attain the JIT goal. The idea of the setup/ordering reduction is proposed by Porteus (Citation1985). Ben-Daya and Hariga (Citation2003) studied a stochastic model wherein both lead time and ordering cost may be reduced at a crashing cost. Chen, Chang, & Ouyang (Citation2001) and Chang et al. (Citation2006) proposed another perspective of the setup/ordering cost reduction and assumed that setup/ordering cost and lead time reduction act dependently. In other words, reducing lead time results in decreasing setup/ordering cost accordingly. Kim and Sarkar (Citation2017) proposed a joint replenishment inventory model with multistage quality improvement and lead time-dependent ordering cost. Braglia, Castellano, and Frosolini (Citation2016) proposed a new approach for safety stock planning in an integrated vendor–buyer supply chain model with controllable lead time and stochastic demand.
Many complex production/inventory systems are characterized by uncertain capacities due to unexpected breakdowns, unplanned repairs, etc. This important issue is usually ignored in the inventory control literature. Ciarallo, Akella, and Morton (Citation1994) first proposed an inventory model in which the demand and capacity are probabilistic variables. Wang and Gerchak (Citation1996) analyzed the effect of variable capacity in both EOQ model as well as (Q,r) model with backlogging. Hariga and Haouari (Citation1999) proposed EOQ models with random supplier capacity consideration. They showed that the expected inventory is a pseudo-convex function. Wu (Citation2001) proposed a continuous-review inventory model with the negative exponential random supplier capacity and controllable lead time in which the order quantity, reorder point and lead time are the decision variables. They first assumed the normal approximation to the lead time demand. Then they ignored this assumption and used the minimax distribution-free procedure for solving their proposed model. Moon, Ha, and Kim (Citation2012) proposed three extended models with variable capacity. Firstly, they presented an EOQ model with random yields. Secondly, they extended a multi-item EOQ model with an investment constraint and solve the model based on the Lagrangian method. Thirdly, they applied a distribution-free approach to lot-size reorder-point inventory model. Atasoy, Güllü, and Tan (Citation2012) proposed a dynamic programming approach for an inventory model with non-stationary and deterministic demand and random capacity. They considered the distribution of capacity as the all-or-nothing type. Ross (Citation1996) presented a periodic review mathematical model with variable capacity wherein the retailer is loss averse.
Investigating the inventory management literature related to the models with controllable lead time, the majority of researchers have assumed that the demand per unit time follows the normal distribution or have considered demand distribution to be unknown. However, the results of investigations on the demand distribution in the inventory control literature have shown that the normal distribution assumption to the lead time demand will usually cause great financial damage to a system since the true distribution is often skewed to the right for a wide range of inventory items. Therefore, the motivation of this study is to consider the log-normal distribution to provide a right-skewed distribution for the lead time demand. We have shown in numerical examples when the log-normal distribution for the demand is considered, the expected inventory cost decreases meaningfully by changing the optimal policies. Moreover, the limiting distribution of the log-normal is normal and there is no loss of generality in using the log-normal distribution rather than the normal. Also, the previous research regarding the stochastic available capacity has assumed that the available capacity follows the negative exponential distribution. Hariga and Haouari (Citation1999) considered three distributions, the uniform, the negative exponential and the truncated normal distributions for available capacity. However, the stochastic capacity may be a right-skewed distribution which hasn’t discussed in the previous studies. Hence, in this paper, it is considered that stochastic capacity follows the Erlang distribution that represents a right-skewed distribution. Also, according to the values of the shape parameter, the Erlang distribution’s shape is changed from a decreasing function, thorough unimodal bell-shaped right-skewed distribution, to the normal form of distribution. In other words, the shape of the distribution includes the negative exponential distribution to the normal distribution. Hence, the Erlang distribution covers a wide range of distributions with non-negative values for stochastic available capacity. Also, regarding the studies for the stochastic available capacity system, Wu (Wu, Citation2001) focused on an inventory model with variable capacity and controllable lead time and assumed the ordering cost as a fixed parameter. However, in this paper, we control the lead time and ordering cost simultaneously to improve the performance of total expected cost function.
2. Notations and assumptions
The following notations have been used in this paper:
= | Order quantity | |
= | Reorder point | |
= | The fraction of demand which is lost during stockout period, | |
= | Average demand per year | |
= | Stockout cost per unit short | |
= | Marginal profit per unit | |
= | Holding cost per year per unit | |
= | Fixed ordering cost per order | |
= | Capital investment required to achieve ordering cost | |
= | Fractional opportunity cost of capital per unit time | |
= | Percentage decrease in ordering cost | |
= | Length of lead time | |
= | Total lead time crashing cost per order | |
= | Maximum inventory investment | |
= | Maximum available space | |
= | Demand during lead time | |
= | Random available capacity | |
= | Maximum value of x and 0 | |
= | Mathematical Expectation |
The developed model is based on these assumptions:
The random demand per unit of time,
, follows a log-normal distribution.
The shortage cost is time invariant.
The time the system is out of stock during a cycle is small compared to the cycle length.
It is assumed that the capital investment regarding controlling buyer’s ordering cost,
, has a logarithmic form in terms of ordering cost,
, which is given below.
Where is the fraction of the reduction in
per dollar increase in investment.
(5) The lead time consists of m mutually independent components. The ith component has a minimum duration
, the normal duration
and a crashing cost
per unit time. Further, for convenience, we rearrange
such that
.
(6) If we let
and
be the length of lead time with components
crashed to their minimum duration, then
can be expressed as
and the lead time crashing cost
per cycle for a given
is given by
(7) The components of lead time are crashed one at a time starting with component 1(because it has a minimum unit crashing cost) and then component 2 and so on.
3. Mathematical modeling
We model a continuous-review, single-product, inventory system wherein the objective is to minimize the total expected cost per unit of time including ordering, holding and shortage costs. For the system, we assume that shortage is allowable and partially backordered. The form of uncertain demand distribution is right-skewed which is fitted by the log-normal distribution. The lead time and ordering cost can be reduced with investments. The minimization of the total cost function will be done by a heuristic based on nonlinear programming approach which works by optimizing the order quantity, the reorder point, the lead time, and the ordering cost. We also assume that the random available capacity for every replenishment,, is a continuous probabilistic variable that follows the Erlang distribution, with probability density function,
, which is given as follows:
Thus, the received amount of a product is a random variable and its function can be defined as the minimum of the ordered amount and the random available capacity which can be expressed by
Considering the above random variable, the first two moments of the random available capacity are obtained as follows:
and
Due to the demand fluctuation during the lead time, the shortage may occur when the demand is larger than the reorder level. Therefore, shortage amount is a random variable which is given by
Hence, denotes the expected number of the shortage per cycle. In this study, we consider the partial backorder policy for the system. Consequently, the expected backorder is equal to
. Considering
as expected total inventory per cycle, the total expected cost per cycle can be expressed by
Therefore, taking the expected value with respect to, the expected total cost per cycle is as follows:
Also, the expected cycle time is
In order to obtain the total expected cost function, we utilize the renewal-reward theorem (Ross, Citation1996). By dividing the expected cost per cycle to the expected cycle time, we have
In addition, it is considered that the ordering cost can be reduced through the capital investment with a logarithmic function (see assumption 4). Hence, the ordering cost is changed as a decision variable and the resulting total expected cost is transformed as follows:
3.1. Log-normal distribution for lead time demand
As mentioned previously, the demand per unit time,, follows the log-normal distribution, i.e.,
, if and only if
, which has a PDF as given below
For any . The expected value and the variance of
are as follows:
The lead time demand distribution is obtained by the sum of mutually independent identically distributed log-normal random variables as follows:
Wherein each with mean and variance are given in (12) and (13). Then, the mean of lead time demand,
, is equal to
and the variance is equal to
. Considering Fenton–Wilkinson (FW) approximation, the lead time demand follows the log-normal distribution with parameters
and
. Thus, we have
Solving for and
, gives
Thus, we have
The expected shortage when the lead time demand follows the log-normal distribution is (see Kumar & Kumar, Citation2016)
Hence, the model expressed in (10) is updated for the log-normal-distributed lead time demand which is given as follows:
where
In order to find the optimal values of the above inventory model, first, the partial derivatives of the expected total cost function with respect to ,
and
are calculated. This leads to
where
Also, it can be shown that the optimal lead time occurs at the end of points of the interval (see Liao & Shyu, Citation1991). In other words, the total expected cost function is an increasing function in
. This result will simplify considerably the search for the optimal solution to this inventory problem. On the other hand, for fixed
, the total expected cost per unit of time,
may not be convex for the point
by examining the second-order sufficient conditions. However, the expected total cost per unit of time
is quasi-convex in
.
Lemma1. For a given , the expected total cost per unit of time,
is a quasi-convex in
.
Proof. Differentiating respect to
, we have
Hence, is an increasing function in terms of
. Also, we have
Thus, we have
If there exists a (
) that satisfy
, hence, over
,
is smaller than zero (
) and over
,
is larger than zero (
).
When
then
Thus, we have
As a result, we conclude that for fixed amounts of ,
are quasi-convex in terms of
.
In the next lemma, we show that the total expected cost, , is jointly convex in
.
Lemma2. For a given , the expected total cost per unit of time,
, is jointly convex in
.
Proof. Taking second partial derivative respect to and
, we have
The Hessian matrix, H, for objective function with respect to and
is as follows:
The first principal minor of H is
The second principal minor of H is
Hence, we conclude that the expected total cost per unit of time, , is jointly convex in
for a given
and
.
By considering lemmas (1) and (2), we propose the following solution procedure for finding the approximate optimal order quantity, reorder point, lead time, and ordering cost in order to minimize the total expected cost function.
3.2. Algorithm
Step 1. Define and
where
is a large integer.
Step 2. Divide the interval into
equal subintervals and
is large enough. Consider
.
Step3. For each , perform step to .
Step 4. For given , find
and
from Equations (22) and (23) respectively.
Step 5. Compare and
.
Step 5-1. If ,
is feasible. Otherwise Take
and go to step
Step 6. Compute the corresponding total expected cost
Step 7. For find
Step 8. For find
, then
is approximate optimal solution.
4. Numerical example
In order to show the performance of the developed model in this study, we assume the model input parameters as follows:
We assume that the distribution of weekly demand follows the log-normal distribution as. Also, the data of the lead time components are listed in Table . In addition, we consider
per $ per year and
. Results of the developed algorithm for finding optimal policy are shown in Table for different Erlang-distributed capacity parameters (i.e.
,
) and lead time amounts. As can be seen in Table , for a fixed
and
, the total expected cost is a convex function in terms of different lead time amounts and their corresponding crashing costs. Therefore, the optimal policy of the proposed mathematical model can be obtained by comparing the total expected cost for different lead time amounts and the summary of optimal results is presented in Table . As can be seen in Tables and , the outcomes show that with an increase in
and decrease in
, the expected cost function decreases accordingly. From an economic viewpoint, this implies that with an augment in
or a reduction in
, the expected available capacity increases consequently. Therefore, the optimal expected total cost can be reduced by increasing in optimal order quantity. It is also noted that for large amounts of shape parameters, we have
Table 1. Lead time data
Table 2. Results of solution procedure for various random capacity parameters
Table 3. Summary of the results
Hence, the model is reduced to.
In order to compare the log-normal approximation and the normal approximation to the lead time demand, we calculate the optimal when the lead time demand follows the normal distribution and list the results in Table . As can be seen in the table, savings from 5% to 22% occur when we fit the log-normal distribution to the random demand. For instance, for case
, the optimal solution for the normal distribution case is obtained as
. Therefore, the obtained total expected cost for the log-normal and the normal distributions is
and
, respectively. Hence, it is concluded that if the actual random demand follows the log-normal distribution, but we fit the normal distribution to the random demand, the expected additional cost that the system may be paid is $1004. Thus, using the log-normal approximation to lead time demand reduces the inventory system cost by 22% against implementing the normal approximation. Also, for this case, the optimal lead time for the normal distribution is obtained 4 weeks and for the log-normal distribution is calculated 3 weeks. Comparing with other the other
and
amounts, it is observed that the impact of lead time as the controllable variable is high for the problem. Furthermore, comparing the optimal values of the order quantity and the reorder point regarding the log-normal demand model and the normal demand model, it is observed that the optimal values for the normal demand model are always less than the log-normal demand model for the same mean and variance which is correct because the normal distribution continues to negative infinity. Similar results can be interpreted for the other
and
amounts regarding comparing the log-normal and the normal distributions.
Table 4. Comparison of the log-normal distribution and the normal distribution cases
Table 5. Comparison of the variable ordering cost and fixed ordering cost models
Table 6. Optimal result with changing important system parameters
Also, to investigate the effect of variable ordering cost model, we tabulate optimal values of fixed ordering cost problem in Table . As shown in the table, when we compare our model with variable ordering cost against the model with fixed ordering cost such as Wu (Citation2001), we realize that savings from 60$ to 791$ occur which indicates the controllable ordering cost model may meaningfully reduce the total expected cost per unit of time.
Moreover, a sensitivity analysis is performed regarding the important parameters of the model (i.e., the backorder rate and the parameters of the log-normal demand). Table shows the values for changing parameters to be used in the sensitivity analysis. As can be seen in the table, when the backorder rate increases, the optimal reorder point () and the optimal expected annual cost (
) decrease simultaneously. An economic viewpoint is as follows. A larger value of backorder rate shows a smaller shortage cost. Therefore, the optimal reorder level (
) should be decreased to reduce the optimal expected annual cost (
). Also, due to higher demand per unit time parameters,
, ordering quantity, ordering cost, reorder point are increasing simultaneously, but optima lead time decreases. Besides, the total expected cost function,
, increases consequently.
In the end, considering the inventory management literature regarding controllable lead time (e.g., Ben-Daya & Hariga, Citation2003; Braglia et al., Citation2016, Citation2017; Chandra & Grabis, Citation2008; Chang et al., Citation2006, Citation2006; Chen et al., Citation2001; Gholami-Qadikolaei et al., Citation2015; Hariga & Ben-Daya, Citation1999; Jauhari, Citation2014; Kim & Sarkar, Citation2017; Kumar & Kumar, Citation2016; Liao & Shyu, Citation1991; Moon & Gallego, Citation1994; Ouyang et al., Citation1996; Pan et al., Citation2002; Porteus, Citation1985; Tahami et al., Citation2016), we observe that the authors have assumed that demand during lead time follows the normal distribution or, in a general case, they have assumed that the distribution of lead time demand is unknown and they have utilized the minimax distribution-free procedure to find effective solutions. In this paper, we have proposed a new mathematical model where the lead time demand follows the log-normal distribution and we have derived when the distribution of the demand is right-skewed, implementing a model on the basis of the normality of the demand for finding optimal policy may lead to a large error. Also, investigating the literature review regarding stochastic available capacity (e.g., Atasoy et al., Citation2012; Ciarallo et al., Citation1994; Hariga & Haouari, Citation1999; Liu, Song, Li, & Wu, Citation2015; Moon et al., Citation2012; Wang & Gerchak, Citation1996; Wu, Citation2001), we realize that the authors have utilized many distributions to show the random capacity. In this paper, we have generalized the form of distribution by considering a gamma-type distribution to cover a wide range of distribution shapes from the negative exponential distribution to the normal distribution which is more complete case against the previous works.
5. Conclusion and managerial implications
One of the significant steps in computing optimal policy of an inventory/production system is related to identifying the distribution of the random demand. It is obvious that using inappropriate demand distribution causes to incorrect optimization of the decision variables and therefore it arises great financial loss for an inventory/production system. Often in practice, when the historical data regarding an item are available, a probability distribution is fitted to the uncertain demand pattern and then the expected inventory cost function is modeled based on the properties of the fitted probability distribution. As mentioned by many researchers in the inventory management literature regarding stochastic demand problems, the distribution of the demand is the right-skewed type for many items and products. However, usually due to arising difficulties during mathematical modeling of the cost function, the normal distribution is utilized to formulization of the demand distribution. Therefore, in this paper, we have considered the log-normal distribution when demand distribution is right-skewed type and we have shown with a thorough analysis that utilizing the log-normal distribution rather than the normal distribution results in correct and accurate optimization of decision variables and reduces the expected cost meaningfully. Also, studying the literature regarding the random available capacity, researchers have used distributions such as the truncated normal, negative exponential and uniform to show the pattern of random capacity. In order to generalize the previous works in this area, we have modeled the random capacity based on the gamma distribution to provide a wide range of distribution shapes in order to fit an accurate distribution based on historical data. Based on the above-mentioned contributions and in the controllable lead time and ordering cost environments, we have proposed a continuous-review infinite-horizon inventory model with a mixture of backorder and lost sale wherein order quantity, reorder level, lead time, and ordering cost are deterministic decision variables and A solution procedure has proposed to find approximate optimal values. The performance of the model has been clarified by numerical examples. Based on the proposed numerical examples we have proved that using our model rather than previous models in the presented area can gain more profit for an inventory production system. We have shown that there is a large difference between the optimal decision variables of our proposed model with the log-normal demand and the gamma available capacity comparing with the standard lot-size reorder point model. Also, we have illustrated for a right-skewed demand distribution, the impact of lead time may be high in the total expected cost. In summary, according to the numerical examples, the proposed mathematical model in this study may reduce the expected costs of the inventories more than 20% which is high compared to the previous models. For future research, we can consider several ways to expand the developed mathematical model in this paper. For instance, lead time crashing cost can be considered as a function of both ordering quantity and reduced lead time. Considering this situation, the solution procedure is changed for finding optimal values. Investigating on other distributions such as the Weibull distribution for available capacity can be considered for the model. Some kinds of constraints like budget, storage space could be added to this model in order to make the system more close to the real environment.
Acknowledgements
The authors acknowledge the constructive comments made by the anonymous referees on an earlier version of the paper.
Additional information
Funding
Notes on contributors
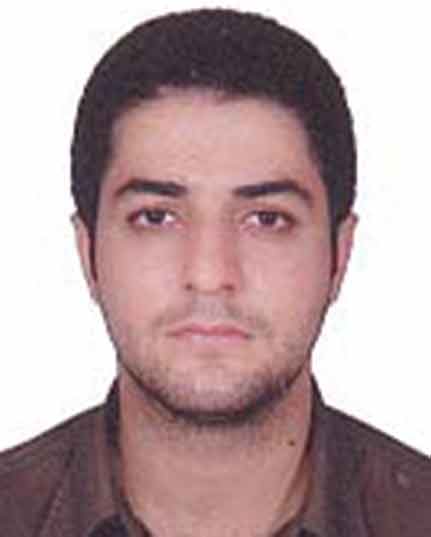
Abolfazl Mirzazadeh
Aref Gholami received his MSc in industrial engineering from Kharazmi University. He is a PhD student in industrial engineering at Kharazmi University. He has published more than 10 papers in the field of inventory/production control in international journals.
Abolfazl Mirzazadeh is a professor of industrial engineering at Kharazmi University, Tehran, Iran. His research areas are Uncertain Decision Making, Production/Inventory Control, Supply and Operations Management, and Quality Management Tools. He has more than 80 published papers in high-quality journals and more than 55 international conference papers. He is now Editor-in-Chief of IJSOM (www.ijsom.com), and also Scientific Committee manager of the International Conference. He earned several awards as the best researcher, best faculty member in the international collaborations, and the best lecturer. Also, he is member of Journal’s Editorial Board, Conferences Scientific Committee, and International Associations (https://www.linkedin.com/in/a-mirzazadeh-48459936/).
References
- Atasoy, B., Güllü, R., & Tan, T. (2012). Optimal inventory policies with non-stationary supply disruptions and advance supply information. Decision Support Systems, 53(2), 269–281. doi:10.1016/j.dss.2012.01.005
- Bagchi, U., & Hayya, J. C. (1984). Demand during lead time for normal unit demand and Erlang lead time. Journal of the Operational Research Society, 35, 131–135. doi:10.1057/jors.1984.19
- Ben-Daya, M., & Hariga, M. (2003). Lead-time reduction in a stochastic inventory system with learning consideration. International Journal of Production Research, 41, 571–579. doi:10.1080/00207540210158807
- Braglia, M., Castellano, D., & Frosolini, M. (2016). A novel approach to safety stock management in a coordinated supply chain with controllable lead time using present value. Applied Stochastic Models in Business and Industry, 32, 99–112. doi:10.1002/asmb.v32.1
- Braglia, M., Castellano, D., & Song, D. (2017). Distribution-free approach for stochastic Joint-Replenishment Problem with backorders-lost sales mixtures, and controllable major ordering cost and lead times. Computers & Operations Research, 79, 161–173. doi:10.1016/j.cor.2016.11.002
- Burgin, T. A. (1975). The gamma distribution, and inventory control. Journal of the Operational Research Society, 26, 507–525. doi:10.1057/jors.1975.110
- Chandra, C., & Grabis, J. (2008). Inventory management with variable lead-time dependent procurement cost. OMEGA, 36, 877–887. doi:10.1016/j.omega.2006.04.009
- Chang, H.-C., Ouyang, L.-Y., Wu, K.-S., & Ho, C.-H. (2006). Integrated vendor-buyer cooperative inventory models with controllable lead time and ordering cost reduction. European Journal of Operational Research, 170, 481–495. doi:10.1016/j.ejor.2004.06.029
- Chen, C. K., Chang, H. C., & Ouyang, L. Y. (2001). A continuous review inventory model with ordering cost dependent on lead time. International Journal of Information and Management Science, 12(3), 1–13.
- Ciarallo, F. W., Akella, R., & Morton, T. E. (1994). A periodic review, production planning model with uncertain capacity and uncertain demand-optimality of extended myopic policies. Management Science, 40, 320–332. doi:10.1287/mnsc.40.3.320
- Cobb, B. R., Rumí, R., & Salmerón, A. (2013). Inventory management with log-normal demand per unit time. Computers & Operations Research, 40, 1842–1851. doi:10.1016/j.cor.2013.01.017
- Gholami-Qadikolaei, A., Mirzazadeh, A., & Tavakkoli-Moghaddam, R. (2015). Lead time and ordering cost reductions in budget and space restricted probabilistic inventory models with imperfect items. RAIRO-Operations Research, 49, 215–242. doi:10.1051/ro/2014031
- Hadley, G., & Whithin, T. (1963). Analysis of inventory system. Prentice-Hall.
- Halkos, G., Kevork, I., & Tziourtzioumis, C. (2014). On the convexity of the cost function for the (Q, R) inventory model. Munich Personal RePEc Archive (MPRA).
- Hariga, M., & Ben-Daya, M. (1999). Some stochastic inventory models with deterministic variable lead time. European Journal of Operational Research, 113, 42–51. doi:10.1016/S0377-2217(97)00441-4
- Hariga, M., & Haouari, M. (1999). An EOQ lot sizing model with random supplier capacity. International Journal of Production Economics, 58, 39–47. doi:10.1016/S0925-5273(98)00086-3
- Hariga, M. A. (2010). A single-item continuous review inventory problem with space restriction. International Journal of Production Economics, 128, 153–158. doi:10.1016/j.ijpe.2010.06.008
- Jauhari, W. A. (2014). Lot size decisions for vendor-buyer system with quantity discount, partial backorder and stochastic demand. Advances in Operations Research, 2014, 1–7. doi:10.1155/2014/597626
- Kim, M.-S., & Sarkar, B. (2017). Multi-stage cleaner production process with quality improvement and lead time dependent ordering cost. Journal of Cleaner Production, 144, 572–590. doi:10.1016/j.jclepro.2016.11.052
- Kouki, C., Zied Babai, M., Jemai, Z., & Minner, S. (2018). Solution procedures for lost sales base-stock inventory systems with compound Poisson demand. International Journal of Production Economics. doi:10.1016/j.ijpe.2018.01.021
- Kumar, S., & Kumar, N. (2016). An inventory model for deteriorating items under inflation and permissible delay in payments by genetic algorithm. Cogent Business & Management, 3. doi:10.1080/23311975.2016.1239605
- Lee, H. L., & Nahmias, S. (1993). Single-product, single location models: Handbooks in operations research and management science (Vol. 4, pp. 3–55). Elsevier: North Holland.
- Liao, C.-J., & Shyu, C.-H. (1991). An analytical determination of lead time with normal demand. International Journal of Operations & Production Management, 11, 72–78. doi:10.1108/EUM0000000001287
- Liittschwager, J. M. (1965). Results of a gamma, lognormal and Weibull sampling experiment. Industrial Quality Control, 22, 124–127.
- Liu, W., Song, S., Li, B., & Wu, C. (2015). A periodic review inventory model with loss-averse retailer, random supply capacity and demand. International Journal of Production Research, 53, 3623–3634. doi:10.1080/00207543.2014.985391
- Mohebbi, E., & Posner, M. J. M. (1998). A continuous review inventory system with lost sales and variable lead time. Naval Research Logistics, 45, 259–278. doi:10.1002/(ISSN)1520-6750
- Montgomery, D. C., Bazaraa, M. S., & Keswani, A. K. (1973). Inventory models with a mixture of backorders and lost sales. Naval Research Logistics, 20, 255–263. doi:10.1002/(ISSN)1931-9193
- Moon, I., & Gallego, G. (1994). Distribution free procedures for some inventory models. Journal of the Operational Research Society, 45, 651–658. doi:10.1057/jors.1994.103
- Moon, I., Ha, B. H., & Kim, J. (2012). Inventory systems with variable capacity. European Journal of Industrial Engineering, 6, 68–86. doi:10.1504/EJIE.2012.044811
- Ouyang, L.-Y., Yeh, N.-C., & Wu, K.-S. (1996). Mixture Inventory Model with Backorders and Lost Sales for Variable Lead Time. Journal of the Operational Research Society, 47, 829–832. doi:10.1057/jors.1996.102
- Pan, J. C., Hsiao, Y.-C., & Lee, C.-J. (2002). Inventory models with fixed and variable lead time crash costs considerations. Journal of the Operational Research Society, 53, 1048–1053. doi:10.1057/palgrave.jors.2601354
- Porteus, E. L. (1985). Investing in Reduced Setups in the EOQ Model. Management Science, 31, 998–1010. doi:10.1287/mnsc.31.8.998
- Rojas, F. (2017). A methodology for stochastic inventory modelling with ARMA triangular distribution for new products. Cogent Business and Management, 4, 1270706.
- Ross, S. M. (1996). Stochastic processes (2nd ed.). New York, NY: Wiley.
- Tadikamalla, P. R. (1978). Applications of the Weibull distribution in inventory control. Journal of the Operational Research Society, 29, 77–83. doi:10.1057/jors.1978.11
- Tadikamalla, P. R. (1979). The lognormal approximation to the lead time demand in inventory control. Omega, 7, 553–556. doi:10.1016/0305-0483(79)90074-4
- Tadikamalla, P. R. (1984). A comparison of several approximations to the lead time demand distribution. Omega, 12, 575–581. doi:10.1016/0305-0483(84)90060-4
- Tahami, H., Mirzazadeh, A., Arshadi-Khamseh, A., & Gholami-Qadikolaei, A. (2016). A periodic review integrated inventory model for buyer’s unidentified protection interval demand distribution. Cogent Engineering, 3. doi:10.1080/23311916.2016.1206689
- Wang, Y., & Gerchak, Y. (1996). Continuous review inventory control when capacity is variable. International Journal of Production Economics, 45, 381–388. doi:10.1016/0925-5273(95)00128-X
- Wanke, P., Ewbank, H., Leiva, V., & Rojas, F. (2016). Inventory management for new products with triangularly distributed demand and lead time. Computers & Operations Research, 69, 97–108. doi:10.1016/j.cor.2015.10.017
- Wu, K.-S. (2001). A mixed inventory model with variable lead time and random supplier capacity. Production Planning & Control, 12, 353–361. doi:10.1080/09537280152004978