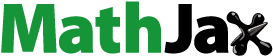
Abstract
This paper studies the relationship framework between individual and group acts of stock trading activities in the Tehran stock exchange which leads to the development of a cohesive relationship model for individual and group acts of stock trading behaviors. It has a quantitative–qualitative hybrid approach in which the model is designed by using the Delphi technique at the first stage, and it is tested and assessed by using the structural equation modeling method at the second stage. The model shows that areas of financial capability, financial capacity, word-of-mouth communication, mass media, financial consultation, stock trading behaviors, and personality are considered as causal conditions of relationship framework of individual and group acts that affect that process positively and meaningfully.
PUBLIC INTEREST STATEMENT
After the development of behavioral finance theory, the experimental literature in this domain has been exclusively oriented toward investigating the relationship between investors’ inclinations and variations of stocks’ prices. There hasn’t been any independent research on investigating factors contributing to behavioral mistakes of investors and how they can be moderated. Also, due to the cognitive and environmental complexities and the multitude of variables influencing the capital market investment process, investor decision criteria are a set of personal and group factors, and relying only on the rational basis of knowing decision criteria is not very compatible with reality. So, this paper studies the relationship framework between individual and group acts of stock trading activities in the Tehran stock exchange which leads to the development of a cohesive relationship model for individual and group acts of stock trading behaviors.
1. Introduction
The Iranian Stock Exchange may be the only example in the world where are many state-owned companies that play a key role in stock market volatility and trading volumes, although they are not generally required to provide transparent reports. Unfortunately, the stock market organization has not been supervised in this regard (Badri, & Ezabadi, Citation2015). For this reason, studying the stock trading activity of these companies and the process of their formation is very important.In recent years, Increasing the responsibility of individuals for retirement saving has resulted in more participation in the stock market (Campbell, John Y., Jens Dietrich Hilscher., and Jan Szilagyig Citation2011; Alessie, Van Rooij, and Lusardi (Citation2011); Lusardi -and Mitchell, Citation2007). Meanwhile, the complexity of financial products and the risk of failure in financial markets are challenges they facing in investing rationally (Lusardi & Mitchell, Citation2011a). According to researchers’ findings, some households are not enough literate in financial matters (Lusardi & Mitchell, Citation2007), and have no information about financial markets (Guiso & Jappelli, Citation2006), and commit biased behaviors (Kahneman, Knetsch, & Thaler, Citation1991), and trade excessively (Barber & Odean, Citation2001), which in turn leads to poor financial decision-making (for example, Campbell, Citation2006; Goetzmann & Kumar, Citation2008; Polkovnichenko, Citation2005). Considering the limited ability of individual investors in making informed trading decisions in the presence of uncertainty, where the estimation of expected returns and the probability distribution of returns is difficult, the key sources of information play an important role in determining financial alternatives for investors. They may obtain some information from different sources; some sources are specialized (for example, consulting with financial experts), but others are not specialized (for example, friends, peers, and other means of social interaction). Each source of information is different for its value and investment costs. In the past literature, the subject of non-specialized information and the way it is used have not received much attention in terms of personality and trading behavior of investors. The present study examines how the investor behaves in the stock market regarding his/her personality traits and the way information sources are used. Recent empirical studies that investigated trading activities of different groups of investors showed that the investors followed various trading patterns as one of the market anomalies, and most of them adopted a contrarian or momentum strategy and sometimes demonstrated herding behavior. Studies conducted on emerging markets showed that institutional investors purchased past losers and sold past winners (contrarian trading strategy) and individual investors followed herding behavior; and hence, they had different performance regarding market timing and stock selection. It seems to the reasons for changes in stock trading activities need to more investigating. The changes that occur in the sale of a stock, rather than the performance of the company, are due to the behaviors of investors and activists in the capital market. It starts with word of mouth and financial advisory and spreads to stock market players in an increasing wave. This article examines the trend of these movements and their relation to stock changes.
2. Theoretical foundations and literature review
Studies show that the majority of investors are less interested in speculation and high-risk trades (Saedi & Mokhtarian, Citation2009). Therefore, most rational models generally have less efficiency in financial decision-making. Efficient Market Hypothesis (EMH), which is formed based on the normative model of traditional financial theory, assumes that market participants exactly know the rational process of investment with all related sources of information. In asset pricing theories, such as capital asset pricing model and arbitrage pricing model, it assumes that if an asset is incorrectly priced, all or at least some investors quickly trade it in unlimited quantities; so that the prices are quickly adjusted and the price equalization point is achieved. In fact, according to EMH, which is one of the most fundamental financial theories, investors cannot obtain abnormal returns through price anticipation. Whereas, in the last three decades, with the emergence of anomalies and the development of a new field in the financial sciences called behavioral finance, the efficiency of the market has been challenged. In fact, many investors seek to gain abnormal returns by anticipating market price trends. Furthermore, EMH believes that markets are completely efficient since all market information is already integrated into existing securities prices, hence no new information or accurate analysis should be involved to outperform a benchmark. Indeed, the market often has overreacted to intangible information which is due to overconfidence bias, and once new news is released, the expectations will be adjusted (Bazzaz Zadeh Sorbate, Malekian, & Kamyabi, Citation2016). On the other hand, behavioral finance theory shows how the behavior of market participants is shaped which is consistent with the descriptive model of psychological decision-making. The descriptive model shows how investors are not able to process all market information since, like the decision results in other life aspects, trading decisions of investors may also be affected by various psychological processes such as effect, cognition, and heuristics. A human being cannot process all related information since his/her behavior is influenced by “affective reactions” such as feelings, mood, and emotion or “cognitive reactions” such as being influenced by available information. On the other hand, individuals also use “heuristics”, namely rules of thumb, which people used to make decisions in a limited period to reduce complexity in a certain situation (Pitters & Oberlechner, Citation2014).
In the emerging literature of behavioral finance, some studies have investigated the trading behavior of investors based on different psychological explanations. Odean (Citation1998), Gervais and Odean (Citation2001), and Daniel, Hirshleifer, and Subrahmanyam (Citation1998) suggested theoretical models in which investors are not able to exactly evaluate their investment ability because they are prone to behavioral biases. Investors in their models become overconfident about their investment skills in a period. Barber and Odean (Citation2001) examined overconfidence biases. They noticed that portfolios of overconfident investors show excessive trading activities but poor investment performance. Grinblatt and Keloharju (Citation2009) demonstrated that investors who are more susceptible to the psychological trait of sensation seeking trade stocks more frequently. They also showed that the act of purchasing and selling stocks can have more and new variations for these investors than a buy and hold strategy. Other researchers showed that anomalies of the investors’ trading behavior are more related to other psychological foundations (for example, Barber & Odean, Citation2002, Citation2008; Dhar, Goetzmann, & Zhu, Citation2004; Rashes’, Citation2001). In one of the subjects of psychology, namely personality psychology, it is argued that personality is a key factor in human behavior. In a certain situation, personality forms a specific inclination towards a certain characteristic reaction; therefore, personality can be considered as a key factor of human behavior (Heinström, Citation2003). Psychologists believe that trading decisions of investors are a type of human behavior that can be associated with investor personality. In behavioral finance literature, several conducted studies have modeled personality psychology for the trading behavior of investors. Pompian and Longo (Citation2004) believed that via identifying the main investor personality trait, advisors can help in reducing job satisfaction rates stemming from behavioral biases.
Van Witteloostuijin and Muehlfeld (Citation2008) showed that personality traits of traders such as maximizing tendency, locus of control, self-monitoring, regret disposition, and type-A and type-B are relevant to trading behavior. Durand, Newby, and Sanghani (Citation2008) showed that the Big Five personality is related to investors’ trading behavior. They found a negative relationship between extroversion and trading. These findings are unusual in comparison to the previous theoretical prediction that extroverted individuals incline to trade more. Durand, Newby, Tant, and Trepongkaruna (2013) also found a negative relation between extroversion and trading. They stated that extroverted individuals have more tendencies toward less trading and hence are not willing to trade frequently. Durand et al. (Citation2008) found a positive relationship between negative emotions and trading frequency, which is by the argument that nervous investors seek to reduce unpleasant feelings arising from external stimuli. Durand, Newby, Peggs, and Pedds (Citation2013) found a positive relationship between conscientiousness and trading behavior. These findings are also consistent with those of Durand et al. (Citation2013) who believed that conscientious individuals try to achieve desirable results and hence trade more. Recently, in a study by Conlin et al. (Citation2015) on analyzing the relationship between personality traits of Finnish individuals and stock trading behavior, it became clear that different personality traits are important predictors of investors’ stock market participation. The aforementioned studies found how differences in investors’ psychological characteristics lead to diversifications of their financial behavior. These diversifications are yet largely unknown, and hence, new approaches should be adopted to discuss the psychological characteristics of investors. Financial advisors may influence the trading behavior of investors in two opposite directions. As financial professionals are less likely to suffer from behavioral biases, they can limit the excessive trading of their clients (Shapira & Venezia, Citation2001). Some studies categorize behavioral biases. Some define biases as heuristics, beliefs, judgments, and preferences while others categorize them into cognitive and emotional biases. Cognitive biases arise from defective reasoning and encompass heuristics, availability, and representative biases. In contrast, emotional biases stem from impulse or intuition and include loss aversion, self-attribution, and conservatism. Both types yield non-rational judgments, therefore, better information may help investors to avoid the harmful effects of these biases (Pompian, Citation2012). On the other hand, the fact that financial advisors have incentives to gain more trading commission can make them encourage their clients to trade more (Haigh & List, Citation2005; List, Citation2003). Financial advisors can monitor trading activity (Campbell & Viceira, Citation2003). It has been argued that the quality of the information source has a positive impact on trading since news from reliable sources results in more investment to adjust securities (Epstein & Schneider, Citation2008). Fischer and Gerhardt (Citation2007) stated that financial advice has a positive effect on trading because it allows investors to have better self-assessment of their own skills and hence leads to more rational investment decisions. Shapira and Venezia (Citation2001) found that the number of various stocks and stock transactions per year increases when investors receive professional advice. These findings suggest that financial advisors help to diversify their clients’ portfolios, showing a positive impact of financial advice on trading. Using data from German banks, Gerhardt and Hackethal (Citation2009) examined the effect of financial advisor on households’ trading which showed a negative relationship between financial advice and trading. These findings were confirmed by Karabulut (Citation2013) who also showed the negative relationship between financial advice and trading frequency. On the other hand, Hackethal, Haliassos, and Jappelli (Citation2012), while studying German investors from internet brokerage firm, found that investors increase trading when they receive financial advice. Kramer (Citation2012) also found similar evidence when examining Dutch investors. Investors rely on social interaction to exchange information concerning the stock market by means of word-of-mouth communication. Ivković and Weisbenner (Citation2007) showed that the stock market participation of households is affected by the stock purchasing of their neighbors. They suggested that taking into account a lack of expertise for assessing investment decisions, individual investors tend to reduce search costs by relying on word-of-mouth communication with people around them. These findings are consistent with those of Lusardi and Mitchell (Citation2011b) and Van Rooij, Lusardi, and Alessie (Citation2011) who also showed that financial investors take financial guidance from non-specialized sources such as family, friends or peers rather than taking advice from professionals. Madrian and Shea (Citation2000) suggested that social interaction has a significant impact on employee decisions to participate in retirement plans because individuals tend to follow the saving choices of their co-workers. These findings are by those of Duflo and Saez (Citation2002) and Duflo and Saez (Citation2003) who also showed that co-workers’ choices of saving plans play an important role in individuals’ saving choices. Hong, Kubik, and Stein (Citation2004) suggested a model showing that investors propagate information concerning stocks to one another through word-of-mouth communication. They found that stock market participation of social investors is affected by the trading activity of their peers. These findings were confirmed by Hong, Kubik, and Stein (Citation2005) who, by examining the influence of social interaction on mutual funds managers’ trading behavior, noted that fund managers of the same city would trade a specific stock if other funds mangers are trading a similar one. Pool, Stoffman, and Yonker (Citation2015) found that U.S. fund managers living in the same neighborhood tend to have holdings and trading patterns that are similar to those living in different neighborhoods. Describing the characteristics of investors who participate in trading concerning social interaction, Brown, Zoran, Smith, and Weisbenner (Citation2008) showed that individuals living in a social group tend to invest in the stock market when other people in the same social group have active stock market participation. And, this community effect on individuals’ investment behavior might be driven by word-of-mouth interaction between individuals and social groups. They also argued that this community effect has a stronger impact on more sociable communities. These findings match with those of Changwony, Campbell, and Tabner (Citation2014) who found that the active participation of an individual in a social group has a positive effect on a company’s stock market. Accordingly, Heimer (Citation2014) found that active investors are more extensively involved in social interaction than passive investors. Ng and Wu (Citation2010) found that Chinese investors of the same branch of brokerage firm make similar trading decisions. These findings are in contrast to the previous study conducted by Feng and Seasholes (Citation2004) in China. In this paper, to explain the reasons for investors’ behavioral changes, a conceptual framework is developed to make a decision considering different sources of information. This theoretical model seeks to explain investors’ behavior based on personality, its susceptibility to psychological processes, the way of acquiring and interpreting information from different sources, and how these factors affect their trading decisions in the stock market.
3. Research background
Empirical studies have found a variety of trading patterns utilized by different types of investors. Various studies showed that investors apply different trading strategies, and observed that some investors follow collective market behavior.
In foreign studies, Tauni, Hong, and Amjad (Citation2017), asserting the importance of financial advice in stock trading behavior, showed that key sources of information resulting from a financial advisor and word-of-mouth communication promote the relationship between personality traits of investor and stock trading behavior. The above study was conducted based on five personality measures from the Big Five theory of Costa and McCrae (Citation1989). The data was collected from 541 individual investors of the Chinese stock market. The results showed that investors with openness and neuroticism traits, acquiring information from a financial advisor, purchase more stocks, while extraverted and conscientious investors, acquiring information from a financial advisor, purchase fewer stocks but become more susceptible to purchase stocks when acquiring information through word-of-mouth communication. In this study, investors with openness, conscientiousness, and neuroticism traits had more trade frequency. The results of a study conducted by Walid M.A. Ahmed (Citation2014) in the Qatar Exchange, using investment flows and an autoregressive model, showed that individual investors followed contrarian trading strategy whereas institutional were engaged in momentum trading strategy. Various studies that try to discover trading patterns of different investors often examine the trading performance of investors. Their findings show that trading patterns of different investors lead to different trading performance, and their investigation on the investment flows of individual and institutional investors demonstrates that the latter has better market timing performance while the former has undesirable market timing performance. Identifying the trading behavior of four groups of investors in Thailand Exchange, Fung and Durand (Citation2014) examined the trading performance of these groups. They found that although in Thailand market institutional investors have better performance concerning the timing of trades; their stock-selection performance is poor. Their results also confirmed those of previous studies, suggesting that the collective behavior of individual investors leads to poor performance due to improper timing of trades. In addition to identifying trading patterns of Norwegian individual investors, Li (Citation2014) found that their good performance arises from the desirable timing of trades. Testing gambling results, Fréchette, Schotter, and Trevino (Citation2014) investigated the contribution of Big Five personality framework to the type of information investors acquire under uncertainty, and showed how acquired information affects gambling. They found that personality determines the type of acquired information which in turn influences the selection of gambling options. The authors established an indirect path to test personality impacts on economic choices resulting from information acquisition. Yang, Hsu, and Tu. (Citation2012) demonstrated that financial advisors affected the confidence level of investors and stock trading volume. They found that advisors with extroverted and conscientious personality most likely gain investors’ confidence to trade more. Karabulut (Citation2013) showed that the demand for financial advice was considerably lower among biased investors. He also showed that overconfident investors were less interested in financial advice. These findings are consistent with evidence suggesting that overconfident investors less rely on information from financial advisors and prefer to collect required information by themselves (for example, Barber & Odean, Citation2001; Guiso & Jappelli, Citation2006). Karabulut (Citation2013) also stated that investors whose subjective competence was higher, less sought to acquire information from financial advisors. This arises from the fact that more competent investors are more willing to bet on their own judgments (consistent with the findings of Graham, Harvey, & Huang, Citation2009; Heath & Tversky, Citation1991).
In domestic studies, yet no study has been conducted on separating different types of investors to identify trading strategies of investors in Iran investment market. However, several studies examine the utility of contrarian and momentum strategies and identify the collective trading behavior of investors. As an example, Ghorbani Pahlevan and Bashashi (Citation2017) conducted a study entitled “the impact of investors’ personality traits on information acquisition and trading behavior of investors in Zanjan Stock Exchange”, in which they examined the impact of investors’ personality traits on the relationship between information acquisition and trading behavior of investors in Zanjan Stock Exchange. The coefficient of hierarchical regression showed that investors’ personality traits have a meaningful effect on the relationship of information acquisition and trading behavior of investors in the Zanjan Stock Exchange. Also, personality traits dimensions including extroversion, openness to experience, neuroticism, conscientiousness, and agreeableness in terms of subsidiary assumptions influenced the relationship of information acquisition and trading behavior of investors using the path analysis technique. Sobouti and Shamakhi (Citation2016) in a study entitled “the impact of behavioral finance factors on the investors’ returns in Tehran Stock Exchange” investigated the impact of behavioral finance factors on the investors’ returns in Tehran Stock Exchange. Its statistical population consists of listed companies in Tehran Stock Exchange, and the sample under study involves 115 companies from different industries. The results indicated that variables including regret aversion, disposition effect, herding behavior, and overconfidence, and social effects bias influence investors’ returns on the Tehran Stock Exchange. Haratian and Faez (Citation2015) conducted a study entitled “Designing a mental model of financial planning to give financial advice” to design a mental model of financial planning to give financial advice to individuals. Regarding the fact that managing personal financial affairs is significantly important for every individual, the authors, comparing their designed model to the basic smart mental financial planning, tried to find its strengths and weaknesses, and finally give smart personal financial advice and offer strategies for smart financial management and personal financial decision making by using this information. In a study by Badri and Ezabadi (Citation2015) entitled “Investor type trading behavior and trade performance in Tehran Stock Exchange”, using an autoregressive model and a Grinblatt & Titman portfolio performance measure in a weekly manner, trading patterns and elements of trading behavior of individual and institutional investors in Tehran Stock Exchange during 2008–2012 was examined. The results showed that, in general, individual investors followed collective behavior, whereas institutional investors utilized a contrarian trading strategy. However, no evidence found concerning that individual and institutional investors adopted a momentum strategy. Adopting contrarian strategy, institutional investors had better trading performance over most trading horizons, and a considerable amount of returns was due to the proper timing of trades, though a part of their returns was overshadowed by selecting improper stocks. In contrast, individual investors lost some part of their trading performance returns due to inappropriate market timing despite good performance regarding stock selection. Toghiani (Citation2014) conducted a study entitled “Investigating the impact of social factors on investors’ trading behavior in Tehran Stock Exchange” in which he examined the impact of social and psychological variables on the Iran stock market, and proposed a model to understand and predict the stock market. In a study by Jahangiri Rad, Marfoo, and Salimi (Citation2014) entitled “Studying herding behavior of investors in Tehran Stock Exchange” herding behavior of investors in that market during 2006–2011 was examined. The model of Chang, Cheng, and Khurana (Citation2000) were used in this study. Robust regression was used to estimate the model. The results showed that investors exhibited herding behavior in the Tehran Stock Exchange, and this behavioral type occurred more in the bullish market rather than in the bearish market. Baghdar Eram (Citation2011) conducted a study entitled “Investigation of behavioral finance factors affecting investors’ decision making in Tehran Stock Exchange” in which he identified and explained some behavioral finance factors affecting investors’ decision making in Tehran Stock Exchange. These tested behavioral finance factors include overconfidence, escalation of commitment, herding behavior. The findings suggested that herding behavior affected investors’ decision making. In a study by Jamshidian (Citation2012) entitled “behavioral factors affecting the decision making of active individual investors in Tehran Stock Exchange” he investigated the behavioral finance field and its contribution to affecting investment decisions of active individual investors in Tehran Stock Exchange. The author identified effective behavioral factors and determined their levels of effectiveness by measuring and applying four well-known theories in behavioral finance field, i.e., prospect theory including variables such as loss aversion, regret aversion, and mental accounting; heuristic theory including five variables, namely representativeness, overconfidence, anchoring, gambler’s fallacy, and availability; market theory including variables such as market information, past trends of stocks, price changes, fundamentals of underlying stocks, customer preference, and over-reaction to price changes; and herding behavior theory including speed of herding, buying or selling decisions, choice of stock to trade, volume of stock to trade. The results showed that herding was selected as a predominant theory among other considered theories. The results also showed that fundamental analysis was the most widely used decision-making tool among active individual investors in Tehran Stock Exchange.
4. Development of conceptual model
The library and field methods were used to formulate theoretical foundations and determine the components related to individual and group acts in stock trading activities. In the field method, using the Delphi method, the final model is modified and formulated, and the relations between components are then established. The obtained findings are categorized into two individual and group acts categories to formulate theoretical foundations. Individual acts encompass those activities of investors that result from the individual abilities and personal characteristics, and he/she is directly responsible for those activities (Tauni et al., Citation2017). Group acts involve those investment activities which result from collective dynamics and occur as a result of individual interaction with different official and unofficial groups (Karabulut, Citation2013). Group acts indirectly affect individual investors’ decisions, and actually individual acts of investors are the ultimate determinant of financial decisions. To establish a theoretical framework via the Delphi method, panels of professionals were formed in which the members’ interaction was conducted by the chairman or supervisor of the panel. Internal communications of participants are anonymous and opinions, predictions, and desires are not attributed to their providers. This information will be released without identifying the providers. Generally, the main components in the primary methods of Delphi include structuralizing information flow, providing feedback to participants, and anonymity of the participants. Using a questionnaire that was given to the individuals, they were asked, in their opinions, how much-identified factors could be effective in conceptual and operational criteria. And, in the second round, they were asked, as a researcher, what factors they considered important in this respect. To gather information, the questionnaires were given to professors and experts consisting of 20 individuals, and sampling was carried out in an unlimited population by using the snowballing method. The Delphi survey method was performed in two rounds. In the first round, those components that scored less than mean were omitted. This method showed the minimum scores of selected variables in the first round based on mean scores obtained by all variables and according to the calculated mean of standard deviation. Hence, those variables which obtained scores lower than the above score were excluded from the list of variables related to the proposed model. In the second round, new components and sub-components were added to the framework dimensions of the model. The ranking of derived components was based on the literature review of this study. The second part of the questionnaire pertained to the components that were less addressed in the literature review understudy but were imported from the respondents’ perspective. No criteria or standard methods were considered for selecting specified variables in the first round. However, in many scientific types of research, it is tried to encourage almost all participants of the first round to participate in the second round considering noted criteria in the first round, considering the opinions of relevant professors and experts, and considering follow-ups (by email or telephone). The second-round results of the Delphi method which totally consist of 9 components and 34 sub-components are presented in Table .
Table 1. Statistical findings related to criteria understudy in the first round of the Delphi method
According to relation (1), all items are qualified to be selected in the second stage since they are higher than the mean threshold of 2.97. The results of the first and second rounds represent convergence among the means of components. Table shows that there is no meaningful difference among means related to the scores of variables under study.
Table 2. The results obtained from T-test to compare the means of two independent samples
Notwithstanding the various studies conducted on investors’ decision making, there are not enough researches regarding investment success, and hence no criteria, which are acceptable to most researchers, have been defined to assess success parameter in investors’ decision making. In literature, the scale of achievement to performance objectives is considered as the conventional parameter. There is no consensus among researchers about defining success assessment of investors’ decision making, their performance, and in essence their success or failure; and certain criteria are not available to assess success rate (Tauni et al., Citation2017). The conceptual model is designed and proposed based on the theoretical foundations of the present study. The conceptual model is illustrated in the diagram Figure .
5. Research methodology
This study first designed the model and next verified it using qualitative and quantitative methods, respectively. So, the research was conducted in a hybrid (qualitative-quantitative) way. The study approach in designing step started by developing a theoretical framework and then steps of stating hypotheses and drawing a logical inference from the results follow. In the second step, the model was verified through collecting data, constructing hypotheses, and subsequently providing theory based on the hypotheses. Data mentioned in this study was provided from two different sources. Secondary data was obtained from books, articles, and documents. Primary data were obtained from interviews and questionnaires. The steps of hypothesis testing and the path to achieving the hypotheses through the main questions of the study in the conceptual framework of the relationship between individual and group acts in stock trading behavior are presented in Table . It can be observed that fourteen hypotheses represent the relations between the factors of the model. In the following sections, truth or falseness of those relations was tested.
Table 3. Relations of the research components
In the second stage, the statistical population included active investors in Tehran Stock Exchange to test the hypotheses. The sample was randomly selected by referring to the stock exchange market on several different days. The sample involved three groups. The first group encompassed investors relevant to finance and investment fields (accounting, management, and economics disciplines), the second one involved investors irrelevant to finance and investment fields (disciplines other than the first group disciplines), and the third one included investors without academic qualifications. Taking into account the normality of data, the first Pearson correlation coefficient between every two variables (based on the conceptual model) was tested by using SPSS software; then the causal relation of these variables was tested with the aid of SMART PLS software via structural equation modeling method. In the beginning, the volume of a statistical population in this study was unknown due to a large number of investors. Therefore, the number of statistical samples was estimated from the following relation:
In this relation, the most important parameter required to be estimated is S2 which is the same variance of the primary sample. To calculate S2, several questionnaires are distributed and the variance of the primary sample is calculated. Hence, the designed questionnaire was distributed among 20 experts considered in the first round. According to the statistical table, Z2α/2 would be 1.96. d takes the same fault level or equals 0.05. The sampling method in this research equaled 130 individuals. By estimating the variance of the primary sample at a 95% confidence interval, the volume of the sample was calculated from the following relation:
Z2α/2 = 1.96
d = 0.05
S2 = 0.085
6. Data analysis
Once data is collected, to analyze it by using structural equation modeling, it is first essential that construct validity is examined to determine whether the selected indices for measuring intended constructs have the necessary accuracy. To achieve this, conformity factor analysis (CFA) was used. Accordingly, the value of the construct reliability coefficient ranges from 0 to 1, and values higher than 0.7 are accepted but values lower than 0.6 are considered undesirable. The value of this coefficient for each factor is provided in Table , all of which are verified. The average variance extracted (AVE) was used to validate convergence, which showed a high correlation of indices of a construct in comparison to the correlation of indices of other constructs. The value of this coefficient also ranges from 0 to 1 and the values higher than 0.5 are accepted (Fornell & Larcker, Citation1981). The value of this coefficient for each factor is provided in Table . Cronbach’s Alpha coefficient is another factor whose value ranges from 0 to 1 and the values higher than 0.7 are accepted (Cronbach, Citation1951). The value of this coefficient for each factor is provided in Table . The results show the consistency and validity of these factors.
Table 4. Coefficients for consistency and validity of the factors
Fornell-Larcker measurement notes that the square root of AVEs of each construct should be higher than the correlation values of that construct with other constructs. The correlation values are among latent variables with current dimensions of the model in correlation section of latent variables in SMART PLS software and it is characterized by the main diagonal of the matrix equaling 1. Then, the existing values on the main diagonal of the matrix were replaced with the above square root of AVEs to provide Table .
Table 5. Fornell-Larcker table
As mentioned before, existing values on the main diagonal of the matrix should be higher than all existing values in the relevant column. In Table , i.e. Fornell-Larcker table, the value of the square root of AVEs of latent variables in this research which are placed on the main diagonal of the matrix, is higher than correlation value among them which are placed below and left to main diagonal. Hence, it can be stated that in the present study, the constructs (latent variables) in the model have more interaction with their own indices rather than other constructs. In other words, the divergent validity of the model is desirable. After drawing a conceptual model and primary PLS analysis, a conceptual model of this research with the standard coefficient can be observed in Figure .
Model modification: Path coefficients should be meaningful at least at confidence interval 0.05 (i.e., t is higher than 1.96) which can be observed by using the bootstrap technique on the model. For this purpose, the two-sided t-test is used. The hypotheses can be examined by using an internal model. The hypotheses are confirmed or disconfirmed by comparing the t-value calculated for each path coefficient. Thus, if the value of t-statistic is higher than the absolute value of 1.96, the path coefficient is meaningful at confidence interval %95 and if the value of t-statistic is higher than 2.58, the path coefficient is meaningful at confidence interval %99 (Momeni, 2013). According to Figure , the path coefficient of financial learning to financial capability (hypothesis 12) with the value of −0.070 and path coefficient of financial soundness to stock trading behavior (hypothesis 10) with the value of −0.045 is invalid. By omitting the path of financial soundness to stock trading behavior, the financial soundness variable would not practically be determinant of the relationship framework of individual and group acts in stock trading behavior. By omitting this variable from a conceptual framework, inevitably hypothesis 5 (path of financial advice to financial soundness) has no impact on the monitoring and assessment model either. The conceptual model was remodified and tested. The results can be observed in the following diagram Figure ().
7. Research findings
In the first stage of this research, the resulting data from Delphi loops were provided which modified in three steps by using exploratory factor analysis (EFA). Then, the conceptual model was designed based on 9 factors and 34 items which produced 14 hypotheses. Next, structural equation models of this research were examined and tested. In this stage, divergent validity and fitness of measurement models were first confirmed. Using partial least squares method, hypotheses 5, 10, and 12 out of 14 hypotheses were not accepted and the other 11 hypotheses were confirmed. Thus, it became evident that the developed method in areas of financial capability, financial capacity, word-of-mouth communication, mass media, financial consultation, stock trading behaviors, and personality are considered as causal conditions of relationship framework of individual and group acts which affect that process positively and meaningfully.
8. Concluding remarks
By concluding all matters presented in this research, it can be decided that the researcher succeeded in achieving research objectives and answering research questions. By reviewing research questions, it became clear that after examining literature review and classifying derived components being effective in designing the framework of this research, the researcher purposefully provided the conceptual model based on nine novel factors and studied their relations in the case study, i.e. Tehran Stock Exchange. It is necessary to explain that in this study, by using a new approach, the researcher tried to design and clarify the framework of individual and group acts in stock trading behavior which was well represented in the designed framework with the help of experts and professionals of the panel. The innovative aspect of the proposed model is the existence of a general attitude governing the model which takes it out of the scope of a mere project and translated it to an observational framework relative to individual and group acts. Because in addition to content and structural factors, it addressed the governing structure circumscribing the model (financial capability and financial capacity), social and cultural factors (mass media), and process factor (financial learning) and can be used and considered a procedure for structuring the factors associated with stock trading behavior. In other words, it can be concluded that the researcher designed and explained the framework according to the governing conditions. Considering the results of examining the fourteen hypotheses in this research using the regression model fitted to them, it was noted that 11 hypotheses out of 14 hypotheses were accepted at confidence interval 95%. The summary of existing relations and research findings is as follows. In literature, the theory of change, the conceptions of participation and planning are used to advance individual and group acts. The theory of change is defined in a long-term framework and a road map to provide necessary preconditions for change (Brest, Citation2010). The notion “financial advice” used in this research was also the driving factor of developing the framework of individual and group acts. As Kotter (Citation2012) stated, there are 8 necessary bases for changes including 1) forming a body of supportive factors; 2) sensing the urgency of changes; 3) communications; 4) developing a change vision; 5) empowering and capacity development in line with the vision; 6) generating short-term achievements; 7) consolidating developments and pursuing changes; 8) internalizing changes (Kotter, Citation2012). Therefore, financial advice, word-of-mouth communication, and personality are the most effective components in the process of changes. Moreover, among two components (financial capability and financial capacity) affecting the ultimate cause of this framework, i.e. stock trading behavior, financial capability is more effective since it has a more meaningful standard coefficient and value compared to financial capacity.
9. Recommendations and research limitations
To study the possible subjects in the formation process of stock trading behavior, the followings should be considered: 1) effectiveness-based attitude for creating or improving the systems of individual and group acts; 2) learning from countries that failed to implement the systems of financial learning (financial literacy); 3) coordination and coherence internalized with financial capability and financial capacity drives; 4) enhancing the participation of mass media to form better stock trading behavior; 5) identifying and emphasizing the excellent performance examples of planning financial learning and financial capability and educational monitoring and assessment techniques.
In his work, the researcher faced several limitations including inaccessibility to some foreign scientific websites and facing various obstacles in obtaining references, the time-intensiveness of receiving some valid first-hand international articles that naturally made the time frame of collecting and integrating theoretical literature longer in the research process, hesitation of some experts to accept the emerging concepts such as the factors existing in conceptual model of the relationship framework of individual and group act in stock trading behavior, and the lack of sponsorship by administrative agencies to conduct this research.
Additional information
Funding
Notes on contributors
Saeideh Nosratabadi
Saeideh Nosratabadi is PhD student in the department of accounting at the Islamic Azad University Sirjan Branch in Iran. Her main research interests are asset pricing, behavioral finance, and auditing.
Zadollah Fathi
Zadollah Fathi is an assistant Prof of accounting at the Islamic Azad University Tehran Branch. His research interests relate to the risk management, asset pricing, and investors’ behavior. He has published many research papers in the field of accounting in leading international journals.
Abbas Shoul
Abbas Shoul, is an assistant Prof of accounting at the Vali-e-Asr University in Iran. His research interest includes investor sentiment, accounting information and stock price. He has been doing research on the behavioral finance in Iran.
References
- Alessie, R, Van Rooij, M, & Lusardi, A. (2011). Financial literacy and retirement preparation in the netherlands. Journal Of Pension Economics and Finance, 10(4), 527–19.
- Badri, A., & Ezabadi, B. (2015). Investor type trading behavior and trade performance in Tehran stock exchange. Financial Research Journal, 17(1), 21–38. https://scholar.google.com/scholarhl=fa&as_sdt=0%2C5&q=+Investor+type+trading+behavior+and+trade+performance+in+Tehran+Stock+Exchange.&btnG=
- Baghdar Eram, F. (2011). Investigation of behavioral finance factors affecting investors’ decision making in the Tehran stock exchange (M.Sc. Thesis). Islamic Azad University. Central Tehran Branch.
- Barber, B. M., & Odean, T. (2001). Boys will be boys: Gender, overconfidence, and common stock investment. Quarterly Journal of Economics, 116(1), 261–292. doi:10.1162/003355301556400
- Barber, B. M., & Odean, T. (2002). Online investors: Do the slow die first? Review of Financial Studies, 15(2), 455–488. doi:10.1093/rfs/15.2.455
- Barber, B. M., & Odean, T. (2008). All that glitters: The effect of attention and news on the buying behavior of individual and institutional investors. Review of Financial Studies, 21(2), 785–818. doi:10.1093/rfs/hhm079
- Bazzaz Zadeh Sorbate, H., Malekian, E., & Kamyabi, Y. (2016). Market reaction to intangible information: The modifying role of institutional investors. Quarterly Journal of Financial Accounting, 8(31), 1–29.
- Brest, P. (2010). The power of theories of change. Stanford social innovation review. Spring 2010.
- Brown, J. R., Zoran, I., Smith, P. A., & Weisbenner, S. (2008). Neighbors matter: Causal community effects and stock market participation. The Journal of Finance, 63(3), 1509–1531. doi:10.1111/j.1540-6261.2008.01364.x
- Campbell, J. Y. (2006). Household finance. The Journal of Finance, 61(4), 1553–1604. doi:10.1111/j.1540-6261.2006.00883.x
- Campbell, J. Y., & Viceira, L. M. (2003). Strategic asset allocation – Portfolio choice for long-term investors. New York: Oxford University Press.
- Campbell, John Y., Hilscher., Jens Dietrich, & Szilagyi, Jan. (2011). Predicting financial distress and the performance of distressed stocks. Journal of Investment Management, 9(2), 14-34.
- Chang, E. C, Cheng, J. W, & Khorana, A. (2000). An examination of herd behavior in equity markets: an international perspective. Journal Of Banking And Finance, 24(10), 1651-1679.
- Changwony, F. K., Campbell, K., & Tabner, I. T. (2014). Social engagement and stock market participation. Review of Finance, 1–50, rft059.
- Conlin, A., Kyröläinen, P., Kaakinen, M., Järvelin, M., Perttunen, J., & Sventog, R. (2015). Personality traits and stock market participation. Journal of Empirical Finance, 33, 34–50. doi:10.1016/j.jempfin.2015.06.001
- Costa, P. T., & McCrae, R. R. (1989). The NEO-PI/NEO-FFI manual supplement. Odessa, FL: Psychological Assessment Resources, Inc.
- Cronbach, L. J. (1951). Coefficient alpha and the internal structure of tests. Psychometrika, 16(3), 297-334. doi: 10.1007/bf02310555
- Daniel, K., Hirshleifer, D., & Subrahmanyam, A. (1998). Investor psychology and security market under- and overreactions. The Journal of Finance, 53(6), 1839–1885. doi:10.1111/0022-1082.00077
- Dhar, R., Goetzmann, W. N., & Zhu, N. (2004). The impact of clientele changes evidence from stock splits. Yale ICF Working Paper No. 03–14.
- Duflo, E, & Saez, E. (2002). Participation and investment decisions in a retirement plan: the influence of colleagues' choices. Journal Of Public Economics, 85(1), 121–148.
- Duflo, E., & Saez, E. (2003). The role of information and social interactions in retirement plan decisions: Evidence from a randomized experiment. The Quarterly Journal of Public Economics, 118(1), 815–842. doi:10.1162/00335530360698432
- Durand, R. B, Newby, R, Peggs, L, & Siekierka, M. (2013). Personality. The Journal Of Behavioral Finance, 14(2), 116–133.
- Durand, R. B., Newby, R., & Sanghani, J. (2008). An intimate portrait of the individual investor. The Journal of Behavioral Finance, 9(4), 193–208. doi:10.1080/15427560802341020
- Durand, R. B, Newby, R, Tant, K, & Trepongkaruna, S. (2013). Overconfidence, overreaction and personality. Review Of Behavioral Finance, 5(2), 104–133.
- Epstein, L. G., & Schneider, M. (2008). Ambiguity, information quality, and asset pricing. The Journal of Finance, 63(1), 197–228. doi:10.1111/j.1540-6261.2008.01314.x
- Feng, L., & Seasholes, M. S. (2004). Correlated trading and location. The Journal of Finance, 59(5), 2117–2144. doi:10.1111/j.1540-6261.2004.00694.x
- Fischer, R., & Gerhardt, R. (2007). The missing link between investors and portfolios: Introducing financial advice. In URL: http://ssrn.com/abstract Vol. 967374).
- Fornell, C, & Larcker, D. F. (1981). Structural equation models with unobservable variables and measurement error: algebra and statistics. Journal Of Marketing Research, 18(3), 382–388.
- Fréchette, G. R., Schotter, A., & Treviño, I. (2014). Personality, information acquisition and choice under uncertainty: An experimental study. Available at SSRN no. 1935707.
- Fung, L., & Durand, R. B. (2014). Personality traits. In H. K. Baker & V. Ricciardi (Eds.), Investor behavior: The psychology of financial planning and investing (pp. 99–155). Hobo- ken, New Jersey: John Wiley & Sons, Inc.
- Gerhardt, R., & Hackethal, A. (2009). The influence of financial advisors on household portfolios: A study on private investors switching to financial advice. Available at SSRN1343607.
- Gervais, S., & Odean, T. (2001). Learning to be overconfident. Review of Financial Studies, 14(1), 1–27. doi:10.1093/rfs/14.1.1
- Ghorbani Pahlevan, H., & Bashashi, Z. (2017). The impact of investors’ personality traits on information acquisition and trading behavior of investors in the Zanjan stock exchange. Annual Conference of New Paradigms of Management in Intelligence Field, Tehran. Permanent Secretariat of Conference, University of Tehran.
- Goetzmann, W. N., & Kumar, A. (2008). Equity portfolio diversification. Review of Finance, 12(3), 433–463. doi:10.1093/rof/rfn005
- Graham, J. R., Harvey, C. R., & Huang, H. (2009). Investor competence, trading frequency, and home bias. Management Science, 55(7), 1094–1106. doi:10.1287/mnsc.1090.1009
- Grinblatt, M., & Keloharju, M. (2009). Sensation seeking, overconfidence, and trading activity. The Journal of Finance, 64(2), 549–578. doi:10.1111/j.1540-6261.2009.01443.x
- Guiso, L., & Jappelli, T. (2006). Information acquisition and portfolio performance. Centre for Studies in Economics and Finance Working Paper No. 167.
- Hackethal, A., Haliassos, M., & Jappelli, T. (2012). Financial advisors: A case of babysitters? Journal of Banking & Finance, 36(2), 509–524. doi:10.1016/j.jbankfin.2011.08.008
- Haigh, M. S., & List, J. A. (2005). Do professional traders exhibit myopic loss aversion? An experimental analysis. The Journal of Finance, 60(1), 523–534. doi:10.1111/j.1540-6261.2005.00737.x
- Haratian, H., & Faez, A. (2015). Designing a mental model of financial planning to give financial advice. The Third International Conference of Applied Studies in Management and Accounting, Tehran, University of Shahid Beheshti.
- Heath, C., & Tversky, A. (1991). Preference and belief: Ambiguity and competence in choice under uncertainty. Journal of Risk and Uncertainty, 4(1), 5–28. doi:10.1007/BF00057884
- Heimer, R. Z. (2014). Friends do let friends buy stocks actively. Journal of Economic Behavior & Organization, 107, 527–540. doi:10.1016/j.jebo.2014.04.019
- Heinström, J. (2003). Five personality dimensions and their influence on information behavior. Information Research, 9, 1.
- Hong, H., Kubik, J. D., & Stein, J. C. (2004). Social interaction and stock-market participation. The Journal of Finance, 59(1), 137–163. doi:10.1111/j.1540-6261.2004.00629.x
- Hong, H., Kubik, J. D., & Stein, J. C. (2005). Thy Neighbor’s portfolio: Word-of-mouth effect in the holdings and trades of money managers. The Journal of Finance, 60(6), 2801–2824. doi:10.1111/j.1540-6261.2005.00817.x
- Ivković, Z., & Weisbenner, S. (2007). Information diffusion effects in individual Investors’ common stock purchases: Covet thy neighbors’ investment choices. Review of Financial Studies, 20(4), 1327–1357. doi:10.1093/revfin/hhm009
- Jahangiri Rad, M., Marfoo, M., & Salimi, M. (2014). Studying herding behavior of investors in the Tehran stock exchange. Empirical Studies of Financial Accounting, 11(42), 139–156.
- Jamshidian, M. (2012). Behavioral factors affecting the decision making of active individual investors in the Tehran stock exchange (M.Sc. Thesis). The University of Allameh Tabatabaei.
- Kahneman, D., Knetsch, J. L., & Thaler, R. H. (1991). Anomalies: The endowment effect, loss aversion, and status quo bias. The Journal of Economic Perspectives, 5(1), 193–206. doi:10.1257/jep.5.1.193
- Karabulut, Y. (2013). Financial advice: An improvement for worse? Available at SSRN 1710634.
- Kotter, J. (2012). The 8-step process for leading change. Zugriff is 9.03.2013. Verfügbar unter:http://www.kotterinternational.com/ourprinciples/changesteps.
- Kramer, M. M. (2012). Financial advice and individual investor portfolio performance. Financial Management, 41(2), 395–428. doi:10.1111/fima.2012.41.issue-2
- Li, G. (2014). Information sharing and stock market participation: Evidence from extended families. The Review of Economics and Statistics, 96(1), 151–160. doi:10.1162/REST_a_00301
- List, J. A. (2003). Does Market Experience Eliminate Market Anomalies? The Quarterly Journal Of Economics, 118(1), 41–71.
- Lusardi, A., & Mitchell, O. S. (2007). Baby boomer retirement security: The roles of planning, financial literacy, and housing wealth. Journal of Monetary Economics, 54(1), 205–224. doi:10.1016/j.jmoneco.2006.12.001
- Lusardi, A., & Mitchell, O. S. (2011a). Financial Literacy and Planning: Implications for Retirement Wellbeing. National Bureau of Economic Research Working Paper No. 17078. Available at nber.org/papers/w17078
- Lusardi, A, & Mitchell, O. S. (2011b). Financial literacy around the world: an overview. Journal Of Pension Economics and Finance, 10(4), 497-508. doi: http://dx.doi.org/10.1017/S1474747211000448
- Madrian, B. C., & Shea, D. F. (2000). The power of suggestion: Inertia in 401 (k) participation and savings behavior. National bureau of economic research (No. w7682).
- Ng, L, & Wu, F. (2010). Peer effects in the trading decisions of individual investors. Financial Management, 39(2), 807–831.
- Odean, T. (1998). Volume, volatility, price, and profit when all traders are above average. The Journal of Finance, 53(6), 1887–1934. doi:10.1111/0022-1082.00078
- Pitters, J., & Oberlechner, T. (2014). The psychology of trading and investing. In H. K. Baker & V. Ricciardi (Eds.), Investor behavior: The psychology of financial planning and investing (pp. 459–476). Hoboken, New Jersey: John Wiley & Sons, Inc.
- Polkovnichenko, V. (2005). Household portfolio diversification: A case for rank-dependent preferences. Review of Financial Studies, 18(4), 1467–1502. doi:10.1093/rfs/hhi033
- Pompian, M. M. (2012). Behavioral finance and investor types. Managing behavior to make better investment decisions. Hoboken, NJ: John Wiley & Sons.
- Pompian, M. M., & Longo, J. M. (2004). A new paradigm for practical application of behavioral finance: Creating investment programs based on personality type and gender to produce better investment results. The Journal of Wealth Management, 7(2), 9–15. doi:10.3905/jwm.2004.434561
- Pool, V. K., Stoffman, N., & Yonker, S. E. (2015). The people in your neighborhood: Social interactions and mutual fund portfolios. The Journal of Finance, 70(6), 2679–2732. doi:10.1111/jofi.12208
- Rashes, M. S. (2001). Massively confused investors making conspicuously ignorant choices (MCI–MCIC). The Journal of Finance, 56(5), 1911–1927. doi:10.1111/0022-1082.00394
- Saedi, R., & Mokhtarian, O. (2009). Investigation of the effective factors in investors’ decision-making in the Tehran stock exchange. Quarterly Journal of Financial Accounting, 1(3), 147–168.
- Shapira, Z., & Venezia, I. (2001). Patterns of the behavior of professionally managed and independent investors. Journal of Banking & Finance, 25(8), 1573–1587. doi:10.1016/S0378-4266(00)00139-4
- Sobouti, M., & Shamakhi, M. (2016). The impact of behavioral finance factors on the investors’ returns on the Tehran stock exchange. The Fifth International Conference on New Achievements of Humanities and Management, Shiraz.
- Tauni, M. Z., Hong, X. F., & Amjad, I. (2017). the role of financial advice and word-of-mouth communication on the association between investor personality and stock trading behavior: Evidence from the Chinese stock market. Personality and Individual Differences Journal, 108(2017), 55–65. doi:10.1016/j.paid.2016.11.048
- Toghiani, Z. (2014). Investigating the impact of social factors on investors’ trading behavior in the Tehran stock exchange (M.Sc. Thesis). Islamic Azad University, Central Tehran Branch.
- Van Rooij, M. C., Lusardi, A., & Alessie, R. J. (2011). Financial literacy and retirement planning in the Netherlands. Journal of Economic Psychology, 32(4), 593–608. doi:10.1016/j.joep.2011.02.004
- Van Witteloostuijin, A., & Muehlfeld, K. (2008). Trader’s personality and trading performance: A framework and financial market experiment (no. 8–28). Working Paper. Utrecht School of Economics, Utrecht University Retrieved from http://econpapers.repec.org/paper/usetkiwps/0828.htm
- Walid, M. A. (2014). The trading patterns and performance of individual vis-à-vis institutional investors in the qatar exchange. Review Of Accounting and Finance, 13(1), 24-42.
- Yang, S., Hsu, Y., & Tu., C. (2012). How do traders influence the investor’s confidence and trading volume? A dyad study in the futures market. Emerging Markets Finance and Trade, 48(Sup 3), 23–34. doi:10.2753/REE1540-496X4805S302