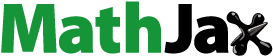
Abstract
This work adds to the debate on the determinants of the demand for life insurance providing new insights for the unexplored Romanian market, and the new perspective of spatial econometric analysis. Results show that there are spatial interactions between the Romanian counties regarding the life insurance density, positively and significantly conditioned by the level of the local human development. The latter is included in the analysis in the form of the Local Human Development Index (LHDI) developed and computed for the Romanian counties and update for this study. The spatial econometrics regression models emphasize significant diffusion and contagion processes on the local Romanian life insurance market. We also identified a significant positive impact of income, urbanization and medical staff over the life insurance demand.
PUBLIC INTEREST STATEMENT
This empirical study, conducted at sub-national level, emphasizes the role of human development upon the evolution of the life insurance market, in the presence of spatial diffusion effects among regions. Sub-national approaches have some peculiarities due to which they deserve specific studies and attention. In most cases, the circulation of goods, people, capital and information is much higher within countries than between countries. Consequently, the effects of spatial diffusion and contagion are far more pronounced within a nation. This study uses data for the 42 Romanian counties in 2014. We found that the Local Human Development Index positively influences the demand for life insurance. Income, urbanization and medical sector are significant as control factors. Spatial analyses emphasize the diffusion effects of the financial behaviour from the North-West counties, geographically closer to Central Europe, to the South-Eastern ones, closer to the Balkan countries.
1. Introduction
As financial intermediaries with long investment horizons, life insurance companies can contribute to the provision of long-term finance and can create a general framework for a more effective risk management. The role of life insurers as providers of financial services is increased in developed countries, but their role and the consumption of life insurance still remain low in emerging countries—Beck and Webb (Citation2003).
Our research focuses on the Romanian life insurance market, which in the last 20 years experienced periods of upward and downward trends. After 45 years of communism and over 25 years of transitional economy, Romania can be considered an interesting study case due to the fact that is a country of socio-economic and financial contrasts, related to the opportunities of development for its life insurance market.
The Romanian life insurance densityFootnote1 experiences very modest levels compared with those for other European countries. According to Insurance Europe (Citation2015), from 32 European countries, Romania had the lowest value of life insurance density in the year 2004 (6 Euros/inhabitant for Romania compared to 3643 Euros/inhabitant for Switzerland), the gap with developed countries remaining almost the same in the year 2015 (17 Euros/inhabitant for Romania compared to 3562 Euros/inhabitant for Finland).
Romania is one of the most polarized countries in the European Union in terms of income (Busega, Citation2016). The disparities for Romania can be emphasized also by using a composite index of regional inequality (Goschin, Constantin, Roman, & Ileanu, Citation2007). This phenomenon of heterogeneity is present both at the macro-regional and at the intra-regional level (Cristea et al., Citation2017). Similar conclusions regarding the disparities between the Romanian counties in terms of productivity, level of education and specialization can be found in the study of Dachin and Popa (Citation2011). The roots of these disparities in Romania come from the communist period, when, in a centralized way, authorities decided to create mono-industrial regions.
Previous research in the field of life insurance evaluates the determinants of life insurance either on a micro-scale, at individual level, or at the macroeconomic, national scale. Different variables are assessed, but all having a core idea—the level of development (either at individual or at national level; Asongu and Odhiambo, Citation2019; Lee, Chong, & Sia, Citation2018, Emamgholipour, Arab, & Mohajerzadeh, Citation2017 or Chang & Lee, Citation2012). The novelty of this research if the fact that the analysis is conducted at county level, thing that allows for a better evaluation of the local specificities in a wider national environment. Another important aspect is that we introduce a composite way to measure the level of development locally, through the use and update of the Local Human Development Index (LHDI) (construction principles and method presented in Burduja et al., Citation2014). Additionally, even though most of the previous studies rely on spatial data, assessment is not based on such evaluation methods, spatial interactions being ignored by classical methodologies. By using Spatial Econometrics methods, we are able to account for neighbourhood and spatial effects, such as contagion and diffusion on the Romanian county life insurance market. We point out that the local level of human development significantly conditions the spatial diffusion process on this market in Romania.
As stated above, in order to express the differences across the Romanian counties regarding the available resources and the available conditions for education and health care, we use in our study the Local Human Development Index. We emphasize that not only the economic growth, but people and their abilities, are also important criteria for assessing the degree of development of a country.
We analyze the influence of the Local Human Development Index on the consumption of life insurance products across the 42 Romanian counties. The life insurance consumption is expressed through life insurance density. Our study tests the contagion and diffusion processes encountered on the Romanian life insurance market at the county level by using a spatial approach. We started from the hypothesis that spatial interactions should exist on the life insurance market between the Romanian counties. We presume that high internal migration will lead to an increase in the exchange of information between the Romanian regions with different levels of development, leading to a significant life insurance market development. The main reason is that we expect diffusion and contagion processes to take place among neighbouring Romanian regions.
We estimated a spatial regression model between the life insurance density and LHDI. The spatial models are able to account for neighbouring effects, which are very intense in a population like the counties of a state. Due to the fact that they incorporate the neighbouring scheme (expressed through the spatial weights matrix), they allow for evaluating processes like contagion and diffusion and the transmission channels for them. Additionally, aspects that condition such processes are put into light through such methods.
As control factors, we considered income, unemployment, urbanization, criminality, cultural vitality and medical staff.
We prove that life insurance density is positively related to the human development index computed at the local level, even in the presence of control factors. Also, significant diffusion processes take place between the Romanian counties in terms of life insurance.
The reminder of the paper is the following: Section 2 reviews the literature and presents the working hypothesis, Section 3 presents the data and methodology, Section 4 contains the results and discussions and Section 4 presents the conclusions and the policy implications.
2. Literature review and hypothesis development
2.1. Literature review
A large literature is devoted to the analysis of the relationship between the demand for life insurance and a wide range of country- and individual-level determinants. The dependent variable for these studies has been either the life insurance density (number of euros spent annually on life insurance per capita) or the insurance penetration (life premium volume divided by GDP).
The studies using macroeconomic data, as well as those using household data, show that life insurance consumption is positively related to income.Footnote2 At national level income is usually measured by the real gross domestic product (GDP)/capita, while at individual-level income is measured by personal earnings (wage, salary). Education is also an important determinant for the life insurance consumption, and is usually expressed through the percentage of people with university studies in the total population. In the literature the results are mixed, the most recent studies leading to no significant link between education and life insurance demand.Footnote3 The impact of employment on the life insurance purchasing is also considered in the literature, the results showing a significant positive relationship.Footnote4 Life expectancy has an ambiguous effect over the life insurance purchasing because of the three components sold as life insurance: mortality products, annuities and savings. Still, few studies found a positive correlation between life expectancy and life insurance demand.Footnote5 Urbanization has a positive effect over the life insurance demand mainly in emerging markets and in Asia,Footnote6 the factor being insignificant in developed countries and in Europe.Footnote7
In a study using cross-sectional household data from the surveys for 1952 and 1961, conducted by the Survey Research Center of the University of Michigan, Hammond, Houston, and Melander (Citation1967) show that income, education and occupation have a positive significant influence over the life insurance demand. They also subdivided the data on the basis of income into three groups of equal size, showing that as the incomes of households increase, they spend proportionately less on life insurance premiums. Also, life insurances are the most attractive for the middle-income level group. In a survey of young married couples for the period 1968–1971, Anderson and Nevin (Citation1975) found that the current income impacts life insurance demand. The impact is positive for low and high-income families and negative for mid-income families. Also, this study reveals a negative relationship between education and life insurance demand.
Truett and Truett (Citation1990) show, in a time series study for US and Mexico population, that education and income positively impact the life insurance demand. They also conclude that Mexicans have a higher income elasticity of the demand for life insurance than Americans.
Browne and Kim (Citation1993) analyse, in a cross-sectional study for 45 countries, the life insurance in force, for the years 1987 and 1980 and the premiums for the year 1987 as proxies for the life insurance consumption. They find, using ordinary least squares, a positive significant influence of national income, education, inflation and government spending on social security over the life insurance demand. On the contrary, Auerbach and Kotlikoff (Citation1989) point out the negative effect of education over the life insurance demand also.
Beck and Webb (Citation2003), using a panel data for 68 economies in 1961–2000, find that income per capita along with cultural (religion) and institutional factors are robust predictors of life insurance demand. They also explain that education appears not to have any robust influence on the life insurance consumption because the period of dependency is increasing. Beck and Webb (Citation2003) found that life expectancy has no robust association with life insurance consumption. The ambiguous correlation with the demand for life insurance comes from the fact that they considered three components of life insurance products with different mechanisms.
Dragos (Citation2014) shows in a panel data study for emerging countries from Central and Eastern Europe and Asia, for the period 2001–2011, that the tertiary education is not an appropriate proxy for risk aversion because of the complexity of life insurance products. Income is the main determinant of life insurance demand for both Asia and CEE, while urbanization has a positive influence over the life insurance purchasing only in emerging Asia.
In a study on 10 ASEAN countries for the period 1990–2013, Lee et al. (Citation2018) show that income, young dependency ratio and tertiary education influence the life insurance consumption, while inflation, urbanisation, life expectancy and secondary education exert no significant influence.
Asongu and Odhiambo (Citation2019) investigate on a panel of 48 African countries, for the period 2004–2014, the role of insurance on economic development. They find that increasing life insurance premiums with at least 4,1% of GDP, positively affects economic prosperity.
Many of the above-mentioned studies use national data, either panel or cross-section. But they all treat the subject as in the case of survey samples, using classical methodological approaches. Spatial interdependencies and the effects related to them are not assessed in any of these researches. When spatial units such as countries are considered, there are neighbouring effects that impact the relationships between variables and specific processes take place (Zangger, Citation2019). Additionally, spatial data are characterized by high heterogeneity, which classical approaches cannot treat. Spatial method usage is traditional in other sectors of the real life (criminality, European integration, trade, etc.; see, for ex. (Ciccarelli & Elhorst, Citation2018) for the Italian cigarette market; (Alexa, Cismas, Rus, & Pop Silaghi, Citation2019) for regional convergence in the European Union versus competitiveness; (Lopez-Bazo, Del Barrio, & Artis, Citation2002, Citation2005) for regional unemployment assessment; or (Maguain, & Fréret, Citation2013) for welfare spending).
The need for using spatial econometrics methods in the financial sector, in general, and in insurance, in particular, has been recently pointed out. For example, Millo and Carmeci (Citation2015) used a panel approach with spatial effects to analyze the demand for life insurance in Italy over the period 1996–2001. They found a positive influence of income and young dependency ratio over the life insurance purchasing and a negative impact of education. Spatial convergence on the European Union’s life insurance market and the factors conditioning it is assessed by Mare, Dragos, Dragota, Muresan, and Urean (Citation2016). In this study, they highlight the need for using the spatial approach in such studies, as other methodologies are not able to properly evaluate this type of interactions.
2.2. Hypothesis
The academic literature regarding the life insurance demand is abundant at cross-national level, but at county-level in exchange the literature is limited (Millo & Carmeci Citation2015).
However, these sub-national approaches have some peculiarities due to which they deserve specific studies. First of all, the variability of the phenomenon is smaller from one territorial unit to another than in the case of the countries, so the causal relationships are harder to highlight. Second, some macroeconomic variables (such as inflation) are constant from one sub-national territorial unit to another, although they vary widely from country to country. For other variables, there are only data at country level, although it varies within its territory (e.g. Freedom from Corruption, cultural variables—Hoffstede, etc.), making them unusable in subnational studies. Thirdly, in most cases, the circulation of goods, people, capital and information is much higher within countries than between countries. This makes, for many phenomena, the effects of spatial diffusion and contagion far more pronounced within a nation.
Starting from the existing literature and taking into account the specificities noted in subnational studies, we formulate the following hypotheses:
H1: The human development of the society positively influence the Romanian life insurance consumption at county level
The literature using the Human Development Index (HDI) as determinant of the life insurance demand is rather scarce: the studies of Outreville (Citation1996) and Beck and Webb (Citation2003) do not find a robust relationship between the HDI and the proxies for life insurance consumption, while the studies of Truett and Truett (Citation1990), Browne and Kim (Citation1993) and Li, Moshirian, Nguyen, and Wee (Citation2007) find a positive and significant correlation between the demand for life insurance and the HDI. These conflicting results may come from the fact that the study of Beck and Webb (Citation2003) considers two different types of life insurance policies: saving products (annuities) as well as mortality risk products. Life expectancy is differently related to those financial products. In our study, we take into consideration only the mortality risk products.Emamgholipour et al. (Citation2017), using a panel data approach for the period 2004–2012, show that in MENA countries the effect of HDI on life insurance demand in significant and positive. This means that improved life standards, educational status and life expectancy will increase the life insurance consumption.
Derived from the HDI, the Local Human Development Index (Burduja et al., Citation2014) examines, at the county level, some basic dimensions, namely, health, overall knowledge level of adults and the standard of living.
Our study tests if the four key dimensions for the local development—human capital,Footnote8 health capital,Footnote9 vital capitalFootnote10 and material capitalFootnote11—aggregated into the Local Human Development Index, positively influence the Romanian life insurance consumption at the county level.
H2: Spatial differences between counties in terms of level of development may significantly impact the diffusion and contagion on the life insurance market
There are high differences between regions in terms of the level of development. Consequently, such an analysis must be conducted by taking into account spatial differences and arrangements that exist, as these may significantly impact the development of the life insurance market in a region or another. As high interactions exist between Romanian counties, diffusion and contagion are to be found on the life insurance market. This working hypothesis is intended to test if these processes are conditioned by the level of human development. To the best of our knowledge, there is no such study that combines spatial interactions with the determinants of life insurance at micro-level. Spatial analyses have been conducted, but for the non-life insurance sector. In a cross-sectional study for Italian data, Guiso and Jappelli (Citation1998) consider the spatial heterogeneity between sub-regions, and find (1) a positive relation with the expected income, the levels of education and urbanization, and with the professional status of self-employment, (2) a non-linear correlation with age, and (3) no relation with family size. A recent study of Millo and Carmeci (Citation2011) deepens the analysis of these regional disparities and their impact on the size of the non-compulsory non-life insurance market, using the NUTS3 aggregation level. They confirm the positive relation between the non-life insurance density and the levels of income and wealth, and also with the level of trust and the density of insurance agencies.
Using the spatial lag model, Cracolici, Cuffaro, and Nijkamp (Citation2007) prove that the Italian labour market in 2003 is very polarized in terms of unemployment rates. They find a neighbouring effect, justified by the positive spatial autocorrelation, which means that regions with low and, respectively, high values of unemployment rates tend to cluster in space. These situations are explained by the clustering of the activities (Cracolici et al., Citation2007; Overman & Puga, Citation2002). In our study, we consider the unemployment rate as a control factor in order to see if there are neighbouring effects for the life insurance density at the county level.
3. Data and methodology
3.1. Data
The endogenous variable is life insurance density at the county level (LDENSITY), which is a proxy for the size of the regional life insurance market. Life insurance density is defined as premiums per capita, showing how much each individual from a county spends on insurance, on average.
3.1.1. Local human development index (LHDI)
Composite indexes are more and more used in socio-economic applications. For example, there are cultural indexes (Hofstede), institutional indexes (World Governance Indicators), environmental (Environmental Protection Index), etc. They measure more complex phenomena, starting from specific aspects expressed through quantitative or qualitative variables. There is a wide range of aggregation methods used for composite indexes that can be subjective. Practically, such an index is validated only when it turns out statistically significant in regression models that assess causality relationships in the socio-economic field for which they were constructed.
The Local Human Development Index (LHDI)Footnote12 is used as an exogenous county-level variable to see how contextual characteristics relate to perceptions over the specificities of the life insurance market. For the Romanian case, the LHDI (Burduja et al., Citation2014) takes into account four key dimensions for the local development: (1) human capital (expressed through the education stock), (2) health capital (expressed through the life expectancy at birth), (3) vital capital (expressed through the medium age of adult population of 18 years old and over), and (4) material capital that stands for the standard of living (expressed through the living floor area by house, the private cars to 1000 inhabitants and the distribution of gas for household consumption by locality inhabitant). This index was developed for the World Bank and presented in Burduja et al. (Citation2014). But it was computed only for the census years. Following the same methodology, we updated the LHDI for the year 2014 and used it in the present analysis. The four main dimensions, along with the final form of the index are aggregated based on the geometrical mean.
To evaluate the robustness of the results, we employed several control variables derived from the field’s literature: income, urbanization, unemployment, criminality, medical staff (doctors) and cultural vitality (spectators). The data source for them is the Romanian National Institute of Statistics (Citation2015) and PRIMM Insurance & Pensions Review. (Citation2015) for life insurance density.
The variables used are for 2014. This is the last year for which information related to life insurance contracts is available at the county level in Romania. Administrative structural changes occurred and data were not collected anymore. Only nationally aggregated information is available nowadays. Consequently, this is the newest data that could be obtained. But this is not necessarily a drawback of the research, as the main goal is to emphasize transmission channels and the aspects conditioning the intensity of the diffusion and contagion processes on the local life insurance market. The study could be easily replicated to any country to evaluate such processes, if required data are available.
A descriptive analysis of the variables used in the spatial analysis is provided in Table .
Table 1. Descriptive analysis of the variables used in the spatial analysis
3.2. Methodology
We tested the relationship between the level of the local human development index (LHDI) and the life insurance density (LDENSITY) as a proxy for the size of the regional life insurance market.
A major drawback of the field’s literature is that most of the studies, even though they are applied to spatial units of different levels, do not take into account spatiality and neighbouring effects (see, for example, Dragos, Citation2014; Dragos et al, Citation2017). To encompass these effects into the analysis we opted for Spatial Econometrics methods.
Data were descriptively analysed based on maps. Out of the types constructed, we present in the Results and Discussion section the ones based on quartiles (for LHDI and the Life Insurance Density) and the smoothed spatial rates one. The latter is constructed to assess the relationship between LHDI and income. The simple ratio between the two variables is spatially smoothed using the spatial weights matrix, in order to treat variability that may exist in spatial data. This type of map can also emphasize clusterization or polarization processes, along with diffusion patterns.
In order to run the final regression analysis, the neighbourhood scheme must be established through the spatial weights matrix. The latter is specific to a spatial population and is the base for the assessment of our working hypothesis. For the present analysis, the contiguity matrix in the queen form was the most appropriate, as all the counties have neighbours and there is no island in the spatial population.
To test our working assumptions, spatial econometrics modelling was applied. Validation appears, on one hand, if the coefficient of LHDI is positive and statistically significant and, on the other, if the classical OLS model is rejected and there is the need of model re-specification with spatial components. We started from the following OLS model (stated in a matrix form) with the queen spatial weights matrix attached:
EquationEquation (1)(1)
(1) is then assessed in terms of spatial dependences, based on the Lagrange Multipliers (LM) and the Moran’s I statistic for the errors in the model. Spatial problems were emphasized in the form of spatially correlated errors (Moran’s I Z_I = I-E(I)/sqrt(V(I)) = 2.51, p-value = 0.012 and LM(error) = 4.27, p-value = 0.039—see results in Table ). Thus, the spatial component was introduced in the model and EquationEquation (1)
(1)
(1) is respecified in the spatial error correction form (SEM). EquationEquation (1)
(1)
(1) became:
Table 2. Spatial regression analysis—estimations for EquationEquations (1)(1)
(1) and Equation(2)
(2)
(2)
Where:
LDENSITY is the 42 × 1 vector of the endogenous variable,
LHDI is the 42 × 1 vector of observations of the regressor,
WLDENSITY is the spatial lag of the dependent variable,
α, β are the regression coefficients,
ρ is the 42 × 1 vector of spatially autocorrelated residuals and W ρ is the spatial lag of the error term,
λ is the autoregressive coefficient of the spatially lagged error term,
μ is the final error term, which is iid.
Relationships found were then tested for robustness using post-estimation validation procedures and several control variables, described in Table .
All required transformations were applied upon the variables (natural log, rates, etc.). Analyses were run in GeoDa 1.8.16.4 and GeoDaSpace, on the 42 Romanian counties.
4. Results and discussions
The study focuses on the spatial evaluation of the relationship between the human development and the size of the life insurance market. According to the descriptive analysis based on maps (see the quartile maps in Figures and ), clusterization processes take place as regards the above-mentioned indicators. In Figure it can be observed that counties from the Centre region have higher life insurance density in comparison with those from the Eastern or Southern part of Romania, with few exceptions.
In terms of the local human development level, a clusterization process in the form of centre—periphery can be identified. Still, the Eastern and Southern parts of the country have the lowest scores for this variable.
The coefficient of LHDI is positive and highly significant even from the OLS approach estimated by EquationEquation (1)(1)
(1) (see Table ). But spatial diagnosis tests reveal the need for spatial effects in the model in the form of spatial error correction. Through this respecification (EquationEquation (2)
(2)
(2) ), LHDI remains the same—positive and highly significant. Results are in accordance with the two working assumptions. In order to finally validate H1 and H2, we test the robustness of this relationship between LHDI and life insurance density in the presence of control factors.
The introduction of control variables in the spatial regression model (Table ) does not alter the sign and the significance of LHDI. We can conclude that H1 is accepted, the level of local human development measured for the Romanian counties positively influences the development of the life insurance market. This is an expected result, as more developed areas have higher education stocks, higher access to information, higher longevity of the population, more private cars per 1000 inhabitants, in other words, a higher standard of living. Additionally, individuals from more developed regions are expected to be more opened towards intricate products such as life insurances, due to the fact that these policies are quite expensive. The OLS model is rejected, and the relationship needs to be re-specified by introducing the spatial error component in the form of the spatial error correction model (SEM). Taking into account the fact that the spatial error coefficient—λ is also significant and positive (in both Tables and ), the diffusion process conditioned by the level of human development is confirmed. Through these results, we validate H2.
Table 3. Spatial regression analysis—robustness check using control variables
Out of the control variables, income is highly significant, just as it is emphasized by the cited literature related to emerging countries. This result cannot be generalized for developed countries, where the impact of income upon buying a life insurance contract remains debatable.
In emerging countries like Romania, discrepancies between the urban and rural areas are high. This explains the significance and the sign of the coefficient of the urbanization rate.
The share of doctors in the population is positively influencing the life insurance market. This can be explained by the fact that such insurance products also have additional health insurance components. The existence of a well-developed medical network is an incentive for subscribing life insurance policies.
The criminality rate, the unemployment rate and the cultural vitality (SPECTATORS) are not significant. However, they are used only as control variables, the study does not have as a goal to explain the influence of these variables upon the development of the life insurance market at the county level.
There is a positive correlation between LHDI and INCOME (Pearson’s coef. = 0.45), as the latter provides the basis for human development. However, INCOME is a necessary, but not sufficient condition for human development. Some counties were more performant in using this basis than others. When the two variables are visualized together in a spatially smoothed rate map, a clusterization process is put into light, confirming, once again, the contagion and diffusion processes. In Figure , the ratio LHDI to INCOME shows high spatial rates in Western Romania, region which is closer to Central Europe from the point of view of the economic behaviour.
5. Conclusions
We examined an important and widely studied topic in the literature, the determinants of the demand for life insurance, from a different perspective which was not used until now in this research field, using the spatial econometric analysis. We choose this approach because we presume that high internal migration and commuting fluxes should led to an increase in the exchange of information between the Romanian regions with different levels of development.
We updated the values of the Local Human Development Index for the year 2014, using a methodology constructed and applied for the World Bank. We find a positive and significant correlation between the size of the life insurance market and the levels of standard of living, longevity and educational stock at the county level. Furthermore, the life insurance market is more developed in counties with a higher urbanization rate and a higher proportion of specialized medical staff in the county’s population.
Taking into consideration the levels of the indicators life insurance density and Local Human Development Index, the Centre counties and also the capital Bucharest-Ilfov county are the most developed ones.
Our article provides policymakers and practitioners with new evidence linking the local level of human development to the financial behaviour of the population.
We have shown that the relationship between human development and population incomes can be heterogeneous in territorial profile. Moreover, for the development of the insurance market, human development is a more important factor than income. This result could also be valid for other components of the financial sector. As a result, central and regional government institutions need to focus their policies on the channels of income transmission toward the different components of human development, because human development is no longer just a goal, by the welfare brought to the population, but also an economic development factor.
Insurance regulatory institutions can consider the causal relationships identified in our study, in order to develop coherent fiscal and administrative policies, consistent with the market potential. Insurance companies can find precise benchmarks regarding the level of undervaluation or overvaluation of regional markets, developing targeted strategies for product promotion or human resources strategies.
The study has several limitations, imposed in particular by the lack of data for some variables. For example, there are no county-level data on the quality of institutions, a factor that has been highlighted in empirical research at cross-national level. Also, due to the inconsistency of the data, we have not been able to evaluate the time effects, on panel data, which could show whether the diffusion effects are consistent for a longer period, or are temporary. However, these limitations open the way for future research directions. Further studies could also identify more precisely the channels through which space diffusion is happening.
Considering the fact that Romania is an ex-communist country which has socio-cultural similarities with other Central and Eastern European countries, our results could be generalized for these countries, at least in part, especially if no research is made in this field.
Acknowledgements
We would like to express our gratitude to the Media XPRIMM group representatives, and especially to Mrs. Daniela Ghețu, Editorial Director at the Media XPRIMM, who provided us a part of the preliminary database. The results and conclusions of the paper are entirely our responsibility and the Media XPRIMM group cannot be held liable for any of the opinions stated in our research paper. We wish to thank Professor Dumitru Sandu, from the Bucharest University, Faculty of Sociology and Social Work, for providing us the methodology necessary for the update of the Local Human Development Index, his guidance throughout the process and the feedback.
Additional information
Funding
Notes on contributors
Codruța Mare
Codruta Mare is an Associate Professor at the Babeș-Bolyai University, Cluj-Napoca, Romania. She gained her Ph.D. in Economics from University of Trieste, Italy. She has published scientific papers which include applications of panel econometrics and spatial econometrics in the areas of macroeconomics, business, finance and administrative sciences. She is the Scientific Director of the Interdisciplinary Centre for Data Science, in the same university.
Simona Laura Dragoș
Simona Laura Dragos is an Associate Professor at the Babes-Bolyai University, Cluj-Napoca, Romania. She holds a PhD in Finance from Babes-Bolyai University and Orleans University, France. Her research interests include: insurance, risk management, pensions, especially estimations for life and non-life products.
Ingrid-Mihaela Dragotă
Ingrid Mihaela Dragotă is a Professor, PhD at the Bucharest University of Economic Studies, Bucharest, Romania. Her research areas include: corporate finance, investments, insurance, and social protection. She is a member of the Euro Working Group on Financial Modelling.
They published articles in journals like Geneva Papers on Risk and Insurance – Issues and Practices, Economic Research, Social Indicators Research, etc.
Notes
1. Amount of Euros spent annually on life insurance per capita.
2. Fortune (Citation1973), Beenstock, Dickinson, and Khajuria (Citation1986), Lewis (Citation1989), Truett and Truett (Citation1990), Browne and Kim (Citation1993), Outreville (Citation1996), Ward and Zurbruegg (Citation2002), Beck and Webb (Citation2003), Li et al. (Citation2007), and Dragos, Mare, Dragota, Dragos, and Muresan (Citation2017).
3. Beck and Webb (Citation2003).
4. Lenten and Rulli (Citation2006).
5. Beenstock et al. (Citation1986) and Outreville (Citation1990, Citation1996).
6. Dragos (Citation2014), Hwang and Gao (Citation2003).
7. Beck and Webb (Citation2003), Nesterova (Citation2008).
8. Educational stock.
9. Life expectancy at birth.
10. Medium age of adult population.
11. The living floor area by house, the private cars to 1000 inhabitants and the distribution of gas for household consumption by locality inhabitant.
12. For this study, we updated the Local Human Development Indicator at the county level for 2014 and used it for the first time as a factor in research studies.
References
- Alexa, D., Cismas, L. M., Rus, A. V., & Pop Silaghi, M. I. (2019). Economic growth, competitiveness and convergence in the European regions. A spatial model estimation. Economic Computation & Economic Cybernetics Studies & Research, 53(1), 107–15. doi:10.24818/18423264/53.1.19.07
- Anderson, D. R., & Nevin, J. R. (1975). Determinants of young marrieds’ life insurance purchasing behavior: An empirical investigation. The Journal of Risk and Insurance, 42, 375–387. doi:10.2307/251694
- Asongu, S. A., & Odhiambo, N. M. (2019). Insurance policy thresholds for economic growth in Africa. The European Journal of Development Research, 1–18. doi:10.1057/s41287-019-00234-2
- Auerbach, A. T., & Kotlikoff, L. J. (1989). How rational is the purchase of life insurance? Working paper presented at the National Bureau of Economic Research, no 3063.
- Beck, T., & Webb, I. (2003). Economic, demographic and institutional determinants of life insurance consumption. The World Bank Economic Review, 17(1), 51–88. doi:10.1093/wber/lhg011
- Beenstock, M., Dickinson, G., & Khajuria, S. (1986). The determination of life premiums: An international cross-section analysis, 1970–1981. Insurance: Mathematics and Economics, 5, 261–270.
- Browne, M. J., & Kim, K. (1993). An international analysis of life insurance demand. The Journal of Risk and Insurance, 60, 617–634. doi:10.2307/253382
- Burduja, S. I., Gaman, F., Giosan, V., Glenday, G., Huddlestan, E. N., Ionescu-Heroiu, M., … Ha Ti Tu Vu. (2014). Identification of project selection models for the regional operational program 2014–2020. Washington, DC: World Bank.
- Busega, I. (2016). The impact of economic crisis on regional disparities in Romania. Testing Williamson’s hypothesis in the context of economic and financial turmoil. Romanian Review of Regional Studies, 12(1), 13–24.
- Chang, C. H., & Lee, C. C. (2012). Non-linearity between life insurance and economic development: A revisited approach. The Geneva Risk and Insurance Review, 37, 223–257. doi:10.1057/grir.2011.10
- Ciccarelli, C., & Elhorst, J. P. (2018). A dynamic spatial econometric diffusion model with common factors: The rise and spread of cigarette consumption in Italy. Regional Science and Urban Economics, 72, 131–142. doi:10.1016/j.regsciurbeco.2017.07.003
- Cracolici, M. F., Cuffaro, M., & Nijkamp, P. (2007). Geographical distribution of unemployment: An analysis of provincial differences in Italy. Growth and Change, 38(4), 642–665. doi:10.1111/j.1468-2257.2007.00391.x
- Cristea, M., Mare, C., Moldovan, C., China, A. M., Farole, T., Vintan, A., … Ionescu-Heroiu, M. (2017). Magnet cities: Migration and commuting in Romania. Washington, DC: World Bank.
- Dachin, A., & Popa, R. (2011). Regional disparities in employment structures and productivity in Romania. Romanian Journal of Regional Science, 5(2), 65–75.
- Dragos, S. L. (2014). Life and non-life insurance demand: The different effects of influence factors in emerging countries from Europe and Asia. Economic Research-Ekonomska Istraživanja, 27(1), 169–180. doi:10.1080/1331677X.2014.952112
- Dragos, S. L., Mare, C., Dragota, I. M., Dragos, C. M., & Muresan, G. M. (2017). The nexus between the demand for life insurance and institutional factors in Europe: New evidence from a panel data approach. Economic Research-Ekonomska Istraživanja, 30(1), 1477–1496. doi:10.1080/1331677X.2017.1325764
- Emamgholipour, S., Arab, M., & Mohajerzadeh, Z. (2017). Life insurance demand: Middle East and North Africa. International Journal of Social Economics, 44(4), 521–529. doi:10.1108/IJSE-04-2015-0106
- Fortune, P. (1973). A theory of optimal life insurance: Development and test. Journal of Finance, 27, 587–600.
- Goschin, Z., Constantin, D. L., Roman, M., & Ileanu, B. (2007) Disparităţile regionale din România în contextul politicii de coeziune a Uniunii Europene [Regional Disparities in Romania in the Context of EU’s Cohesion Policy]. Theoretical and Applied Economics, Supplement “Romania in the European Union. Quality of Integration. Regional Development”. Bucharest. pp. 19–26. doi: 10.1016/j.jinorgbio.2006.07.015
- Guiso, L., & Jappelli, T. (1998). Background uncertainty and the demand for insurance against insurable risks. Geneva Papers of Risk and Insurance Theory, 23(1), 7–27. doi:10.1023/A:1008621712979
- Hammond, J. D., Houston, D. B., & Melander, E. R. (1967). Determinants of household life insurance premium expenditures: An empirical investigation. The Journal of Risk and Insurance, 34(3), 397. doi:10.2307/250854
- Hwang, T, & Gao, S. (2003). The determinants of the demand for life insurance in an emerging economy–the case of china. Managerial Finance, 29(5/6), 82–96.
- Insurance Europe. (2015). European insurance in figures – 2015 data. https://www.insuranceeurope.eu/
- Lee, H. S., Chong, S. C., & Sia, B. K. (2018). Influence of secondary and tertiary literacy on life insurance consumption: Case of selected ASEAN countries. The Geneva Papers on Risk and Insurance-Issues and Practice, 43(1), 1–15. doi:10.1057/s41288-017-0050-7
- Lenten, L. J., & Rulli, D. N. (2006). A time-series analysis of the demand for life insurance companies in Australia: Unobserved components approach. Australian Journal of Management, 31(1), 41–66. doi:10.1177/031289620603100104
- Lewis, F. D. (1989). Dependents and the demand for life insurance. American Economic Review, 79, 452–467.
- Li, D., Moshirian, F., Nguyen, P., & Wee, T. (2007). The demand for life insurance in OECD countries. The Journal of Risk and Insurance, 74(3), 637–652. doi:10.1111/jori.2007.74.issue-3
- López-Bazo, E., Del Barrio, T., & Artis, M. (2002). The regional distribution of Spanish unemployment: A spatial analysis. Papers in Regional Science, 81(3), 365–389. doi:10.1007/s101100200128
- López-Bazo, E., Del Barrio, T., & Artis, M. (2005). Geographical distribution of unemployment in Spain. Regional Studies, 39(3), 305–318. doi:10.1080/00343400500087034
- Maguain, D., & Fréret, S. (2013). The determinants of welfare spending in France: A spatial panel econometric approach. Annals of Economics and Statistics, 109/110, 93–129. doi:10.2307/23646428
- Mare, C., Dragos, S. L., Dragota, I. M., Muresan, G. M., & Urean, C. A. (2016). Spatial convergence processes on the European Union’s life insurance market. Economic Computation and Economic Cybernetics Studies and Research, 50(4), 93–107.
- Millo, G., & Carmeci, G. (2011). Non-life insurance consumption in Italy: A sub-regional panel data analysis. Journal of Geographical Systems, 13(3), 273–298. doi:10.1007/s10109-010-0125-5
- Millo, G., & Carmeci, G. (2015). A subregional panel data analysis of life insurance consumption in Italy. Journal of Risk and Insurance, 82(2), 317–340. doi:10.1111/jori.v82.2
- Nesterova, D. (2008). Determinants of the demand for life insurance: Evidence from selected CIS and CEE countries. National University “Kyiv-Mohyla Academy, 1-49.
- Outreville, J. F. (1990). The economic significance of insurance markets in developing countries. The Journal of Risk and Insurance, 18(3), 487–498. doi:10.2307/252844
- Outreville, J. F. (1996). Life insurance markets in developing countries. The Journal of Risk and Insurance, 63(2), 263–278. doi:10.2307/253745
- Overman, H. G., & Puga, D. (2002). Unemployment cluster across Europe’s regions and countries. Economic Policy, 34, 116–147.
- PRIMM Insurance & Pensions Review. (2015). Regional figures: Cluj and timiș dominate the regional insurance market. no 4 (142),year XVI:12–23. Bucharest: Media XPRIMM Romania.
- Romanian National Institute of Statistics. (2015) Retrieved from http://statistici.insse.ro:8077/tempo-online/#/pages/tables/insse-table
- Truett, D. B., & Truett, L. J. (1990). The demand for life insurance in Mexico and the United States: A comparative study. The Journal of Risk and Insurance, 57, 321–328. doi:10.2307/253306
- Ward, D., & Zurbruegg, R. (2002). Law, politics and life insurance in Asia. The Geneva Papers on Risk and Insurance, 27(3), 395–412. doi:10.1111/1468-0440.00181
- Zangger, C. (2019). Making a place for space: Using spatial econometrics to model neighborhood effects. Journal of Urban Affairs, 41(8), 1055–1080. doi:10.1080/07352166.2019.1584530.