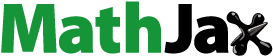
Abstract
This paper explores the impact of corruption on both the banking sector and economic growth; we determine the impact using aggregate data from the World Bank covering 120 countries over the period 2004–2017. The results of 3SLS regressions show that the relationship between corruption and the ratio of nonperforming loans was positive, thus deteriorating the soundness of the banking system. In this study, we also find evidence that banking system is a channel that transfers the impact of corruption on economic growth: Corruption leads to higher levels of nonperforming loans in the banking sector; as a result, it decreases economic growth. The findings survive a battery of robustness tests, including alternative corruption measurement and System Generalized Method of Moments regression for dynamic models with an interaction term.
PUBLIC INTEREST STATEMENT
Corruption can be both beneficial and detrimental to the development of an economy. Numerous studies have delved on the impacts of corruption on economic growth via its influence on investment, human capital, etc., but little has been done to investigate the direct and indirect effects of corruption on economic growth through the channel of banking sector. Filling this gap, the present study employs a panel of 120 countries spanning a period from 2004 to 2007 and several econometric methods including 3SLS and System Generalized Methods of Moment to ascertain the findings. We find that corruption increases bad loans, or undermines bank’s asset quality. Bad loans, in turn, weaken the economic growth. Therefore, we conclude that banking sector is a channel where corruption exerts its negative impact on economic growth. Based on the research findings, we propose several implications for policymakers as well as academics with regard to their future research directions.
1. Introduction
The banking system plays a vital role in a country’s economy, as it channels funds from lenders to borrowers. However, the banking system does not always work well, and the problems of banking system can be complex, one of which is corruption. After the financial crisis, lending by banks suffered a severe fall (Ivanshina, Citation2009), and corruption may have an impact on the lending behavior of banks. Corruption is one of the major causes of nonperforming loans in many countries (see Bougatef, Citation2016; Goel & Hasan, Citation2011; Park, Citation2012). The effects of corruption on the economy have been a topical issue that has attracted many academics as well as policymakers (Lambsdorff, Citation2005; Mauro, Citation1995). Some studies show that corruption indirectly affects economic growth via channels such as private investment, FDI, public expenditure, human capital, etc. (Cieslik & Goczek, Citation2018; Grundler & Potrafke, Citation2019; Hodge, Shankar, Rao, & Duhs, Citation2011; Mauro, Citation1995; Mo, Citation2001; Murphy, Shleifer, & Vishny, Citation1993; Nguyen, Nguyen, & Tran, Citation2016; Wei, Citation2000).
Prior studies have shown two possible effects of corruption on economic growth. On the other hand, efficient projects will be facilitated further by bribing politicians and credit loan officers to obtain credit approvals (see Mauro (Citation1995) for a similar “speed money” argument). Consistent with the “grease the wheels” hypothesis, Chen, Liu, and Su (Citation2013) find that bribery enables more productive firms to receive larger loans from banks. However, Khwaja and Mian (Citation2005) argue that companies that are in contact with politicians are granted access to credit fast, but they also have a higher default rate. Charumilind, Kali, and Wiwattanakantang (Citation2006) show that firms with a close connection with politicians are required less collaterals when accessing long-term bank credit, leaving too much risk for banks.
The “sand the wheels” hypothesis suggests that corruption can adversely affect financial sector activity (see Cooray & Schneider, Citation2018), and greater corruption may result in the financial crisis (e.g. Park, Citation2012). Corruption may lead to resource misallocation that is damaging to banking sector development, leading to increased amounts of nonperforming loans (Bougatef, Citation2016; Goel & Hasan, Citation2011). In turn, decreasing banks’ asset quality may undermine the productivity of private investment, generating a dragging effect on economic growth (Park, Citation2012).
The goal of this study examines the financial impacts of corruption on the soundness of banking system, particularly through its impact on the quality of banks’ assets. In addition to examining the direct impact of corruption, the present study investigates whether corruption may affect economic growth indirectly by means of its impact on banks’ nonperforming loans. Ahlin and Pang (Citation2008) and Blackburn and Forgues-Puccio (Citation2010) opine that the interaction between financial development and corruption affects economic growth and development.
First, our paper contributes to the growing empirical literature on corruption-finance nexus. Our findings point to the necessity of more research on the nonperforming loans (Manz, Citation2019, p. 34). We offer a possible explanation of why crises have taken place more often in countries with high levels of corruption. In our study, corruption is found to have a negative association with bank asset quality, i.e. higher levels of nonperforming loans, which may ultimately lead to crises. Second, we provide evidence that corruption is likely to weaken economic growth via the channel of banking sector. We reach this conclusion using 3SLS regression for a system of two equations and a battery of robustness tests. The current study is closely related to Park (Citation2012), which shows that corruption is positively related to the level of nonperforming loans. This paper helps extend Park (Citation2012) in that the latter study only examines a sample of 70 economies in a mere three-year period (2002–2004). We contribute to the current literature by updating the data to the most updated period available with a longer time span (2004–2017) for a sample comprising 120 economies to examine whether the relationship also exists. Finally, we provide robustness tests to ascertain the findings in the current study.
2. Literature review and hypothesis development
The literature on the nexus between corruption and growth is voluminous, and the mainstream findings tend to suggest that corruption negatively affects economic growth. Mauro (Citation1995) indicates that corruption degrades the quality of private investment and lowers economic growth. Corruption has a significant negative effect on investment, the efficiency of public expenditure, the accumulation of human capital, the inflow of foreign direct investment, the effectiveness of international aid, etc., thus exerting detrimental impact on economic growth (Cieslik & Goczek, Citation2018; Grundler & Potrafke, Citation2019; Mo, Citation2001; Murphy et al., Citation1993; Shleifer & Vishny, Citation1993; Wei, Citation2000). On the other hand, several researchers find that corruption might be desirable, suggesting that corruption helps firms to circumvent bad policies, thereby stimulating economic growth in countries with weak institutions (Dreher & Gassenbner, Citation2013; Johnson, Citation1975; Lef, Citation1964; Leys, Citation1965; Nye, Citation1967; Wedeman, Citation1997).
Despite numerous empirical works on the corruption-development nexus, the literature dissecting the relationship between corruption and financial sector (including banking system) is scant (Chen, Jeon, Wang, & Wu, Citation2015). In addition, the literature on the relationship between corruption and bank activity offers mixed findings. The “sand the wheels” hypothesis suggests that corruption can negatively affect bank activity: Khwaja and Mian (Citation2005) and Charumilind et al. (Citation2006) show that firms that are linked with politicians are able to be given credit lines, but these firms have higher default rates ex-post, causing high levels of nonperforming loans for the banks. Furthermore, the more corrupt a country is, the more risk a banking system is prone to expose to. Chen et al. (Citation2015) find that corruption is likely to nurture banks’ risk-taking behavior. Consistently, Toader, Onofrei, Popescu, and Andrieș (Citation2017) show that countries with lower levels of corruption bank stability are more secured. The alternative view is that corruption “greases the wheels” with regard to bank activities. For example, “speed money” would facilitate individuals to avoid administrative delay (Mauro, Citation1995). Chen et al. (Citation2013) find that bribery is an enabling factor for the access of private firms to larger bank loans.
Recent empirical studies lean toward a common finding suggesting that corruption leads to an increase in nonperforming loans and a decrease in the soundness of the banking sector (Barth, Caprio, & Levine, Citation2004; Bougatef, Citation2015, Citation2016; Goel & Hasan, Citation2011; Park, Citation2012). Using the sample of 107 countries, Barth et al. (Citation2004) find a significantly positive correlation between corruption and nonperforming loans, and the relationship conditions on the degree of development of governance and supervisory mechanisms. Using a sample of 76 countries over the period 2002–2004, Park (Citation2012) investigates the impact of corruption on the banking sector and his results suggest that corruption increases bank nonperforming loans. Bougatef (Citation2015) estimates the impact of corruption on the soundness of a system of 69 Islamic banks for the period 2008–2010 and notes that corruption increases bank nonperforming loans and deteriorates bank financial soundness. Bougatef (Citation2016) analyzes the impact of corruption on loan portfolios of 22 emerging economies for the period 2008–2012 and his results show that corruption deteriorates the banks’ assets quality, that is, increases the level of nonperforming loans. Using a large sample of countries, Goel and Hasan (Citation2011) show that countries with higher corruption are more likely to be plagued with a higher level of bank nonperforming loans.
Other studies have examined the interaction between corruption and financial development on economic development. For instance, Ahlin and Pang (Citation2008) find that financial development and corruption to be substitutes for their impacts on growth. However, they do not consider the case in which corruption indirectly impacts economic growth through a financial sector channel. In another paper, Blackburn and Forgues-Puccio (Citation2010) study the effect of international financial integration on economic development when the quality of governance may be compromised by corruption. In the presence of weak institutions, financial liberalization will result in higher corruption and may discourage growth. Although these studies reveal some empirical evidence demonstrating the impact of corruption on economic growth and development through the interaction between financial development and corruption, they do not explore the effects of both banking sector and corruption on economic growth. Very few exceptions (see, for example, Park, Citation2012) provide evidence on the role of banking system as a channel that transfers the impact of corruption on economic growth.
In summary, in addition to examining the direct impact of corruption on nonperforming loans, we argue that corruption may affect a country’s economic growth indirectly, particularly via its impact on banks’ nonperforming loans. This leads to our following testable hypotheses:
Hypothesis 1: corruption is positively related to nonperforming loans.
Hypothesis 2: banking system is a channel that transfers the impact of corruption on economic growth.
3. Methodology
3.1. Data
The current study utilizes a dataset covering 120 countries for the period from 2004 to 2017 from World Development Indicators (WDI) of the World Bank. The main independent variable of interest is corruption. Given the uncertainty and likely errors in measuring corruption, the robustness of the results is ascertained by resorting to two different corruption proxies. Our main measure of cross-country corruption is Control of Corruption index (CCI) collected from the World Bank, ranging from −2.5 to 2.5. Control of Corruption captures perceptions of the extent to which public power is exercised for private gain, including both petty and grand forms of corruption, as well as “capture” of the state by elites and private interests (Kaufmann, Kraay, & Mastruzzi, Citation2009).
We use the International Country Risk Guide (ICRG) corruption index as an alternative measurement of corruption. The ICRG index ranges from 0 (totally corrupt) to 6 (not corrupt), or higher values of the index denote better control of corruption. The ICRG index provides annual ratings of corruption for countries from surveys with country experts. It is a measure of corruption within the political system that threatens foreign investment by distorting the economic and financial environment, reducing the efficiency of government and business by enabling people to assume positions of power through patronage rather than performance, thereby leading to political instability (Political Risk Services Group, Citation2020).
3.2. Research models
To verify whether banking system is a channel that transfers the impact of corruption on economic growth is similar to the examination of whether the boost in nonperforming loan in the banking sector may ultimately contribute to the decrease of economic growth. Therefore, we seek to quantify the effect of corruption on nonperforming loans and nonperforming loans on economic growth. This, however, creates a problem because nonperforming loans are a function of economic growth, which is one of the independent variables in the nonperforming loans equation. To tackle the simultaneity (a cause of endogeneity problem), we employ a simultaneous equations model composed of NPLR and RGDP equations. Belsley (Citation1988) suggests that 3SLS is more efficient than 2SLS, a relative advantage that increases with the strength of the interrelations among the error terms. Nosier and El-Karamani (Citation2018) suggest that 3SLS is efficient in verifying the indirect effect of a factor on economic growth. Therefore, the 3SLS estimator which combines 2SLS, which uses instrumental variable estimation, and SUR is used in our study to estimate the above-mentioned simultaneous equation.
The following simultaneous equations models are estimated by 3SLS:
Where: RGDPi,t and NPLRi,t represent the two endogenous variables in the above simultaneous equation system. In line with previous studies, e.g. Park (Citation2012), Bougatef (Citation2015), the ratio of nonperforming loans/total loans is used as a measurement of nonperforming loans.
3.2.1. Independent variables
CCI (Control of Corruption index) is the measure of the corruption at the national level, ranging from −2.5 to 2.5, with a higher value of CCI indicating lower corruption. This variable is a commonly adopted indicator of corruption (Chen et al., Citation2015).
3.2.2. Bank characteristics
Efficiency: The effect of efficiency on nonperforming loans is ambiguous. Under the “skimping hypothesis” of Berger and DeYoung (Citation1997), banks will have an increasing level of nonperforming loans if banks devote less resources to monitor loan. On the contrary, under the “bad management” hypothesis, higher cost inefficiency could increase nonperforming loans. Efficiency is measured by bank overhead costs divided by total assets.
Profitability is measured by return on assets (ROA), i.e. net income divided by total assets. Under the “bad management” hypothesis of Berger and DeYoung (Citation1997), highly profitable banks have fewer incentives to engage in high-risk activities, thereby reducing their nonperforming loans.
CAP is measured by the ratio of bank capital and reserves to total assets, similar to Park (Citation2012). The effect of bank capitalization on nonperforming loans is theoretically ambiguous. Under the “moral hazard” hypothesis of Keeton and Morris (Citation1987), the higher the ratio, the less likelihood of banks being involved in risky operations, so CAP is inversely related to nonperforming loan ratio. On the other hand, managers in banks that are highly capitalized may take excessive risks leading to rising nonperforming loans (Rajan, Citation1994).
LIQ is measured by the ratio of bank liquid reserves to bank assets. This indicator shows the ability to ensure the bank liquidity, thus the higher the ratio, the higher the level of bank soundness. By contrast, the higher level of liquidity holding by a bank may actually reflect the bank’s appetite for more risk (Chen et al., Citation2015).
3.2.3. Macroeconomic factors
In the estimation, we include three macroeconomic variables, namely, the real growth rate of GDP, household final consumption expenditures, and government expenditures. The source of these data is from the World Bank.
RGDP is the real GDP growth. It represents the overall economic environment. When the economy grows, the nonperforming loan ratio will decrease as the repayment capacity of individuals and businesses increases (Louzis, Vouldis, & Metaxas, Citation2012). By contrast, economic expansion may be associated with the higher nonperforming loan since credit may be extended to lower-quality debtors. So, the impact of the overall economic environment on the nonperforming loans is an empirical matter.
HHC is the growth rate of household final consumption expenditures. Household spending represents personal credit and is considered one of the factors that affect nonperforming loan (Park, Citation2012). We expect a positive correlation between household final consumption expenditures and nonperforming loan ratio.
GOV is the rate of government expenditures over GDP. It represents the impact of government factors on nonperforming loans. The effect of government expenditures is ambiguous. High public spending can put a constraint on the financial system of a country and increase nonperforming loans (Cooray, Citation2011; Cooray & Schneider, Citation2018). However, the government expenditures also represent the degree of government intervention in economic development, creating a strong correlation between the quality of bank assets (Chen, Feng, & Wang, Citation2018).
For the RGDP equation, we introduce a simple growth equation in which FIXED, POPG, GOV are used as the components of the usual production function (Levine & Renelt, Citation1992). FIXED is used to proxy for the total investment of a country, and the number is the gross fixed capital formation divided by the nominal GDP. POPG is included to proxy for the growth rate of the population. GOV: rate of government expenditures over GDP. Other variables are defined the same as in EquationEquation (1)(1)
(1) .
4. Empirical results
4.1. Descriptive statistics
Table briefly outlines the basic parameters of the research variables. The average corruption level (CCI) is 0.128, with the lowest being −1.72 and the highest at 2.47. For the dependent variable, the nonperforming loan ratio is 6.2% on average. GDP growth (RGDP) is approx. 4% per year on average for the whole sample.
Table 1. Descriptive statistics
Table provides a correlation matrix for the variables in the models. CCI is negatively related to NPLR, and NPLR is negatively related to RGDP (GDP growth). However, CCI is also negatively associated with RGDP. Also, the coefficients between pairs of variables are not so high, which suggests that multicollinearity should not be a concern in this study. Nevertheless, these coefficients do not constitute a valid base for interpreting the impact of CCI on RGDP and NPLR, so we continue to provide regression results to examine the hypotheses.
Table 2. Correlation matrix
4.2. Regression Results
4.2.1. 3SLS estimation results
4.2.1.1. Effects of corruption on nonperforming loans
From Table , the coefficient of control of corruption index (CCI) is −2.436 with significance at the 1% level, suggesting that corruption deteriorates the asset quality of the banking sector. As corruption in a country increases, banks’ risk tolerance increases, and bank financing is allocated to bad projects, reducing the probability of repaying loans on time and resulting in an increase in nonperforming loans, suppressing the soundness of the banking system. As a result, the banking system becomes vulnerable, which supports the “sand the wheels” hypothesis. Therefore, corruption can be one of the factors that precede a financial crisis. These findings are in line with the studies of Park (Citation2012) and Bougatef (Citation2015), which conclude that corruption significantly aggravates the bank asset quality.
Table 3. Corruption, nonperforming loans, and economic growth
RGDP, HHC, GOV, Efficiency, CAP, and Liquidity have insignificant impacts on nonperforming loans. The bank profitability as measured by the return on asset (ROA) is inversely related to nonperforming loans. This evidence supports the “bad management” hypothesis of Berger and DeYoung (Citation1997), i.e. highly profitable banks have fewer incentives to engage in high-risk activities, thereby reducing their nonperforming loans. This evidence is consistent with findings in Louzis et al. (Citation2012) and Bougatef (Citation2015).
4.2.1.2. Effects nonperforming loan and economic growth
In Table , NPLR has an expected negative coefficient with a 1% level of significance. Other variables such as FIXED, POPG, and GOV in the RGDP equation also have significant expected signs. Based on the above results, there is some evidence of the existence of a channel through which corruption undermines economic growth: corruption increases the nonperforming loans, which in turn decreases the growth rate of an economy. Our results are in line with Park (Citation2012).
4.2.2. System generalized method of moment estimation
Simultaneous equations modelling is asymptotically more efficient than the estimation based on a single equation, but the former approach requires thorough modelling as all the structural parameters will be biased even when only one of the equations is incorrectly specified (Arminen & Menegaki, Citation2019). To avoid this shortcoming, Blundell and Bond (Citation1998) and Arellano and Bover (Citation1995) suggest the use of the dynamic panel modelling, to be estimated by the Differenced and System GMM estimators. These estimators rely on internal instruments, which helpfully overcomes the challenge of acquiring instruments not included in the model.
To ascertain the findings, we consider the estimation technique of a dynamic two-step System generalized method of moment (System GMM). The System GMM approach uses lagged endogenous and predetermined variables as instruments for the endogenous and predetermined variables in first differences, and uses lagged differences of endogenous and predetermined variables as instruments for their respective variables in levels (Roodman, Citation2009). As mentioned in the previous section that GDP growth rates, nonperforming loans, and corruption can have a mutual relationship, we reckon them as endogenous variables. Furthermore, POPG (population growth) and GOV (governmental expenditures) can be affected by RGDP, thus also being endogenous. Therefore, we apply Roodman (Citation2009)’s treatment in finding instruments for the endogenous variables. By employing this method, we aim to address several issues such as endogeneity, heteroskedasticity, as well as the potential of the dynamic modelling of the dependent variable.
To quantify whether the banking system is a channel that transfers the impact of corruption on economic growth, we introduce an interaction term between corruption and nonperforming loans in the below dynamic model:
As can be seen from Table , consistent with the previous results obtained with the 3SLS regression, the interaction between corruption and nonperforming loans is negatively related to economic growth. If corruption control helps contain the proliferation of nonperforming loans and the latter exerts a negative impact on GDP growth, then the corruption control should alleviate or dampen the negative influence of corruption on economic growth. The signs of the individual factors (NPLR, CCI) and interaction between these two factors (CCI*NPLR) on GDP growth provide evidence in support of this argument.
Table 4. System generalized method of moment estimation
5. Robustness tests
To ascertain the robustness of the findings, we conduct the same analyses replacing CCI with the International Country Risk Guide (ICRG) corruption index. The ICRG index ranges from 0 (totally corrupt) to 6 (not corrupt), with higher values of the corruption index implying better control of corruption. In Table , ICRG has a significantly negative relationship with nonperforming loans. Using the alternative indicator of corruption has reinforced the argument that corruption reduces the soundness of the banking system, hence it decreases economic growth. As shown in Table , there is no significant change in the main results of the control variables.
Table 5. Robustness test: alternative corruption index
6. Conclusion
Using a sample of 120 countries over the period of 2004 to 2017, the current research seeks to examine whether corruption effects the nonperforming loans of banking system. We find some significant results through the empirical analyses. First, we find important evidence that there is a positive association between corruption and the ratio of nonperforming loans, suggesting that corruption deteriorates the soundness of the banking system. Second, we find some evidence that banking system is a channel through which corruption decreases economic growth: corruption leads to an increase in bad loans in the banking sector and bad loans in turn negatively affect the economic growth. This result is robust to an alternative econometric methodology (System GMM) and a second corruption indicator (ICRG).
Even though the literature on the corruption-economic growth nexus is voluminous, the empirical works on the financial sector’s channel where corruption exerts its impact on the economic development are scant. Our results are consistent with Park (Citation2012) which outlines the negative impact of corruption on banks’ asset quality. In other words, the current study and Park (Citation2012) suggest banking sector is a channel through which corruption undermines economic growth. Also, the evidence in our paper is consistent with the view of “sand the wheels” hypothesis in Cooray and Schneider (Citation2018), confirming the negative repercussions of corruption.
From the results of this study, the article offers some policy implications given the important role of the banking soundness in stimulating economic growth as follows: The significantly positive relationship between corruption and nonperforming loan ratio is a warning sign for policymakers. The detrimental impact of corruption on banking soundness justifies the exerting efforts to fight against corruption in order to continue the impetus for economic development. Along with the Fourth Industrial Revolution, one solution could be to increase the e-readiness to reduce corruption in the economy (Ntemi & Mbamba, Citation2016).
This study uses the two main country-level corruption indicators: Control of Corruption index (CCI) and International Country Risk Guide (ICRG) corruption indices. Therefore, future studies could focus on corruption in banking sector instead of public corruption to verify whether the findings in this study still hold.
Additional information
Funding
Notes on contributors
Tran Hung Son
Dr. Tran Hung Son is currently working for the University of Economics and Law, Vietnam National University, Hochiminh and Institute for Development and Research in Banking Technology. His research interests cover contemporary issues in banking and finance, financial crisis, financial development. Dr. Liem Nguyen is a Research Fellow at the Institute for Development and Research in Banking Technology. He mainly works in the field of corporate governance, innovation and bank stability and performance. Dr. Khuong Nguyen is a lecturer of Faculty of Auditing and Accounting, whose interests are in the fields of auditing and accounting, and corporate finance issues. The authors have published several papers on quality journals, such as Pacific Accounting Review, Sustainability, Post-Communist Economies, etc.
References
- Ahlin, C., & Pang, J. (2008). Are financial development and corruption control substitutes in promoting growth? Journal of Development Economics, 86, 414–12. doi:10.1016/j.jdeveco.2007.07.002
- Arellano, M., & Bover, O. (1995). Another look at the instrumental variable estimation of error‐components models. Journal of Econometrics, 68(1), 29–51. doi:10.1016/0304-4076(94)01642-D
- Arminen, H., & Menegaki, A. N. (2019). Corruption, climate and the energy-environment-growth nexus. Energy Economics, 80, 621–634. doi:10.1016/j.eneco.2019.02.009
- Barth, J., Caprio, G., Jr., & Levine, R. (2004). Bank regulation and supervision: What works best? Journal of Financial Intermediation, 13, 205–248. doi:10.1016/j.jfi.2003.06.002
- Belsley, D. A. (1988). Two-or three-stage least squares? Computer Science in Economics and Management, 1(1), 21–30. doi:10.1007/BF00435200
- Berger, A. N., & DeYoung, R. (1997). Problem loans and cost efficiency in commercial banks. Journal of Banking & Finance, 21(6), 849–870. doi:10.1016/S0378-4266(97)00003-4
- Blackburn, K., & Forgues-Puccio, G. F. (2010). Financial liberalization, bureaucratic corruption and economic development. Journal of International Money and Finance, 29, 1321–1339. doi:10.1016/j.jimonfin.2010.05.002
- Blundell, R., & Bond, S. (1998). Initial conditions and moment restrictions in dynamic panel data models. Journal of Econometrics, 87(1), 115–143. doi:10.1016/S0304-4076(98)00009-8
- Bougatef, K. (2015). The impact of corruption on the soundness of Islamic banks. Borsa Istanbul Review, 15(4), 283–295. doi:10.1016/j.bir.2015.08.001
- Bougatef, K. (2016). How corruption affects loan portfolio quality in emerging markets? Journal of Financial Crime, 23(4), 769–785.
- Charumilind, C., Kali, R., & Wiwattanakantang, Y. (2006). Connected lending: Thailand before the financial crisis. The Journal of Business, 79, 181–218.
- Chen, F.-W., Feng, Y., & Wang, W. (2018). Impacts of financial inclusion on non-performing loans of commercial banks: Evidence from China. Sustainability, 10, 3084.
- Chen, M., Jeon, B. N., Wang, R., & Wu, J. (2015). Corruption and bank risk-taking: Evidence from emerging economies. Emerging Markets Review, 24, 122–148.
- Chen, Y., Liu, M., & Su, J. (2013). Greasing the wheels of bank lending: Evidence from private firms in China. Journal of Banking and Finance, 37(7), 2533–2545.
- Cieslik, A., & Goczek, L. (2018). Control of corruption, international investment, and economic growth – Evidence from panel data. World Development, 103, 323–335.
- Cooray, A. (2011). The role of the government in financial sector development. Economic Modelling, 28(3), 928–938.
- Cooray, A., & Schneider, F. (2018). Does corruption throw sand into or grease the wheels of financial sector development? Public Choice, 177, 111–133.
- Dreher, A., & Gassenbner, M. (2013). Greasing the wheels? The impact of regulation and corruption on firm entry. Public Choice, 155, 413–432.
- Goel, R. K., & Hasan, I. (2011). Economy-wide corruption and bad loans in banking: International evidence. Applied Financial Economics, 21(7), 455–461.
- Grundler, K., & Potrafke, N. (2019). Corruption and economic growth: New empirical evidence. European Journal of Political Economy, 60, 101810.
- Hodge, A., Shankar, S., Rao, D. S., & Duhs, A. (2011). Exploring the links between corruption and growth. Review of Development Economics, 15(3), 474–490.
- Ivashina, V. (2009). Asymmetric information effects on loan spreads. Journal of Financial Economics, 92(2), 300–319.
- Johnson, E. (1975). An economic analysis of corrupt government, with special application for less developed countries. Kyklos, 28, 47–61.
- Kaufmann, D., Kraay, A., & Mastruzzi, M. (2009) Governance matters VIII: Aggregate and individual governance indicators, 1996-2008 (World Bank Policy Research Working Paper No. 4978).
- Keeton, W., & Morris, C. (1987). Why do banks’ loan losses differ?. Federal reserve bank of Kansas city Economic review, 72, 3–21.
- Khwaja, A. I., & Mian, A. (2005). Do lenders favor politically connected firms: Rent provision in an emerging financial market. The Quarterly Journal of Economics, 120(4), 1371–1411.
- Lambsdorff, J. G. (2005). Consequences and causes of corruption—what do we know from a cross-section of countries? (Discussion Paper V-34-05), University of Passau.
- Lef, N. (1964). Economic development through bureaucratic corruption. American Behavioral Scientist, 82, 337–341.
- Levine, R., & Renelt, D. (1992). A sensitivity analysis of cross-country growth regressions. American Economic Review, 82(4), 942–963.
- Leys, C. (1965). What is the problem about corruption? Journal of Modern African Studies, 3, 215–230. (Reprinted in Political corruption: A handbook, A. J. Heidenheimer, M. Johnston, & V. T. LeVine (Eds.), Oxford: Transaction Books, 51–66, 1989).
- Louzis, D., Vouldis, A. T., & Metaxas, V. L. (2012). Macroeconomic and bank-specific determinants of non-performing loans in Greece: A comparative study of mortgage, business and consumer loan portfolios. Journal of Banking & Finance, 36(4), 1012–1027.
- Manz, F. (2019). Determinants of non-performing loans: What do we know? A systematic review and avenues for future research. Management Review Quarterly, 69(4), 351–389.
- Mauro, P. (1995). Corruption and Growth. The Quarterly Journal of Economics, 110(3), 681–712.
- Mo, P. H. (2001). Corruption and economic growth. Journal of Comparative Economics, 29(1), 66–79.
- Murphy, K. M., Shleifer, A., & Vishny, R. W. (1993). Why is rent-seeking so costly to growth? American Economic Review, 83(2), 409–414.
- Nguyen, N. A., Nguyen, N. M., & Tran, B. (2016). Corruption and economic growth, with a focus on Vietnam. Crime Law Soc Change, 65(4–5), 1–18.
- Nosier, S., & El-Karamani, A. (2018). The indirect effect of democracy on economic growth in the MENA region (1990-2015). Economies, 6(61), 1–24.
- Ntemi, W. D., & Mbamba, U. O. (2016). The relationship between electronic readiness and corruption reduction: Countrywide data analysis. Cogent Business & Management, 3(1), 1–14.
- Nye, J. (1967). Corruption and political development: A cost-benefit analysis. American Political Science Review, 61, 417–427.
- Park, J. (2012). Corruption, soundness of the banking sector, and economic growth: A cross-country study. Journal of International Money and Finance, 31(5), 907–929.
- Political Risk Services Group. (2020, January 24). Guide to data variables. Retrieved from https://epub.prsgroup.com/list-of-all-variable-definitions
- Rajan, R. (1994). Why bank policies fluctuate: A theory and some evidence. Quarterly Journal of Economics, 109, 399–441.
- Roodman, D. (2009). How to do xtabond2: An introduction to difference and system GMM in stata. The Stata Journal, 9(1), 86–136.
- Shleifer, A., & Vishny, R. W. (1993). Corruption. The Quarterly Journal of Economics, 108(3), 599–617.
- Toader, T., Onofrei, M., Popescu, A., & Andrieș, A. M. (2017). Corruption and banking stability: Evidence from emerging economies. Emerging Markets Finance and Trade, 54(4), 591–617.
- Wedeman, A. (1997). Looters, rent-scrapers, and dividend-collectors: Corruption and South Korea, and the Philippines. Journal of Developing Areas, 31, 457–478.
- Wei, S. (2000). How taxing is corruption on international investors? Review of Economics and Statistics, 82, 1–11.