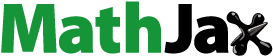
Abstract
Systemic risk is of concern for economic welfare as systemic financial crisis has the potential to inefficiently lower the supply of credit to the nonfinancial sector. Conventional systemic risk measures are parsimonious in nature and are used to assess the current systemic risk contributions of financial institutions and does not highlight future systemic risk. In order to overcome the limitations of conventional systemic risk, is used predict future systemic risk and the literature outlines the use of firm and state level variables in the computation. This study contributes to the existing body of knowledge by providing empirical evidence on improved computation of forward systemic risk by corroborating the distinctive impact of sector’s nature: munificence, dynamism and level of concentration. The comparison of conventional and proposed forward CoVaR provides interesting insights into the role of sector level variables in the buildup of systemic risk. The results highlight that the forecasting ability of forward ∆CoVaR predicted with sector level variables is reasonably higher than that of predicted without sector level variables. The study provides guidelines to the policy makers by providing an improved measure of future systemic risk that can that can help to avoid procycality pitfall by introducing countercyclical policies before the happening of systemic event. The results present new insights on the drivers of financial instability and provide implications for the micro- and macroprudential regulations of the banks.
PUBLIC INTEREST STATEMENT
Economic growth plays a pivotal role in the development of the economy. Economic growth depends on the scale of investment that eventually transforms in to increased gross domestic product. Financial institutions are an important source of providing impetus to investment by mobilizing savings and channelizing them to meet much needed capital requirements of other sectors. Financial institutions also accept deposits from general public and systemic financial crisis can potentially deprive the depositors from their hard-earned money. Moreover, investors of nonfinancial sector, employees and customers can also get useful information about the contagious events that can put the whole system in the crisis. This study can enlighten the general public about the risk that can potentially jeopardize the whole economy and how it can be curbed. Moreover, analyzing forward systemic risk of financial institutions risk is very important as introducing policies based on contemporaneous systemic risk can further aggravate the repercussions of systemic event.
1. Introduction
The global financial crisis unveiled that inadequate analysis of the risk can annihilate the financial system and repercussions can encompass the whole economy. The managers of the financial institutions were not able to measure and predict the risk exposure of the financial institutions and the cascade destroyed the whole system. Consistent with this purview, Lee et al. (Citation2019) postulate that overconfidence of chief executive officers contributed significantly to the 2008–2009 financial crisis. After the global financial crisis, risk assessment in the financial sector has become a prominent topic in the banking literature. Consistent with these arguments, Avramidis and Pasiouras (Citation2015) emphasize that in the aftermath of global financial crisis, there is a dire need of efficient measurement and prediction of risk.
The fragility of financial system can be avoided by adequately analyzing the risk exposure of the financial institutions (Andrieş et al., Citation2018). The risks faced by the organizations are broadly categorized as systematic and idiosyncratic risk along with the comparatively novel manifestation of systemic risk. Systematic risk is embedded in the system whereas idiosyncratic risk emanates from bank specific activities. Systemic risk refers to the spillover effects that result from the fall of one financial institution or a bank in supply chain and the cascade encompasses the whole financial system. Systemic risk has three pivotal characteristics namely contagion, externality and universality. Contagion effect rises due to interlinkages between financial institutions. The loss of large segment of the market is categorized as universality whereas negative externality refers to the spillover effect from one financial institution to others.
Despite a large number of published studies, there is no single consensus definition of systemic risk. For instance, Huang et al. (Citation2009) shed light on systemic risk as a phenomenon in which large financial institutions default simultaneously. Bartram et al. (Citation2007) define systemic risk as collapse of sizeable financial institutions with large social and economic costs. Acharya (Citation2009) defines systemic risk in the context of the correlation of returns on the asset side of bank balance sheets resulting in joint failure risk. In addition to that, Billio et al. (Citation2012) also explicated that systemic risk jeopardizes the stability or public confidence in the financial system.
In a recent study, Adrian and Brunnermeier (Citation2016) argue that conventional systemic risk measures are parsimonious in nature and are used to assess the current systemic risk contributions of financial institutions and does not highlight future systemic risk. The global financial crisis has also highlighted the need to predict forward systemic risk to minimize the adverse effects of systemic event. Adrian and Brunnermeier (Citation2016) postulate that the regulations introduced during the time of crisis make the event worse. This lays down foundation for introducing counter-cyclical policies, i.e. controlling the systemic risk when it is building. The forward measure of Adrian and Brunnermeier (Citation2016) can be used to measure the build up of systemic risk during the expansion periods. According to Adrian and Boyarchenko (Citation2012), low volatility in booms entices the managers of financial institutions to take more risk which eventually makes them more vulnerable to shocks.
The banking sector of Pakistan has shown robust growth in the recent times. The happening of systemic event can adversely affect the phenomenal growth achieved during the recent times. This warrants a need to estimate the forward systemic risk of financial institutions as forward ∆CoVaR addresses the issue of procyclicality and lays down a solid foundation to introduce countercyclical macroprudential policies. Moreover, the role of sector level variables in building forward CoVaR is not assessed till date which warrants a need for examination. The comparative analysis of forward CoVaR predicted with and without sector level variables can elucidate the significance of sectoral environment in comprehending the risk dynamics. Similarly, the analysis of forward systemic risk allows the policy makers to address the issue of procycality and introduce countercyclical regulations before the happening of systemic event. The results present new insights on the drivers of financial instability in Pakistan and provide implications for the micro- and macroprudential regulations of the banks including the Pigovian tax.
2. Literature review
This section starts with literature on contemporaneous and forward systemic risk measures that is followed by description of sector level variables that are expected to influence the prediction of forward systemic risk. A brief account of the relationship between forward and contemporaneous CoVaR is also elucidated.
2.1. Systemic Risk measures
2.1.1. Contemporaneous CoVaR
Adrian and Brunnermeier (Citation2011) introduced conditional value at risk (CoVaR) to measure systemic risk contribution of an individual financial institution. They define CoVaR as the value at risk (VaR) of the market returns conditional on the distress of a financial institution. It is one of the most flexible measures as it can be used to examine systemic risk contributions of countries, markets and institutions. This measure is used by many researchers since it is proposed by Adrian and Brunnermeier (Citation2011). For instance, Lee et al. (Citation2019) apply ∆CoVaR to analyze systemic risk of the banking sector in Korea. Furthermore, Kleinov and Nell (Citation2015) apply ∆CoVaR to assess the systemic risk of European banks.
2.1.2. Forward ∆CoVaR
Later on, Adrian and Brunnermeier (Citation2016) argue that conventional CoVaR measure is parsimonious in nature and is used to assess the current systemic risk contributions of financial institutions and does not account for the volatility paradox. Implementing the regulations on the basis of contemporaneous CoVaR can be counterproductive as it can further aggravate the problem and expose the economy to procycality pitfall. To overcome this limitation of conventional CoVaR, Adrian and Brunnermeier (Citation2016) introduce forward to predict future systemic risk.
2.2. Sector level variables
2.2.1. Concentration (HHI Index)
Industry concentration refers to the level of competitiveness with more concentration referring to less competition and vice versa. Previous literature presents mixed evidence on banking concentration. For instance, Kleinov et al. (Citation2017) report that concentration increases stability and reduce systemic risk. Similarly, Beck et al. (Citation2006) postulate that increase in competition can increase the fragility of the financial system. Meanwhile, Jiménez et al. (Citation2013) also divulge that low competition (high concentration) reduces risk taking. Concomitantly, Anginer et al. (Citation2014) use Hirschmann-Herfindahl Index to measure concentration and report insignificant impact on systemic risk. HHI is the sum of the squares of the market shares (assets) of each bank in the financial system.
2.2.2. Munificence
Beard and Dess (Citation1984) define munificence as the capacity of an environment to maintain a persistent growth. According to Almazan and Molina (Citation2005) high munificence leads to higher level of opportunities which can eventually augment the financial performance of the organization. Extant literature attributes higher growth in banking sector to the buildup of systemic risk. The empirical research on credit booms and financial crises divulge positive impact of credit expansion on financial stress (Crowe et al., Citation2011; Dell’Ariccia et al., Citation2014). The relevance of credit volume is also highlighted by Zeda and Kannas (Citation2017). They espouse that during good times the lending activities of the banks grow which results in amplification of systemic risk.
2.2.3. Dynamism
Generally, dynamism measures the extent to which an environment is stable or unstable (Smith et al., Citation2014). By definition, more dynamic environments are less stable. Firms operating in a dynamic environment tend to deal with more uncertainty regarding growth (Boyd, Citation1995). As discussed earlier systemic risk builds up in tranquil times. In contrast, dynamism refers to the situation in which the environment is less stable. Moreover, Adrian and Boyarchenko (Citation2012) explicate that low volatility in booms entices the managers of financial institutions to take more risk which eventually makes them more vulnerable to shocks. Resultantly a proposition can be developed.
2.3. Forward and Contemporaneous ∆CoVaR
According to Adrian and Boyarchenko (Citation2012), systemic risk breeds in tranquil times. If policy makers don’t address that volatility paradox, it might expose the financial system to procycality pitfall. Consistent with these arguments, Adrian and Brunnermeier (Citation2016) highlight the need to analyze the forward systemic risk as contemporaneous CoVaR does not divulge the buildup of systemic risk. They introduce forward
to predict future systemic risk. The main factors that were considered to predict forward
are size, leverage, maturity and market to book value. The results divulge that size, leverage and higher equity valuation as factors significantly influencing
one quarter, one year and two years later. Furthermore, Adrian and Brunnermeier (Citation2016) propose that other balance sheet variables can also be linked to forward
. Besides that, they also use forward
to predict realized
during the crisis and divulge that two year ahead forward
as of the end of 2006Q4 was able to explain over one third of the variation of realized
CoVaR during the crisis.
3. Data and methodology
This section sheds light on the data collection and data analysis techniques used in the study.
3.1. Sources of data and sample population
As discussed in the introduction section only financial institutions are source of contagion and Souza et al. (Citation2015) even state that contagion is only generated by banks so only financial sector is included in the analysis. According to state bank of Pakistan nonbanking financial institutions of Pakistan are only 6% of the banking sector. These figures dilute the systemic importance of non-banking financial institutions in Pakistan and good part of systemic risk literature also highlights that only banks are a source of contagion. In the light of these arguments only banks are included in the sample. There are 35 scheduled banks operating in Pakistan. All the listed banks with data availability are included in the sample. This makes the sample of study 20 banks. The reason behind selecting the listed banks is requirement of stock prices to compute measures of systemic risk. In the absence of stock price data these measures cannot be estimated.
The data on country level variables is extracted from Publications of State Bank of Pakistan, IMF, World Bank Governance and Development Indicators, Economic Surveys and Federal Bureau of Statistics. Weekly data on share prices of the banks listed at Pakistan Stock Exchange is retrieved from Brecorder.com. The frequency of state variables and bank returns is weekly to be computed for the calculation the contemporaneous ∆CoVaR. In order to match the frequency of institution and sector level variables, annual Geometric mean of the weekly ∆CoVaR is computed and is multiplied by market value of Equity of financial institution and divided by cross-sectional average. Forward ∆CoVaR of 2006 and 2007 is computed using the methodology mentioned in the paper and is used to predict variation in contemporaneous ∆CoVaR in 2008. The frequency of state variables, institution characteristics and sector level variables is set at annual (annual average of state variables is calculated) to compute forward ∆CoVaR.
3.2. Variables
The following section provides the measurement of dependent and independent variables along with the empirical evidence on the measurement. The discussion of measurement of contemporaneous and forward systemic risk is presented below.
3.2.1. ∆ CoVaR (Contemporaneous systemic risk)
The CoVaR sheds light on the return of the financial system when value at risk of the individual institution is breached. Adrian and Brunnermeier (Citation2016) introduce ∆CoVaR and applied quantile regression as an estimation approach. Koenker and Bassett (Citation1978) introduced quantile regression technique and later on augmented the initial contribution (Koenker, Citation2005). It is a comparatively more refined form of ordinary least squares regression as OLS results divulge conditional mean of the response variable, whereas quantile regression unveils the conditional median or any other quantile of the response variable. This makes quantile regression more suitable for the analysis as 1% value at risk is to be calculated for computation of CoVaR.
Adrian and Brunnermeier (Citation2016) define as the
of institution j (or of the financial system) conditional on some event C(
) of institution i. Then
is the
quantile of the conditional probability distribution of returns of j.
The CoVaR of the financial institution is applied in five stages. The first stage is to assess the returns of institution “i” as a function of state variables as referred by Adrian and Brunnermeier (2014).
This analysis incorporates value from publically available data hence, (Return, Losses) are computed as
In Equationequation (3.1)(3.1)
(3.1)
is the constant,
is the vector of lag of state variables and
is the error term. After that 1% quantile of market returns is estimated using quantile regressions.
In the second stage 1% Value at risk of every financial institution is computed. All the insignificant variables in EquationEquation (3.2)(3.2)
(3.2) are eliminated and only significant variables are incorporated in EquationEquation (3.3)
(3.3)
(3.3) .
In EquationEquation (3.3)(3.3)
(3.3)
are estimates from EquationEquation (3.2)
(3.2)
(3.2) .
In the third step return of financial system (portfolio consisting of all the banks listed at Pakistan stock exchange) will be computed using equation (4).
In EquationEquation (3.4)(3.4)
(3.4)
is the constant,
is the contribution of financial institution i to the returns of financial system,
is lag of the set of state variables and
is the error term. After that, 1% quantile of returns is computed using quantile regressions.
In the fourth stage CoVaR will be computed that is VaR of the system conditional on value at risk of individual financial institution “i” at 1% quantile. In order to compute CoVaR, value at risk (1%) from EquationEquation (3.3)(3.3)
(3.3) and all the significant state variables from EquationEquation (3.4)
(3.4)
(3.4) will be computed in EquationEquation (3.5)
(3.5)
(3.5) .
In the last stage ∆CoVaR is estimated. It is the difference between CoVaR of the system at 1% and 50% quantile. The calculation of 50% CoVaR is similar to that of 1% quantile
In addition to that, Adrian and Brunnermeier (Citation2016) introduce that also considers the size of institution “i.” The utility of this measure largely increases while making cross-sectional comparisons institutions with different size. Adrian and Brunnermeier (Citation2016) quantify size by market value of equity and calculate
as
State Variables
According to Adrian and Brunnermeier (Citation2016), state variables are used to condition the mean and variability of risk measures and should not be construed as systemic risk factors. The first function of state variables is to capture the time variation in certain conditions. Furthermore, they are also liquid. The lags of state variables are used in estimations. In order to calculate time varying VaR and CoVaR following state variables are used.
∆ Three months yield The data is published by State Bank of Pakistan. The weekly change in three-month Treasury bill rate is used as change captures the extreme returns better than level.
Weekly Market Returns The weekly market return is calculated from KSE100 Index.
∆ Slope of the yield curve The weekly change in slope of yield curve is calculated by taking the difference between long term bond and Treasury bill rate. This data is also retrieved from the publications of State Bank of Pakistan.
Inflation rate Consumer Price Index is incorporated in the analysis as a proxy for the inflation rate of Pakistan. Monthly inflation rate (Chen, Wu, Jeon & Wang, Citation2017) is extracted from the website of State Bank of Pakistan and data frequency is collapsed in to weekly.
Equity volatility Equity volatility is calculated as the 22day rolling standard deviation of the weekly KSE100 index equity market return.
Credit Spread The change in credit spread is weekly difference between Moody’s Baa rated bonds and ten-year treasury bond rate.
3.2.2. Forward CoVaR
In a subsequent study, Adrian and Brunnermeier (Citation2016) argue that conventional CoVaR measure is parsimonious in nature and is used to assess the current systemic risk contributions of financial institutions and does not highlight future systemic risk. To overcome this limitation of conventional CoVaR, Adrian and Brunnermeier (Citation2016) introduce forward to predict future systemic risk. Forward systemic risk is computed as a function of firm characteristics i.e. size, leverage, maturity mismatch and a boom indicator and state variables. Forward
can be used to predict realized systemic risk that makes it an important development in the analysis of systemic risk.
Adrian and Brunnermeier (Citation2016) compute forward for each firm by regressing the
on institution i’s characteristics and state variables. More specifically, for a forecast horizon h = 4 and 8 quarters (1 and 2 years), the estimated regressions are
In equation (8), is set of institution i characteristics,
is set of state variables lagged “h” quarters and
is the error term.
The h quarters (years) predicted value is termed as forward.
Adrian and Brunnermeier (Citation2016) emphasize that systemic risk breeds in tranquil times when contemporaneous volatility is low and growth rate is rising in the credit market. This lays down a solid foundation to use munificence and dynamism as predictors of forward systemic risk. Literature also highlights concentration as important determinant of contemporaneous systemic risk (Kleinov et al., Citation2017; Strobl, Citation2016). Therefore, this study also incorporates sector level variables in addition to state and firm level variables to predict the forward CoVaR that modifies the EquationEquations (3.8(3.8)
(3.8) ) and (Equation3.9
(3.9)
(3.9) ) to,
The results of forward CoVaR computed with and without sector level variables are used to forecast realized ∆CoVaR. The forecast horizon in the study is 1 and 2 years.
Institution’s Characteristics
Leverage is calculated as debt to equity ratio
Size is calculated as logarithm of total assets
Market-to-book, defined as the ratio of the market to the book value of total equity (Adrian & Brunnermeier, Citation2011).
Sector Level Variables Proposed in This Research
Munificence
Dynamism
Concentration
State Variables
State variables are same as mentioned in the calculation of CoVaR.
Sector Level Variables Formulation and Expected Influence on Systemic
Risk (3.1)
3.3. Data analysis techniques
Consistent with the aforementioned arguments the study attempts to examine the ability of forward CoVaR to predict the realized
CoVaR. For that purpose, forward
CoVaR is calculated for each bank. Two-year forward CoVaR is calculated up to 2006 and one year forward
CoVaR up to 2007 using the variables specified by Adrian and Brunnermeier (Citation2016). In addition to that, forward CoVaR is also calculated by incorporating sector level variables in the model of Adrian and Brunnermeier (Citation2016). Afterward, the realized
CoVaR of 2008 is regressed against the two year and one year forward
CoVaR estimated with and without sector level variables. EquationEquation (3.12)
(3.12)
(3.12) includes forward CoVaR with bank and state level variables whereas in EquationEquation (3.13)
(3.13)
(3.13) bank, sector and state level variables are incorporated to compute. forward CoVaR.
Firm and State Level Variables
Forward CoVaR with Firm, State and Sector Level Variables
The results of both the models will be used to draw comparison as if incorporation of sector level variables improve the forecasting ability of forward CoVaR.
4. Results and interpretations
4.1. Summary statistics
Table presents the summary statistics of the state variables used in the analysis to compute . The difference between long-term and short-term treasury rate is showed by Term spread and difference seems to be sizeable. The mean value of Change in yield of T-bill is not high that shows low volatility in short-term risk-free rates.
Table 1. Descriptive statistics of state level variables
Table shows average Munificence of the banking sector is high at 10.11 highlighting the robust performance of banks. Dynamism is also high with mean value of 2.96. The environmental dynamism was at its peak during 2008–2009.
4.2. Predicting forward CoVaR using contemporaneous CoVaR
Consistent with the objectives of the study, the issue of procycality can be addressed by analyzing a forward-looking measure of systemic risk. As discussed previously, Adrian and Brunnermeier (Citation2016) suggest inclusion of bank and country level variables to determine forward ∆CoVaR. Table shows the summary statistics of firm and sector level variables. Firstly, size CoVaR is regressed on bank and country level variables to compute forward CoVaR. Two-year forward CoVaR is calculated by incorporating two years lag of bank and country level variables discussed in section three. The predicted value is two years forward CoVaR. The two-year forward CoVaR is calculated up to year 2006. Subsequently, one-year lag of bank and country level variables are computed to predict one-year forward CoVaR. Once the forward CoVaR is calculated, the predicted value is used as independent variable to assess as if forward CoVaR explains any variations in realized CoVaR of 2008 (crisis CoVaR). The dynamic model highlights the significance of lag of ∆CoVaR as significant predictor of current ∆CoVaR but analysis of forward CoVaR brings more sophistication and clarity in the examination. The results in Table suggest that forward CoVaR significantly explains variations in realized CoVaR. Two-year forward CoVaR explains nearly 26% variation in realized CoVaR, whereas one-year forward CoVaR explains more than 33% variation in realized CoVaR.
Table 2. Summary statistics of institution and sector level variables
Table 3. Forward and contemporaneous CoVaR (without sector level variables)
In addition to the bank and state variables suggested by Adrian and Brunnermeier (Citation2016), the study also incorporates two-year and one-year lag of sector level variables munificence, dynamism and concentration to predict one and two years forward CoVaR. The forward CoVaR predicted with sector level variables is used as an independent variable to assess variation in contemporaneous CoVaR. The results in Table suggest that both one year and two years forward CoVaR are significant in explaining variation in realized CoVaR. The forward CoVaR predicted without sector level variables is also significant in explaining variation in realized CoVaR. The question arises whether the forward CoVaR predicted with sector level variables is better at explaining the realized CoVaR or not. It can be discerned from Table that two years forward CoVaR explains nearly 39% variation in realized CoVaR. In order to make comparison, Table show that two-year forward CoVaR predicted without sector level variables explains only 26% variation in realized CoVaR. Moreover, Table show that one-year forward CoVaR predicted with sector level variables explain nearly one half of variation in realized CoVaR. The explanation power significantly jumps from 33.06% to 49.22%. The results confirm that sector level variables improve predicting forward CoVaR along with bank and state level variables.
Table 4. Forward and contemporaneous CoVaR (with sector level variables)
5. Conclusion
The study introduces an innovation drive by introducing sector level in the estimation of forward CoVaR. Adrian and Brunnermeier (Citation2016) incorporated bank and country level variables to predict forward CoVaR. This study extends the work of Adrian and Brunnermeier (Citation2016) by incorporating sector level variables along with bank and country level variables to predict forward CoVaR. The results signify that forward CoVaR (predicted with sector level variables) explains more variation in realized CoVaR than the one estimated without sector level variables. The results augment the existing literature by providing empirical evidence on the significant role of sector level variables in the modeling of contemporaneous and forward CoVaR that revolves around bank and country level variables. The study introduces methodological innovation in computation of forward systemic risk that can be used to introduce stability in the financial system and economy as a whole. Analyzing forward systemic risk of financial institutions risk is very important as systemic event has repercussions for whole economy. As the inclusion of sector level variables improve the forecasting ability of forward CoVaR, regulators can rely on current innovation and also peruse the role sector level variables in the build-up of systemic risk. This refers to having a close eye on munificence, dynamism and concentration of banking sector. The 2008 financial crisis revealed that systemic risk can quickly spread through the economy causing negative macroeconomic shocks. The study lays down a solid foundation to predict the build-up of systemic risk and brings in complete hierarchy of variables. Furthermore, analysis of forward systemic risk allows the policy makers to address the issue of procycality and introduce countercyclical regulations before the happening of systemic event. It is very important to address the issue of procycality pitfall as introducing stringent regulations during the crisis can further aggravate the crisis. This study brings in the counter-cyclical aspect of systemic risk by introducing methodological innovation that improves the forecasting ability of the forward systemic risk. The findings of the study can be used to curb the build-up of systemic risk when the activities of financial institutions appear to be normal or even showing growth. This could also include the imposition of Pigovian tax on financial institutions with high forward systemic risk to minimize the negative cost of externalities.
Additional information
Funding
Notes on contributors
Hasan Hanif
Hasan Hanif, a PhD candidate at SZABIST Islamabad and lecturer at Air University Islamabad. I am supervising research projects of MBA and MS students. Moreover, I have research publications indexed in ESCI, Scopus and HEC recognized research journals. My area of interest is risk management.
Dr. Muhmmad Naveed, associate professor at SZABIST Islamabad. I am supervising research work of MS and PhD students. I have research publications indexed in ESCI, Scopus and HEC recognized journals. I have research papers on dividend policy and risk management.
Dr. Mobeen Ur Rehman is working as an associate Professor of Finance and works in the field of financial econometrics, financial economics and financial markets. He has many journal publications in his area of expertise and also presented his work in many international conferences. He also acts as reviewer of many impact factor journals.
References
- Acharya, V. (2009). A theory of systemic risk and design of prudential bank regulations. Journal of Financial Stability, 5(3), 224–12. https://doi.org/10.1016/j.jfs.2009.02.001
- Adrian, T., & Boyarchenko, N. (2012). Intermediary leverage cycles and financial stability, FRB of New York Staff Report, 567. doi: 10.1094/PDIS-11-11-0999-PDN.
- Adrian, T., & Brunnermeier, M. (2016). CoVaR. American Economic Review. https://doi.org/10.1108/JRF-11-2016-0141.
- Adrian, T., & Brunnermeier, M. K. (2011). CoVaR. NBER Working Paper 17454. National Bureau of Economic Research, Inc.
- Almazan, A., & Molina, C. (2005). Intra-industry capital structure dispersion. Journal of Economics and Management Strategy, 14(2), 263–297. https://doi.org/10.1111/j.1530-9134.2005.00042.x
- Andrieş, A. M., Nistor, S., & Sprincean, N. (2018). The impact of central bank transparency on systemic risk—Evidence from Central and Eastern Europe. Research in International Business and Finance, 51 (C), 1-14. https://doi.org/10.1016/j.ribaf.2018.06.002
- Anginer, D., Demirguc-Kunt, A., & Zhu, M. (2014). How does competition affect bank systemic risk? Journal of Financial Intermediation, 23(1), 1–26. https://doi.org/10.1016/j.jfi.2013.11.001
- Avramidis, P., & Pasiouras, F. (2015). Calculating systemic risk capital: A factor model approach. Journal of Financial Stability, 16, 138–150. https://doi.org/10.1016/j.jfs.2015.01.003
- Bartram, S. M., Brown, G. W., & Hund, J. E. (2007). Estimating systemic risk in the international financial system. Journal of Financial Economics, 86(3), 835–869. https://doi.org/10.1016/j.jfineco.2006.10.001
- Beard, W., & Dess, G. (1984). Dimensions of organizational task environments. Administrative Science Quarterly, 29(1), 52–73. https://doi.org/10.2307/2393080
- Beck, T., Demirgüç-Kunt, A., & Levine, R. (2006). Bank concentration, competition, and crises: First results. Journal of Banking & Finance, 30(5), 1581–1603 doi:10.1016/j.jbankfin.2005.05.010
- Billio, M., Getmansky, M., Lo, A. W., & Pelizzon, L. (2012). Econometric measures of connectedness and systemic risk in the finance and insurance sectors. Journal of Financial Economics, 104(3), 535–559. https://doi.org/10.1016/j.jfineco.2011.12.010
- Boyd, B. K. (1995). CEO duality and firm performance: A contingency model. Strategic Management Journal, 16(4), 301–312. https://doi.org/10.1002/smj.4250160404
- Chen, M., Wu, J., Jeon, N. B., & Wang, R. (2017). Monetary policy and bank risk-taking: Evidence from emerging economies. Emerging Markets Review, 31, 116–140. https://doi.org/10.1016/j.ememar.2017.04.001
- Crowe, C., Dell’Ariccia, G., Igan, D., & Rabanal, P. (2011). Policies for macrofinancial stability: Options to deal with real estate booms. IMF Staff Discussion.
- Dell’Ariccia, G., Laeven, L., & Marquez, R. (2014). Real interest rates, leverage, and bank risk-taking. Journal of Economic Theory, 149 (C), 65–99. https://doi.org/10.1016/j.jet.2013.06.002
- Huang, X., Zhou, H., & Zhu, H. (2009). A framework for assessing the systemic risk of major financial institutions. Journal of Banking & Finance, 33(11), 2036–2049. https://doi.org/10.1016/j.jbankfin.2009.05.017
- Jiménez, G., Lopez, J. A., & Saurina, J. (2013). How does competition affect bank risk-taking?. Journal of Financial Stability, 9(2), 185–195 doi:10.1016/j.jfs.2013.02.004
- Kayo, E., & Kimura, H. (2011). Hierarchical determinants of capital structure. Journal of Banking and Finance, 35(2), 358–371. https://doi.org/10.1016/j.jbankfin.2010.08.015
- Kleinov, J., Horsch, A., & Molina, M. G. (2017). Factors driving systemic risk of banks in Latin America. Journal of Economics and Finance, 41(2), 211–234. https://doi.org/10.1007/s12197-015-9341-7
- Kleinov, J., & Nell, T. (2015). Determinants of systemically important banks: The case of Europe. Journal of Financial Economic Policy, 7(4), 446–476. https://doi.org/10.1108/JFEP-07-2015-0042
- Koenker, R. (2005). Quantile Regression. Cambridge University Press.
- Koenker, R., & Bassett, G. S. (1978). Regression quantiles. Econometrica, 46(1), 33–50. https://doi.org/10.2307/1913643
- Lee, J., Lin, E. M. H., Lin, J. J., & Zhao, Y. (2019). Bank systemic risk and CEO overconfidence. The North American Journal of Economics and Finance, 100946. https://doi.org/10.1016/j.najef.2019.03.011
- Smith, J. D., Chen, J., & Anderson, D. H. (2014). The influence of firm financialposition and industry characteristics on capital structure adjustment. Journal of Accounting and Finance.55(4), 1135-1169, https://doi.org/10.1111/acfi.12083.
- Souza, R. S., Tabak, M. B., Silva, C. T., & Guerra, M. S. (2015). Insolvency and contagion in the Brazilian interbank market. Physica, 43(1), 140–151. https://doi.org/10.1016/j.physa.2015.03.005
- Strobl, S. (2016). Stand-alone vs systemic risk-taking of financial institutions. The Journal of Risk Finance, 17(4), 374–389. https://doi.org/10.1108/JRF-05-2016-0064
- Zedda, S., & Cannas, G. (2017). Analysis of banks systemic risk contribution and contagion determinants through the leave-one-out approach. Journal of Banking and Finance. doi:doi:10.1016/j.jbankfin.2017.06.008