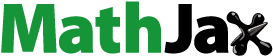
Abstract
The research investigated the impact of macroeconomic variables on the volatility of the commodity futures market in India (together with oil futures, agricultural commodity futures and metal futures). The monetary policies, financial market information and economic environments are determined by the macroeconomic variables. The low-frequency macroeconomic variables and daily price volatility is studied in the research employed by the GARCH-MIDAS model. This model simplifies the series of volatility into long- and short-run modules, which allow for the testing of the macroeconomic variables can control the long-run variance or not. The current study reveals the effect on long-run volatility factor in the commodity market, and the majority of verified data have shown that low-frequency variables have a positive impact in the long-run variance of the commodity futures market. The outcome of the study suggested that the national and international economic variables perform a substantial part in assessing the price volatility of the commodity futures market in India.
PUBLIC INTEREST STATEMENT
Research investigated the impact of macroeconomic variables on the volatility of the commodity futures market.
The low-frequency macroeconomic variables and daily price volatility is studied in the research employed by the GARCH-MIDAS model.
The study investigated the factors affecting volatility more precisely through merging the high frequency returns information with low-frequency macroeconomic factors in the existing model of the paper.
The contributory association among price unpredictability and its factors can be more precisely examined and results revealed that, the long-term variance prediction is better as it comprises of macroeconomic evidence.
1. Introduction
The price volatility of the Indian commodity futures market has significant impact on the Indian stock market. Several researchers discussed that the growth in commodity futures market is the dire requirement of embryonic Indian commodity markets. The Indian commodity markets play a very significant role in India; it is valuable to examine the factors of the volatility of commodity futures market (Baffes and Savescu (Citation2014)). The present research assessed the effects of macroeconomic factors on the unpredictability of the commodity market, by decomposing the unpredictability into short- as well as long-term mechanisms. The study found that it is a research gap to analyse the impact of macro economic variables on commodity future prices. The short-term unpredictability caused by stock market returns and currency rate, whereas the long-run volatility is influenced by macroeconomic factors. Based on the earlier research, the study identified two problems in the commodity futures market (Chai et al. (Citation2011)). The first problem is the proxy for volatility. Majority of the researches have considered long-term volatility as low-frequency volatility, which is implicit to be strong-minded by gradually developing macroeconomic variables. In connection with the above objectives, it is identified that the macroeconomic factors of the unpredictability of commodity markets are significant to research numerous causes. The first cause is to understand the volatility changing aspects is serious for manufacturers, customers and plan makers. The identified questions to address the research problems are 1. What are the factors influence the variability of commodity market price volatility 2. What are the macro economic variables impacts commodity future prices. Keep in iew the above research gap and problems the study developed its objectives. The prime objective of the research is to observe the macroeconomic variables causes of the volatility of the commodity market in India. This research study has another two minor objectives; the first one is to investigate the extrapolative power of changes in macroeconomic factors for the Indian commodity future market volatility. The next objective is to examine the effect of ambiguity in macroeconomic factors in Indian commodity futures market unpredictability. Additionally, the present research designed the hypothesis that the volatility of the commodity futures market affects the daily market rate information. Preceding research emphasis on the factors of short-term volatility; nevertheless, the volatility of the commodity futures market is affected by daily market evidence (Jegadeesh and Titman (Citation1993)). The second cause is the developing commodity markets have begun to play an important role in the international commodity market; yet, insufficient research studies are emphasizing the emerging commodity markets as pointed out by Srinivas (Citation2018). This study highlights an inclusive range of commodity future from emerging markets and studies the relationship amongst the macroeconomic variables and commodity futures in the Indian context.
The current study make the following contributions to the existing literature 1. This research adds to the consisting data in many conducts. At first, the study investigated the factors affecting volatility more precisely through merging the high-frequency returns information with low-frequency macroeconomic factors in the existing model of the paper. The contributory association among price unpredictability and its factors can be more precisely examined and results reveled that the long-term variance prediction is better as it comprises macroeconomic evidence. 2. The study has an emphasis on extensive series of commodities in the Indian emerging market. The outcomes may have significant suggestions to other emerging markets.
The suggestions of study have significant pointers for economic strategies, concerning reducing assumptions and attaining financial consistency, particularly for the policymakers in Indian emerging commodity markets. Hence, the effect of international macroeconomic factors circumstances on the volatility of commodity should be considered while making economic policies. The Indian government overlooking an emerging market must practise interpositions in fiscal and monetary policy moderately than utilising the price control in the commodities market. Finally, the study concluded that the unpredictability of commodity is not only influenced by macroeconomic variables but also affected by the international macroeconomic environment (RBI Report (Citation2015)). Hence, this study adds up to the volatility of existing data by affording further volatility factors in the Indian context.
2. Background
Since 2003, the commodity futures market has gained a rising trend and the market price attained a peak in mid of 2010. During 2010–2011, the commodity market prices suffered a great extent and had a significant decline due to the international financial market crisis. The volatility change among volume converts is an important factor in the scheme establishment for hedging, derivatives trading and portfolio optimization (Arango, Arias, Flórez (Citation2012)). The manufacturers and customers can make their investment alternatives more judiciously by recognizing the exact dynamic forces of volatility in Indian commodity markets. The commodity market exchanges in India is the largest emerging market and ranked as one of top 10 commodity exchanges market in the world. The emerging-market has begun to perform an important part in the condition of commodity prices and market instability. The prime reasons for the commodity price volatility are the government regulatory policies. The regulatory policies have shown greater impact on stock markets. So, there is a need to make a study to analyse the volatility of commodity future prices.
To acquire long-term volatility, numerous research papers used traditional GARCH models, which is usual categorical volatility as persistent in an Indian commodity market. This persistent volatility is improbable to seizure the factual changing aspects of long-term market volatility. According to Ch Murthy Chodi setty and Raja Babu (Citation2018), the discordance of data frequency among the macroeconomic variable quantity and commodity markets in India. Macroeconomic variables usually arise on a daily or monthly, while the commodity futures market charges have moderately greater data frequency, i.e., daily or intra-daily.
The daily changes in the commodity market price are caused by two important factors: the first factor is related to data that appears in the market, like the stock market returns and interest rate in the short-term period as mentioned by Rajan Lakshmi, A., (2017). The second factor is related to the macroeconomic factors updates every quarter. Divide the daily price volatility into two parameters that are triggered by each of the two factors (Morana (Citation2013)). To bridge the research gap, the study used Mixed Data Selection or sample (MIDAS) model, which permits data of diverse frequencies. The MIDAS model is one type of regressions, contains time series data experimented at several frequencies, making the investigation between high and low-frequency macroeconomic factors. According to, Engle et al. (Citation2013) a GARCH-MIDAS model inside the MIDAS background is suggested to examine the time-varying unpredictability, in this background, the provisional variance has been disintegrated into two parameters in long-term and short-term variances. The main benefit of this method is that it permits the association among the daily observation on return series and the macroeconomic variables. The influence of macroeconomic factors on the commodity market unpredictability (Batten et al., Citation2013).
3. Theoretical literature review
The commodity futures markets consist of a financial asset, but it is exclusive. Primarily, they don’t create assets for firms to enlarge their business. Moreover, commodity futures markets correspond to the prospect for future spot prices (Choi & Hammoudeh, Citation2010). The shareholders contemplate commodity futures as coverage for the expected future price of the commodity. The commodity prices will deteriorate to the response of the low demand for raw material. Though, the long-term revenues opportunities perform an inverse correlation with interest rate changes.
4. Empirical literature review and hypotheses development
The research study pursued several past types of research on commodities markets and the influence of macro-economic factors on it. Macroeconomic variables are known as a significant factor in asset prices. The dynamics change the connection to the stock market execution consist of manufacturing assembly, inflation, interest rates and oil prices fluctuation (S. Shanmugam, A. Rathgeber, T. Schlegl). A few types of research analysing the factors influencing the commodity markets contend that the commodity market variation is not the only relationship with supply-demand changing aspects. The standard financial assets such as debentures or bonds and equities are constantly deemed as firms’ liabilities, which can increase peripheral resources for the firms10. Hence, long-term prospects and interest rates are important elements for the determination of values of financial assets (Jain & Ghosh, Citation2013). The time duration will decide the operational excellence Sekhara Rao and Boya.
The study connected to macroeconomics variables consultations on commodity market price fluctuations residues back to the 2000s, when scholars and academicians contended that the macroeconomic variables performed an important part in oil, agricultural and mineral commodity market prices Sailaja. In the initial phase, the majority of the studies used CAPM (capital asset pricing models) used in equity markets to determine the commodity futures market price (Romala Vijaya Srinivas and Kafila (Citation2018)). A high-frequency dataset, examine the influence of macroeconomic statements on the gold market and illustrated that the market is obstructed by work generation, GDP, CPI and per capita income.
The recent studies (Malik & Hammoudeh, Citation2007) make use of the FAMA model with time-series data for 18 commodity products, and illustrated that shareholders are remunerated by taking interest rate hazard. In supplement, (Ch Murthy Chodi setty and Raja Babu (Citation2018)) illustrated that, the monetary policy and the fragility in the dollar have impacted the latest performance of commodity prices. These researches investigate only the features, which are properly analysed in the stock market returns in the Indian context. As there is an incredible escalation in the challenges faced by emerging markets, certain scholars have changed their emphasis to the commodity markets. Magrini and Donmez (Citation2013) contend that the commodity price change is very well connected to the economic movement of Indian developing economy. The International Monetary Fund (Citation2010) postulates that the upsurge in the Indian and other developing markets have proceeded to a structural modification in long-term price movements.
Equated to returns, volatility is an additional valuable way for containing data. It can facilitate supplementary valuable data for understanding the price structure. Consequently, more study to do examine the factors of commodity prices from a volatility viewpoint (Arouri & Nguyen, Citation2010). Identifying the volatile rates of agriculture commodities is a key parameter for manufacturers and customers. (Mishra & Smyth, Citation2016) uses the variance decomposition and panel regression model to examine the environment and causes of volatility in 21 global trade agricultural commodities.
According to Kumar (Citation2004) the existing review associated with volatility component of agricultural commodities is classified into three groups: one volatility modelling does not determine the pivotal relationship amongst commodity return volatility and their components.
Jacks and Stuermer (Citation2018) applied the GARCH model launched by Engle and Rangel (Citation2008), decomposing the volatility due to short- and long-term factors and can assess the relationship amid volatility and the macroeconomic variables. (Dumitru & Urga, Citation2012) illustrated that there is a positive association between low-frequency volatility and various commodities; moreover, inflation rate and the exchange rate contribute largely to the price hike in Indian agricultural commodities. Chinn and Coibion (Citation2014), examining the price volatility in agricultural, energy and metal futures markets for 10 years, explain that the macroeconomic variables produce parallel effects contained by the same commodity market group, and the conclusions are different across different commodity markets.
The GARCH-MIDAS model permits to integrate low-frequency macroeconomic information promptly using the mixed data testing filter. Chen et al. (Citation2014) assess the key drivers of the agricultural commodity price volatility in the US market. The outcomes indicate that the GARCH-MIDAS model presents a superior fit than the traditional GARCH model. Furthermore, over time, supply-demand values and traditional assumption and alternatives are important in describing the low-frequency factor of the volatility (Rajan Lakshmi, 2017). Chaves and Viswanathan (Citation2016), used the distorted power GARCH model to examine the macroeconomic variable impact on gold cash and futures prices. The outcome suggests that dollar price indicates a pessimistic association with the volatility of return on gold, but an optimistic relationship with the macroeconomic variables. Additionally, Power and Robinson, (2013b) investigated the macroeconomic factors of periodical price volatilities of selected valuable metals such as platinum, silver, gold and palladium. The macroeconomic elements consist of variables linked to the commercial cycle, the monetary nature and the financial market reaction. The findings for several commodities are different; in specific, gold volatility is illustrated to be described by monetary variables. Thuraisamy et al. investigated the correlation among economic variability and commodity return volatility by evaluating volatility for the cumulative commodity market and numerous factors.
According to Cuddington (Citation2003), who similarly used a GARCH-MIDAS model, to examine the influence of 4 aspects—the economic course, the budget policy, the investor sentiment, the unpredictable movement, the convenience yield and the Indian economy on 4 dealt commodities in India. The outcome indicates that the macroeconomic factors help estimate volatility for the long-run period. Concentrating on the oil price market, 35investigate the part of speculation and economic basics in the Indian oil market by using the GARCH-MIDAS model. The research outcomes indicate that the market demand shock is the only element that boosts long- or short-term oil volatility during sample time.
Macroeconomic factors are an important consideration for commodity market manufactures, hedgers, speculators and represents, which stimulate this research study. After vigilant assessment of secondary data, it is explained that most research in the field indirectly adopt that the effect of macroeconomic factors on the volatility can be whichever showed over daily data through financial market variables pretentious no impact openly on the daily volatility while concrete bulletin is out, or over the monthly volatility of the commodity market, in Europe also it is same. To bridge the research gap, the paper creates the query “the macroeconomic variables define the long-run volatility of commodity in the emerging market like India?” and makes use of a complicated model to examine daily volatility produced by high-frequency variables and low-frequency variables. Applying the GARCH-MIDAS model, the study also examined the association of low-frequency variables with the macroeconomic variable’s performance. The study derives a list of prospective macroeconomic variables that will be analysed.
5. Research design
This research study used commodity futures information from the Indian emerging economy. The information of daily closing prices acquired from the MCX and CMIE database. According to Brooks and Prokopczuk (Citation2013), many kinds of commodities are different; hence, it is important to consider them as a single level commodity other than as a whole asset parameter. Present study prudently selected the commodities; the commodity has been selected from three commodity areas, which furnishes a better understanding of the distinctive of the whole commodity market. The three sectors are agriculture, energy and metals. More preciously, the focus was on copper, aluminium, gold, soybean, corn, sugar, nickel, mustard seed, castor seed and crude oil. Commodity futures deals data has been taken from the MCX and CMIE database. The study has been selected macroeconomic variables such as: Consumer Confidence Index, Consumer Price Index, Industrial Production Index, Short-term Interest Rate, Term-spread, Stock Market Index, Composite Economic Leading Indicator, Exchange Rate and Money supply from the literature review and the selected period from 2003 to 2020 for the data analysis on commodity futures in the Indian market.
The commodity futures are scheduled on various futures exchange on a different period, hence, the illustration periods vary for futures agreements at multiple levels, but the study consists the extensive period probable for each commodity futures, that is given in . Concerning the time series data returns, the log profit is applied for conformity relationship between the commodity sectors. The model is explained below.
Table 1. Macroeconomic variables and select data period of commodity futures
From equation no 1, where, Rt is indicated that the log return at time t and Pt is the daily market price at time t.
Observes: presents a summary of statistics for Indian commodity futures market returns. Mean, median, std. dev, skewness, maximum, minimum are express returns in percentage. The other tools like kurtosis, Jarque-Bera are used to verify whether the residuals of the return series values follow a normal distribution or not, while all statistics summary should equal zero. ***, **, * denote insignificance of the null hypothesis at 1%, 5% and 10% levels, correspondingly.
Table 2. Summary statistics of Indian commodity futures market returns (monthly data from 1/1/2003 to 1/8/2020)
explores the statistics for the India commodity futures market. Observation, Mean, median, standard deviation, skewness, kurtosis, maximum and minimum values are obtained in percentage. While some of the commodity products in commodity market have a similar data period, the commodity market might have a few days with mislaid values. In this connection, this study ignores the days of misplaced numbers, as an outcome, the commodities have separate numbers of samples. The table shows the average earnings of all commodities futures market in India are encouraging and nearby to zero value, apart from aluminium. The standard deviation of revenues of copper and aluminium are the lowermost among all commodity futures products, although the standard deviation of crude oil is 2.206%, which totals as the maximum values. This shows the high risk limited in crude oil prices. In relations of the kurtosis and skewness, the statistics illustration that Castor seed, Nickel, copper, and sugar are negatively skewed, while aluminium, gold, soybean meal, soybean oil, corn and crude oil have positive kurtosis. It is observed that, majority of commodity futures illustration the positive Skewness. The Jarque-Bera tool was applied to test the hypothesis that residuals values are normally distributed. The Jarque-Bera tool was used for all commodity products in the Indian market, the commodities are found to be more than the accepted value or 1% critical value. In this view, it can be concluded that all earning series are not normally distributed.
5.1. Methodology
The methodology of this research study to ascertain the effect of macroeconomic variables on the price volatility of commodities, the research used the GARCH-MIDAS model for assessment of the volatility percentage in commodity products. The GARCH-MIDAS model, initially projected by Engle et al. (Citation2013), is calculated to indicate data with various frequencies. Valkanov (Citation2006), illustrated the use of various MIDAS regressions analysis to study the standard risk-return trade-off. As used in Engle and Rangel (Citation2008), explain the macroeconomic variables to permits the integration of low-frequency financial and macroeconomic variables. The study followed the methodology presented by Engle and Rangel (Citation2008)40, the unpredicted earnings can be illustrating as follows:
From the equation 2, the variables explain hereby the where ri,t, is indicating that the log return i during time) t; this sub variables explain here is the conditional prospect in this given equation, the arbitrary period has determined the based on the t;
is the data set plus the returns up today i-1 of time t. The volatility is disintegrated into two distinct factors components: gi t, financial records for day-to-day variance, assuming that the
) is equal to µ from this we can frame the following equation is as follows.
This study used the assumed volatility changes of the factor gi,t, explains that the daily processing of the GARCH model, which can frame the equation according to as follows:
From the Equationequation 4(4)
(4) , where α, β, is different to the technique shown by Shang et al. and additional conformist methods, which applied the previous value as the size of interest, the GARCH and MIDAS models assumed long-run period factor by a weighted function of the macroeconomic variables impact on the commodity markets.
From Equationequation 5(5)
(5) , the study illustrated that the values of the equation, where, RVt is indicating that the commodity futures market volatility at the time of fixed, t explains that the following form:
Further, from Equationequation 5(5)
(5) hereby providing that the φk (ω1ω2) the purpose of explaining the weighted average value of the MIDAS model filters. Hence, this paper contemplated only the Beta function, which is framed in the model as:
From Equationequation 6(6)
(6) , it can be observed that, where
the weights combinations. These model advantages are oppressed to associate different representations with several t time durations and different values of lags K. the fixed duration τt factor: principally, from the equation (4), which parallels to the class of models that permit to determine macroeconomic variables. It can be framed by the following equation:
The equation 7 illustrates that, where RVt-k denotes that the k is the lag of the covariance, the study is explained here by the link to the low-frequency factor τt. The Equationequation 7(7)
(7) signifies the key factor for structure direct connections between price volatility and macroeconomic factor. To integrate the macroeconomic time-series data, the GARCH and MIDAS model has to be accustomed. From equation 7, the volatility can be reformed based on the following macroeconomic variables:
The equation 8 explains, where, is illustrated, the k is the lag of a macroeconomic factors and it will be connected to the low-frequency factor of market volatility. The L is representative that the values are grouping the level of the macroeconomic factors.
5.2. Determination of macroeconomic variable’s volatility
By volatility, the study measured the scheduled variance in the macroeconomic determined variable. Particularly, the study used an autoregressive method with periodical models to evaluation regular macroeconomic volatility in the commodity market:
The equation 9 explains where the components (εt)2 hypotheses the quarterly volatility time-series data for any macroeconomic factors Xt. The assumed variables AQ for the evaluation of seasonality in the time data, and were particularly such that AQ2 is for the periodical data. AQ3 and AQ4 have explained the variance of the commodity futures market volatility.
6. Empirical results and discussion
The study captures the dynamics of variance into short-run and long-run factors by applying the GARCH and MIDAS model. The study used an extensive series of macroeconomic factors that include the economic situation, monetary policy and macroeconomic variables. The study assumed that the nominated macroeconomic variables can regulate the long-term variance of the commodity futures market in India. The study also assessed the controlled variables in the equation no (5), The results are presented in . The study examines the macroeconomic factors of the long-run variance based on individual commodity futures markets. explain the outcomes of domestic macroeconomic parameters at equal and volatility in the commodity market, correspondingly, explains the outcomes of macroeconomic factors at equal and volatility in the commodity market. Finally, demonstrates the outcomes of macroeconomic determining factor at equal and volatility in the commodity market, individually.
Table 3. Parameter evaluations for GARCH-MIDAS model with realized variance values in the Indian commodity futures market
Table 4. Assessment outcomes θ for domestic macroeconomic variables determining
Table 5. Assessment 0utcomes θ for international macroeconomic variables
In sequence to construct an appropriate model with macroeconomic time sequences data study, it is critical to give meaning to the finest time duration “t” and MIDAS model lag period determined the k, which are applied in the MIDAS method details of the τt element. The outcomes propose that the low-frequency is finest defined by the scheduled specification. Additionally, the maximum quantity of lags is carefully chosen to make the finest use of the BIC (Bayesian Information Criteria). illustrates that the Indian commodity market and the volatility of selected macroeconomic variables determined factors expect copper and gold can be a decent substitution for the long-run period of volatility in the Indian market. discusses the coefficient estimates of the GARCH and MIDAS model with volatility as assessed by equation 3 to 9 it shows θ is the variable of interest, which illustrates the association among the volatility of MIDAS lag period and the low-frequency factors of volatility. In the Indian commodity markets, in all factors θ is powerfully worth and positive, apart from gold, copper and crude oil. This specifies that the evidence confined in the log of the variance of MIDAS lag period and it supports to clarify that the low-frequency factor of volatility and the elevated levels of previous t log of variance control to a higher level of τ. Here, the log of variance in copper, crude oil and gold has a significant and positive impression on the low-frequency element of volatility in the Indian market.
shows the outcomes of the domestic macroeconomic variable’s performance in the Indian market and illustrates that the outcomes of the international macroeconomic determining factor. illustrates the outcomes of the association amid the macroeconomic factors and the volatility of commodity in the commodity market. Considering the economic viewpoint, the effect of macroeconomic variable quantity on commodity market volatility is insignificant. Further, the volatility of the commodity market is significantly determined by the macroeconomic variables. More precisely, commodity supply from several segments responds to deviations in macroeconomic circumstances in a different aspect. The economic actions and the monetary situation (IPI, GDP and MS) indicate a proper and optimistic connection with gold, copper and aluminium. This shows that when IPI, GDP and MS upsurge, the volatilities in gold, copper and Aluminium upsurge too. The upsurge in gross domestic product replicates the economic development. The growth in money supply replicates the economic factors indicating poor, and the commodity market prices are extra volatile in the recession time. Though, variables influence that signifies economic actions, and monetary situation is revealed to hurt agricultural commodity and crude oil markets. This opposite association may be a reason for the more government involvements in markets. The unpredictability in the agricultural commodity market might principal to either quick or excess of money supply, which will extremely harm both customers and manufacturers. CCI is an economic important factor, which denotes the upcoming prospect of the business series. It shows that there is a substantial negative association with the volatility of the commodity market. This specifies that the decline in the prospects of the business cycle will root an upsurge in the volatility of Indian commodity futures market and also indicated that the customers’ assurance in the market has a substantial negative association with the volatility of commodity futures, apart from the crude oil. Lastly, the actual exchange rate directs that the growth in Indian Rupees reasons a reduction in the volatility of the commodity market, excluding for agricultural commodities and crude oil. Later investigation of the effect of macroeconomic variables at level, the study surveys the impact of volatility of domestic macroeconomic variables on commodity futures volatility. makes available evidence about the connection among the volatility of the commodity market and the fluctuations of the macroeconomic variable. Equated to the outcomes of the level and the outcomes of volatility indicates a well economic implication. Thus, the analysis in the above table specifies that the upsurge in the volatility of CPI inflation roots an upsurge in commodity market fluctuation. As an outcome, its influence on the volatility of the commodity market can be either optimistic or adverse. In relations to stock market volatility, the outcomes explain that all commodity futures volatility is certainly associate with stock market volatility. This shows that the volatile stock market primes to an upsurge in the volatility of the commodity futures market.
As per the analysis given in the above table, it is found that CCI indicates a positive association amongst the volatility of economic factors of the commodity market and the volatility of commodity price volatility, apart from crude oil concern in the context of international market level. The economic implication of the CCI volatility is comparatively equated to remaining macroeconomic volatilities. The results show that the volatility of CPI is significantly negatively connected to the volatility of the commodity futures market at international standard level, excluding for metals. The volatile IR shows that customers are re-approximating the probability of the future more normally. The outcomes of the exchange rate of India are different from the different commodities prices. Based on the results given in , the study can be accomplished that the agricultural commodities are additionally pretentious by the ambiguity in the US dollars value. Since the Indian commodity market has played an important part in the International context, the volatility of the Indian commodity markets may not be exaggerated by the macroeconomic environments of the Indian market only. In this connection, the study observes that the impression of the US macroeconomic variables on the volatility of Indian commodity futures is evident; the outcomes confirm from . indicates the association among the US macroeconomic variables and the volatility of India commodity futures. In overall, the volatility of selected commodity market products is suggestively correlated to macroeconomic variables in the US market. Eliminating the metals and the remaining all additional commodity futures products indicates an important positive relation with CCI. This specifies that an upsurge in price rises in the US market causes an escalation in volatility in Indian commodity futures. In relations to IPI, the outcomes illustrated that the macroeconomic variables are negatively associated with commodity futures volatility, apart from the copper and crude oil. Though, the outcomes for MS (money supply) are diverse. In the relation of financial markets data, the answers display that, the volatility of gold, sugar and crude oil is suggestively and negatively correlated to the money supply. This shows that a rise in the stock market return will lead to a reduction in commodity market volatility. Finally, it can be concluded that the macroeconomic variables display a negative association with metals commodities, except for CPI and interest rate. Concerning the volatility series of macroeconomic variables, the important macroeconomic volatilities display a negative association with the volatility of metals. This negative association depends on the outcome of the Indian commodity market. The exclusions give the impression in CPI, IPI, MS and SMI, which indicates a noteworthy positive relationship with the volatility of agricultural commodities.
7. Summary and conclusion
The volatility of commodity is correlated with high-frequency market data. Though, the effect of macroeconomic factors should be considered, particularly throughout the stages when commodities from diverse industries interchange in a similar way. The study was unnoticed this because of the lack of accessible data. Since the models that can section volatility in various data frequencies are very inadequate. The study examines the macroeconomic factors of the volatility of the commodity market by applying the GARCH-MIDAS model. The model proved that the commodity market may be affected by both domestic economic conditions and the international macroeconomic environment. The information discussed from the analysis concerning the Indian market shows that both national and international macroeconomic variables have a low economic influence on the volatility of commodity futures in India. Moreover, the risk of both domestic and international macroeconomic variables is a vital factor for the volatility of commodity futures, especially for aluminium. Furthermore, the outcomes of the volatility form are additionally constant, which illustrates that, the ambiguity of macroeconomic variables had a significant positive impact on the volatility of the commodity market. The utmost stimulating outcomes seem in mustard seed, corn and crude oil commodities, which continuously display a conflicting symbol to the outcomes of other commodity markets. The possible motive is high government involvement in the market. The outcomes from the Indian market demonstrate that both national and international macroeconomic variables have a positive impression on the volatility of the commodity market.
Further, the study also supports policymakers for their decision process construction. The association between the macroeconomic variable and the volatility of the commodity markets specifies that the government policymakers must be careful on the influence of macroeconomic variables rather than utilising direct price control involvement, which may lead to counterproductive outcomes such as the development of a market in the commodity futures.
Additional information
Funding
References
- Arango, L., Arias, F., & Flórez, A. (2012). Determinants of commodity prices. Applied Economics, 44(1), 135–17. https://doi.org/https://doi.org/10.1080/00036846.2010.500273
- Arouri, M. E. H., & Nguyen, D. K. (2010). Oil prices, stock markets and portfolio investment: Evidence from sector analysis in Europe over the last decade. Energy Economics, 38(8), 4528–4539. https://doi.org/https://doi.org/10.1016/j.enpol.2010.04.007
- Baffes, J., & Savescu, C. (2014). Monetary conditions and metal prices. Applied Economics Letters, 21(7), 447–452. https://doi.org/https://doi.org/10.1080/13504851.2013.864029
- Batten, J. A., Ciner, C., Lucey, B. M., Szilagyi, P. G., et al. (2013). The structure of gold and silver spread returns. Quantitative Finance, 13(4), 561–570. https://doi.org/https://doi.org/10.1080/14697688.2012.708777
- Brooks, C., & Prokopczuk, M. (2013). The dynamics of commodity prices. Quantitative Finance, 13(4), 527–542. https://doi.org/https://doi.org/10.1080/14697688.2013.769689
- Ch Murthy Chodi setty, R. S., & Raja Babu, P. (2018). A study of global foreign direct investment (GFDI) impact on indian foreign direct investment (IFDI). Journal of Advanced Research in Dynamical and Control Systems, 10(8), 235–239. ISSN 1943023X. https://www.jardcs.org/backissues/abstract.php?archiveid=3919
- Chai, J., Guo, J.-E., Meng, L., & Wang, S.-Y. (2011). Exploring the core factors and its dynamic effects on oil price: An application on path analysis and BVAR-TVP model. Energy Economics, 39(12), 8022–8036. https://doi.org/https://doi.org/10.1016/j.enpol.2011.09.057
- Chaves, D. B., & Viswanathan, V. (2016). Momentum and mean-reversion in commodity spot and futures markets. Journal of Commodity Markets, 3(1), 39–53. https://doi.org/https://doi.org/10.1016/j.jcomm.2016.08.001
- Chen, P. F., Lee, C. C., Zeng, J. H., et al. (2014). The relationship between spot and futures oil prices: Do structural breaks matter? Energy Economics, 43(2014), 206–217. https://doi.org/https://doi.org/10.1016/j.eneco.2014.03.006
- Chinn, M. D., & Coibion, O. (2014). The predictive content of commodity futures. Journal of Futures Markets, 34(7), 607–636. https://doi.org/https://doi.org/10.1002/fut.21615
- Choi, K., & Hammoudeh, S. (2010). Volatility behavior of oil, industrial commodity and stock markets in a regime-switching environment. Energy Economics, 38(8), 4388–4399. https://doi.org/https://doi.org/10.1016/j.enpol.2010.03.067
- Cuddington, J. (2003). Long-Run Trends in 26 Primary Commodity Prices: A Disaggregated Look at the Prebisch-Singer Hypothesis. Journal of Development Economics, 39, 207–227
- Dumitru, A. M., & Urga, G. (2012). Identifying jumps in financial assets: A comparison between nonparametric jump tests. Journal of Business and Economic Statistics, 30(2), 242–255. https://doi.org/https://doi.org/10.1080/07350015.2012.663250
- Engle, R. F., Ghysels, E., & Sohn, B. (2013). Stock market volatility and macroeconomic fundamentals. Review of Economics and Statistics, 95(3), 776–797. https://doi.org/https://doi.org/10.1162/REST_a_00300
- Engle, R. F., & Rangel, J. G. (2008). The spline-GARCH model for low-frequency volatility and its global macroeconomic causes. Review of Financial Studies, 21(3), 1187–1222. https://doi.org/https://doi.org/10.1093/rfs/hhn004
- International Monetary Fund (IMF). (2010). From Stimulus to Consolidation: Revenue and Expenditure Policies in Advanced and Emerging Economies. Washington.
- Jacks, D. S., & Stuermer, M. (2018). What drives commodity price booms and busts? Energy Economics. https://doi.org/https://doi.org/10.1016/j.eneco.2018.05.023
- Jain, A., & Ghosh, S. (2013). Dynamics of global oil prices, exchange rate and precious metal prices in India. Resources Policy, 38(1), 88–93. https://doi.org/https://doi.org/10.1016/j.resourpol.2012.10.001
- Jegadeesh, N., & Titman, S. (1993). Returns to buying winners and selling losers: Implications for stock market efficiency. The Journal of Finance, 48(1), 65–91. https://doi.org/https://doi.org/10.1111/j.1540-6261.1993.tb04702.x
- Kumar, R. (2004). Price Discovery and Market Efficiency: Evidence from Agricultural Commodities Futures Markets. South Asian Journal of Management, 11(2). New Delhi
- Magrini, E., & Donmez, A. (2013). Agricultural commodity price volatility and its macroeconomic determinants: A GARCH-MIDAS approach. JRC Working Papers JRC84138, Joint Research Centre (Seville site).
- Malik, F., & Hammoudeh, S. (2007). Shock and volatility transmission in the oil, US and Gulf equity markets. International Review of Economics & Finance, Elsevier, 16(3), 357–368. https://doi.org/https://doi.org/10.1016/j.iref.2005.05.005
- Mishra, V., & Smyth, R. (2016). Are natural gas spot and futures prices predictable? Economic Modelling, 54(2016), 178–186. https://doi.org/https://doi.org/10.1016/j.econmod.2015.12.034
- Morana, C. (2013). Oil price dynamics, macro-finance interactions and the role of financial speculation. Journal of Banking & Finance, 37(1), 206–226. https://doi.org/https://doi.org/10.1016/j.jbankfin.2012.08.027
- RBI Report. (2015). Macroeconomic and monetary policy. published by RBI, India.
- Romala Vijaya, S. and Kafila. (2018). Co-integration Analysis Between International Macroeconomic Factors and S&P Sensex Movements. International Journal of Innovative Technology and Exploring Engineering (IJITEE), 8(11). doi:https://doi.org/10.35940/ijitee.K2304.0981119
- Srinivas, P. (2018). Price discovery and asymmetric volatility spillovers in Indian spot-futures gold markets. International Journal of Economic Sciences and Applied Research, 5(3), 65–80
- Valkanov. (2006). Predicting volatility: getting the most out of return data sampled at different frequencies. Journal of Econometrics, 131(1-2). https://doi.org/https://doi.org/10.1016/j.jeconom.2005.01.004