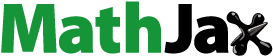
Abstract
The use of information and communications technology (ICT) can improve business competitiveness and provide competitive advantages for organisations—from small to large enterprises. The public sector is even investigating whether cloud-based services can transform its ICT infrastructure to not only reduce costs but also ease maintenance, support and upgradeability. This study aims to analyse the order of important factors influencing cloud adoption and examine the group of causal relationships among the factors influencing cloud adoption in Thailand’s public sector. The data were collected quantitatively through self-administered questionnaires, randomly distributed to 210 sample respondents who had previously used the government cloud service. Then, 16 experts were consulted to determine the degree of direct influence between two factors in the scales of 0 and 4 through a pairwise comparison using purposive sampling. Assurance was identified as the most significant factor, which is also a significant causal factor, and flexibility as the least important one that influence cloud adoption in Thailand’s public sector. Moreover, the key criteria for each factor were identified, and the impact relation maps were obtained. This study contributes to the literature on cloud adoption, provides future research directions and includes suggestions that could help managers and policymakers develop better cloud service platforms for Thailand’s public sector.
PUBLIC INTEREST STATEMENT
The current study presents the ranking order and the cause–effect groups of factors that influence the adoption of the G-Cloud service in Thailand. It analyses the importance order of the factors that influence the adoption and examines the cause-and-effect relationships of these factors. This study was conducted by surveying the opinions of system users and experts. The findings suggest that reliability and responsiveness, assurance, security, perceived usefulness, flexibility, system feature and empathy are the key influencing factors of cloud adoption in the Thai public sector. These findings are significant because the study results could be used as guidelines for assessing and selecting government cloud services suitable for different departments in the organization. Furthermore, it could help reduce budget costs, increase the government service’s competitive ability and operational efficiency, and improve convenience. Moreover, it could help managers and policymakers develop better cloud service platforms for the Thai public sector.
1. Introduction
Currently, changes from digital disruption with regard to different services and transactions have growing importance on people’s lives and businesses. This was evidenced through a survey of internet users in Thailand in 2019, who constituted over 50.1 million or 75% of the population (Electronic Transactions Development Agency, Citation2020). This finding corresponds to the 2020 United Nations E-Government Index report, which showed high numbers of broadband internet users via mobile devices. These growth numbers have increased online transactions for shopping, services, food delivery and payments for products and services, including public sector services. Because of these economic and social changes, different sectors have adjusted their operations to suit the changing behaviours and needs of service recipients. Furthermore, the COVID-19 pandemic is another factor that has led to the “new normal”. Thailand’s administrative development plan, which corresponds to its social and physical distancing policy, has led to the need for digital technology to enhance service efficiency, especially that of the public sector. Based on the changing economic and social needs, the public sector has witnessed the need to change its services, service format and operational process through “digital transformation” in order to match the behaviours and situational needs for online service of people and the business sector. This is operated under the Digital Government Development Agency (Public Organization), which is driven by Thailand’s Digital Government Development Plan 2020–2022 under the digital platform and services project. For example, the government cloud service (henceforth G-Cloud) has adopted the cloud computing service guidelines (Digital Government Development Agency (Public Organisation; DGA), Citation2019).
Cloud computing is a technology of interest because of its technological potential in reducing business costs, especially the costs of investing in information technology infrastructure (Chang et al., Citation2013; Dargha, Citation2009) to create an operational system for either new products or service development. Furthermore, it is used for industrial revolution and personal cloud data storage, which are undergoing rapid changes (Kim et al., Citation2009). Chang et al. (Citation2013) revealed the benefits of a cloud computing system, which can be used to reduce the cost of computer-driven operations and communication systems. It can also reduce the operational time of information technology departments. Additionally, it can reduce the cost of information technology infrastructure investment. These findings are consistent with those of Bharadwaj and Lal (Citation2012), who reported that using cloud computing in an organisation ensures “organisation flexibility”, thus enabling it to promptly respond to environmental and business changes, including market needs and trends. Thus, the organisation can compete, grow and survive. Borgman et al. (Citation2013) asserted that executives invest in cloud computing with the expectation that it would benefit the organisation in several aspects, such as reducing business expenses or costs, which includes money, time and resources. Convenience in responding to the fluctuation or changes in the business environment enables an organisation to make immediate changes based on its needs and increase business opportunities. As the system is highly flexible, business operations are easily changed and adjusted to different formats for responding to the new forms of market needs, and products or services can be launched on the market faster.
G-Cloud provides a form of basic infrastructure, comprising host computers, licensed software, operating systems and databases that each department can manage themselves as if these are devices that they use in their department. When resources are scarce, central processing unit, memory and storage can be promptly added. This can be operated through the government information network or public internet. This network is a large-sized network used by G-Cloud. In terms of security, the system is protected internally and externally with a clear separation between the services of each unit (Digital Government Development Agency (Public Organisation; DGA), Citation2019). However, the use of cloud computing systems still poses some risks as there is no 100% guarantee that this technology is safe, although the Digital Government Development Agency (Public Organization) has a policy to ensure that the public sector workers are confident that there is a very low risk that the department’s data stored in the system would be lost or leaked because of system failure, electricity issues or other risks. These policies increase the government agencies’ confidence and trust in G-Cloud. However, G-Cloud is still dynamic and highly changeable, and it requires continuous development in different aspects, especially its service quality. Therefore, research conducted to develop G-Cloud’s quality for it to be trustworthy, reliable and acceptable from the users’ perspective is important, especially for a future Thailand’s G-Cloud, as it would lead to the sustainable use of the cloud system.
This study presents the rank of factors that influence the acceptance of G-Cloud and the cause–effect relations among the factors and indicators of each factors of acceptance of G-Cloud in Thailand. The findings can be used as guidelines for service quality improvement by the Digital Government Development Agency (Public Organization) as a service provider and government agencies as service users. Furthermore, the results can be used as guidelines for assessing and selecting software to be used in G-Cloud, which would be suitable for each organisation. Applying the findings can help reduce budget costs and increase the competitive ability and operational efficiency of government services and improve convenience.
1.1. Research questions
What are the factors that influence the acceptance of Thailand’s G-Cloud?
How does the group of causal relationships among the factors influence the acceptance of Thailand’s G-Cloud?
1.2. Research objectives
1. To analyse the order of importance of the factors that influence the acceptance of G-Cloud in Thailand, which are reliability and responsiveness, assurance, security and perceived usefulness, flexibility, system features and empathy
2. To analyse the group of causal relationships among factors that affect the acceptance of Thailand’s G-Cloud
2. Literature review
2.1. Service quality
Research on concepts related to service quality have been prevalent for a long time; for example, the service quality (SERVQUAL) model by Parasuraman et al. (Citation1985) assessed consumers’ or service users’ attitudes towards the service providers’ service quality. Service quality comprises the following 10 factors: reliability, responsiveness, competence, access, courtesy, communication, credibility, security, understanding of customers and tangible devices and staff to provide services. These 10 factors were subsequently grouped into the following 5 factors: reliability, responsiveness, empathy, assurance and tangible devices and staff to provide services. However, the quality of traditional service, where users go to the service provider’s office, is different from that of electronic service as it is provided through websites and because other factors are involved, such as security, ease in using the service through websites and channels used to provide the services, which are the service providers’ websites (Rotchanakitumnuai, Citation2017).
In recent years, the service quality concept has been adopted to develop electronic service quality or the quality of services provided through the internet; examples of such concepts include the electronic service quality model (Al-dweeri et al., Citation2019; Baber, Citation2019; Camilleri, Citation2021; Chen, Citation2021; Kang et al., Citation2016; Li et al., Citation2021; Rahayu & Saodin, Citation2021), modified e-SERVQUAL model (Raza et al., Citation2020), electronic hedonic service quality model (e-HSQ; Shatnawi, Citation2019), electronic tail quality model (eTailQ; Ahmad et al., Citation2017; Al-Adwan & Al-Horani, Citation2020; Al-dweeri et al., Citation2019; Jhaveri & Nenavani, Citation2020), site quality (SITEQUAL) model (Jamie et al., Citation2021; Olaleye et al., Citation2018; Pattnaik, Citation2019), website quality (WebQual) model (Al-dweeri et al., Citation2019; Candiwan & Cokro, Citation2021; Longstreet et al., Citation2021; Olaleye et al., Citation2018; Singh et al., Citation2020; Wijaya et al., Citation2021), electronic service quality (e-SQ) model (Khan et al., Citation2019; Othman et al., Citation2020; Rodríguez et al., Citation2021; Trivedi et al., Citation2021), eTransQual model (Al-dweeri et al., Citation2019) and other research on the quality of service provided through the internet (Chen, Citation2021; Cheng et al., Citation2021; Foris et al., Citation2021; Gunasekar et al., Citation2021; Qalati et al., Citation2021). Previous studies have examined the quality of electronic services, such as the quality of websites or data (Qalati et al., Citation2021; Pandjaitan et al., Citation2021; Le et al., Citation2020). Moreover, several studies have investigated the quality of data, design aesthetic, fast service, security, privacy, etc. (Akram et al., Citation2020; Barusman, Citation2019; Grange & Barki, Citation2020; Thekkat & Anandkumar, Citation2021).
Instruments for service quality assessment or the SERVQUAL model by Parasuraman et al. (Citation1985) has been popularly used to assess the quality of service providers. The study showed that, internationally, the SERVQUAL model has been adopted to assess the quality of information technology services in many forms. Scholars have used the SERVQUAL model in different contexts. The traditional SERVQUAL model was used for direct assessment of service by adjusting some research questions to suit the service contexts or by improving the SERVQUAL measure to match the service whose quality assessment of other statistical methods is needed. The measure can be developed in many ways. However, the most prevalent method for new and efficient assessment of service quality developed for the context of this study is factor analysis and other statistical methods, such as reliability.
2.2. Decision-making trial and evaluation laboratory method
The decision-making trial and evaluation laboratory (DEMATEL) method was first developed from 1972 to 1979 by the Battelle Memorial Institute of Geneva under the Science and Human Affairs Programme to study the methods of solving complicated and ambiguous decisions. The DEMATEL method has been generally accepted as the best method to solve complicated and ambiguous decisions and show the cause-and-effect relationships between conditions used to assess a decision (C.-L. Lin & Tzeng, Citation2009; Chiu et al., Citation2006; Lee et al., Citation2009; Tzeng et al., Citation2007; Wu & Lee, Citation2007). Additionally, the DEMATEL method has been adopted to analyse and create cause-and-effect relationship diagrams that indicate the conditions for assessing and ranking the order of importance or showing a relationship diagram of factor groups (C.-L. Lin & Tzeng, Citation2009). The examples of used the DEMATEL method include assessment of airline security (Liou et al., Citation2007), evaluation of E-learning systems (Tzeng et al., Citation2007), development of a world-class business manager’s administrative performance (Wu & Lee, Citation2007), ranking the order of importance of responses for improving operational methods with an analytic hierarchy process for decision-making (Yu & Tzeng, Citation2006), multi-criteria decision-making, analytic network process to solve highly complicated decisions (Yang et al., Citation2008) and the order of importance of factors influencing the success of hospital service quality (Shieh et al., Citation2010). The operational steps of the DEMATEL method are as follows.
Step 1: Assessing experts’ opinions. The mean scores of the matrix Z relationship were calculated. The scores were obtained by surveying experts on effect rates among the factor groups by using the matching principle so that the assessment had accurate influence and directions among the factors through the use of linguistic variables. The influence was divided into five levels, ranging from no influence (Level 0) to very high influence (Level 4). After all the results from the experts were collected, the average scores of matrix Z were calculated using the following formula:
where z is the direct-relation matrix; zij is the average of matrix z when comparing the influence level between the factor groups in row i and column j; m is the number of experts who evaluated the influence level of the factors and is the score of each expert (k) evaluated as the influence level between the factor groups in row i and column j.
Step 2: After calculating the average of matrix Z, the normalised initial direct-relation matrix, which was substituted with matrix D, was calculated. The normalised initial direct-relation matrix D was substituted with D = [dij]. The values of each member in matrix D are between [0, 1]. The normalised initial direct-relation matrix D can be calculated using the following formula:
or
where λ is the regression coefficient matrix value, which can be calculated using the following formulas:
Step 3: After the normalised initial direct-relation matrix was calculated by substituting it with matrix D, the results were used to calculate the total relation of matrix T. I was presented as identity matrix numbers n × n. The number of members of tij represents the indirect effect of the factor in row i that affects the factor in column j. The sum of the matrix T relationship was calculated using Formulas (6) and (7):
by
Step 4: In this step, the sum of row (r) and the sum of column (c) of matrix T from Step 3 were calculated. The sum of the row and column were presented separately using vectors r and c as follows:
If ri is the sum of row ith in matrix T, the value of ri indicates all the effects, both direct and indirect, that factor i has on other factor groups. If cj is the sum of column Jth in matrix T, the value of cj indicates all the effects, both direct and indirect, that other factor groups have on factor j. If j is equal to i and the value of the axis is (ri + cj), factor i is placed in both the cause and effect groups. Conversely, the value of axis (ri—cj) shows the net surplus of factor i compared with that of other factor groups. Moreover, if the value of axis (ri—cj) is positive, factor i is placed in the cause group, and when the value of axis (ri—cj) is negative, factor i is placed in the effect group (Chiu et al., Citation2006; Lee et al., Citation2009; Liou et al., Citation2007; Yang et al., Citation2008).
Step 5: The threshold value (α) was determined by calculating the mean scores of the number of members in matrix T from Step 3 using the following formula:
Step 6: The cause-and-effect relationship diagram was designed. The values of (ri + cj) and (ri—cj) were used to build a model to depict the complicated relationships among the factors and to help determine the rankings of the importance of the factors and their effect on other factor groups (Shieh et al., Citation2010). The causal diagram was divided into two axes. The horizontal axis (ri + cj) shows the rankings of the importance of the factors, whereas the vertical axis (ri—cj) divides the factors into cause and effect groups such that when the value of axis (ri—cj) is positive, the factors are placed in the cause group, and when the value of axis (ri—cj) is negative, the factors are placed in the effect group (Yang et al., Citation2008).
2.3. Service quality dimensions and the characteristics of cloud technology that influence cloud-based adoption
A review of previous literature shows that there are many factors of the SERVQUAL measure, such as tangibles, responsiveness, assurance and empathy, that can be adapted to study the service quality dimensions of G-Cloud. The present study changed the “tangibles” factor to “system features” to suit the study context.
Tangible service attributes refer to the look of the actual surroundings of the service, which includes physical facilities, building designs, employees, tools or equipment, the service’s mode of communication and other customers in the service facility (Parasuraman et al., Citation1994). Dandis et al. (Citation2021) found a link between “tangibles” and self-reported loyalty intentions in their study of loyalty programmes. The “tangibles”, in this case, allude to the system elements of cloud-based technologies.
Hadi et al. (Citation2021) reported that users’ perceptions of cloud-based technology’s compatibility with their present infrastructure, operational capabilities and organisational demands substantially impact their decision to employ cloud computing software as a service. Regarding cloud computing adoption, Hasan et al. (Citation2015) stated that the quality of the system features mostly determines users’ behavioural intentions. As per Raut et al. (Citation2019), cloud computing adoption is influenced by practical qualities, such as hardware scalability and standardisation. In light of these findings, the G-Cloud system’s quality is expected to increase its use in Thailand’s government sector.
Reliability is defined as the ability to consistently and reliably carry out the promised operation (Parasuraman et al., Citation1994). It is the capacity to conduct a reliable and accurate survey of the services provided. Moreover, it is one of the five aspects offered in the SERVQUAL model that examines quality based on cloud technology. Reliability refers to the overall efficiency of the structure and operation of the cloud-based technology system or website as well as dealing with technical difficulties of the cloud-based technology users’ interface. Additionally, it covers the system’s correctness and supplies all of the services that the system has promised (Almaiah & Al-Khasawneh, Citation2020). An improvement in trustworthiness will encourage users to use the system (Naveed et al., Citation2021). According to Almaiah and Al-Khasawneh (Citation2020), trust, which refers to the degree of dependability and trustworthiness of the services provided by mobile cloud service providers, has a favourable influence on the desire to use mobile cloud computing. Furthermore, organisations’ willingness and trust in putting their data in the cloud and under third-party supervision have positively influenced their decision to utilise cloud computing (Alkhater et al., Citation2018). Thus, according to the aforementioned study findings, the reliability of the G-Cloud technology will enhance its use in Thailand’s public sector.
A responsiveness service refers to G-Cloud providers’ readiness to deliver a faster service to Thailand’s public sector consumers (Parasuraman et al., Citation1994). According to Dandis et al. (Citation2021), responsiveness corresponds favourably with self-reported loyalty intentions. Almaiah and Al-Khasawneh (Citation2020) noted that the perceived degree of a specific system’s general performance and its service to end-users has a beneficial effect on the intention to use mobile cloud computing. As per Alkhater et al. (Citation2018), cloud computing provides services to end-users through the internet at any time and from any location. Therefore, a good level of service to customers will boost the likelihood of an organisation adopting cloud technologies. Furthermore, Senarathna et al. (Citation2018) proposed that the quality of service capable of satisfying certain needs—that is, reaction time and throughput of cloud computing providers—positively impacts an organisations’ willingness to use cloud computing. Based on these findings, the G-Cloud responsiveness service will lead to increased use in Thailand’s public sector.
Assurance refers to an employee’s knowledge and benevolence and their ability to inspire trust and confidence (Parasuraman et al., Citation1994). Dandis et al. (Citation2021) found a strong association between workers’ expertise and civility, their capacity to express trust and confidence, and their self-reported loyalty intentions. Additionally, Naveed et al. (Citation2021) revealed that users’ increased trust and confidence boosts their real cloud e-learning system utilisation. Almaiah and Al-Khasawneh (Citation2020) highlighted that maintaining the quality of service in assurance is a critical step for mobile cloud providers for boosting the likelihood of consumers adopting mobile cloud technology. According to Raut et al. (Citation2019), a service-level agreement is a contract that details the cloud provider’s services and sets the performance requirements that the provider has committed to achieving. These are both essential elements that affect cloud computing adoption. Thus, as per the aforementioned study findings, G-Cloud guarantees will raise its usage in Thailand’s public sector.
Empathy refers to the amount of concern and individual attention that cloud-based technology companies deliver to their consumers to make them feel valued and unique (Parasuraman et al., Citation1994). According to Naveed et al. (Citation2021), everyone desires joy, happiness, fun, fulfilment and vitality in their job, and the existence of these elements stimulates the use and adoption of an online web-based system. Additionally, Dandis et al. (Citation2021) identified empathy as the most important component influencing attitudinal loyalty. Almaiah and Al-Khasawneh (Citation2020) and Raut et al. (Citation2019) revealed that quality and on-time delivery were significant variables of cloud computing service providers’ goods or services that influenced corporate adoption. Thus, according to the aforementioned study findings, empathy in G-Cloud will influence the public sector in Thailand to boost its use.
Additionally, the literature review reveals some important factors in assessing G-Cloud’s service quality, such as flexibility and security; however, such factors have not been investigated as part of the outstanding features of the online software service via G-Cloud. These are essential factors that need to be considered because the service is provided through the internet and is paid based on actual usage.
The flexibility of cloud technology is described as the rapid deployment of technological components supported by a business or information technology infrastructure (Garrison et al., Citation2015). Therefore, flexibility is especially pertinent to the adoption of cloud computing in organisations (Zhao et al., Citation2014). Additionally, cloud technology affects how services are provided and the locations where access to such services can be remote or on-demand. Cloud technology therefore allows for more corporate flexibility, which is the major factor driving its adoption (Attaran & Woods, Citation2019; Yoo & Kim, Citation2019). According to Gui et al. (Citation2021), cloud flexibility influences the adoption of cloud computing in small- and medium-sized businesses. As per Raut et al. (Citation2019), the basic competencies of simplicity of use and convenience of cloud technology (access to data from any location by any device or instrument) are important factors affecting cloud computing acceptance. Thus, the aforementioned study findings show that Thailand’s government will increasingly use the flexibility of G-Cloud technology.
Cloud technology security is defined as a worry of secure data transit and storage via electronic databases and media (Wang et al., Citation2020). The difficulties associated with cloud computing security are often viewed as concerns about confidentiality, usefulness and integrity of data to third parties for cloud users. Furthermore, the confidence levels against data hosting—which cannot be viewed as completely responsible—data leakage, vulnerability virtualisation and hypervisor vulnerability are key challenges pertinent to cloud protection (Gui et al., Citation2021). Security is a critical problem in cloud technology adoption because it impacts many aspects of information and communications technology, such as how data is stored, communicated and destroyed; moreover, it includes confidentiality, violation of privacy and its usefulness in organisational operations (Gangwar et al., Citation2015). Hadi et al. (Citation2021) revealed that security has a major impact on the intention of public organisations to use cloud computing software as a service. Furthermore, Alkhater et al. (Citation2018) asserted that security concerns are one of the primary barriers that prevent many organisations from developing an intention to embrace cloud services. Thus, according to the aforementioned study findings, security in G-Cloud technology will expand its adoption in Thailand’s public sector.
2.4. Conceptual framework
This study investigates the factors that influence the acceptance of G-Cloud in Thailand and ranks their order of importance. Moreover, it identifies the cause-and-effect relationships of factor groups and indicates conditions. The conceptual framework is derived based on the literature review ().
3. Research method
This research is a quantitative study. The data collection was divided into two phases. The details of each phase are as follows.
3.1. Phase 1
3.1.1. Sampling and data collection procedures
This phase started with collecting data from the sample group, comprising the staff working in government agencies who participated in the G-Cloud project and had used G-Cloud. The researcher had no definite population. The sample size was determined by estimating the average population at the 95% confidence level (p = 0.05; Vanichbuncha, Citation2005) using the following formula:
where n represents the number or size of the sample, z represents the 95% confidence level and a significance level of 0.05 at 1.96 and σ2 represents the population variance when the standard deviation of the population is unknown. The standard deviation of samples from previous studies was used, and the items were measured on a 5-point Likert scale whose value was equal to 1.53 (Swaid & Wigand, Citation2009). E represents the accepted error rates, which were calculated by multiplying the acceptable margin of error with the lowest mean of the related research. The mean score is 4.80, and the margin of error is 0.05. Therefore, E equals to 0.058 (Swaid & Wigand, Citation2009).
The calculation of the sample size is as follows:
Thus, a sample size of 156 participants was required. Thirty-six questions were used to measure the variables. By applying the rule of thumb, there should be approximately five examples in each question. Therefore, for this study, data had to be collected from at least 180 participants. However, to minimise sampling errors, the sample size of the present study was 210 participants. The data were collected through a paper-based questionnaire employing a purposive sampling method.
3.1.2. Instrument design and measures
The instrument for data collection was a questionnaire comprising two parts:
1) Demographic characteristics of the participants, comprising six questions; and
2) The factors that influence the acceptance of G-Cloud, comprising the following:
(a) Nine questions assessing reliability and responsiveness, which were adapted from Parasuraman et al. (Citation1994), Rehman et al. (Citation2012), Naveed et al. (Citation2018), and Al-Fraihat et al. (Citation2020);
(b) Three questions assessing assurance, which were adapted from Tehrani and Shirazi (Citation2014), Vanessa (Citation2014), Gangwar et al. (Citation2015), and Safari et al. (Citation2015);
(c) Six questions assessing security and perceived usefulness, which were adapted from Davis (Citation1989), Zhu et al. (Citation2006), Luo et al. (Citation2010), Ghobakhloo et al. (Citation2011), Shin (Citation2013), Oliveira and Martins (Citation2011), and Oliveira et al. (Citation2014), and Tehrani and Shirazi (Citation2014), and Gangwar et al. (Citation2015), and Safari et al. (Citation2015);
(d) Four questions assessing flexibility, which adapted from Oliveira et al. (Citation2014) and Tehrani and Shirazi (Citation2014);
(e) Three questions assessing system feature, which were adapted from Featherman and Pavlou (Citation2003), Lin (Citation2010), and Gangwar et al. (Citation2015) and Mohammed et al. (Citation2017) and
(f) Three questions assessing empathy, which were adapted from Parasuraman et al. (Citation1994), Kauffman et al. (Citation2014), Mero and Mwangoka (Citation2014), and Hapsari et al. (Citation2017).
The format for all the questions was a 5-point Likert scale. The instruments were tested by examining the quality of the questionnaire. Three experts assessed the content validity using the index of congruence with acceptance rates >0.50 to ensure that the questions were consistent with the study objectives and variables to be measured (Rovinelli & Hambleton, Citation1977). The results show that every question passed the minimum requirement (0.67–1.00). A pilot test was conducted with another sample of 30 participants to analyse Cronbach’s alpha; for variables to be accepted as reliable, the rate should be >0.7 (Hair et al., Citation2010). The results show that the reliability levels of all six variables passed the threshold (0.738–0.902).
3.1.3. Data analysis
The researcher processed the questionnaire results using the SPSS software to analyse the demographic characteristics of the participants. Factor analysis was used to analyse the responses of 210 participants in order to reduce the number of variables and group the new factors for consistency. The reliability of each variable was tested by analysing Cronbach’s alpha.
3.2. Phase 2
In this phase, the factors were grouped using mind mapping to develop a questionnaire in order to survey the importance of the factors and indicating conditions based on the opinions of 16 experts, who were selected using the purposive sampling method. The experts were selected based on their knowledge and experience in providing G-Cloud and developing its system. The experts consulted were suitable for the study as they had appropriate qualifications. They are responsible for G-Cloud; hold at least a bachelor’s degree in computer science or other related fields and have more than 3 years of experience with G-Cloud as project managers, business analysts, software analysts or designers, database administrators, programmers/software developers and testers. The data were analysed using the DEMATEL method to outline the idea and variables affecting G-Cloud adoption and determine the final influencing factors through a literature review and factor analysis. However, determining the relevance of each component and the causal relationship between numerous elements remains challenging. There is currently a dearth of quantitative evidence to support an understanding of the essential components. Following that, this study uses the DEMATEL method to conduct a systematic quantitative analysis of the factors influencing G-Cloud adoption.
3.3. Protection of participants’ rights
This study was approved by the Institutional Review Board Research Ethics Committee of Silpakorn University (approval number: COE 63.0729–061.) The researcher explained the protection of participants’ rights on the first page of the research questionnaire. The data were collected only from participants who signed the consent form to participate in the study and the overall data analysis for academic purposes.
4. Results
4.1. Demographic characteristics
The descriptive statistics of the demographic characteristics of the participants, comprising gender, age, educational level, job position, relationship with G-Cloud and job duration with G-Cloud are presented in .
Table 1. Demographic characteristics of the sample group
The descriptive statistics of experts who answered the questionnaires, which comprise their job position and experience in working with G-Cloud, are presented in .
Table 2. Demographic characteristics of experts
4.2. Exploratory factor and reliability analyses
The results of the factor analysis show that the Kaiser–Meyer–Olkin (KMO) Measure of Sampling Adequacy value is 0.724, which is close to 1 (>0.5). This shows that all the data analysed using factor analysis were at an appropriate level because the KMO value is quite high. Bartlett’s test of sphericity, which shows the correlation matrix of the variables and their appropriateness for factor analysis, indicates a significant correlation among the variables (chi-square = 5807.454; df = 406; p-value < 0.05). Subsequently, the variables were grouped using the factor analysis technique. The factors were reduced using principal component factor analysis with varimax rotation. In this study, the number of factors was selected using an eigenvalue that is >1 and a factor loading value that is >0.5. Based on the results, the factors could be categorised into the following six groups as factors that are related to: (1) reliability and responsiveness, (2) assurance, (3) security and perceived usefulness, (4) flexibility in using the system, (5) system features and (6) empathy. Additionally, based on the threshold of >0.7, the Cronbach’s alpha analysis results show that the questionnaire is reliable (Nunnally & Bernstein, Citation1994), and every factor passed the threshold (0.826–0.930), as shown in .
Table 3. Factor loadings by exploratory factor analysis (pattern matrix of the factors and items)
4.3. Results of the order of importance and analysis of the cause-and-effect relationship of factor groups
After obtaining the results of the factor analysis of the factor groups affecting the acceptance of G-Cloud, the researcher divided the factors into groups using mind mapping. Later, the questionnaire was designed to collect data from the experts by conducting a survey about the importance of factors influencing the acceptance of G-Cloud and indicating conditions of each factor. The data were then analysed to identify the importance and relationship between the factors influencing the acceptance of G-Cloud and indicating conditions of each factor. The survey results were analysed using the DEMATEL method.
Step 1. Opinions of the experts were collected. The average scores of relations of matrix Z were calculated, as shown in .
Table 4. Comparative information of groups of factors influencing the acceptance of G-Cloud using the linguistic principle
After the linguistic scores were obtained, they were converted into linguistic values by calculating the relation of the linguistic values with matrix Z. The results of matrix Z calculated with Formula (1) are shown in Formula (11).
Step 2. After obtaining the relationship value of matrix Z, the normalised initial direct-relation matrix D was calculated using Formulas (2)–(5), and the average scores of the normalised initial direct-relation matrix D were calculated as presented in Formula (12).
Step 3. From the normalised initial direct-relation matrix D value, the total relation matrix T can be calculated using Formulas (6) and (7). The total relation matrix T values are presented in Formula (13).
Step 4. The r value or the sum of rows and the c value—or the sum of columns—of matrix T were calculated. The r and c values were used to calculate the (ri + cj) and (ri—cj) values presented in .
Table 5. Values used to analyse the importance of factors and grouping of the relationships among factors into cause-and-effect groups
Step 5. The threshold value was calculated using Formula (10), which is as follows..
Threshold value = 62.9709/36 = 1.7492
Step 6. The cause-and-effect relationship diagram was created. The (ri + cj) values were used to show the order of importance of the factors, which is based on the calculated values. The (ri—cj) values were used to divide the factors into cause and effect groups. When the values of axis (ri—cj) are positive, the factors are placed in the cause group, and when the values of axis (ri—cj) are negative, the factors are placed in the effect group ().
From the analysis conducted using the DEMATEL method, the order of importance of factors influencing the acceptance of G-Cloud based on (ri + cj; and ) is as follows. The first rank belongs to the group of factors related to assurance. This is the most important factor affecting the acceptance of G-Cloud in Thailand, with an importance value of 21.8997. The second rank belongs to the group of factors related to security and perceived usefulness, with an importance value of 21.6333. The third rank belongs to the group of factors related to reliability and responsiveness, with an importance value of 21.3502. The fourth rank belongs to the group of factors related to system features, with an importance value of 20.5932. The fifth rank belongs to the group of factors related to empathy, with an importance value of 20.4044. The sixth rank belongs to the group of factors related to flexibility in using the system, with an importance value of 20.0609. To illustrate, the group of factors related to assurance is important for the acceptance of G-Cloud in Thailand. However, the group of factors related to security and perceived usefulness as well as reliability and responsiveness cannot be overlooked.
Table 6. Comparative information pertaining to groups of factors influencing the acceptance of G-Cloud using the linguistic principle
Based on axis (ri—cj), the values of F1, F5 and F6 are −0.2175, −0.3809 and −0.6429, respectively, which are negative. To illustrate, the group of factors related to reliability and responsiveness (F1), system features (F5) and empathy (F6) is categorised as the effect group. The positive values or cause group comprise the group of factors related to assurance (F2), security and perceived usefulness (F3) and flexibility in using the system (F4), with values of 0.7856, 0.1435 and 0.3123, respectively. The results of the groupings of factors show that the group of factors related to assurance has the most important influence on the acceptance of G-Cloud in Thailand. Further, it is highly correlated with the group of factors related to flexibility in using the system to effectively build the system users’ confidence ().
Moreover, the indicating conditions of each factor were analysed to determine the most important indicating condition in each group of factors influencing the acceptance of G-Cloud in Thailand. The analysis of the cause-and-effect relationship of the indicating conditions of each factor reveals the cause-and-effect relationship of the indicating conditions of each group of factors, as shown in .
Table 7. Cause-and-effect relationship of indicating conditions of each group of factors
In the group of factors that are related to reliability and responsiveness, “the system administrators understand and specifically acknowledge your problems” (C6) is the most important indicating condition ().
Figure 3. The cause and effect of the group of indicating conditions in the group of factors related to reliability and responsiveness.
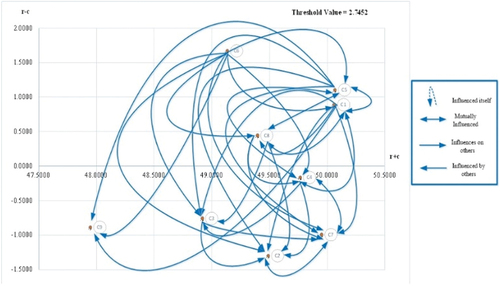
For the group of factors related to assurance, “the service administrators clearly explain how to use the system” (C10) is the most important indicating condition in the cause group ().
Figure 4. The cause and effect of the group of indicating conditions in the group of factors related to assurance.
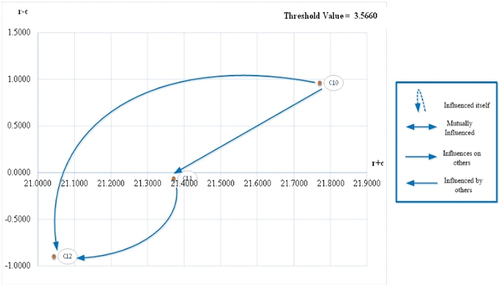
Regarding the group of factors related to security and perceived usefulness, “the privacy policy of the system is clear” (C16) is the most important indicating condition in the cause group ().
Figure 5. Diagram of the cause and effect of the group of indicating conditions in the group of factors related to security and perceived usefulness.
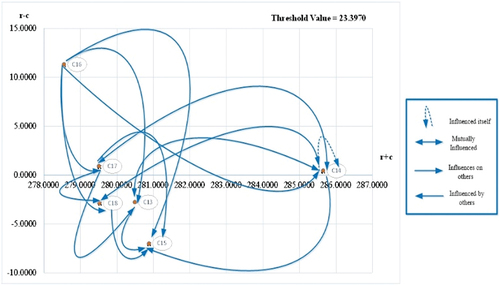
For the group of factors related to flexibility in using the system, “using the system helps you not to carry data storage devices” (C19) is the most important indicating condition in the cause group ().
Figure 6. The cause and effect of the group of indicating conditions in the group of factors related to flexibility in using the system.
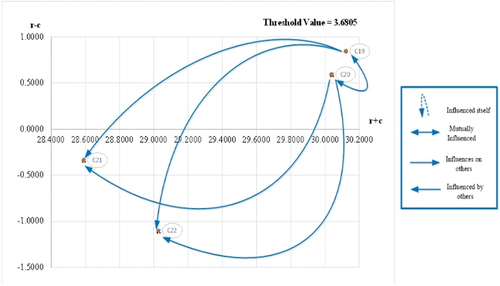
Regarding the group of factors related to system features, “the system works well and fast as expected” (C25) is the most important indicating condition in the cause group ().
Figure 7. The cause and effect of the group of indicating conditions in the group of factors related to the system features.
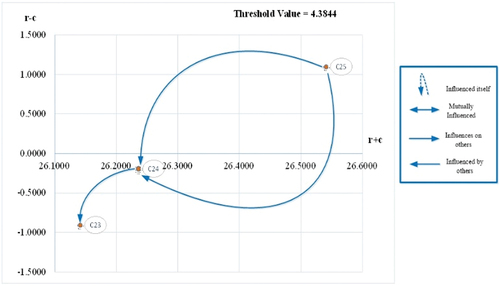
Regarding the group of factors related to empathy, “there is a system administrator who is ready to listen to your operational problems privately” (C26) is the most important indicating condition in the cause group ().
5. Discussion
This study analyses the order of importance and the cause-and-effect relationship of the factors influencing the acceptance of G-Cloud in Thailand through a survey of the opinions of the system users and experts. The results were analysed using factor analysis and the DEMATEL method. The results indicate that the order of the importance of factors influencing the acceptance of G-Cloud in Thailand is as follows. The first rank belongs to factors related to assurance. The second rank belongs to factors related to security and perceived usefulness. The third rank belongs to factors related to reliability and responsiveness. The fourth rank belongs to factors related to system features. The fifth rank belongs to factors related to empathy, and the sixth rank belongs to factors related to flexibility in using the system. The factors influencing the acceptance of G-Cloud in Thailand can also be divided into the following groups based on cause and effect: (1) the cause factor group comprising assurance, security and perceived usefulness and the flexibility in using the system and (2) the effect factor group comprising reliability and responsiveness, system features and empathy.
The results of the order of importance of factors influencing the acceptance of G-Cloud in Thailand indicate that the group of factors related to assurance is the most important cause factor influencing the acceptance of G-Cloud in Thailand. “The system administrators can clearly explain how to use the system” is the most important indicating condition in this group of factors. This implies that users of G-Cloud, who work for different government agencies in Thailand, accepted and used G-Cloud mainly because of confidence in using the system. This is because of the ability of the administrators to clearly explain the process of using the cloud system and building users’ confidence in and reliability on the system. Additionally, when contacted, the administrators demonstrate sincerity, honesty and willingness to serve. This is consistent with the findings of Priyadarshinee et al. (Citation2017), Alkhater et al. (Citation2018), C. Lin and Lin (Citation2019), and Khayer et al. (Citation2020), and Ali and Osmanaj (Citation2020) that enhancing the system users’ confidence is an important positive factor that affects the acceptance of cloud systems. Furthermore, the results are correlated with the findings of Liang et al. (Citation2021), who indicated that the reliability of cloud service positively affects the staff of government agencies in accepting to use the cloud system.
Regarding the group of factors related to security and perceived usefulness, “the privacy policy of the system is clear” is the most important indicating condition in this group. This implies that G-Cloud users, who are staff of different government agencies in Thailand, will accept and use G-Cloud if they perceive security and privacy in using the system. This is the result of a clear privacy policy, the measures that the system administrators employ to store and restore data after emergencies or disasters, the users’ perception of the usefulness of G-Cloud in their work, the development of the system’s standards for secured data by specifying the users’ rights to access data within the scope of the responsibilities of each level of position and the development of reliable data protection and security. This finding is consistent with that of Ali et al. (Citation2018), Yuvaraj (Citation2016), Alsmadi and Prybutok (Citation2018), and Mokwena and Hlebela (Citation2018), and Ali and Osmanaj (Citation2020), who found that the factors related to security positively affect the acceptance of cloud service users, especially those who work in government agencies (Amron et al., Citation2021; Bhardwaj et al., Citation2021; Hadi et al., Citation2021; Wahsh & Singh, Citation2015). Moreover, Wahsh and Singh (Citation2015), Palos-Sanchez et al. (Citation2017), Kumar et al. (Citation2018), Mokwena and Hlebela (Citation2018), and Hadi et al. (Citation2021), and Bhardwaj et al. (Citation2021) indicated that perceived usefulness is an important factor affecting the acceptance to use a cloud service.
The last is the group of factors related to flexibility in using the system. “Using the system can help you not to carry data storage devices” is the most important indicating condition in this group. This implies that the acceptance and use of G-Cloud by the users, who are staff from different government agencies in Thailand, is based on the accessibility of the service from anywhere and at any time as they do not desire to carry data storage devices because the data are already stored on the cloud system. Additionally, because the users can access the service via the internet network, they do not have to waste their time on programme installation. These factors can lead to faster operation and flexibility in using the service to meet users’ needs. The results are consistent with those of Mohammed et al. (Citation2016), Mohammed et al. (Citation2017) and Qasem et al. (Citation2018), who found that flexibility in using a cloud service that can respond to users’ needs has a positive impact on the users’ acceptance and use of the cloud service. Arpaci (Citation2017), Alsmadi and Prybutok (Citation2018), C. Lin and Lin (Citation2019), and Amron et al. (Citation2021) revealed that the satisfying experience of users, such as convenience in accessing the service via the internet from anywhere and at any time without having to carry any data storage devices, is an important factor that increases the rates that users accept for using the cloud service.
6. Suggestions
6.1. Suggestions for applying the research implications
The field of technology acceptance model has a long history of research. Nevertheless, most past studies have concentrated on determining adoption factors across various technologies and use conditions or the effect of the relationship between technological features and adoption results. This study contributes to the body of knowledge by merging service quality aspects and cloud technology features in the context of cloud adoption in Thailand’s public sector. This is the first complete study to employ a sophisticated, mixed approach to discover, prioritise and examine the cause–effect relationships between the variables. The authors conducted an extensive literature study. The factor analysis approach enabled the number of variables to be minimised and allowed the new components for consistency to be grouped—the DEMATEL method aided in prioritising and evaluating the cause–effect relationship between these factors. The findings of this research may provide a framework for evaluating and selecting the software for usage in a cloud-based service that is suitable for each organisation.
6.2. Suggestions for applying the research results
The present study provides useful guidelines to those involved in G-Cloud, that is, the Digital Government Development Agency (Public Organization) and policymakers, whose main responsibility is to develop and provide G-Cloud, service providers and programme developers. The following subsections show how the results can be applied to ensure service quality improvement.
1) The Digital Government Development Agency (Public Organization) and policymakers can apply the study results, which found that the factors related to the system users’ confidence enhancement are the most important cause factors that influence the acceptance of G-Cloud. “System administrators who can clearly explain how to use the system” is the most important indicating condition in the factor group. Thus, this is can be set as a goal for service quality improvement, which can be divided based on the roles of those who are responsible as follows.
1.1) Project administration managers can apply the study results to plan strategies and missions for project and resource management and efficiently and effectively control the quality of works to be submitted in different projects by improving the administrators’ skills in providing such services. This is essential because serviceability is an important supporting factor that enhances an organisation’s success in business contact with system users. In particular, staff who work in the service sector should receive continuous service skill training to enhance the operational potential and promote the service standards and positive image among the system users. Developing ability of the staff who work in the service sector as administrators will lead to obvious outcomes and become the foundation for an organisation’s competitive ability. Moreover, this will improve the ability and skills of the staff in providing excellent service to the system users. The focus should be on communicative skills required to build good relationships with customers through communication styles and body language that are appropriate to different types of users. Moreover, the development of skills—such as speaking, listening, writing and communicating through telephones—and techniques for rejecting customers without hurting their feelings should be promoted. This would enable the staff to know, understand and clearly explain how to use the system to the users rather than investing in different resources inside the organisation to improve the system with complicated features. Moreover, this will assurance and help the users to have confidence in using the system and service.
1.2) Staff who are responsible for technology transfer can apply the study results to understand the development of operational processes and training related to listening, speaking, reading and writing skills so that they can provide advice and suggestions related to using the system. This will help the staff of government agencies to effectively and efficiently carry out their work.
2) The Digital Government Development Agency (Public Organization) and policymakers can apply the research results that show that for factors related to security and perception of usefulness, “the privacy policy of the system is clear” is the second most important indicating condition in the factor group as a goal for service quality improvement. This guideline can be divided according to the roles of those who are responsible as follows.
2.1) Security standard development managers can apply the study results to develop a clear users’ privacy policy that protects the personal information of users of G-Cloud. This covers issues related to the collection of personal information, use of personal information, rights to control the system users’ personal information, security of personal information, cookies policy and adjustments of the privacy policy via written announcements supported by laws. The users would therefore feel comfortable and not worry that their information would be accessed without permission, sold or exposed to outsiders.
2.2) System developers can apply the results of this research as guidelines to develop an operational system to secure the system users’ personal information by building algorithms used for data coding to cover all important issues, such as confidentiality of the information, in order to prevent those who do not have the right to access the information from accessing it, enhance the integrity of the information for ensuring that the data are in their complete state and develop authentication or nonrepudiation of the information senders to check who they are and for protection against false claims. These points would help build the users’ confidence in the security system and privacy of G-Cloud.
2.3) Database administrators can apply the study results as guidelines to assign rights to database access by placing importance on the system users’ privacy policy. For instance, the right to access the database may be reserved only for those involved, and the database should be coded and regularly audited, which are important foundations for security. No matter how well the database is checked and followed up on, without coding and masking, ill-wishers or hackers can bypass the database and easily steal the data.
3) The Digital Government Development Agency (Public Organization) and policymakers can apply the research results that show that for factors related to flexibility in using the system, “using the system helps you not to carry information storage devices” is the most important indicating condition of causes in this factor group as a goal for improving the service quality. This guideline can be divided based on the roles of those who are responsible as follows.
3.1) Research and development managers can apply the research results as guidelines for administering and setting frameworks and research plans to ensure flexibility at work. They can do this by developing the system to be on-demand. In other words, when the users use the programme anywhere or anytime, they can return to the programme at any time. Moreover, they can increase the ability to add or reduce data storage space, with no effect on the system, customise the system characteristics to match business needs and improve the system for complete connection with other systems. For example, if the organisation’s internal system is complicated or uses other online software systems, every system should be connected.
3.2) System development staff can apply the study results as guidelines to develop the system to be user-friendly and adjust its characteristics to match the users’ needs, such as adding more data storage space for users to support the increasing need for future data storage. These changes will enhance the users’ desire to use the system because they do not have to carry any data storage device. They can immediately retrieve information through the network.
6.3. Recommendations for future research
The present study has recommendations for future research. Because the framework is only based on the literature review, future studies should use interviews or the Delphi technique to gain specific information on factors that affect the acceptance of using G-Cloud by relevant decision-makers, administrators and experts. This is essential as there might be many other factors influencing the acceptance of G-Cloud in Thailand.
Institutional Review Board Statement
The study was conducted according to the guidelines of the Declaration of Helsinki and approved by the Human Research Ethics Committee of Silpakorn University (COE Number: COE 63.0729-061; Date of approval: 29 July 2020).
Informed Consent Statement
Informed consent was obtained from all subjects involved in the study.
Disclosure statement
No potential conflict of interest was reported by the author(s).
Data Availability Statement
The datasets generated during and/or analysed during the current study are not publicly available because of IRB stipulations but are available from the author on reasonable request.
Additional information
Funding
Notes on contributors
Thadathibesra Phuthong
Thadathibesra Phuthong is a lecturer in the Exhibition and Event Management Department, Faculty of Management Science, Silpakorn University, Thailand. He has taught in many fields, including Information Systems for Logistics and Supply Chain, Information and Communication Technology for the Tourism Industry, Microsoft Excel for Accountants, Statistics for Business Decisions, Innovation in the MICE Business and Innovation and Design. His current research interests include Technology and Innovation Adoption, Technology Innovation Management and Emerging Technologies.
References
- Ahmad, A., Rahman, O., & Khan, M. N. (2017). Exploring the role of website quality and hedonism in the formation of e-satisfaction and e-loyalty: Evidence from internet users in India. Journal of Research in Interactive Marketing, 11(3), 246–28. https://doi.org/10.1108/JRIM-04-2017-0022
- Akram, U., Ansari, A. R., Fu, G., & Junaid, M. (2020). Feeling hungry? let’s order through mobile! examining the fast food mobile commerce in China. Journal of Retailing and Consumer Services, 56(C), 102142. https://doi.org/10.1016/j.jretconser.2020.102142
- Al-Adwan, A. S., & Al-Horani, M. A. (2020). Boosting customer e-loyalty: An extended scale of online service quality. Information, 10(12), 380. https://doi.org/10.3390/info10120380
- Al-dweeri, R. M., Ruiz Moreno, A., Montes, F. J. L., Obeidat, Z. M., & Al-dwairi, K. M. (2019). The effect of e-service quality on Jordanian student’s e-loyalty: An empirical study in online retailing. Industrial Management & Data Systems, 119(4), 902–923. https://doi.org/10.1108/IMDS-12-2017-0598
- Al-Fraihat, D., Joy, M., Sinclair, J., & Sinclair, J. (2020). Evaluating E-learning systems success: An empirical study. Computers in Human Behavior, 102(2020), 67–86. https://doi.org/10.1016/j.chb.2019.08.004
- Ali, M. B., Wood-Harper, T., & Mohamad, M. (2018). Benefits and challenges of cloud computing adoption and usage in higher education. International Journal of Enterprise Information Systems, 14(4), 64–77. https://doi.org/10.4018/IJEIS.2018100105
- Ali, O., & Osmanaj, V. (2020). The role of government regulations in the adoption of cloud computing: A case study of local government. Computer Law & Security Review, 36(2020), 105396. https://doi.org/10.1016/j.clsr.2020.105396
- Alkhater, N., Walters, R., & Gary, W. (2018). An empirical study of factors influencing cloud adoption among private sector organisations. Telematics and Informatics, 35(1), 38–54. https://doi.org/10.1016/j.tele.2017.09.017
- Almaiah, M. A., & Al-Khasawneh, A. (2020). Investigating the main determinants of mobile cloud computing adoption in university campus. Education and Information Technologies, 25(4), 3087–3107. https://doi.org/10.1007/s10639-020-10120-8
- Alsmadi, D., & Prybutok, V. (2018). Sharing and storage behavior via cloud computing: Security and privacy in research and practice. Computers in Human Behavior, 85(2018), 218–226. https://doi.org/10.1016/j.chb.2018.04.003
- Amron, M. T., Ibrahim, R., & Bakar, N. A. A. (2021). Cloud computing acceptance among public sector employees. TELKOMNIKA (Telecommunication Computing Electronics and Control), 19(1), 124–133.http://dx.doi.org/10.12928/telkomnika.v19i1.17883124
- Arpaci, I. (2017). Antecedents and consequences of cloud computing adoption in education to achieve knowledge management. Computers in Human Behavior, 70(2017), 382–390. https://doi.org/10.1016/j.chb.2017.01.024
- Attaran, M., & Woods, J. (2019). Cloud computing technology: Improving small business performance using the Internet. Journal of Small Business Entrepreneurship, 31(6), 495–519. https://doi.org/10.1080/08276331.2018.1466850
- Baber, H. (2019). E-SERVQUAL and its impact on the performance of Islamic Banks in Malaysia from the customer’s perspective. Journal of Asian Finance, Economics and Business, 6(1), 169–175. https://doi.org/10.13106/JAFEB.2019.VOL6.NO1.169
- Barusman, A. R. P. (2019). The effect of security, service quality, operations and information management, reliability &trustworthiness on e-loyalty moderated by customer satisfaction on the online shopping website. International Journal of Supply Chain Management, 8(6), 586–594. https://ojs.excelingtech.co.uk/index.php/IJSCM/article/view/4117/2073
- Bharadwaj, S. S., & Lal, P. (2012). Exploring the impact of cloud computing adoption on organizational flexibility: A client perspective. Paper presented at the 2012 International Conference on Cloud Computing Technologies, Applications and Management (ICCCTAM), 8-10 Dec. 2012. IEEE, Dubai, United Arab Emirates.
- Bhardwaj, A. K. G., Garg, A., L., Gajpal, Y., & Gajpal, Y. (2021). E-Learning during COVID-19 outbreak: Cloud computing adoption in indian public universities. Computers, Materials & Continua, 66(3), 2471–2492. https://doi.org/10.32604/cmc.2021.014099
- Borgman, H. P., Bahli, B., Heier, H., & Schewski, F. (2013). Cloudrise: Exploring cloud computing adoption and governance with the TOE framework. Paper presented at the 2013 46th Hawaii International Conference on System Sciences, 7-10 Jan. 2013. IEEE. Wailea, HI, USA.
- Camilleri, M. A. (2021). E-commerce websites, consumer order fulfillment and after-sales service satisfaction: The customer is always right, even after the shopping cart check-out. Journal of Strategy and Management, ahead-of-print(ahead–of–print), 1–20. https://doi.org/10.1108/JSMA-02-2021-0045
- Candiwan, C., & Cokro, W. (2021). Analysis of the influence of website quality to customer’s loyalty on e-commerce. International Journal of Electronic Commerce Studies, 12(1), 83–102. http://dx.doi.org/10.7903/ijecs.1892
- Chang, B.-Y. H., Pham, S., Dong-Won, L., Jong-Hun, Y., & Seunghyun. (2013). The determinant of adoption in cloud computing in Vietnam. Paper presented at the 2013 International Conference on Computing, Management and Telecommunications (ComManTel), Ho Chi Minh City, Vietnam.
- Chen, C.-H. (2021). Leveraging the power of online referral for E-business: The moderated mediation model. Journal of Theoretical and Applied Electronic Commerce Research, 16(7), 2594–2607. https://doi.org/10.3390/jtaer16070143
- Cheng, -C.-C., Chang, -Y.-Y., & Chen, C.-T. (2021). Construction of a service quality scale for the online food delivery industry. International Journal of Hospitality Management, 95(2021), 102938. https://doi.org/10.1016/j.ijhm.2021.102938
- Chiu, Y.-J., Chen, H.-C., Tzeng, G.-H., & Shyu, J. Z. (2006). Marketing strategy based on customer behavior for the LCD-TV. International Journal of Management and Decision Making, 7(2/3), 143. https://doi.org/10.1504/IJMDM.2006.009140
- Dandis, A. O., Wright, L. T., Wallace-Williams, D. M., Mukattash, I., Al Haj Eid, M., Cai, H. (Helen), & Corona, C. G. (2021). Enhancing consumers’ self-reported loyalty intentions in Islamic Banks: The relationship between service quality and the mediating role of customer satisfaction. Cogent Business & Management, 8(1), 1892256. https://doi.org/10.1080/23311975.2021.1892256
- Dargha, R. (2009). Cloud Computing Key Considerations for Adoption. Infosys – White Paper. Retrieved at 6 February 2020. https://docplayer.net/4694363-Cloud-computing-key-considerations-for-adoption-abstract-ramkumar-dargha.html/
- Davis, F. D. (1989). Perceived usefulness, perceived ease of use, and user acceptance of information technology. MIS Quarterly, 13(3), 319–340. https://doi.org/10.2307/249008
- Digital Government Development Agency (Public Organisation) (DGA). (2019). Government Cloud. Retrieved at 6 February 2020. https://www.dga.or.th/our-services/foundation/infrastructure/g-cloud/
- Electronic Transactions Development Agency. (2020). THAILAND INTERNET USER BEHAVIOR 2020. Retrieved at 6 February 2020. https://www.thaipdf.com/2092-thailand-internet-user-behavior-2020/
- Featherman, M. S., & Pavlou, P. A. (2003). Predicting e-services adoption: A perceived risk facets perspective. International Journal of Human-Computer Studies, 59(4), 451–474. https://doi.org/10.1016/S1071-5819(03)00111-3
- Foris, D., Popescu, M., & Hârțescu, M. (2021). Quality assessment of hotel services on the basis of online guests’ reviews: A case study in Romania. Quality - Access to Success, 22(183), 118–123. https://www.srac.ro/calitatea/en/arhiva/2021/QAS_Vol.22_No.183_Aug.2021.pdf
- Gangwar, H., Date, H., & Ramaswamy, R. (2015). Understanding determinants of cloud computing adoption using an integrated TAM-TOE model. Journal of Enterprise Information Management, 28(1), 107–130. https://doi.org/10.1108/JEIM-08-2013-0065
- Garrison, G., Wakefield, R. L., & Kim, S. (2015). The effects of IT capabilities and delivery model on cloud computing success and firm performance for cloud supported processes and operations. International Journal of Information Management, 35(4), 377–393. https://doi.org/10.1016/j.ijinfomgt.2015.03.001
- Ghobakhloo, M., Arias-Aranda, D., & Benitez-Amado, J. (2011). Adoption of e-commerce applications in SMEs. Industrial Management & Data Systems, 111(8), 1238–1269. https://doi.org/10.1108/02635571111170785
- Grange, C., & Barki, H. (2020). The nature and role of user beliefs regarding a website’s design quality. Journal of Organizational and End User Computing, 32(1), 75–96. https://doi.org/10.4018/JOEUC.2020010105
- Gui, A., Fernando, Y., Shabir, M., Shaharudin, M., Mokhtar, I. G. M. K., & Suryanto. (2021). Drivers of cloud computing adoption in small medium enterprises of Indonesia creative industry. JOIV: International Journal on Informatics Visualization, 5(1), 69–75. http://dx.doi.org/10.30630/joiv.5.1.461
- Gunasekar, S., Kumar, D. S., Purani, K., Dixit, S. K., Menon, D., & Menon, D. (2021). Understanding service quality attributes that drive user ratings: A text mining approach. Journal of Vacation Marketing, 27(4), 400–419. https://doi.org/10.1177/13567667211003246
- Hadi, H. J., Omar, M. A., & Osman, W. R. S. (2021). Investigating the determinants of CC-SaaS adoption in Iraqi’s public organisations from the perspective of IT professionals. International Journal of Engineering Research and Technology, 14(2), 130–143. https://www.ripublication.com/irph/ijert21/ijertv14n2_06.pdf
- Hair, J. F., Black, W., Babin, C., Barry, J., & Anderson, R. E. (2010). Multivariate Data Analysis. Prentice Hall.
- Hapsari, R., Clemes, M. D., & Dean, D. (2017). The impact of service quality, customer engagement and selected marketing constructs on airline passenger loyalty. International Journal of Quality and Service Sciences, 9(1), 21–40. https://doi.org/10.1108/IJQSS-07-2016-0048
- Hasan, L. M., Zgair, L. A., Ngotoye, A. A., Hussain, H. N., & Najmuldeen, C. (2015). A review of the factors that influence the adoption of cloud computing by small and medium enterprises. Scholars Journal of Economics, Business and Management, 2(8A), 842–848. http://saspjournals.com/wp-content/uploads/2015/08/SJEBM-28A842-848.pdf
- Jamie, C., Rahman, S. M., Rahman, M. M., Wyllie, J., & Voola, R. (2021). Engaging Gen Y customers in online brand communities: A cross-national assessment. International Journal of Information Management, 56(2021), 102252. https://doi.org/10.1016/j.ijinfomgt.2020.102252
- Jhaveri, C. A., & Nenavani, J. M. (2020). Evaluation of eTail services quality: AHP approach. Vision, 24(3), 310–319. https://doi.org/10.1177/0972262919898093
- Kang, D., Jang, W., & Park, Y. (2016). Evaluation of e-commerce websites using fuzzy hierarchical TOPSIS based on E-S-QUAL. Applied Soft Computing Journal, 42(C), 53–65. https://doi.org/10.1016/j.asoc.2016.01.017
- Kauffman, R. J., Ma, D., & Yu, M. (2014). A metrics suite for firm-level cloud computing adoption readiness. In J. Altmann, K. Vanmechelen, and O. Rana (Eds.), Economics of grids, clouds, systems, and services. GECON 2014. Lecture notes in computer science, (vol 8914, pp. 19–35). Springer. https://doi.org/10.1007/978-3-319-14609-6_2
- Khan, M. A., Zubair, S. S., & Malik, M. (2019). An assessment of e-service quality, e-satisfaction and e-loyalty: Case of online shopping in Pakistan. South Asian Journal of Business Studies, 8(3), 283–302. https://doi.org/10.1108/SAJBS-01-2019-0016
- Khayer, A., Talukder, M. S., Bao, Y., & Hossain, M. N. (2020). Cloud computing adoption and its impact on SMEs’ performance for cloud supported operations: A dual-stage analytical approach. Technology in Society, 60(2020), 101225. https://doi.org/10.1016/j.techsoc.2019.101225
- Kim, W., Kim, S. D., Lee, E., & Lee, S. (2009). Adoption issues for cloud computing. Paper presented at the Proceedings of the 7th International Conference on Advances in Mobile Computing and Multimedia, Kuala Lumpur, Malaysia, Association for Computing Machinery, 2–5. https://doi.org/10.1145/1821748.1821751
- Kumar, R., Sachan, A., Mukherjee, A., & Kumar, R. (2018). Factors influencing e-government adoption in India: A qualitative approach. Digital Policy, Regulation and Governance, 20(5), 413–433. https://doi.org/10.1108/DPRG-02-2018-0007
- Le, V. H., Nguyen, H. T. T., Nguyen, N., & Pervan, S. (2020). Development and validation of a scale measuring hotel website service quality (HWebSQ). Tourism Management Perspectives, 35(2020), 100697. https://doi.org/10.1016/j.tmp.2020.100697
- Lee, A. H. I., Kang, H.-Y., Hsu, C.-F., & Hung, H.-C. (2009). A green supplier selection model for high-tech industry. Expert Systems with Applications, 36(4), 7917–7927. https://doi.org/10.1016/j.eswa.2008.11.052
- Li, M., Li, Y., Peng, Q., Wang, J., & Yu, C. (2021). Evaluating community question-answering websites using interval-valued intuitionistic fuzzy DANP and TODIM methods. Applied Soft Computing, 99(2021), 106918. https://doi.org/10.1016/j.asoc.2020.106918
- Liang, Y., Wang, W., Dong, K., Zhang, G., Qi, G., & Cano, J. C. (2021). Adoption of mobile government cloud from the perspective of public sector. Mobile Information Systems, 2021 1 , 1–20. https://doi.org/10.1155/2021/8884594
- Lin, C.-L., & Tzeng, G.-H. (2009). A value-created system of science (technology) park by using DEMATEL. Expert Systems with Applications, 36(6), 9683–9697. https://doi.org/10.1016/j.eswa.2008.11.040
- Lin, C., & Lin, M. (2019). The determinants of using cloud supply chain adoption. Industrial Management & Data Systems, 119(2), 351–366. https://doi.org/10.1108/IMDS-12-2017-0589
- Lin, H.-F. (2010). An investigation into the effects of IS quality and top management support on ERP system usage. Total Quality Management & Business Excellence, 21(3), 335–349 . https://doi.org/10.1080/14783360903561761
- Liou, J. J. H., Tzeng, G.-H., & Chang, H.-C. (2007). Airline safety measurement using a hybrid model. Journal of Air Transport Management, 13(4), 243–249. https://doi.org/10.1016/j.jairtraman.2007.04.008
- Longstreet, P., Brooks, S., Featherman, M., & Loiacono, E. (2021). Information technology & people, Vol. ahead-of-print No. ahead-of-print ahead–of–print (pp. 1–27). https://doi.org/10.1108/ITP-05-2020-0328
- Luo, X., Gurung, A., & Shim, J. P. (2010). Understanding the determinants of user acceptance of enterprise instant messaging: An empirical study. Journal of Organizational Computing and Electronic Commerce, 20(2), 155–181. https://doi.org/10.1080/10919391003709179
- Mero, R., & Mwangoka, J. (2014). Road map towards eco-efficient cloud computing adoption in higher learning institutions in Tanzania. In 2014 Pan African Conference on Science, Computing and Telecommunications (PACT), 14-18 July 2014, Arusha, Tanzania, (pp. 154–159). IEEE.
- Mohammed, F., Ibrahim, O., & Ithnin, N. (2016). Factors influencing cloud computing adoption for e-government implementation in developing countries. Journal of Systems and Information Technology, 18(3), 297–327. https://doi.org/10.1108/JSIT-01-2016-0001
- Mohammed, F., Ibrahim, O., Nilashi, M., & Alzurqa, E. (2017). Cloud computing adoption model for e-government implementation. Information Development, 33(3), 303–323. https://doi.org/10.1177/0266666916656033
- Mokwena, S., & Hlebela, C. (2018). Factors affecting the adoption of software as a service in south african small medium enterprises. Paper presented at the 2018 Open Innovations Conference (OI), 3-5 Oct. 2018, IEEE, (pp. 1–6). Johannesburg, South Africa.
- Naveed, Q. N., Alam, M. M., Qahmash, A. I., & Quadri, K. M. (2021). Exploring the determinants of service quality of cloud e-learning system for active system usage. Applied Sciences, 11(9), 4176. https://doi.org/10.3390/app11094176
- Naveed, Q. N., Hoda, N., & Ahmad, N. Enhancement of E-Learning performance through OSN. In Proceedings of the 2018 IEEE 5th International Conference on Engineering Technologies and Applied Sciences (ICETAS), Bangkok, Thailand, 22–23 November 2018; IEEE: Bangkok, Thailand, 2018.
- Nunnally, J. C., & Bernstein, I. H. (1994). Psychometric Theory. McGraw-Hill.
- Olaleye, S. A., Sanusi, I. T., Ukpabi, D. C., & Okunoye, A. (2018). Evaluation of Nigeria Universities websites quality: A comparative analysis. Library Philosophy and Practice, 2018(1717), 1–16. https://digitalcommons.unl.edu/libphilprac/1717
- Oliveira, T., & Martins, M. F. (2011). Literature review of information technology adoption models at firm level. The Electronic Journal of Information Systems Evaluation, 14(1), 110–121 https://academic-publishing.org/index.php/ejise/article/view/389/352.
- Oliveira, T., Thomas, M., & Espadanal, M. (2014). Assessing the determinants of cloud computing adoption: An analysis of the manufacturing and services sectors. Information and Management, 51(5), 497–510. https://doi.org/10.1016/j.im.2014.03.006
- Othman, A. K., Hamzah, M. I., & Abu Hassan, L. F. (2020). Modeling the contingent role of technological optimism on customer satisfaction with self-service technologies: A case of cash-recycling ATMs. Journal of Enterprise Information Management, 33(3), 559–578. https://doi.org/10.1108/JEIM-09-2019-0295
- Palos-Sanchez, P. R., Arenas-Marquez, F. J., & Aguayo-Camacho, M. (2017). Cloud computing (SaaS) adoption as a strategic technology: Results of an empirical study. Mobile Information Systems, 2017(1), 1–20. https://doi.org/10.1155/2017/2536040
- Pandjaitan, D. R. H., Mahrinasari, M. S., & Hadianto, B. (2021). Website Quality, E-satisfaction, and E-loyalty of Users Based on The Virtual Distribution Channel. Journal of Distribution Science, 19(7), 113–121. https://doi.org/10.15722/JDS.19.7.202107.113
- Parasuraman, A., Zeithaml, V. B., & Len, L. (1985). A conceptual model of service quality and its implications for future research. Journal of Marketing, 49(4), 41–50. https://doi.org/10.2307/1251430
- Parasuraman, A., Zeithaml, V., & Berry, L. (1994). Reassessment of expectations as a comparison standard in measuring service quality: Implications for further research. Journal of Marketing, 58(1), 111–124. https://doi.org/10.1177/002224299405800109
- Pattnaik, S. (2019). Measuring service quality of food delivery apps-SITEQUAL. Journal of Advanced Research in Dynamical and Control Systems, 11(10), 419–423. https://doi.org/10.5373/JARDCS/V11SP10/20192824
- Priyadarshinee, P., Raut, R. D., Jha, M. K., & Gardas, B. B. (2017). Understanding and predicting the determinants of cloud computing adoption: A two staged hybrid SEM - Neural networks approach. Computers in Human Behavior, 76(C), 341–362. https://doi.org/10.1016/j.chb.2017.07.027
- Qalati, S., Yuan, A., W., L., Khan, M. A. S., & Anwar, F. (2021). A mediated model on the adoption of social media and SMEs’ performance in developing countries. Technology in Society, Elsevier, 64(C), 101513. https://doi.org/10.1016/j.techsoc.2020.101513
- Qasem, Y. A. M., Abdullah, R., Atan, R., & Jusoh, Y. Y. (2018). Mapping and analyzing process of cloud-based education as a service (CEaaS) model for cloud computing adoption in higher education institutions. Paper presented at the 2018 Fourth International Conference on Information Retrieval and Knowledge Management (CAMP), 26-28 March 2018, IEEE, (pp.1–8). Kota Kinabalu, Malaysia.
- Rahayu, K. S., & Saodin. (2021). E-ServQual on E-Trust, E-Satisfaction and Online Repurchase Intention. Review of International Geographical Education Online, 11(4), 1426–1439. https://doi.org/10.48047/rigeo.11.04.135
- Raut, R. D., Gardas, B. B., Narkhede, B. E., & Narwane, V. S. (2019). To investigate the determinants of cloud computing adoption in the manufacturing micro, small and medium enterprises: A DEMATEL-based approach. Benchmarking: An International Journal, 26(3), 990–1019. https://doi.org/10.1108/BIJ-03-2018-0060
- Raza, S. A., Umer, A., Qureshi, M. A., & Dahri, A. S. (2020). Internet banking service quality, e-customer satisfaction and loyalty: The modified e-SERVQUAL model. The TQM Journal, 32(6), 1443–1466. https://doi.org/10.1108/TQM-02-2020-0019
- Rehman, M., Esichaikul, V., & Kamal, M. (2012). Factors influencing e-government adoption in Pakistan. Transforming Government People Process and Policy, 6(3), 258–282. https://doi.org/10.1108/17506161211251263
- Rodríguez, P. G., Villarreal, R., Valiño, P. C., & Blozis, S. (2021). A PLS-SEM approach to understanding E-SQ, E-Satisfaction and E-Loyalty for fashion E-Retailers in Spain. Journal of Retailing and Consumer Services, 57(C), 102201. https://doi.org/10.1016/j.jretconser.2020.102201
- Rotchanakitumnuai, S. (2017). The multi-channel e-service quality for Thai electronic tourism. In Proceedings of The 17th International Conference on Electronic Business (pp. 258–265). ICEB, Dubai, UAE, December 4-8.
- Rovinelli, R., & Hambleton, R. K. (1977). On the use of content specialists in the assessment of criterion-referenced test item validity. Tijdschrift voor Onderwijsresearch, 2 2 , 49–60 https://files.eric.ed.gov/fulltext/ED121845.pdf.
- Safari, F., Safari, N., Hasanzadeh, A., & Ghatari, A. R. (2015). Factors affecting the adoption of cloud computing in small and medium enterprises. International Journal of Business Information Systems, 20(1), 116–137. https://doi.org/10.1504/IJBIS.2015.070894
- Senarathna, I., Wilkin, C., Warren, M., Yeoh, S., & Salzman, S. (2018). Factors that influence adoption of cloud computing: An empirical study of Australian SMEs. Australasian Journal of Information Systems, 22(2018), 1–31. https://doi.org/10.3127/ajis.v22i0.1603
- Shatnawi, T. (2019). Development of an integrated conceptual framework for electronic hedonic service quality (e-HSQ): An exploratory study. Journal of Internet Commerce, 18(4), 395–427. https://doi.org/10.1080/15332861.2019.1639127
- Shieh, J.-I., Wu, -H.-H., & Huang, -K.-K. (2010). A DEMATEL method in identifying key success factors of hospital service quality. Knowledge-Based Systems, 23(3), 277–282. https://doi.org/10.1016/j.knosys.2010.01.013
- Shin, D. H. (2013). User centric cloud service model in public sectors: Policy implications of cloud services. Government Information Quarterly, 30(2), 194–203. https://doi.org/10.1016/j.giq.2012.06.012
- Singh, S., Sahni, M. M., & Kovid, R. K. (2020). What drives FinTech adoption? A multi-method evaluation using an adapted technology acceptance model. Management Decision, 58(8), 1675–1697. https://doi.org/10.1108/MD-09-2019-1318
- Swaid,S., & Wigand,R. (2009). Measuring the quality of e-service: Scale development and initial validation. Journal of Electronic Commerce Research, 10(1), 13–28. http://www.jecr.org/sites/default/files/10_1_p02.pdf
- Tehrani,S.R., & Shirazi,F. (2014). Factors influencing the adoption of cloud computing by small and medium size enterprises (SMEs). In Yamamoto S. (eds), Human interface & management of information: Information & knowledge in applications and services. Lecture Notes in Computer Science 8522 (pp. 631–642). Springer International Publishing.
- Thekkat, A. K., & Anandkumar, S. V. (2021). Luxury shopping websites: The impact of ‘Hygiene’ design factors on trust and distrust. FIIB Business Review, 10(3), 290–299. https://doi.org/10.1177/23197145211032413
- Trivedi, D., Bhatt, A., Trivedi, M., & Patel, P. V. (2021). Digital Library Perspectives, 37(4), 384–400..
- Tzeng, G., Chiang, C., & Li, C. (2007). Evaluating intertwined effects in e-learning programs: A novel hybrid MCDM model based on factor analysis and DEMATEL. Expert Systems with Applications, 32(4), 1028–1044. https://doi.org/10.1016/j.eswa.2006.02.004
- Vanessa, R. (2014). A US-China comparative study of cloud computing adoption behavior: The role of consumer innovativeness, performance expectations and social influence. Journal of Enterprise in Emerging Economies, 6(1), 53–71. https://doi.org/10.1108/JEEE-07-2013-0019
- Vanichbuncha, K. (2005). Statistics for research. CUpress.
- Wahsh, M. A. D., & Singh, J. (2015). A systematic review of factors affecting the adoption of cloud computing for e-government implementation. ARPN Journal of Engineering and Applied Sciences, 10(23), 17824–17832. http://www.arpnjournals.org/jeas/research_papers/rp_2015/jeas_1215_3241.pdf
- Wang, Z., Wang, N., Su, X., & Ge, S. (2020). An empirical study on business analytics affordances enhancing the management of cloud computing data security. International Journal of Information Management, 50(2020), 387–394. https://doi.org/10.1016/j.ijinfomgt.2019.09.002
- Wijaya, I. G. N. S., Triandini, E., Kabnani, E. T. G., & Arifin, S. (2021). E-commerce website service quality and customer loyalty using WebQual 4.0 with importance performances analysis, and structural equation model: An empirical study in shopee. Register: Jurnal Ilmiah Teknologi Sistem Informasi, 7(2), 107–124. https://doi.org/10.26594/register.v7i2.2266
- Wu, -W.-W., & Lee, Y.-T. (2007). Developing global managers’ competencies using the fuzzy DEMATEL method. Expert Systems with Applications, 32(2), 499–507. https://doi.org/10.1016/j.eswa.2005.12.005
- Yang, Y.-P., Shieh, H.-M., Leu, J.-D., Tzeng, & Wo-hshiung. (2008). A novel hybrid MCDM model combined with DEMATEL and ANP with applications. International Journal Operational Research, 5(3), 160–168.
- Yoo, S.-K., & Kim, B.-Y. (2019). The effective factors of cloud computing adoption success in organization. Journal of Asian Finance, Economics and Business, 6(1), 217–229. https://doi.org/10.13106/jafeb.2019.vol6.no1.217
- Yu, R., & Tzeng, G.-H. (2006). A soft computing method for multi-criteria decision making with dependence and feedback. Applied Mathematics and Computation, 180(1), 63–75. https://doi.org/10.1016/j.amc.2005.11.163
- Yuvaraj, M. (2016). Determining factors for the adoption of cloud computing in developing countries. The Bottom Line, 29(4), 259–272. https://doi.org/10.1108/BL-02-2016-0009
- Zhao, F., Scheruhn, H. J., & Von Rosing, M. (2014). The impact of culture differences on cloud computing adoption. In M. Kurosu (Ed.), Human-computer interaction. Applications and services. HCI 2014. Lecture notes in computer science (Vol. 8512, pp. 776–785). Springer. https://doi.org/10.1007/978-3-319-07227-2_74
- Zhu, K., Dong, S., Xu, S. X., & Kraemer, K. L. (2006). Innovation diffusion in global contexts: Determinants of post-adoption digital transformation of European companies. European Journal of Information Systems, 15(6), 601–616. https://doi.org/10.1057/palgrave.ejis.3000650