Abstract
The study aims to review the challenges of implementing big data by Malaysian small- and medium-size enterprises (SMEs)as well as to figure out the relationship between architecture, social, law and market. Currently, there is limited research and literature examining the challenges of big data analysis in Malaysia SMEs. This paper has opted for a thorough analysis of the complexities of implementing big data at SMEs in Malaysia by applying a comprehensive literature search from different databases, journals, Google scholar, IEEE and content review. This research offers practical insights into the complexities of taking Big Data in SMEs in Malaysia. It is proposed that law, architecture, social and market can be applied by Malaysian SMEs to adopt big data analytic. The authors used quantitative survey. This is done by soliciting criteria from general managers/managing directors/CEOs or data analysts from LinkedIn group. Data wereanalysed by partial least square. This research makes contribution to academics and practitioners to the emerge of predictive model to suit the challenges of big data adoption in Malaysia SMES.
PUBLIC INTEREST STATEMENT
This study figured the challenges faced by Malaysia SMEs based on the legal, architecture, social and market issues. Based on the review of previous literature studies in this field, it is evident that there is a need to highlight a more comprehensive framework for identifying the challenges faced by Malaysia SMEs. The results show that architecture has significant effect on market, architecture has significant effect on social, law has significant effect on market and social has significant effect on market. The limitation of this research is small sample sizes that may be impossible to generalise the findings of this study. Future research will be conducted in more geographical area as well to increase the sample sizes to obtain more generalizing results.
1. Introduction
Small- and medium-size enterprises (SMEs) can be defined as small, medium and micro-sized enterprises. Medium-sized businesses have less than 250 employees and a turnover of less than 50 million euros or a monetary record aggregate of less than 43 million euros. (European Commission, Citation2016). Small-sized organisations, on the other hand, have fewer than 50 employees and a turnover of less than ten million euros or an accounting report aggregate of less than ten million euros (European Commission, Citation2016). Micro-sized businesses have less than ten employees and a turnover of less than two million euros (European Commission, Citation2016). The Ministry of International Trade (MITI) is the largest supporter of SMEs in Malaysia, assisting and protecting Malaysian interests in international trade and encouraging the development of industrial operations (Hashim & Wafa, Citation2002; Mori, Citation2005; Zizah et al., Citation2010). The Malaysian Industrial Development Association (MIDA), established under the Malaysian Investment Development Authority Act, is the government’s primary body for promoting Malaysia’s manufacturing and service industries and ensuring that the country’s economy improves (Hashim & Wafa, Citation2002; Mori, Citation2005; Zizah et al., Citation2010).
The Small and Medium Industries Development Corporation (SMIDEC) is another government body that is responsible for expanding skilled and resilient Malaysian SMEs so that they may compete globally (Hashim & Wafa, Citation2002; Mori, Citation2005; Zizah et al., Citation2010). MATRADE (Malaysia External Trade Development Corporation) is tasked with promoting Malaysia’s exports and assisting many local businesses in gaining new market share in international markets (Hashim & Wafa, Citation2002; Mori, Citation2005; Zizah et al., Citation2010). Even with the exponential expansion of big data in SMEs, adoption of big data by SMEs is still limited (MacGregor & Vrazalic, Citation2005) and large organisations are shown to profit more from SMEs in terms of enhanced sales and cost reductions enabled by big data (Riquelme, Citation2002).
The awareness of investing big data analytic in SMEs is important (Ghobakhloo et al.). According to Soroka et al. (Citation2017), big data adoption enables SMEs to achieve overall measures in cost, profitability, revenues, growth and agility through performance analysis, productivity, efficiency, new market divisions, financial stability and control and innovation. The potential value of big data shines when used to carry out the decision-making process (Provost & Fawcett, Citation2013). Big data can boost SMEs’ competitiveness by integrating supply chain partners and inter-organizational functions, while also providing critical information (Bhagwat & Sharma, Citation2007). Big data can be of great assistance to SMEs by providing the infrastructure required to ensure that the right types of information are available at the right time (Premkumar, Citation2003).
2. Literature review
The approach employed in this study involves a comprehensive library search and literature review on Malaysia SMEs and various challenges highlighted, irrespective of economical, organisational or managerial aspects. The library search consists of online and offline content, ranging from newspaper articles to book articles.
A summary of the challenges of big data analytics faced by Malaysia SMEs are shown in .
Table 1. Summary of challenges of big data analytics faced by Malaysia SMEs
2.0.1. Legal perspectives: Concerns about data protection and data privacy
One of big challenge in big data analysis for SMEs stated by Jagadish et al. (Citation2014) is data protection and data privacy. There is risk of personal data particulars are not appropriately used during data analysis process (Jambunathan & Venkatesan, Citation2016). The usage of big data especially in funding agencies, academic and medical sectors in term of data protection and security is much lower (Mardis, Citation2016). Various researchers have studied on data privacy and security issues in data analysis. For instance, Sorensen et al. (2010) examined privacy issues concerning the hosting of open accessible servers. Furthermore, it is difficult and need extra budget to find a lawyer consulting data protection (Coleman et al., Citation2016).
2.0.2. Social perspectives
Social issues especially organisation culture is another concern of implementing big data analysis in Malaysia SMEs. Coleman et al. (Citation2016) pointed out that people in the organisation are not ready for co-ownership information and there is a must to develop a non-hierarchical culture of trust. Organisations need to be open minded and willing to change to new environmental challenges (Manyika et al.). Smith (Citation2007) pointed out that the social norms in the nation also influenced the adoption of big data analysis particularly in SMEs.
2.0.3. Architecture perspectives: Lack of infrastructure to process high volume data efficiently
Infrastructure is another challenge of big data analysis in SMEs. Companies need a good equipment, hardware, software and middleware to process big data to handle and interpret data quickly and efficiently (Laney, Citation2001). Still yet, SMEs find it difficult to handle challenge as they are not able to process data rapidly as a result of the lack of infrastructure (Del Vecchio et al., Citation2018).
Market perspectives: Highly complex analytics solutions in the software market
Market issues especially lack of availability of existing analytical software tools in the market is another challenge of implementing big data in companies. For example, it is hard to identify analytical solutions with powerful analytical capabilities together with interactive user-friendly features (Coleman et al., Citation2016). Coleman et al. (Citation2016) and Iqbal et al. (Citation2018) stated that analytical software tools that exist in the market are two types. However, these two types of software are not suitable owing to the absence of expertise in data analytics (Coleman et al., Citation2016) and simple but not useful (Coleman et al., Citation2016; Iqbal et al., Citation2018).
3. Hypothesis
Big data harnessing can lead to many challenges, which include design of system for processing, the means for analysing and the problems of scientific research. The authors form hypotheses as below:
Hypothesis 1: Architecture has significant effect on market
Hypothesis 2: Architecture has significant effect on social
Hypothesis 3: Law has significant effect on market
Hypothesis 4: Social has significant effect on market
4. Methodology
Typically, the survey was conducted in the duration of seven months, from January 2021 to July 2021. First, the heads of Human Resource or Public Relation department staffs of the Malaysia SMES were contacted through a phone conversation or through e-mail. The e-mail was sent to Human Resource or Public Relation department to invite them whether the companies are interested to participate in the survey and inform the companies the estimated time that might be taken for the survey. The companies were informed that the survey is for research purpose only and ensured that the participant’s identities were kept secret, not disclosed to any unauthorised third party. Next, if the companies agree to participate in the survey, survey link was sent to the participants to fill up the survey. To improve the response rate, invitation also was sent in various Facebook group and LinkedIn group, such as Malaysia big data group, Malaysia SMES group, big data analysis group. Among 121 survey questionnaires sent, only 43 respondents were obtained. The response rate is 35%. below shows the demographics of the respondents.
Table 2. Type of industries
shows the distribution of the type of the industry of the companies that participated in the survey. It is found that out of 43 respondents, others industry was the highest (37.2%), followed by financial services (27.9 %), manufacturing (14 %), oil and gas processing (7%), hotel and restaurants (4.7%), healthcare and life sciences (4.7%) and telecommunication (2.3%).
indicates the company’s size. With respect to the size of an organisation, it is classified into micro (with less than 10 employees), small (10–49 employees) and medium (50–250 employee). It was found that maximum number of the participants came from the medium companies (76.7%), followed by micro-companies (14%) and from the small companies (9.3%).
Table 3. Company’s size distribution
5. Results and analysis
In this research, the software SmartPLS Version 2.0 has been used. Partial least square (PLS) is a technique for analysing and modelling data (Reinartz et al., Citation2009). PLS has been widely used in the study of information systems and other business disciplines (Henseler et al., Citation2009). PLS is powerful tool especially to deal with small data (Majchrzak et al., Citation2005). Hair et al. (Citation2011) introduced “10-times rule” technique, which widely used minimum sample size estimation method in PLS-SEM. It is based on the concept that the sample size should be more than 10 times the maximum number of inner or outer model linkages pointing at any latent variable in the model. In this research, only 4 variables were involved, and hence according to Hair et al. (Citation2011), the minimum sample should be 40. From the survey conducted, 44 respondents were obtained, and thus this fulfilled the c) rule.
presents the PLS-SEM structure. The PLS-SEM structure is two exogenous variable (social and market) and two endogenous variables (law and architecture).
Architecture: 5 items
Law: 3 items
Social: 4 items
Market: 4 items
shows the loadings, composite reliability and average variable extracted (AVE) value for latent variables (architecture, law, social and market). It was found from that all the indicators have achieved value more than 0.6. Wong (Citation2013) stated that minimum acceptance level of indicator reliability is 0.4. So, the loading of all the indicators are reliable and valid.
Table 4. Loading, composite reliability and AVE value for latent variables
It also found that composite reliability of all the latent variables have achieved value more than 0.7. Wong (Citation2013) stated that minimum acceptance level composite reliability is 0.7. So, the composite reliability of all the latent variables are well established.
In term of convergent validity, aAVE for latent variables is examined. indicates the AVE value for all the latent variables that are greater than the acceptable threshold of 0.5 (Wong, Citation2013), and so convergent validity is confirmed.
5.1. Discriminant validity
Fornell and Larcker (Citation1981) argued that if the square root of AVE in each latent variable is greater than other correlation values among the latent variables, then this value can be used to show discriminant validity. To do so, a table is prepared with the square root of AVE manually calculated and represented in bold diagonally in the table. showed that latent variable is greater than other correlation values among the latent variables, thus discriminant validity is proved.
Table 5. Discriminant validity
shows the value of R squared. Hair et al. (Citation2011) stated that minimum R squared value must be 0.2, while Frost (Citation2014) stated that minimum R squared value must be 0.25. Frost (Citation2014) explained that endogenous (independent) variable is significance, p value must be more than 0.05 and coefficient is 2. Therefore, an increase in one unit of endogenous (independent) variable will result in an increase in two units of exogenous (dependent) variable. In another word, by increasing one unit of exogenous (dependent) variable will result in increasing 0.5 unit of endogenous (independent) variable, and hence R value is 0.5. R squared is 0.5 × 0.5 = 0.25. From , it was found that R squared value for latent variables for market are more than 0.25; hence, the model is considered a good fit. The R squared value for social is 0.143. This mean that the relationship of architecture to social is weak.
Table 6. R squared value
shows the path coefficients
Hypothesis 1: Architecture has significant effect on market.
From the table above, it is found that T statistics for architecture->market is 3.69, which is larger than 1.96; therefore, hypothesis 1 is accepted.
Hypothesis 2: Architecture has significant effect on social.
From the table above, it is found that T statistics for architecture->social is 5.01, which is larger than 1.96; therefore, hypothesis 2 is accepted.
Hypothesis 3: Law has significant effect on market.
From the table above, it is found that T statistics for law->market is 2.52, which is larger than 1.96; therefore, hypothesis 3 is accepted.
Hypothesis 4: Social has significant effect on market.
From the table above, it is found that T statistics for social->market is 3.57, which is larger than 1.96; therefore, hypothesis 3 is accepted.
Table 7. The path coefficients
6. Discusssion
The resource-based view (RBV) theory argued that a source of competitive advantage is the firm’s resources and capabilities (Afsharghasemi et al., Citation2013). The resource-based view indicated that a corporation can only gain a competitive advantage if it has access to distinct value resources such as having good architecture that are difficult for competitors to mimic (Afsharghasemi et al., Citation2013). Market orientation is seen as one of the internal capabilities and resources that might potentially lead to a sustained competitive advantage. It is valuable, rare, not interchangeable and imperfectly imitable (Hult et al., Citation2005; Zhou et al., Citation2008). The RBV is a resource that can help us understand the nature of market orientation. It is an intangible characteristic of a corporation that allows it to supervise market information and give higher value to its consumers (Hunt & Lambe, Citation2000). Kaur and Kaur (Citation2018) stated that there are two types of infrastructure: social and economic infrastructure. The social infrastructure is the infrastructure that assist in the promotion of education, health and community-related standard of the people (Kaur & Kaur, Citation2018). Economic infrastructure is the infrastructure that helps to promote the economic/market environment. Thus, infrastructure plays important role for social and market (Kaur & Kaur, Citation2018). Social norms is defined as various standards, customs, values, beliefs and faith (Ocansey, Citation2017). Cultures produce a great challenge for marketing worldwide (Ocansey, Citation2017). Fathoni and Rodoni (Citation2018) found that there is impact on organization culture towards marketing performance. Based on their ethical culture, organizations may be categorized as ethical or immoral firms (Chadegani & Jari, Citation2016). Khayatmoghadam (Citation2020) proved that there is significant relationship between professional ethics with organization culture.
The RBV stated that a company wants to take advantage of the legal system (law) must not only be able to identify legal resources but also be able to deploy those resources to meet its financial goals (Masson & Shariff, Citation2011). As a result, the company must possess specific aptitudes, such as competence, to utilize its resources efficiently and successfully in accordance with its economic objectives (market; Masson & Shariff, Citation2011).
In term of architecture (infrastructure), the organisations need a good quality of hardware, software and middleware to handle and process big data analysis. However, one of the main challenges of the SME facing is the high cost of infrastructure. Therefore, it is suggested for SME to utilize cloud computing technology without investing so much on the infrastructure. The organisations can use software on demand like SaaS (Software-as-a-Service) to overcome the budget issues.
In short, the research provides a thorough understanding of the challenges associated with big data adoption in SMEs. This study is useful especially for the academic or IT professionals who want to adopt big data in SMEs. The outcome of this research will also help to improve the performance of big data adoption in SMEs from a management standpoint.
7. Conclusion
This study figured the challenges faced by Malaysia SMEs based on the legal, architecture, social and market issues. Based on the review of previous literature studies in this field, it is evident that there is a need to highlight a more comprehensive framework for identifying the challenges faced by Malaysia SMEs. The results show that architecture has significant effect on market, architecture has significant effect on social, law has significant effect on market and social has significant effect on market. The limitation of this research is small sample sizes that may be impossible to generalise the findings of this study. Future research will be conducted in more geographical area as well to increase the sample sizes to obtain more generalizing results.
Disclosure statement
No potential conflict of interest was reported by the author(s).
Additional information
Funding
Notes on contributors
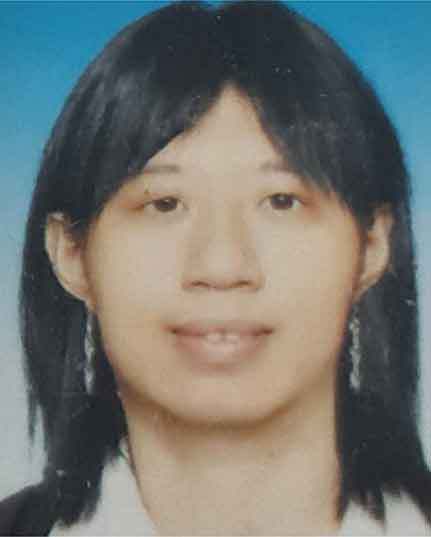
Min-Hooi Chuah
Min-Hooi Chuah, Dr.Chuah MH, received her PhD from Universiti Tunku Abdul Rahman in the area of Business Intelligence in 2019. She was part time lecturer in several university such as UCSI University, Kolej Tunku Abdul Rahman, First City University College. Currently, she is postdoctoral fellow in Universiti Sains Malaysia. She started her teaching career as lecturer with the joined Universiti Tunku Abdul Rahman (UTAR) in the Faculty of Information and Communication Technology in Perak Campus (FICT-PK).
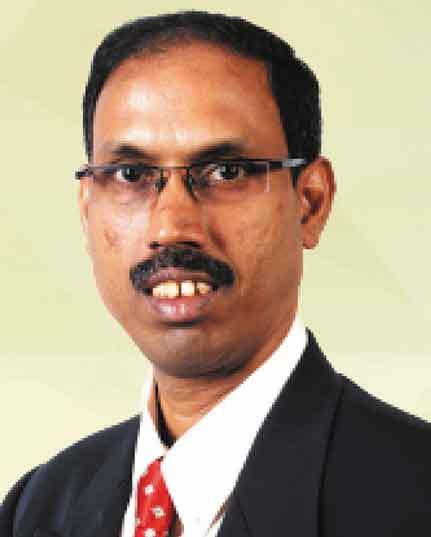
Ramayah Thurusamry
Ramayah Thurusamry, Professor Ramayah Thurasamy is currently attached to the Operations Management section at the School of Management, Universiti Sains Malaysia. He has also presented numerous papers at local and international conferences having won 5 Best Papers award. He also serves on the editorial boards and program committee of several international journals and conferences of repute. He has completed 23 funded research projects. He has published 285 research articles in journals contributed as author/co-author. He is also acting as reviewer for several journals.
References
- Afsharghasemi, A., Zain, M., Sambasivan, M., & Imm, S. N. S. (2013). Market orientation, government regulation, competitive advantage and internationalization of SMEs: A study in Malaysia. Journal of Business Administration Research, 2(2), 13–11. https://doi.org/10.5430/jbar.v2n2p13
- Ardagna, C. A., Ceravolo, P., & Damiani, E. (2016, December). Big data analytics as-a-service: Issues and challenges. In 2016 IEEE international conference on big data (big data), IEEE, 3638–3644.
- Baharuden, A. F., Isaac, O., & Ameen, A. (2019). Factors influencing big data & analytics (BD&A) learning intentions with transformational leadership as moderator variable: Malaysian sme perspective. International Journal Of Management And Human Science, 3(1), 10–20.
- Bhagwat, R., & Sharma, M. K. (2007). Information system architecture: A framework for a cluster of small and medium-sized enterprises (SMEs). Production Planning & Control, 18(4), 283–296 .
- Chadegani, A. A., & Jari, A. (2016). Corporate ethical culture: Review of literature and introducing PP model. Procedia Economics and Finance, 36, 51–61. https://doi.org/10.1016/S2212-5671(16)30015-6
- Coleman, S., Göb, R., Manco, G., Pievatolo, A., Tort‐Martorell, X., & Reis, M. S. (2016). How can SMEs benefit from big data? Challenges and a path forward. Quality and Reliability Engineering International, 32(6), 2151–2164. https://doi.org/10.1002/qre.2008
- Del Vecchio, P., Di Minin, A., Petruzzelli, A. M., Panniello, U., & Pirri, S. (2018). Big data for open innovation in SMEs and large corporations: Trends, opportunities, and challenges. Creativity and Innovation Management, 27(1), 6–22. https://doi.org/10.1111/caim.12224
- European Commission. 2016. User guide to the SME definition, user guide to the SME Definition. Accessed: 24 July 2018. http://ec.europa.eu/growth/smes/business-friendly-environment/sme-definition/index_en.htm
- Fathoni, M. A., & Rodoni, A. (2018). The impact of corporate culture on marketing performance. Iqtishadia: Jurnal Kajian Ekonomi Dan Bisnis Islam STAIN Kudus, 11(1), 129–154. https://doi.org/10.21043/iqtishadia.v11i1.2847
- Fornell, C., & Larcker, D. F. (1981). Evaluating structural equation models with unobservable variables and measurement error. Journal of Marketing Research, 18(1), 39–50. https://doi.org/10.1177/002224378101800104
- Frost, J. (2014). How high should R-squared be in regression analysis. Minitab Blog, 9(1 https://blog.minitab.com/en/adventures-in-statistics-2/how-high-should-r-squared-be-in-regression-analysis).
- Gupta, S., Kar, A. K., Baabdullah, A., & Al-Khowaiter, W. A. (2018). Big data with cognitive computing: A review for the future. International Journal of Information Management, 42, 78–89. https://doi.org/10.1016/j.ijinfomgt.2018.06.005
- Hair, J. F., Ringle, C. M., & Sarstedt, M. (2011). PLS-SEM: Indeed a silver bullet. Journal of Marketing Theory and Practice, 19(2), 139–152. https://doi.org/10.2753/MTP1069-6679190202
- Hashim, M. K., & Wafa, S. A. (2002). Small and medium-sized entreprises in Malaysia: Development issues. Prentice Hall, Pearson Malaysia Sdn Bhd.
- Henseler, J., Ringle, C. M., & Sinkovics, R. R. (2009). The use of partial least squares path modeling in international marketing. Advances in International Marketing, 20, 277–319 .
- Hult, G. T. M., Ketchen, D. J., & Slater, S. F. (2005). Market orientation and performance: An integration of disparate approaches. Strategic Management Journal, 26(12), 1173–1181. http://dx.doi.org/10.1002/smj.494
- Hunt, S., & Lambe, J. (2000). Marketing’s contribution to business strategy: Market orientation, relationship marketing and resourced-advantage theory. International Journal of Management Review, 2(1), 17–43. https://doi.org/10.1111/1468-2370.00029
- Iqbal, M., Kazmi, S. H. A., Manzoor, A., Soomrani, A. R., Butt, S. H., & Shaikh, K. A. (2018, March). A study of big data for business growth in SMEs: Opportunities & challenges. In 2018 international conference on computing, mathematics and engineering technologies (iCoMET), IEEE, 1–7 https://www.ijltemas.in/DigitalLibrary/Vol.5Issue11/67-70.pdf.
- Jagadish, H. V., Gehrke, J., Labrinidis, A., Papakonstantinou, Y., Patel, J. M., Ramakrishnan, R., & Shahabi, C. (2014). Big data and its technical challenges. Communications of the ACM, 57(7), 86–94. https://doi.org/10.1145/2611567
- Jambunathan, V., & Venkatesan, S. (2016). A review on big data challenges and opportunities. International Journal of Latest Technology in Engineering Management & Applied Science, 5(11), 67–70 https://www.ijltemas.in/DigitalLibrary/Vol.5Issue11/67-70.pdf.
- Kalan, R. S., & Unalir, M. O. 2016. Leveraging big data technology for small and medium-sized enterprises (SMEs). in 6th International Conference on Computer and Knowledge Engineering (ICCKE), 1–6.
- Karim, S., Al-Tawara, A., Gide, E., & Sandu, R. (2017, May). Is big data too big for SMEs in Jordan? In 2017 8th International Conference on Information Technology (ICIT), IEEE, 914–922.
- Kaur, A., & Kaur, R. (2018). Role of social and economic infrastructure in economic development of Punjab. International Journal of Innovative Knowledge Concepts, 6(5 https://www.semanticscholar.org/paper/Role-of-Social-and-Economic-Infrastructure-in-of-Kaur-Kaur/a14bb9e98141b2d010d7b4a3a70800d161452e9d).
- Khayatmoghadam, S. (2020). The effect of professional ethics on the organizational culture. Journal Homepage: www.Ijethics.Com 2(1).
- Kızıltan, A. (2018). Challenges of big data adoption in Turkish SMEs: A case study.
- Laney, D. (2001). 3D data management: Controlling data volume, velocity and variety. META Group Research Note, 6(70), 1 https://studylib.net/doc/8647594/3d-data-management--controlling-data-volume--velocity--an.
- Limpeeticharoenchot, S., Cooharojananone, N., Chanvanakul, T., Tuaycharoen, N., & Atchariyachanvanich, K. (2020). Innovative mobile application for measuring big data maturity: Case of SMEs in Thailand. International Journal of Interactive Mobile Technologies (Ijim), 14(18), 87. https://doi.org/10.3991/ijim.v14i18.16295
- MacGregor, R. C., & Vrazalic, L. (2005). A basic model of electronic commerce adoption barriers: A study of regional small businesses in Sweden and Australia. Journal of Small Business and Enterprise Development, 12(4), 510–527. https://doi.org/10.1108/14626000510628199
- Majchrzak, A., Beath, C. M., Lim, R. A., & Chin, W. W. (2005). Managing client dialogues during information systems design to facilitate client learning. MIS Quarterly, 29(4), 653–672. https://doi.org/10.2307/25148704
- Makhele, S. L. 2018. Investigating how SMEs can benefit from big data analytics. (Doctoral dissertation, North-West University).
- Mardis, E. R. 2016. The challenges of big data.
- Masson, A., & Shariff, M. (2011). Through the legal looking glass: Exploring the concept of corporate legal strategy. EBLR, 2011 https://www.academia.edu/34201764/EXPLORING_THE_CONCEPT_OF_CORPORATE_LEGAL_STRATEGY_Through_the_Legal_Looking_Glass_Exploring_the_Concept_of_Corporate_Legal_Strategy.
- Mbassegue, P., Escandon-Quintanilla, M. L., & Gardoni, M. (2016, July). Knowledge management and big data: Opportunities and challenges for small and medium enterprises (SME). In IFIP international conference on product lifecycle management, Springer, Cham, 22–31.
- Mori, J. (2005). Malaysia’s Challenges to Industrial Linkage: Policy Coordination at Local and National Level. The Fletcher School, Tufts University.
- Moyne, J., & Iskandar, J. (2017). Big data analytics for smart manufacturing: Case studies in semiconductor manufacturing. Processes, 5(3), 39. https://doi.org/10.3390/pr5030039
- Nemati, S., & Khajeheian, D. (2018). Big data for competitiveness of SMEs: Use of consumer analytic to identify niche markets. In Competitiveness in emerging markets (pp. 585–599). Springer.
- Ocansey, E. (2017). The influence of culture on global marketing strategies: A confirmatory study. The International Journal of Business & Management, 5, 150–155 https://www.researchgate.net/publication/320775259_The_Influence_of_Culture_on_Global_Marketing_Strategies_A_Confirmatory_Study.
- Premkumar, G. (2003). A meta-analysis of research on information technology implementation in small business. Journal of Organizational Computing and Electronic Commerce, 13(2), 91–121. https://doi.org/10.1207/S15327744JOCE1302_2
- Provost, F., & Fawcett, T. (2013). Data science and its relationship to big data and data-driven decision making. Big Data, 1(1), 51–59. https://doi.org/10.1089/big.2013.1508
- Rajabion, L. (2018, December). Application and adoption of big data technologies in SMEs. In 2018 International Conference on Computational Science and Computational Intelligence (CSCI), IEEE, 1133–1135.
- Reinartz, W., Haenlein, M., & Henseler, J. (2009). An empirical comparison of the efficacy of covariance-based and variance-based SEM. International Journal of Research in Marketing, 26(4), 332–344. https://doi.org/10.1016/j.ijresmar.2009.08.001
- Riquelme, H. (2002). Commercial Internet adoption in China: Comparing the experience of small, medium and large businesses. Internet Research-Electronic Networking Applications and Policy, 12(3), 276–286. https://doi.org/10.1108/10662240210430946
- Seseni, L., & Mbohwa, C. 2018. The effects of using big data in furniture manufacturing SMEs. In Proceedings of the international conference on industrial engineering and operations management, 1404–1412.
- Shah, S., Soriano, C. B., & Coutroubis, A. D. (2017, December). Is big data for everyone? The challenges of big data adoption in SMEs. In 2017 IEEE International Conference on Industrial Engineering and Engineering Management (IEEM), IEEE, 803–807.
- Sivarajah, U., Kamal, M. M., Irani, Z., & Weerakkody, V. (2017). Critical analytics of Big Data challenges and analytical methods. Journal of Business Research, 70, 263–286. https://doi.org/10.1016/j.jbusres.2016.08.001
- Smith, M. (2007). “Real” managerial differences between family and non‐family firms. International Journal of Entrepreneurial Behavior & Research, 13(5), 278–295. https://doi.org/10.1108/13552550710780876
- Soroka, A., Liu, Y., Han, L., & Haleem, M. S. (2017). Big data driven customer insights for SMEs in redistributed manufacturing. Procedia CIRP, 63, 692–697. https://doi.org/10.1016/j.procir.2017.03.319
- Vajjhala, N. R., & Ramollari, E. (2016). Big data using cloud computing-opportunities for small and medium-sized enterprises. European Journal of Economics and Business Studies, 2(1), 129–137. https://doi.org/10.26417/ejes.v4i1.p129-137
- Wong, K. K. K. (2013). Partial least squares structural equation modeling (PLS-SEM) techniques using SmartPLS. Marketing Bulletin, 24(1), 1–32 doi:10.1007/978-3-319-05542-8_15-2.
- Zhou, K. Z., Li, J. J., Zhou, N., & Su, C. (2008). Market orientation, job satisfaction, product quality, and firm performance: Evidence from China. Strategic Management Journal, 29(9), 985–1000. http://doi.org/10.1002/smj.700
- Zizah, C. S., Rosmah, M. I., Scott-Ladd, B., & Lanny, E. (2010). Influential factors for SME internationalization: Evidence from Malaysia. Int. Journal of Economics and Management, 4(2), 285–304 https://espace.curtin.edu.au/handle/20.500.11937/39266.