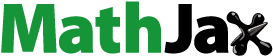
Abstract
There is a large body of research investigating the impact of information and communication technologies (ICT) on economic growth; however, the empirical evidence on growth and its volatility simultaneously driven by ICT diffusion has still remained scarce so far. To fill this void, system two-step generalized method of moments (S-GMM) estimator and a new proxy capturing the product of growth and its fluctuation is employed to determine the nexus between ICT penetration and the interaction of growth and its volatility. Using a comprehensive panel of 122 economies covering the period 2000–2019, we find that all proxies for the ICT revolution show positive impacts on the product of growth and its volatility. This means that an increase in ICT diffusion could lead to both higher growth and lower growth volatility. To offer robust and consistent results, ICT growth and its volatility nexus are confirmed through several econometric techniques such as panel quantile regression, the control of fixed effects, alternative measure for the interaction between growth and its fluctuation, and across countries with different national incomes. The research could give rise to use of this proxy for the product of growth and its volatility with inverse hyperbolic sine transformation in the empirically econometric model for the future studies.
PUBLIC INTEREST STATEMENT
Current research provides a fresh understanding for the traditional nexus between ICT diffusion and growth by capturing both vital aspects of growth such as growth rate of GDP and its fluctuation. Accordingly, the mass of ICT penetration simultaneously increases economic growth and decreases growth volatility. These results stem from a relatively comprehensive sample of emerging, developing, and developed countries, which encourages the implementation of ICT development policy to stimulate economic activities. In addition, growth and its volatility are measured in a new single proxy with inverse hyperbolic sine transformation, which potentially draws more intention for future research on plausible factors driving this indicator or the impact of this variable on other macroeconomic factors.
1. Introduction
The world has changed dramatically in recent decades as the ICT revolution has displayed its expansion into diverse levels such as organization level, sector level, and country level (Vu et al., Citation2020). The tremendous impact of ICT on the volume of international financial transactions has been acknowledged (Dell’Ariccia et al., Citation1998).). Financial institutions could take advantage of ICT development to not only expand their financial services globally but also to supply them at a reasonable cost, thereby stimulating economic growth.
Given the ICT benefits, there has been a lot of research devoted to the contribution of ICT penetration to economic growth (Adeleye & Eboagu, Citation2019; Stanley et al., Citation2018). Vu (Citation2011) providing a thorough analysis shows that many developing countries have been able to utilize their backwardness advantage to stimulate economic progress thanks to considerable progress in internet-based information technology. Following Vu et al. (Citation2020), the impact of ICT on economic growth could be attributed to several mechanisms, such as (i) learning and technological evolution, (ii) high-quality decision making, and (iii) a decrease in cost and extension of choices driving the supply and demand curves.
The effect of ICT diffusion on economic growth has also been widely discussed by scholars in recent years because ICT penetration is one of the main prerequisites for promoting economic expansion. This is confirmed in much empirical research on growth-led ICT penetration such as Jorgenson (Citation2001), Nasab and Aghaei (Citation2009), Vu (Citation2011), and among others. However, the favorable impact of ICT diffusion show heterogeneity among different type of countries as noted in the work of Niebel (Citation2018) who shows that developing and emerging countries are not gaining more from investments in ICT than developed economies.Footnote1 Moreover, the author also shows that it is unknown whether ICT has a greater influence on economic growth in emerging and developing economies than in industrialized economies.Footnote2 We argue that this could be attributed to insufficient aspects captured in economic growth in which both growth and its volatility need to be combined. A well-established link between growth and its volatility for a long time in the typical work of Imbs (Citation2007) who acknowledges that volatility is common to all activities and has a negative correlation with growth. Therefore, we propose that both growth dimensions such as the growth rate of economic activities and its fluctuations should be integrated into one single product of growth and growth fluctuations before investigating determinants of this proxy. One should note that among determinants of the product of growth and its volatility such as corruption (Evrensel, Citation2010), finance (Da Silva et al., Citation2017), and international trade openness (Kim et al., Citation2016), we could not find the role of ICT penetration on this proxy, which encourages us to conduct further investigation on this link and determine whether this link is heterogeneous among different types of countries with various levels of income.
Despite a large number of studies showing a positive association of ICT penetration with higher growth, productivity, and employment (Gómez-Barroso & Marbán-Flores, Citation2020; Vu et al., Citation2020), there is limited research on ICT-driven volatility of economic growth. Instead, several studies have proven the reduction in economic growth as a result of output volatility. For example, Ramey and Ramey (Citation1995) indicate that production volatility and growth have a strong negative relationship due to “uncertainty-induced planning errors by firms”.
In addition, there is another strand of literature attempting to understand the determinants of output volatility (Hakura, Citation2009; Iseringhausen & Vierke, Citation2018; Kose et al., Citation2006; Levchenko & Di Giovanni, Citation2008; Malik & Temple, Citation2009). In typical empirical evidence, Balavac and Pugh (Citation2016) determine several drivers of economic growth volatility such as trade openness, export diversification, and institutions in a sample of 25 economies spanning from 1996 to 2010. Additionally, Iseringhausen and Vierke (Citation2018) support demographics and fiscal policy as potential factors shaping output volatility.
To the best of understanding, there are no studies directly looking at the influence of ICT on the interaction of growth and its volatility. As an example of related research, as one of pioneering research, Zimmermann (Citation1994) shows that in most research cases, technological advancements could affect the business cycle in an open economy rather than in a closed one. To support this finding, Comin et al. (Citation2009) suggest that technological innovation and diffusion are among key drivers of the business cycle. Besides, Dynan et al. (Citation2006) consider financial innovations as a possible contributor to the stabilization of economic activity in the mid-1980s. In addition, the importance of the ICT in explaining growth volatility for a panel of 10 Asian countries was discussed in the work of Ko (Citation2008), suggesting that when it comes to monetary policy shocks, countries with a high level of ICT development yield more output volatility but fewer output fluctuations in the case of fiscal policy shocks. More recently, Cavoli et al. (Citation2020) employ a sample of 100 emerging and developing countries covering the period of 1995–2013, showing that countries with a high level of financial inclusion and lower income tend to face a considerable trade-off between financial inclusion and production stability. Moreover, ICT penetration is employed as a key indicator for financial inclusion (Bhuvana et al., Citation2016), thereby decreasing economic growth instability and reducing poverty and inequality (Mushtaq & Bruneau, Citation2019). In addition, Ferraro (Citation2017) shows that economies with more volatile real GDP per capita growth rates have significantly longer time delays in ICT adoption and lower average growth.
Noting that these empirical findings limited our understanding in ICT-led growth fluctuations and do not directly address the impact of ICT diffusion on the interaction of growth and its volatility. Da Silva et al. (Citation2017) point out the drawback of a widely used measure for growth volatility based on the approach of standard deviation of economic growth, showing the inability to compare the fluctuation among economies with different average growth levels. This gives us a reason to investigate whether the ICT revolution has a significant influence on both growth and its volatility.
The goal of this research is to look at the impact of ICT evolution on the interaction of economic growth and its volatility by employing a sample of 122 countries between 2000 and 2019. Motivated from the work of Da Silva et al. (Citation2017), the economic volatility is constructed by the interaction between average GDP growth and standard deviation of GDP per capita growth in an inverse hyperbolic sine transformation, which is largely ignored from previous studies. We apply a two-step system generalized method of moments to address the issue of endogeneity and the dynamic property of the model. The findings suggest that the mass diffusion of ICT could make a profound contribution to both increase economic growth and decrease its volatility. This finding is consistent with a variety of sensitivity tests such as quantile panel regression, the control of fixed-effects, and an alternative measure for the interaction of growth and its volatility. In addition, the results also hold true when using two sub-samples divided according to country’s income into high-income countries and medium–low income countries. Moreover, current results could consolidate the attempts of many countries to stimulate economic growth and control growth volatility by taking advantage of the ICT revolution.
Current study could add to the literature on the nexus between ICT and economic growth volatility for several points. First, in addition to the use of the different proxy for the ICT in a separate model (for example, internet users, ICT goods import, and mobile subscriptions), we construct the ICT index from these components by the approach of PCA to catch a broad measure for ICT revolution. This could produce the consistency of findings regardless of ICT proxies included. Second, we calculate the interaction of average growth and its volatility by different non-overlapping intervals (i.e., 3-year and 5-year windows) with the inverse hyperbolic sine computation. This approach offers the appropriate comparison in a country’s growth variability with different average growth and produces the robustness of results regardless of the calculation method of non-overlapping intervals. Third, for a wider dataset covering economies including the emerging and developing, and developed countries, we apply the system two-step generalized method of moments (S-GMM) estimator and show the consistent findings through other estimation techniques such as fixed-effect estimation and quantile regression for the impact of ICT on economic growth volatility. Fourth, from the empirical evidence from a panel of comprehensive economies, we extend the understanding to the nexus between ICT and growth volatility by showing the favorable impact of ICT on obtaining high level of growth with lower growth volatility rather than the previous research finding focusing on the positive ICT impact on economic growth or output volatility separately. As a result, additional research on this topic is required and will contribute to ongoing debates.
From this introduction, the rest of our research is organized as follows. In Section 2, the review of related literature and theoretical background is provided. Data and methodologies are described in Section 3, followed by the empirical results and robustness test in Section 4. The final section aims to conclude the paper.
2. Theoretical background and literature review
2.1. Theoretical background
The rapid spread of ICT such as fixed-line telephones, mobile phones, the internet, and broadband has resulted in a dramatic change into an information society during the last several decades. ICT adoption has significantly increased the efficiency of resource allocation, considerably decreased production costs, and boosted demand and investment across all economic sectors (Grimes et al., Citation2012; Jorgenson & Stiroh, Citation1999; Lee et al., Citation2012; Pradhan et al., Citation2015; Vu, Citation2011). Therefore, it does not come as a surprise when ICT-led growth has received a great deal of attention from theoretical and empirical perspectives (Stanley et al., Citation2018).
Prior theories recognize that ICT plays an increasingly important role in accelerating economic growth. Prominent theories, such as neo-Schumpeterian (Schumpeter & Redvers, Citation1934) and neoclassical growth (Solow, Citation1956), have identified the implication of ICT for economic growth. According to these theories, ICT enters the economic supply in the form of capital and improves the production process by deepening capital and producing the technology and labor force with a high-quality advance. As a result, ICT generates added value at the business and sectoral levels, resulting in increased productivity and economic growth at the national level (Aghaei & Rezagholizadeh, Citation2017; Quah, Citation2002).
It is observed that there is an indirect impact of technologies on output fluctuations, which potentially creates research fundamentals for research on the driving impact of ICT diffusion on output volatility. King and Rebelo (Citation1999) show that the business fluctuations could be attributed to technology shocks. It is worth mentioning that the differences between communication and information technologies have gotten more blurred. For instance, cell phones are essentially communication devices. However, with the introduction of wireless apps, users now have access to data and information via their cellular phone. The Internet is primarily a barometer of information technology development (Baliamoune‐Lutz, Citation2003). To some extent, the output volatility is correlated to ICT-related technologies.
Macroeconomists tend to analyze the causes of fluctuations and the drivers of growth separately in recent decades. Recent theoretical and empirical work, however, has cast doubt on this conventional wisdom by finding that volatility might be associated with growth (Kroft & Lloyd-Ellis, Citation2002). However, the theoretical relationship between economic growth and volatility is inconclusive. Endogenous growth will be influenced by business cycle volatility, either negatively or positively, in the presence of irreversibility or diminishing returns to investment, or positively in the presence of prudent saving, innovative creative destruction, liquidity constraints, or if high-return technologies also entail high risks. Ramey and Ramey (Citation1995) demonstrate that nations with highly variable GDP increase at a slower rate, particularly in a sample of OECD countries with a smaller size. Jovanovic (Citation2006) demonstrates that pre-commitment to a hazardous technology produces a negative time series relationship between growth and volatility, but may indicate a positive cross-sectional relationship.
Given these theoretical points, the missing link between ICT-related factors and both aspects of economic activities such as economic growth and output volatility is observed from theoretical perspectives. To address this link effectively, it is critical to note that the growth and its volatility should be integrated into a comprehensive indicator to provide sufficient aspects of economic activity effectiveness. In addition to the ICT diffusion–growth relationship, this research addresses another possible influence of ICT penetration on a growth-related subject, namely, growth volatility. In other words, growth and its volatility need to be included in one single indicator to capture the comprehensive picture of the driving effect of ICT-related factors on the interaction between economic growth and its fluctuation. To the best of our knowledge, the link between ICT diffusion and the interaction between growth and its volatility has not been explicitly captured.
This gives rise to the research question: “Does ICT penetration have a significantly simultaneous impact on both aspects of economic growth, including the growth rate of gross domestic product and its volatility?”. Answering this critical research question could shed further light on the stimulating or deteriorating influence of ICT diffusion on the product of economic output and its fluctuations.
2.2. Related empirical research
Although literature predicts a positive relationship between ICT diffusion and economic growth, empirical studies have produced inconclusive findings. Well-established empirical evidence on the impact of ICT diffusion on economic growth shows that ICT is one of the primary factors for faster economic expansion. For example, ICT-achieved information can be communicated via electronic coding and virtual movement, influencing the development and technology of various businesses and thereby altering economic activity. However, there remains conflicting empirical evidence on the ICT–growth linkage. Some have provided positive impacts (Cheng et al., Citation2021; Crandall & Jackson, Citation2001; Jorgenson, Citation2001; Venturini, Citation2009; Vu, Citation2011; and among others), while others offered ambiguous or negative effects (Dewan & Kraemer, Citation2000; Hassan & Islam, Citation2005; Ishida, Citation2015; Lee et al., Citation2005; Pohjola, Citation2001; Yousefi, Citation2011), among others.
For the first strand of the literature on the link between growth and its volatility, Hardy (Citation1980) using data from 60 countries from 1968 to 1976 and Roller and Waverman (Citation2001) using data from 21 OECD countries from 1970 to 1990, and Madden and Savage (Citation1998) examining a sample of 27 Central and Eastern European countries from 1990 to 1995, among others, found a strong positive relationship between GDP and GDP per capita. It is observed that this research reflects the nexus between growth and its fluctuation, this gives rise to research capturing both aspects of growth, especially when considering the impact of ICT penetration on both economic growth and its volatility.
For the second strand of the literature on the influence of ICT on growth, ICT instruments such as fixed-line telephones on economic growth in industrialized countries were the focus of early cross-country research. Accordingly, recent research has verified that telecommunication technologies such as mobile phones, individual computers, and the usage of Internet have made a significant contribution to the economic growth of many countries, particularly industrialized ones (Gruber & Koutroumpis, Citation2010; Inklaar et al., Citation2005; Koutroumpis, Citation2009; Vu, Citation2011). Andrianaivo and Kpodar (Citation2011) confirmed that ICT contributed significantly to African countries’ economic growth between 1988 and 2007, using a variety of ICT measures such as mobile phone and fixed telephone adoption rates, as well as the cost of local calls.
The third strand of the literature on causal link between ICT and economic growth. Among earliest studies, Roller and Waverman (Citation2001) discovered a substantial positive causal association between investment in telecommunication infrastructure and subsequent economic success using data from 21 OECD nations over a 20-year period (1970–1990). According to the findings of GMM estimation, Vu (Citation2011) indicates that the penetration of personal computers, mobile phones, and internet users had a strong causal effect on economic growth. In addition, Pradhan et al. (Citation2018) employing a model of vector error correction (VECM) for a sample of G-20 countries covering the 2001–2012 period show the empirical evidence of Granger causality relationship among per capita economic growth, ICT infrastructure (the adoption of broadband or internet users), and other control variables such as inflation, labor force, and formation of gross domestic fixed capital. Another growing strand of the literature has pointed out the non-linear relation between ICT and economic growth. For example, Sassi and Goaied (Citation2013) show that the association between ICT import and growth is determined by the proportion of ICT imported in the Mena area. Accordingly, once a threshold of Internet users is achieved, an explosive growth appears.
However, ICT–economic growth and its volatility nexus is under-explored in the prior literature. To the best of knowledge, we find the work of Kim et al. (Citation2016) who examine the promoting and amplifying influence of trade openness on growth and growth volatility, respectively. In addition, Evrensel (Citation2010) indicates that corruption control and government effectiveness have a considerable negative impact on the average growth rate, but increased corruption control, expropriation risk management, government effectiveness, and government consumption reduce growth volatility. More recently, Da Silva et al. (Citation2017) investigate the role of finance on the interaction between growth and its volatility. However, this research differs from our study, which mostly focuses on the impact of ICT diffusion on both aspects of economic growth.
Due to the inexistence of a formal model, considering the various components of aggregate demand to understand how ICT diffusion affects output volatility might be a plausible point to utilize. First, from the standpoint of the labor force, apart from the increasing contributions of ICT services to growth, investments in the ICT sector have greatly increased labor productivity (Henry-Nickie et al., Citation2019). In addition, a higher labor directly decreases output growth rates but indirectly increases output growth rates by lowering its variance (Posch, Citation2011). To support this link, Tang (Citation2002) shows that the higher degree of development, calculated by the average labor productivity, has a tendency to experience less volatility of output growth.
Second, from the view of firms’ investment in digital transformation, the “creative destruction” thesis posits that ICT leads to more innovation (Basl & Doucek, Citation2012), which could strongly drive output volatility. To some extent, technical change has the ability to constantly modify itself, gradually expanding out and increasing production across all areas and industries. Third, from the viewpoint of financial intermediation, financial infrastructure driven by ICT diffusion could enhance the function of financial intermediation (Shamim, Citation2007). One should note that in the long run, financial intermediation boosts growth while decreasing volatility (Beck et al., Citation2014).
Fourth, from the perspective of the reduction of informational asymmetry, recent advances in information and communication technology (ICT) have changed the financial business, allowing for more efficient and innovative service delivery. Digital finance innovations assist vulnerable communities, particularly in developing economies, to access financial resources (Al-Nawayseh, Citation2020). This highlights the critical role of ICT on reducing asymmetric information for financial access and allocation efficiency (Asongu & Moulin, Citation2016), which possibly affects output volatility (Cavoli et al., Citation2020).
Finally, for the driving role of ICT on monetary policy, ICT-induced innovations could fuel the growth of mobile money such that it reaches levels where it could have implications for monetary policy (Weil et al., Citation2012). Ndirangu and Nyamongo (Citation2015) suggest that the rapid progress in financial innovations is also associated with an unstable and volatile money velocity and the money multiplier. In addition, Boivin and Giannoni (Citation2006) argue that an decrease in output volatility could be attributed to the monetary policy. Given these potential mechanisms, we expect that the ICT revolution could have a driving effect on economic growth, which extends the previous literature review. Above arguments and mechanisms shape our main hypothesis: “ICT penetration has stimulating and eliminating effects on growth and its volatility, respectively”.
3. Data and methodology
3.1. Data
We use a comprehensive country-level sample of 122 economies covering the period of 2000–2019. The list of countries is reported in of the Appendix. All variables (except inflation) are transformed by taking logarithmic form to eliminate heteroscedasticity (Sassi & Goaied, Citation2013). Sources for all variables are retrieved from World Indicator Development of World Bank. The countries with missing values of any variable are also excluded.
3.2. Measure for the interaction of growth and its volatility
Following Da Silva et al. (Citation2017), we integrate the average value of growth and its volatility in a single measure () to capture two growth dimensions such as an average economic growth (GDPPCG—the growth rate of gross domestic product per capita) and its fluctuation instead of employing a conventional approach as a standard deviation of economic growth. This measure could facilitate the comparison between economies with the different average value of growth. For a given country i, we calculate
where for the average value of GDP per capita growth and
for the standard deviation of GDP per capita growth. One should note that the traditional variation coefficients calculated as
could be inappropriate due to the possible zero or negative value causing an undetermined value of variation coefficients.
To allow the negative or zero value of , we take the inverse hyperbolic sine transformation of
to create
as measured by
The average growth and its volatility are computed based on the GDPPCG data for each country over a 3-year non-overlapping interval. In this approach, larger values of for a given country could signal that this country reaches higher levels of growth with lesser volatility. For the purpose of robustness test, we provide an alternative measure of
(i.e.,
) to capture the 5-year non-overlapping interval.
To illustrate the relevance of , Figure performs the evolution of this variable through time in several countries consisting of New Zealand, Tanzania, Australia, and China with the similar value of economic growth volatility (approximately 0.6 percent). However, their average growth rates differently range from 1.466 percent in New Zealand to 8.419 percent in China, resulting in
values of 2.786 and 5.463, respectively. It is worth noting that a high
indicates that the country is less susceptible to economic downturns; for example, China always enjoyed positive growth with relatively lower volatility during the period of 2000–2019. Therefore, this comprehensive measure calculated by the interaction between average growth and its fluctuation is relevant to consider simultaneously both aspects of economic growth.
3.3. Measure of ICT index by principal component analysis (PCA)
Following Pradhan et al. (Citation2014), to improve the narrow approach of previous research, we apply principal component analysis (PCA) to create a comprehensive measure by including mobile cellular subscriptions, internet users, and ICT good imports into a single ICT index. This index enters in a separate model to avoid collinearity and to make the comparison of findings when sequentially including other ICT variables into models. In panel A of in the Appendix, PC1 explains about 67.21% of the variation in the original data; hence, PC1 is selected to represent the ICT index. To confirm the relevance of PC1 proxy, we continuously employ a scoring threshold of 0.3 or higher as a determinant of factor score significance (Appiah‐Otoo & Song, Citation2020). In panel B, all scoring significance of mobile subscriptions, internet users, and ICT goods import qualify this threshold, showing the appropriate use of PC1.
3.4. Baseline model
To test the impact of ICT revolution on the product of growth and its volatility, we propose a model as follows:
where proxies consist of several components such as mobile cellular subscriptions per 100 inhabitants (MOBILE), imported ICT goods as a proportion of total imported goods (ICTGOOD) in which ICT goods imports include computers and peripheral equipment, communication equipment, consumer electronic equipment, electronic components, and other information and technology goods (miscellaneous), and individuals using the internet as a share of population and for ICT index (INTERNET).
Following the model of Da Silva et al. (Citation2017) capturing other control variables affecting the interaction of growth and its volatility, we employ as a constant GDP per capita;
stands for general government’s consumption expenditure divided by GDP;
is the duration of secondary education (years);
is the sum of exports and imports for goods and services as a share of GDP. In addition, we utilize
as the banks’ credit to the private sector as a proportion of GDP as proposed by Easterly et al. (Citation2000).
capture the lagged
for the nature of dynamic models. This lag variable is excluded in the fixed-effects model in the robustness test.
is an error term.
Definition of variables is reported in , while pairwise correlation matrix is illustrated in . For more details, all pairwise correlations among explanatory variables are lower than the threshold of 0.8, showing no concern of multicollinearity in the regression model. The pairwise correlation greater than 0.8 (for example, the value of 0.863 for association between internet and mobile) would enter a separate model to avoid spurious regression.
Table 1. Descriptive statistics
Table 2. Matrix of pairwise correlation
3.5. Estimation method
In this research, we first apply the two-step system generalized method of moments (S-GMM) to address the issue of fixed effects, the inclusion of the lagged dependent variable and heteroscedasticity contained in the model (Arellano & Bond, Citation1991). This approach is specifically appropriate for panels with large-N and small-T, which is widely employed in previous research on ICT penetration–economic growth (Appiah-Otoo & song, Citation2021; Cheng et al., Citation2021; Ghosh, Citation2016; Sassi & Goaied, Citation2013). In addition, S-GMM estimator is considered effective in addressing and addressing potential endogeneity, serial autocorrelation, and identification problems. In other words, this approach also captures the situation when independent variables are not strictly exogenous and might be associated with prior and current error disturbances, and where the well-known problems of autocorrelation, heteroskedasticity, and time fixed effects exist. For more details, S-GMM is employed to deal with joint endogeneity of several independent variables via a weighting matrix of internal instruments, which is responsible for serial association and heteroskedasticity.
To capture the potential bias attributed to reverse causality, we employed the lagged values (greater than 1-lag) of the estimators in the dynamic model of S-GMM as instruments. When analyzing changes in financial variables, the GMM method outperforms the classic OLS method (Cheng et al., Citation2021). It is worth noting that the GMM estimator technique can be employed in a one- or two-step approach (Arellano & Bond, Citation1991). When the assumptions of independence and homoscedasticity for the estimated parameters do not hold, a two-step estimator employs the residuals from the first step estimation to generate a weighted consistent variance–covariance matrix. We follow prior examples in the literature in which it was frequently recommended to use a two-step GMM estimator rather than a one-step GMM estimate (Albiman & Sulong, Citation2016; Bahrini & Qaffas, Citation2019; Wamboye et al., Citation2015).
Moreover, according to Koenker and Bassett (Citation1978), the regression parameter should be estimated for each quantile of the dependent variable. In addition, rather than evaluating the marginal influence of explanatory variables on the mean of the dependent variable, quantile regression assists in determining the marginal effect of explanatory variables across the distribution of the dependent variable. A conditional quantile regression of Y in terms of X’s at specific quantile taking the value of range (0,1) is a function
in which the parameter
is chosen to qualify the total minimum variance at a given quantile. The following formula is used to execute this concept:
For the study’s robustness test for the main link between ICT and the product of growth and its fluctuation, we estimate specification (3) with the approach of quantile regression to investigate the impact of ICT revolution on the distributional value of growth volatility. We provide findings in selected typical quantiles at a threshold of 0.1–0.9 with an interval of 0.1.
4. Empirical results
4.1. Results of baseline model
shows the estimation results of EquationEq. (3)(3)
(3) for a sample of 122 countries in the period 2000–2019 with the approach of S-GMM estimator. Columns (1)–(4) use ICT good imports, mobile, internet, PC1, respectively, as dependent variables. All lagged variables of
are statistically significant, confirming the dynamic nature of models. reports that ICT has a positive impact on growth volatility at 1 percent significance level, regardless of ICT proxies included. This indicates the favorable effect of ICT penetration on increasing growth and decreasing output volatility. Concerning the magnitude of coefficients, all other factors being equal, we can see that an increase in 1 percent of ICT goods imports could enhance 43.7 percent increase of growth volatility, while in the extremely low case, 1 percent increase in mobile subscriptions could increase 10.2 percent of growth volatility. For the ICT index, 24.2 percent increase in the interaction of growth and its volatility is as a result of the 1 percent increase in ICT combined index. Therefore, the magnitude impact of ICT on growth volatility varies with different proxies of ICT and imported ICT goods might be an impactful drivers of growth volatility rather than others in our study sample and period.
Table 3. ICT revolution and growth volatility estimated by the S-GMM technique (full sample)
Due to the lack of prior studies on the impact of ICT on both growth and its fluctuation, we provide several channels potentially explaining this link. Accordingly, these results could be attributed to several possible mechanisms through which the ICT penetration could have implications for economic growth and its movements such as the productivity-enhancing impact of labor force, firms’ investment in digital transformation, from the perspective of “creative destruction”, the reduction of informational asymmetry, the well-functioning financial intermediation, and monetary policy, as aforementioned previously. These channels highlight the penetration of ICT on economic activities and its impact on both aspects of growth in a manner that a mass diffusion of ICT could positively drive the growth while decreasing the economic growth fluctuation. In addition, we contribute to classical theories on growth-led ICT proposed by Schumpeter and Redvers (Citation1934) for neo-Schumpeterian and Solow (Citation1956) for neoclassical growth by showing both growth aspects (i.e, economic growth and growth volatility) evidently established by different application-related ICT including ICT goods import, mobile cellular subscriptions, and internet users. Given these statistically empirical findings, we show a potential justification for the conflicting results in previous research in which the positive and negative impact of ICT penetration is observed. That is, the proxy for growth could be insufficient to reflect its real characteristics; when employing the interaction of growth and its fluctuation in the current study, ICT shows a positive and negative influence on growth and growth volatility, respectively.
With respect to controlling variables, the initial gross domestic per capita, inflation, and banks’ credit to private sectors have a significantly negative impact on the product of growth and its volatility at 1 percent significance level. This implies that an increase in income per capita, inflation, and financial development through banks’ credit to the private sector could cause a decrease in growth and an increase in growth fluctuation. However, we observe the stimulating impact of trade measured by the volume of exports and imports divided by GDP in the product of growth and its volatility, which is not in line with Da Silva et al. (Citation2017). This finding suggests that higher openness in trade could create a favorable environment for an increase in economic growth and a decrease in growth volatility. In sum, the country’ average income, inflation, trade, and financial development are one of the significant contributors to growth volatility. In addition, government consumption and secondary education have no statistical impact on the product of growth and its volatility, which is inconsistent with the work of Da Silva et al. (Citation2017).
For the case of quantile panel regression, shows the results of the ICT impact on the value distribution of growth volatility though different quantile thresholds. Panels A–D employ the ICT good imports, mobile subscriptions, internet users, and ICT index, respectively, as dependent variables. For the purpose of brevity, we do not show the control variables but would provide upon request. We observe that internet users, ICT goods import, and the combined index of ICT have a similar and critical impact on most quantiles. The medium and highest quantiles of mobile subscriptions offer a different story in which the favorable impact of ICT penetration is confirmed. Therefore, the impact of ICT on the product of growth and its volatility is not driven by the large-scale economy and the extreme growth volatility of a given country.
Table 4. Quantile regression for baseline model (full sample)
4.2. Robustness test
We display the robustness test in several ways. First, we revisit the impact of ICT penetration on the product of growth and its volatility by controlling panel fixed effects. The results are quite similar to those reported previously, regardless of any ICT proxy integrated. Accordingly, in , all proxies of ICT diffusion such as mobile subscriptions, internet users, ICT goods import, and ICT combined index significantly contribute to an increase in growth together with a decrease in growth fluctuation. In addition, a stronger and significant impact is found when using ICT goods import as a dependent variable of interest.
Table 5. Robustness test by controlling fixed effects
Second, findings of the detailed examination using the approach of quantile panel regression in also appear consistently, confirming the positive impact of ICT on the interaction of growth and its fluctuation, irrespective of proxies of ICT revolution. It even holds true in the case of mobile cellular subscriptions per 100 individuals for all quantile thresholds. As a result, ICT penetration is indeed helpful for both increasing economic growth and decreasing growth volatility.
Table 6. Robustness test for alternative measures for growth volatility
Third, the ICT–growth volatility link is also tested across the distribution value of as an alternative measure for
by using a different non-overlapping pattern with respect to 5-year intervals. shows that the impact of ICT revolution on the economic growth fluctuation consistently appears in most quantiles. In other words, this positive effect is clear in low, medium, and high quantiles, confirming the stimulating and decreasing effect of ICT on growth and its volatility, respectively. To sum up, as verified across econometric approaches, ICT diffusion would perform the stimulating and attenuating role in growth and its volatility, respectively.
Table 7. Quantile regression with an alternative proxy for economic growth volatility
Fourth, the link between ICT diffusion and the product of growth and its fluctuation could be driven by the country’s heterogeneity due to the different level of resources and a degree of ICT penetration. To avoid this problem, we separate full sample into two sub-samples according to income classifications of the World Bank.Footnote3 As a result, we obtain 49 high-income countries (HICs) and 73 middle and low-income (MLI) countries. As reported in , findings remain unchanged compared to those reported previously. These are confirmed by significant levels for almost quantiles of a dependent variable when using panel quantile regression as noted in .The CPA-related ICT indexes for HIC and MLI sub-samples are reported in of the Appendix, respectively. Therefore, the stimulating and eliminating effects of ICT penetration on growth and its volatility hold true, irrespective of country’s income. This means that increasing growth and decreasing growth volatility could be driven by ICT applications such as mobile subscriptions, internet users, and ICT goods import. For comparison, Cheng et al. (Citation2021) show that the positive impact of ICT diffusion on growth is pronounced in developed countries; however, this impact is inconclusive in emerging and developing countries. We challenge this finding by indicating the favorable influence of ICT diffusion on growth-increasing and its fluctuation-decreasing, thereby offering consistent understanding on this link across countries with different nature.
Table 8. ICT revolution and growth volatility estimated by the S-GMM technique (HIC sample)
Table 9. Quantile regression for baseline model (HIC sample)
Table 10. ICT revolution and growth volatility estimated by the S-GMM technique (MLI sample)
Table 11. Quantile regression for baseline model (MLI sample)
5. Conclusion
Academic researchers and policymakers have concentrated on examining the impact of ICT on economic growth at the industrial, national, and cross-country levels in the context of the mass penetration of ICT partly reshaping the globe into the information society. Therefore, it does not come as a surprise that there is much research on ICT-lead growth; however, findings of empirical studies on this link have been equivocal, as compared with the theoretical perspectives of the positive impact of ICT on economic growth. That is, while a number of empirical studies have found that ICT diffusion improves economic growth, particularly in developed countries (Inklaar et al., Citation2005; Koutroumpis, Citation2009; Roller & Waverman, Citation2001), other studies have found that ICT diffusion has a negative impact on economic growth in many countries and regions of the world (Dewan & Kraemer, Citation2000; Pohjola, Citation2002). However, the existing measures for economic growth have limited the understanding of the impact of ICT on average value of growth. To overcome this weak point, we calculate the interaction of economic growth and its volatility following the approach of Da Silva et al. (Citation2017). One should note that this approach of the combined economic growth and its volatility remains scarce in empirical studies, and to the best of our understanding, there is no research employing this measure to examine the influence of ICT penetration on the product of economic growth and its fluctuation so far.
Using the S-GMM estimator addressing several econometric issues such as the inclusion of lagged dependent variable, heterogeneity, and autocorrelation of error term, the result indicates that there is statistically positive influence of ICT penetration on the interaction of growth and its fluctuation, regardless of ICT variables included. This result is consistent with the approach of quantile panel regression due to the similar impact of ICT on the value distribution of the interaction of growth and its volatility. Moreover, the relationship between growth and its volatility is robust when using an alternative measure of the product of growth and its volatility or the control of fixed effects. Due to the lack of previous empirical research on this topic, we provide potential mechanisms through which ICT revolution could have implications on growth and its volatility. There are several channels through which ICT penetration could shape the interaction between growth and its fluctuation. These mechanisms are related to the favorable impact of ICT penetration on labor productivity (Henry-Nickie et al., Citation2019), innovation (Basl & Doucek, Citation2012), reduction of informational asymmetry through digital finance innovations (Al-Nawayseh, Citation2020), and mobile money (Weil et al., Citation2012), and hence potentially affecting both growth and growth volatility.
The use of telecommunications services has increased at an unparalleled rate during the last two decades. The deregulation of wireless technologies and telecommunications markets is primarily responsible for this expansion. As King (Citation2012) argues, to greater extent, in the era of information revolution, mobile financial services enable enterprises to achieve wide access rights across several industries, including telecommunications, retail, and e-commerce, to provide bill payment and other financial services. This tendency will continue, fundamentally altering the regulations of traditional institutions and have implications for the country’s economic growth (Cheng et al., Citation2021). In this context, we observe the favorable impact of ICT diffusion on increasing growth and decreasing growth volatility. Therefore, policy-makers should strengthen and enhance ICT applications in the economy by simultaneously facilitating mobile, internet and ICT goods. In other words, the lack of ICT applications could make the entire economy worse for both growth aspects. Conducting these policy practices helps mitigate the adverse results of growth volatility and promote economic growth. Moreover, this action is beneficial both for high-income and low-income economies as evidently confirmed by the same effect of ICT on the product of growth and its fluctuation across countries with different incomes.
In regard to the contribution to the existing literature, we provide new empirical evidence on the favorable impact of ICT penetration in both stimulating the economic growth and decreasing the output volatility in a broader scenario of international cross-countries. From the lights of research findings, we draw attention to the positive effect of ICT diffusion on lower growth volatility and higher growth simultaneously. Therefore, countries could benefit from ICT development when they could reach an increase in economic growth and a decrease in growth volatility. Based on these results, we could improve on prior research by considering the growth fluctuation in addition to economic growth as an alternatively comprehensive measure of growth volatility, giving a rise to utilize this measure in the future research.
This research could not avoid limitations. First, despite our attempt to include related factors driving the economic growth and its fluctuation, there are other determinants possibly omitted (i.e., country’s corruption). It may be difficult to uncover direct evidence of ICT’s influence on growth and its volatility due to the existence of several confounding variables (Vu et al., Citation2020). Second, our theoretical approach could not directly address the nature of the link between ICT revolution and other growth volatility-related factors. One should note that this issue is also admitted in the work of Evrensel (Citation2010) who employs panel data from 121 countries to test the driving role of corruption on growth and growth volatility.
Given these weakness points, future research could add a comprehensive set of explanatory variables shaping the interaction between growth and its fluctuation. In addition, further research could exploit the measure for the product growth and growth volatility to revisit the impact of corruption or international trade openness on this indicator, which possibly sheds further light on other determinants of these newly emerging proxies for the product of growth and its fluctuation. One should note that when it comes to corruption as a potential determinant of the interaction of growth and its volatility, researchers could face the trade-off between narrowing the scale of sample to obtain sufficient observations at country’s level.
Acknowledgements
The authors are grateful for suggestions and constructive comments from two reviewers that greatly improve the quality of current research. We are responsible for remaining errors.
Disclosure statement
No potential conflict of interest was reported by the author(s).
Additional information
Funding
Notes on contributors
Thanh Phuc Nguyen
Thanh Phuc Nguyen is a Ph.D. Candidate in the School of Finance at the University of Economics Ho Chi Minh City, Vietnam (UEH). His research interests mainly revolve around topics related to banking and finance, risk management, and financial management.
Notes
1. For more example, Usman et al. (Citation2021) show the stimulating impact of ICT on economic growth in the long run, only for the case of India while there is no evidence for this link in other South Asian countries.
2. Fernández-Portillo et al. (Citation2020) also indicate that the majority of scientific research indicates that information and communication technologies (ICT) have a favorable effect on economic growth. In comparison, other studies indicate that this effect is minimal or even nil, implying that there are conflicting findings.
3. See this link for more details: https://datahelpdesk.worldbank.org/knowledgebase/articles/906519. One should note that from the original sample of 122 countries, we split the full sample into 49 high-income countries, 63 middle-income economies, and 10 low-income ones. Due to assuring the effectiveness of S-GMM with a sample with large-N and small-T, we combine low-income and middle-income countries into a larger sample, namely, Middle and Low-Income economies (MLI). This practice is similar to the classification in the work of Cheng et al. (Citation2021). We truly thank one anonymous reviewer for suggesting this classification.
References
- Adeleye, N., & Eboagu, C. (2019). Evaluation of ICT development and economic growth in Africa. NETNOMICS: Economic Research and Electronic Networking, 20(1), 31–27. https://doi.org/10.1007/s11066-019-09131-6
- Aghaei, M., & Rezagholizadeh, M. (2017). The impact of information and communication technology (ICT) on economic growth in the OIC Countries. Economic and Environmental Studies, 17(2 (42), 257–278. https://doi.org/10.25167/ees.2017.42.7
- Al-Nawayseh, M. K. (2020). Fintech in COVID-19 and beyond: What factors are affecting customers’ choice of FinTech applications? Journal of Open Innovation: Technology, Market, and Complexity, 6(4), 1–15. https://doi.org/10.3390/joitmc6040153
- Albiman, M. M., & Sulong, Z. (2016). The role of ICT use to the economic growth in Sub Saharan African region (SSA). Journal of Science and Technology Policy Management, 7(3), 306–329. https://doi.org/10.1108/JSTPM-06-2016-0010
- Andrianaivo, M., & Kpodar, K. R. (2011). ICT, financial inclusion, and growth: Evidence from African countries. IMF Working Papers, 2011(73), pp. 1-44. https://www.elibrary.imf.org/view/journals/001/2011/073/article-A001-en.xml).
- Appiah-Otoo, I., & Song, N. (2021). The impact of ICT on economic growth-Comparing rich and poor countries. Telecommunications Policy, 45(2), 102082. https://doi.org/10.1016/j.telpol.2020.102082
- Appiah‐Otoo, I., & Song, N. (2020). Finance‐growth nexus: New insight from Ghana. International Journal of Finance & Economics, pp. 1-42. https://doi.org/10.1002/ijfe.2294 .
- Arellano, M., & Bond, S. (1991). Some tests of specification for panel data: Monte Carlo evidence and an application to employment equations. The Review of Economic Studies, 58(2), 277–297. https://doi.org/10.2307/2297968
- Asongu, S. A., & Moulin, B. (2016). The role of ICT in reducing information asymmetry for financial access. Research in International Business and Finance, 38, 202–213. https://doi.org/10.1016/j.ribaf.2016.04.011
- Bahrini, R., & Qaffas, A. A. (2019). Impact of Information and communication technology on economic growth: Evidence from developing countries. Economies, 7(1), 21. https://doi.org/10.3390/economies7010021
- Balavac, M., & Pugh, G. (2016). The link between trade openness, export diversification, institutions and output volatility in transition countries. Economic Systems, 40(2), 273–287. https://doi.org/10.1016/j.ecosys.2016.02.001
- Baliamoune‐Lutz, M. (2003). An analysis of the determinants and effects of ICT diffusion in developing countries. Information Technology for Development, 10(3), 151–169. https://doi.org/10.1002/itdj.1590100303
- Basl, J., & Doucek, P. (2012). ICT and innovations in context of the sustainable development in Europe. Interdisciplinary Information Management Talks-IDIMT-2012: Vol. 20, Jindrichuv Hradec, Czech Republic.
- Beck, T., Degryse, H., & Kneer, C. (2014). Is more finance better? Disentangling intermediation and size effects of financial systems. Journal of Financial Stability, 10, 50–64. https://doi.org/10.1016/j.jfs.2013.03.005
- Bhuvana, M., Vasantha, S., & Bharath, R. (2016). Information and Communication Technology (ICT)–A drive for financial inclusion. Journal of Chemical and Pharmaceutical Sciences, 9(4), 1885–1889.
- Boivin, J., & Giannoni, M. P. (2006). Has monetary policy become more effective? The Review of Economics and Statistics, 88(3), 445–462. https://doi.org/10.1162/rest.88.3.445
- Cavoli, T., Gopalan, S., & Rajan, R. S. (2020). Does financial inclusion amplify output volatility in emerging and developing economies? Open Economies Review, 31(4), 901–930. https://doi.org/10.1007/s11079-019-09568-0
- Cheng, C.-Y., Chien, M.-S., & Lee, -C.-C. (2021). ICT diffusion, financial development, and economic growth: An international cross-country analysis. Economic Modelling, 94, 662–671. https://doi.org/10.1016/j.econmod.2020.02.008
- Comin, D. A., Gertler, M., & Santacreu, A. M. (). Technology innovation and diffusion as sources of output and asset price fluctuations. No. w15029. (National Bureau of Economic Research.) () () .
- Crandall, R. W., & Jackson, C. L. (2001). The $500 billion opportunity: The potential economic benefit of widespread diffusion of broadband Internet access. Criterion Economics.
- Da Silva, S. H., Tabak, B. M., Cajueiro, D. O., & Fazio, D. M. (2017). Economic growth, volatility and their interaction: What’s the role of finance? Economic Systems, 41(3), 433–444. https://doi.org/10.1016/j.ecosys.2016.10.008
- Dell’Ariccia, M. G., Detragiache, M. E., Mussa, M. M., & Eichengreen, M. B. J. (1998). Capital Account Liberalization: Theoretical and Practical Aspects. (International Monetary Fund)). .
- Dewan, S., & Kraemer, K. L. (2000). Information technology and productivity: Evidence from country-level data. Management Science, 46(4), 548–562. https://doi.org/10.1287/mnsc.46.4.548.12057
- Dynan, K. E., Elmendorf, D. W., & Sichel, D. E. (2006). Can financial innovation help to explain the reduced volatility of economic activity? Journal of Monetary Economics, 53(1), 123–150. https://doi.org/10.1016/j.jmoneco.2005.10.012
- Easterly, W., Islam, R., & Stiglitz, J. (2000). Explaining growth volatility. Annual World Bank Conference on Development Economics . The World Bank.
- Evrensel, A. Y. (2010). Corruption, growth, and growth volatility. International Review of Economics & Finance, 19(3), 501–514. https://doi.org/10.1016/j.iref.2009.08.002
- Fernández-Portillo, A., Almodóvar-González, M., & Hernández-Mogollón, R. (2020). Impact of ICT development on economic growth. A study of OECD European union countries. Technology in Society, 63, 101420. https://doi.org/10.1016/j.techsoc.2020.101420
- Ferraro, D. (2017). Volatility and slow technology diffusion. European Economic Review, 96, 18–37. https://doi.org/10.1016/j.euroecorev.2017.04.005
- Ghosh, S. (2016). Does mobile telephony spur growth? Evidence from Indian states. Telecommunications Policy, 40(10–11), 1020–1031. https://doi.org/10.1016/j.telpol.2016.05.009
- Gómez-Barroso, J. L., & Marbán-Flores, R. (2020). Telecommunications and economic development–The 20th century: The building of an evidence base. Telecommunications Policy, 44(2), 101904. https://doi.org/10.1016/j.telpol.2019.101904
- Grimes, A., Ren, C., & Stevens, P. (2012). The need for speed: Impacts of internet connectivity on firm productivity. Journal of Productivity Analysis, 37(2), 187–201. https://doi.org/10.1007/s11123-011-0237-z
- Gruber, H., & Koutroumpis, P. (2010). Mobile communications: Diffusion facts and prospects. Communications and Strategies 1 , (77), 133–145.
- Hakura, D. S. (2009). Output volatility in emerging market and developing countries: What explains the “great moderation” of 1970-2003? Czech Journal of Economics and Finance (Finance a Uver), 59(3), 229–254 https://ideas.repec.org/a/fau/fauart/v59y2009i3p229-255.html.
- Hardy, A. P. (1980). The role of the telephone in economic development. Telecommunications Policy, 4(4), 278–286. https://doi.org/10.1016/0308-5961(80)90044-0
- Hassan, A. K., & Islam, M. R. (2005). Temporal causality and dynamics of financial development, trade openness, and economic growth in Vector Auto Regression (VAR) for Bangladesh, 1974-2003: Implication for poverty reduction. Journal of Nepalese Business Studies, 2(1), 1–12. https://doi.org/10.3126/jnbs.v2i1.50
- Henry-Nickie, M., Frimpong, K., & Sun, H. (2019). Trends in the information technology sector. The Brookings Institute. https://www.brookings.edu
- Imbs, J. (2007). Growth and volatility. Journal of Monetary Economics, 54(7), 1848–1862. https://doi.org/10.1016/j.jmoneco.2006.08.001
- Inklaar, R., O’Mahony, M., & Timmer, M. (2005). ICT and Europe’s productivity performance: Industry‐level growth account comparisons with the United States. Review of Income and Wealth, 51(4), 505–536. https://doi.org/10.1111/j.1475-4991.2005.00166.x
- Iseringhausen, M., & Vierke, H. (2018). What drives output volatility? the role of demographics and government size revisited. European Economy Discussion Papers.
- Ishida, H. (2015). The effect of ICT development on economic growth and energy consumption in Japan. Telematics and Informatics, 32(1), 79–88. https://doi.org/10.1016/j.tele.2014.04.003
- Jorgenson, D. W. (2001). Information technology and the US economy. American Economic Review, 91(1), 1–32. https://doi.org/10.1257/aer.91.1.1
- Jorgenson, D. W., & Stiroh, K. J. (1999). Information technology and growth. American Economic Review, 89(2), 109–115. https://doi.org/10.1257/aer.89.2.109
- Jovanovic, B. (2006). Asymmetric cycles. The Review of Economic Studies, 73(1), 145–162. https://doi.org/10.1111/j.1467-937X.2006.00372.x
- Kim, D.-H., Lin, S.-C., & Suen, Y.-B. (2016). Trade, growth and growth volatility: New panel evidence. International Review of Economics & Finance, 45, 384–399. https://doi.org/10.1016/j.iref.2016.07.006
- King, B. (2012). Bank 3.0: Why banking is no longer somewhere you go but something you do. John Wiley & Sons.
- King, R. G., & Rebelo, S. T. (1999). Resuscitating real business cycles. Handbook of Macroeconomics, 1, 927–1007 https://doi.org/10.1016/S1574-0048(99)10022-3.
- Ko, K. W. (2008). Financial integration, information and communication technology, and macroeconomic volatility: Evidence from ten Asian economies. Research in International Business and Finance, 22(2), 124–144. https://doi.org/10.1016/j.ribaf.2007.01.001
- Koenker, R., & Bassett, G., Jr. (1978). Regression quantiles. Econometrica: Journal of the Econometric Society, 46(1), 33–50. https://doi.org/10.2307/1913643
- Kose, M. A., Prasad, E. S., & Terrones, M. E. (2006). How do trade and financial integration affect the relationship between growth and volatility? Journal of International Economics, 69(1), 176–202. https://doi.org/10.1016/j.jinteco.2005.05.009
- Koutroumpis, P. (2009). The economic impact of broadband on growth: A simultaneous approach. Telecommunications Policy, 33(9), 471–485. https://doi.org/10.1016/j.telpol.2009.07.004
- Kroft, K., & Lloyd-Ellis, H. (2002). Further cross-country evidence on the link between growth, volatility and business cycles. Queens University, Department of Economic, Working Paper.
- Lee, S.-Y. T., Gholami, R., & Tong, T. Y. (2005). Time series analysis in the assessment of ICT impact at the aggregate level–lessons and implications for the new economy. Information & Management, 42(7), 1009–1022. https://doi.org/10.1016/j.im.2004.11.005
- Lee, S. H., Levendis, J., & Gutierrez, L. (2012). Telecommunications and economic growth: An empirical analysis of sub-Saharan Africa. Applied Economics, 44(4), 461–469. https://doi.org/10.1080/00036846.2010.508730
- Levchenko, A. A., & Di Giovanni, J. (2008 Trade openness and volatility). IMF Working Paper WP/08/146 ().
- Madden, G., & Savage, S. J. (1998). CEE telecommunications investment and economic growth. Information Economics and Policy, 10(2), 173–195. https://doi.org/10.1016/S0167-6245(97)00020-6
- Malik, A., & Temple, J. R. (2009). The geography of output volatility. Journal of Development Economics, 90(2), 163–178. https://doi.org/10.1016/j.jdeveco.2008.10.003
- Mushtaq, R., & Bruneau, C. (2019). Microfinance, financial inclusion and ICT: Implications for poverty and inequality. Technology in Society, 59, 101154. https://doi.org/10.1016/j.techsoc.2019.101154
- Nasab, E. H., & Aghaei, M. (2009). The effect of ICT on economic growth: Further evidence. International Bulletin of Business Administration, 5(2), 46–56.
- Ndirangu, L., & Nyamongo, E. M. (2015). Financial innovations and their implications for monetary policy in Kenya. Journal of African Economies, 24(suppl_1), i46–i71. https://doi.org/10.1093/jae/eju029
- Niebel, T. (2018). ICT and economic growth – Comparing developing, emerging and developed countries. World Development, 104, 197–211. https://doi.org/10.1016/j.worlddev.2017.11.024
- Pohjola, M. (2001). . Information Technology, Productivity and Economic Growth: International Evidence and Implications for Economic Growth (Oxford University Press), 1–32.
- Pohjola, M. (2002). The new economy: Facts, impacts and policies. Information Economics and Policy, 14(2), 133–144. https://doi.org/10.1016/S0167-6245(01)00063-4
- Posch, O. (2011). Explaining output volatility: The case of taxation. Journal of Public Economics, 95(11), 1589–1606. https://doi.org/10.1016/j.jpubeco.2011.05.009
- Pradhan, R. P., Arvin, M. B., Hall, J. H., & Bahmani, S. (2014). Causal nexus between economic growth, banking sector development, stock market development, and other macroeconomic variables: The case of ASEAN countries. Review of Financial Economics, 23(4), 155–173. https://doi.org/10.1016/j.rfe.2014.07.002
- Pradhan, R. P., Arvin, M. B., & Norman, N. R. (2015). The dynamics of information and communications technologies infrastructure, economic growth, and financial development: Evidence from Asian countries. Technology in Society, 42, 135–149. https://doi.org/10.1016/j.techsoc.2015.04.002
- Pradhan, R. P., Mallik, G., & Bagchi, T. P. (2018). Information communication technology (ICT) infrastructure and economic growth: A causality evinced by cross-country panel data. IIMB Management Review, 30(1), 91–103. https://doi.org/10.1016/j.iimb.2018.01.001
- Quah, D. (2002). Technology dissemination and economic growth: Some lessons for the new economy Technology and the new economy (Cambridge, MA: MIT Press) .
- Ramey, G., & Ramey, V. A. (1995). Cross-country evidence on the link between volatility and growth. The American Economic Review 85 5 . 1138–1151 https://www.nber.org/papers/w4959.
- Roller, L.-H., & Waverman, L. (2001). Telecommunications infrastructure and economic development: A simultaneous approach. American Economic Review, 91(4), 909–923. https://doi.org/10.1257/aer.91.4.909
- Sassi, S., & Goaied, M. (2013). Financial development, ICT diffusion and economic growth: Lessons from MENA region. Telecommunications Policy, 37(4–5), 252–261. https://doi.org/10.1016/j.telpol.2012.12.004
- Schumpeter, J. A., & Redvers, O. (1934). The Theory of Economic Development. An Inquiry into Profits, Capital, Credit, Interest, and the Business Cycle Harvard University Press (Cambridge, MA) .
- Shamim, F. (2007). The ICT environment, financial sector and economic growth: A cross‐country analysis. Journal of Economic Studies, 34(4), 352–370. https://doi.org/10.1108/01443580710817452
- Solow, R. M. (1956). A contribution to the theory of economic growth. The Quarterly Journal of Economics, 70(1), 65–94. https://doi.org/10.2307/1884513
- Stanley, T. D., Doucouliagos, H., & Steel, P. (2018). Does ICT generate economic growth? A meta‐regression analysis. Journal of Economic Surveys, 32(3), 705–726. https://doi.org/10.1111/joes.12211
- Tang, S. H. K. (2002). The link between growth volatility and technical progress: Cross-country evidence. Economics Letters, 77(3), 335–341. https://doi.org/10.1016/S0165-1765(02)00145-3
- Usman, A., Ozturk, I., Hassan, A., Zafar, S. M., & Ullah, S. (2021). The effect of ICT on energy consumption and economic growth in South Asian economies: An empirical analysis. Telematics and Informatics, 58, 101537. https://doi.org/10.1016/j.tele.2020.101537
- Venturini, F. (2009). The long-run impact of ICT. Empirical Economics, 37(3), 497–515. https://doi.org/10.1007/s00181-008-0243-9
- Vu, K. M. (2011). ICT as a source of economic growth in the information age: Empirical evidence from the 1996–2005 period. Telecommunications Policy, 35(4), 357–372. https://doi.org/10.1016/j.telpol.2011.02.008
- Vu, K., Hanafizadeh, P., & Bohlin, E. (2020). ICT as a driver of economic growth: A survey of the literature and directions for future research. Telecommunications Policy, 44(2), 101922. https://doi.org/10.1016/j.telpol.2020.101922
- Wamboye, E., Tochkov, K., & Sergi, B. S. (2015). Technology adoption and growth in sub-Saharan African countries. Comparative Economic Studies, 57(1), 136–167. https://doi.org/10.1057/ces.2014.38
- Weil, D., Mbiti, I., & Mwega, F. (2012). The implications of innovations in the financial sector on the conduct of monetary policy in East Africa. Working Paper 12/0460 (International Growth Centre).
- Yousefi, A. (2011). The impact of information and communication technology on economic growth: Evidence from developed and developing countries. Economics of Innovation and New Technology, 20(6), 581–596. https://doi.org/10.1080/10438599.2010.544470
- Zimmermann, C. (1994). Technology innovations and the volatility of output: An international perspective Working Paper No. 34 (CREFE) .
Appendix
Table A1. List of countries
Table A2. Principal component analysis for ICT index construction (full sample)
Table A3. Principal component analysis for ICT index construction (HIC sample)
Table A4. Principal component analysis for ICT index construction (MLI sample)