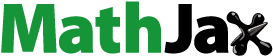
Abstract
To analyze day trading dynamics for Nifty Index futures and options contracts, a detailed study is steered to understand the quantum of volume traded and how volume traded affects the underlying volatility. Day trades are about 30% and 46% of the total trades for futures and options contracts, respectively. This signifies high volatility. Volume traded by individuals is bulk compared to other categories for both intraday and non-day trades. This study estimates the volatility volume dynamics. Volatility is assessed by the minimum-variance unbiased estimator. This method, independent of the drift and opening jumps, provides estimates of the least variance for more accuracy. Volume is segmented into a number of trades and average trade size. To understand the effect of volume, trade size and inventory on volatility, we use the logit regression function. For non-day Nifty Index futures contracts, low volumes are traded as opposed to high volumes for day trades, suggesting high speculative activity. For options contracts, the volume volatility estimates although significant are weak compared to futures contracts.
1. Introduction
Efficient price signals help investors make informed investment decisions, and an efficient price discovery process increases informational flow in the market. Derivative instruments enhance informational efficiency of the underling’s markets as these products improve the price discovery process and provide increased informational flow in the market.
Market liquidity, an important factor that affects market efficiency, is primarily determined by the effectiveness and efficiency of the market’s price discovery function. Financial markets with a high volume traded provide more liquidity for market participants than thinner markets with few available securities and participants and thus limited trading opportunities. The heterogeneity of market participants in terms of transaction needs, risk appetites, and investment horizons can enhance market liquidity. Bearing in mind these factors, this study is an attempt to understand trading dynamics for Nifty Index futures and options contracts traded on the National Stock Exchange (NSE) of India.
To understand trading dynamics, the growth and prominence of derivative trading is studied. Intra-day trades are also analyzed for significant relationships. The popularity of day trading stems out of the ability of the day trader to take advantage and make profits of market inefficiencies as well as market volatility. The Securities and Exchange Board of India (SEBI), the regulator of the Indian Securities market, has not provided any definition for day trading through any of its circulars, rules or regulations. In India, day trading is allowed for retail investors in both the cash and derivative segment. On the other hand, institutional investors day trade only in derivative contracts.
The derivative segment in India has grown by leaps and bounds within a span of 16 years, positioning India among the top five derivative markets in the world. Compared to cash markets, the volumes in the derivative markets have been enormous. Since 2008–09, index options have become the dominant product traded. The percentage turnover in Index options was 75.0 percent of total turnover in the equity derivative segment in 2015–16 The share of single stock futures has decreased to 12.4 percent from 57.7 percent in the same period. This trend continued until the present time in 2021. Index options continue to dominate, and a surge is witnessed in 2019–20 with the premium turnover almost doubling in 20−21 as compared to the previous year for Index as well as stock options. Table provides details about the shifting trading pattern in equity derivative contracts.
Table 1. Shifting trading pattern in the Indian equity derivative contracts
The primary reason for the sharp rise in option trading could be attributed to the securities transaction tax (STT). The Securities Transaction Tax (STT) rate is the highest for cash deliverables. This tax differential between the cash and the derivatives segment has resulted in the rapid growth in the derivative segment, especially option turnover in the Indian stock market. Options contracts have outpaced growth in the futures contracts. Before 2008, for unexercised options (options that were squared off), the tax was levied on the aggregate of the notional value of the transaction and the premium. After the revision in June 2008, the tax was levied only on the premium value, that is, for the seller. The buyer is required to pay a tax on the settlement price only if the option is exercised, which forms a negligible percentage of total options in the Indian stock market. The current scenario in Indian derivatives trading is at par with international markets where options are preferred to futures contracts. Higher outlay, lower liquidity and greater risk in single stock futures could be the reasons behind this shift.
This study analyses the market dynamics for trading Nifty Index futures and options contracts. In order to get a preview of the market microstructure, we begin with the introduction and the review of literature. Section 3 deals with data description and preliminary investigation to understand the quantum of volume traded by different market players. Section 4 provides the methodology, that is, volatility and volume estimate, and establishes a relation between the volume and volatility using the logit regression. Section 5 provides the results and discussion. Section 6 concludes.
2. Review of literature
Theoretical considerations have documented that day trading activity is sentiment-driven and affects the underlying market volatility. In the western context, the query examining the volume and increased volatility has shown mixed results. Schwert (Citation1989) and Tauchen et al. (Citation1992) document a positive relation between volume and volatility in mature markets. Schwert (Citation1990) states that stock market volatility was drastic before and after the crash of 1987. However, the return to normal levels was more quick than past experiences. According to the findings of Robbani and Bhuyan (Citation2005), conditional volatility of intraday (high frequency) returns in posterior derivative periods enhanced volatility of the Dow Jones Industrial Average (DJIA), a consensus on increased liquidity, i.e. increased trading volume. Information processing on account of derivative (option) listing has been documented by Skinner (Citation1989). However, some studies reveal that introduction of derivatives has produced no significant alterations in the conditional volatility of component stocks (Rahman, Citation2001). Malik (Citation2014) reported that the imposition of securities transaction tax (STT) and its subsequent revisions showed a mixed response of volatility and volume to changes in STT.
In order to examine the impact of trades (i.e. selling activities) on the asymmetric volatility relation, Kittiakarasakun et al. (Citation2012) have employed the Computer Trade Reconstruction (CTR) data of NASDAQ-100 index futures distinguishing informed trades and uninformed trades. They find that the selling impact of informed trades on the asymmetric volatility is at most weak. In addition, small-size trades have a greater impact on the asymmetric volatility relation than large-size trades. Results suggest that selling activity of uninformed traders can significantly influence asset return and volatility. Moreover, there is a common consensus that the magnitude of intraday variation is higher in the Indian capital markets compared to other developed and developing markets. According to Agarwalla and Pandey (Citation2012), in other markets (Nikkei-225 and S & P 500), the volatility at intraday peak is around twice the volatility at intraday low; in India, it is almost four times. The intraday five-minute absolute returns range from 0.05% to 0.11% in the case of Nikkei-225. They report high intraday variation in the case of large cap stocks relative to small cap stocks. Higher volatility is also observed in the first one hour of trade after weekends, in the first half-an-hour after the holidays and in the last one hour of trade before the weekends. Temporary scheduled trading halts cause the volatility to rise when the market reopens. Interesting findings about the Indian derivative markets have also been given by Srivastava et al. (Citation2008) They state that the participation of institutional investors in the Indian stock derivative market is extremely limited. It can be attributed to the regulatory restrictions wherein such investors are allowed to use derivative securities mainly for hedging and arbitrage purposes only. Varma Jayanth (Citation2000) provides evidence of the violation of the put-call parity in the Indian markets and shows that short sale in the cash market impedes arbitrage between the cash and derivative segment and overpricing of deep in the money calls.
Similar to the Indian markets are the Chinese and Korean markets that reveal interesting findings. Chou et al. (Citation2015) state that day traders are irrational contrarian traders more conspicuously making losses, although they provide market liquidity by reducing the bid-ask spread, temporary price volatility and the temporal price impacts. Ryu (Citation2012) who analysed day trading on the KOSPI 200 futures market reported that domestic individual traders incur substantial losses by implementing the day trading strategy and their losses clearly increase with their trading frequency and volume. By contrast, foreign institutions, whose day trading explains only a small portion of total day trading activity, make substantial profits from day trading. Frederick et al. (Citation2005), however, present a different view regarding the Chinese markets. They feel that traders manipulate chips by splitting one large trade into many small trades to camouflage trading activities. Such strategic behaviour explains the positive relation between trade frequency and price movement. Theoretical considerations have shown that the Indian markets share characteristics similar to those of other developing markets like the Chinese markets. Another interesting finding on the transactions volume and volatility relation is presented by Jones et al. (2014). They opine that volatility is generated by the number of transactions, and the information provided by the trade size is restricted to the frequency of transactions.
3. Data description and preliminary investigation
3.1. Description of data analyzed
Four categories of traders were identified who traded Nifty Index futures and Nifty Index option. Data have been taken from the NSE Website. They included
Individuals,
Public and private companies/bodies corporate,
Foreign Institutional Investors (FIIs) and
Others, namely, partnership firms, Hindu Undivided Families, Mutual Funds and Trusts, among others.
The data span was 1 January 2006 to 31 December 2020 and included comprehensive details on all transactions undertaken for trading Nifty Index Futures and Nifty Index Option contracts during the above-mentioned period.
The transaction data included the date of the transaction, the direction (buy or sell) of the transaction, the number of contracts traded and transaction price, and volatility for different traders was analyzed. Data were analyzed from the perspective of day as well as non-day trades.
In the present study, day traders are defined as those who buy and sell the same quantity of futures/option contracts and carry no inventory overnight.
3.2. Preliminary investigation
The data for the average daily number of contracts traded and number of trades are presented in Table for the Nifty Index futures contracts and Table , respectively, for the Nifty Index options contracts.
Table 2. Daily number of contracts traded for day trades and all trades by trader type
Table 3. Daily number of trades for day trades and all trades by trader type
Table 4. Daily number of contracts traded for day trades and all trades by trader type for options contracts
Table 5. Number of trades for day trades and all trades by trader type for Nifty Index Options Contracts
Table 6. Correlation between volume traded (V), number of trades (T) and average trade size (AV)
3.3. Data analysis for index futures contracts
Table reports the average daily number of contracts traded for day trades as well as all trades by different traders bifurcated as Individuals, Public and Private Companies/Bodies Corporate, Foreign Institutional Investors and others. An account is defined as a “day trader” account if the number of contracts purchased and sold on a particular day is the same.
Table shows the average daily number of trades for the different categories of traders.
3.3.1. Data analysis for index futures contracts
For Index futures contracts, the day traded volume comprises about 30% of the total volume traded. Individuals trade about 50% of the total day trading volume followed by Public & Private Companies/Bodies Corporate (40%), and the fourth category, i.e., other participants, trades about 10% of the total volume in the day traded Nifty Index futures contracts.
For all trades, i.e. day trades as well as non-day trades, for Nifty Index futures contracts, Public & Private Companies/Bodies Corporate trades a bulk of about 47% of the volume followed by individuals 31% and FIIs 14%, and the other participants trade a negligible 8% of the total volume.
The number of trades traded by the individuals is bulk compared to other categories for both day and non-day trades.
3.3.2. Index options contracts
Table reports the average daily number of contracts traded for day trades as well as all trades by different traders. PE denotes Put Options European Style, and CE denotes Call Option European Style.
Table reports the average daily number of trades for day trades as well as all trades by different traders. PE denotes Put Options European Style, and CE denotes Call Option European Style.
Public & Private Companies/Bodies Corporate trades the maximum volumes, i.e. about 67% in Put Options and call options contracts in relation to total volume traded. They actively trade in both day and non-day call as well as put option contracts. The individual traders are active in the put/call options day as well as non-day trades where they trade about 20% of the total volume of Nifty Index options contracts.
FIIs trade conspicuously in non-day options contracts. Their participation is about 9% of the total Call option volume and about 11% of the total non-day put option volume. Also, their participation is negligible in day trading Nifty Index option contracts for the study period.
The fourth category, which comprises other participants, trades 10%—12% in the put/call option day trading contracts and about 7%—8% in the non-day put/call index option contracts. Data in Table reveal that the number of trades entered into is highest for individuals. However, the institutional investors trade larger volumes although the number of trades entered into is low compared to individuals.
Hence, the number of trades along with the volume traded (i.e. No. of contracts traded) is studied in relation to volatility to understand the volume volatility dynamics for different categories of traders who trade on the NSE in the Nifty Index Futures and Nifty Index Option contracts.
4. Methodology
The two facets, volatility and volume defined in the study, are as follows:
4.1 Estimating volatility
Price volatility is calculated as drift-independent volatility, given a historical contract-wise price volume data set containing n (n > 1) from periods of open, high, low and close (OHLC) prices. The new estimator documented by Yang and Zhang (Citation2000) is the minimum-variance unbiased variance estimator (the smaller the variance, the more accurate is the estimation), which is independent of both the drift and opening jumps of the underlying price movement. This technique has been applied in the study to calculate the variance, i.e. the underlying volatility in the Nifty Index futures and Nifty Index options contracts.
The equation is given by
where V is the uUnknown variance, which is the unknown volatility squared (); k is the constant to minimize the variance of the estimator V and VRs is given by Equationequation (i
(3i)
(3i) ), Vo by equation (ii) and Vc by Equationequation (iii
(iii)
(iii) ),
where u = Ln H1—Ln O1, in which
H1 is the current period’s high during the trading interval and O1 is the opening price of the current period; c is ln C1—ln O1, the normalized close, in which C1 is the closing price of the current period (at time 1) and O1 is the opening price of the current period; d is ln L1—ln O1, the normalized low, in which L1 is the current period’s low during the trading interval and O1 is the opening price of the current period;
where o is ln O1—ln C0, the normalized open, in which O1 is the opening price of the current period and C0 is the closing price of the previous period;
with and
Hence, the result given by “V” in Equationequation 1(1A)
(1A) was used to calculate volatility (return) from the OHLC prices for Nifty Index Futures contracts as well as Nifty Index Option contracts traded on NSE.
4.1. Estimation of volume
Volume (V), i.e. number of contracts traded, is segmented into
number of trades (T)
average trade size = volume/number of trades, i.e. (AV = V/T).
Volume is split up into two components: number of trades (T) and average trade size (AV), where AV is calculated by dividing the volume by number of trades. Similar to volume, T and AV increase as the turnover increases.
4.2. Correlation between volume, number of trades and average trade size 1
The correlation between V, T and AV provides interesting results:
The daily number of trades (frequency of trading) is highly positively correlated with the volume for day/non-day trades for futures contracts (0.91/0.96). Hence, the number of trades is a powerful indicator providing information about the volume for Nifty index futures.
The correlation between AV and V is highly positively correlated for the call options (CE) and the put options (PE). Hence, for options contracts, the average trade size is a strong indicator of volume dynamics
4.2. Effect of volume traded on volatility
Estimation of High and Low Volatility: In this section, we analyze the volatility volume relation using the Logit Regression Model. The Y variable used in the logit regression would be the probability of volatility being high or low. This probability could take values 1 (y) for high volatility and 0 for low volatility (1-y). The limited range of this probability would present problems if used directly in a regression, so the odds, Y/(1-Y), are used instead. If the probability of high volatility is 0.25, the odds of having high volatility are 0.25/(1–0.25) = 1/3. This would be expressed as “3 to 1 odds against having high volatility.” Taking the natural log of the odds makes the variable suitable for a regression.
A best fit logit regression is estimated using the maximum-likelihood method that finds the best fit for the parameters.
For this purpose, volatility is split up into high- and low-volatility periods for the logit binary regression coefficient, 1 if volatility is high and 0 if volatility is low.
4.3.1 Determining high and low volatility
In order to determine whether volatility was high or low, the Markov state dependency developed by Aldridge (Citation2014) was used. The Markov technique divided volatility into high- and low-volatility states and then assessed the probability of transition from high to low probability and vice versa. Specifically, the technique followed is as follows:
A linear regression was estimated on the return series.
After examination of the distribution of the error terms, they were divided into two separate groups of high and low errors based on an arbitrary yet appropriate cut-off point.
Next, the estimate historical transitional probabilities based on the sequential changes from high to low or vice versa were determined.
From each sequential error observation, it was determined whether the error was a change from low to high or high to low, a state in the low-volatility state or high-volatility state.
The totals were counted and expressed in a percentage probability form.
The Index futures and the Index option contracts are separately analyzed for this purpose.
The regression equation is given by
where is the volatility (return) for Index futures contracts,
where is the volatility for the Index option contracts, Volume denotes the number of contracts traded and Trade Size is the number of trades entered into by the participants.
Inventory is the absolute value of the average daily net closing position, that is, the difference between what a trader purchases and sells on a particular day.
5. Results and discussion
The volume traded during periods of high and low volatility for intraday and non-day trades is estimated using the logit regression. provides the estimates.
Table 7. Estimation of regression coefficients of volume trade size and inventory on volatility
reports the results of logit regression of volatility (1 for high volatility, 0 for low volatility) on volume, trade size and Inventory (Equation 3(i) for index futures and equation 3 (ii) for index option contracts). This analysis was carried out on data for volume, trade size and Inventory for intraday/non-day trades. Table values represent estimated standardized coefficients. T values are reported in parentheses below each coefficient.
Nifty Index Futures (Non-Day): Volume/Trade Size: (negative coefficient for non-day trades and futures contracts) This implies that traders who trade larger quantities with a large average trade size are less likely to trade during periods of high volatility for non-day Nifty Index futures contracts.
Nifty Index Futures (Day Trades): Volume/Trade Size: However, a reverse situation is seen for day trades. The volume coefficient for day trades is positive, implying that during periods of high volatility, more trade is likely to take place. Moreover, for day traded Index futures contracts, trade size is highly positively correlated with volume (0.91 as specified in ). This could imply that day traders tend to enter into a large number of trades to gain from miniscule price fluctuations during the day.
Index Options: For day traded Index options, the coefficients for volume are statistically significant but week. Trade size and Inventory are not statistically significant.
Hence, futures contracts reveal a stronger volume-volatility relation compared to options contracts. Investments in futures contracts are made over a longer time horizon for the purpose of hedging or to tide against inflation. For option contracts, volatility volume dynamics is not as pronounced probably due to the nature of the contract, STT levied, leverage and the time decay factor.
6. Conclusion
This work provides an analysis of volume and volatility estimate for futures and options contracts on Nifty Index. Volume dynamics suggest that the frequency of trades for individuals is large (94.13%—frequency/Number of futures contracts traded; 91.14%—frequency/number of options contracts traded). By volume, individuals trade 50% on average compared to others. Hence, the number of trades entered into by retail investors is large, especially when the market gains momentum as they speculate to benefit from meager fluctuations in price changes. Volatility modelled using the minimum variance unbiased estimator allows for the estimation of minimum variance. High- and low-volatility periods were differentiated using the Markov state dependency developed by Aldridge. The logit regression model used to trace the effect of the volume traded on volatility suggested that day trading is pronounced especially during periods of high volatility. Another observation stated that large non-day trades for Nifty Index futures contracts were less likely to take place during periods of high volatility, and preliminary investigation suggests that public and private companies trade approximately 46% by volume of intraday and non-day traded contracts. This suggests that large trades are undertaken for the purpose of hedge. Furthermore, in the Indian context, institutional investors are not allowed intraday-trade in the cash segment. Hence, only retail traders leverage from positions simultaneously taken in the cash and derivative segments.
Based on the above findings, we recommended that excessive trading should be closely monitored as only brokerage and asset management firms who typically lure unsophisticated traders enjoy substantial profits by imposing brokerage and transaction fees for the use of their trading system. Furthermore, day/non-day trading norms are stringent in the US and other advanced countries. Although SEBI may not follow them in toto, registration norms, specifically for day traders, should be made stringent by prescribing qualifications, eligibility criteria and predetermined norms of financial credibility. Adequate disclosure, reporting requirements and margin rules should be made mandatory to check unanticipated speculation.
As volume traded to a certain extent reflects investor sentiment, further study would be worthwhile to examine the possible links between psychological bias such as disposition effect or investors’ overconfidence and its effect on the underlying volatility in the market.
Disclosure statement
No potential conflict of interest was reported by the author(s).
Additional information
Funding
References
- Agarwalla, S. K., & Pandey, A. (2012). Whether Cross-Listing, Stock-Specifi c and Market-Wide Calendar Events Impact Intraday Volatility Dynamics? Evidence from the Indian stock market using high-frequency data. No. 2012-11-03. Indian Institute of Management Ahmedabad W.P.
- Aldridge, I. (2014). High-frequency runs and flash-crash predictability. The Journal of Portfolio Management, 40(3), 113–13. https://doi.org/10.3905/jpm.2014.40.3.113
- Chou, R. K., Wang, G. H., & Wang, Y. Y. (2012). The liquidity and volatility impacts of day trading by individuals in the Taiwan index futures market. The Journal of Futures Markets, 35(5), 399–425. https://doi.org/10.1002/fut.21665
- Chou, R. K., Wang, G. H., & Wang, Y. Y. (2015). The impacts of individual day trading strategies on market liquidity and volatility: Evidence from the Taiwan Index Futures Market. Journal of Futures Markets, 35(5), 399–425.
- Chou, R. K., Wang, G. H., & Wang, Y. Y. (2015). The impacts of individual day trading strategies on market liquidity and volatility: Evidence from the Taiwan Index Futures Market. Journal of Futures Markets, 35(5), 399–425. https://doi.org/10.1002/fut.21665
- Kittiakarasakun, J., Tse, Y., & Wang, G. H. (2012). The impact of trades by traders on asymmetric volatility for NASDAQ‐100 index futures. Managerial Finance, 38(8), 752–767. https://doi.org/10.1108/03074351211239388
- Malik, N. (2014). Securities transaction tax-case study of India (No. 273). Working Paper. Indian Council for Research on International Economic Relations (ICRIER), New Delhi.
- Naacke, G., Penistone, E., & Chekanova, T. (2015). WFE/IOMA 2014 derivatives market survey. WFE, August. World Federation of Exchanges (WFE).
- Rahman, S. (2001). The introduction of derivatives on the dow jones industrial average and their impact on the volatility of component stocks. Journal of Futures Markets: Futures, Options, and Other Derivative Products, 21(7), 633–653. https://doi.org/10.1002/fut.1702
- Robbani, M. G., & Bhuyan, R. (2005). Introduction of futures and options on a stock index and their impact on the trading volume and volatility: Empirical evidence from the DJIA components. Derivatives Use, Trading & Regulation, 11(3), 246–260. https://doi.org/10.1057/palgrave.dutr.1840022
- Ryu, D. (2012). The profitability of day trading: An empirical study using high-quality data. Investment Analysts Journal, 41(75), 43–54. https://doi.org/10.1080/10293523.2012.11082543
- Schwert, G. W. (1989). Why does stock market volatility change over time? The Journal of Finance, 44(5), 1115–1153. https://doi.org/10.1111/j.1540-6261.1989.tb02647.x
- Schwert, G. W. (1990). Stock volatility and the crash of ’87. The Review of Financial Studies, 3(1), 77–102. https://doi.org/10.1093/rfs/3.1.77
- Skinner, D. J. (1989). Options markets and stock return volatility. Journal of Financial Economics, 23(1), 61–78. https://doi.org/10.1016/0304-405X(89)90005-6
- Song, F. F., Tan, H., & Wu, Y. (2005). Trade size, trade frequency, and the volatility- volume relation. The Journal of Risk Finance, 6(5), 424-437. https://doi.org/10.1108/15265940510633497.
- Srivastava, S., Yadav, S. S., & Jain, P. K. (2008). Significance of non-price variables in price discovery: An empirical study of stock option market in India. Vikalpa: The Journal for Decision Makers, 33(2), 15–24. https://doi.org/10.1177/0256090920080202
- Tauchen, G. E., Gallant, A. R., & PE, R. (1992). Stock prices and volume. The Review of Financial Studies, 5(2), 199–242. https://doi.org/10.1093/rfs/5.2.199
- Varma Jayanth, R. (2000) “Mispricing of volatility in the Indian index options market” Indian Institute of management, Ahmedabad Working Paper No. 2002-04-01, April 2002. Indian Institute of Management, IIM Ahmedabad, India.
- Yang, D., & Zhang, Q. (2000). Drift‐independent volatility estimation based on high, low, open, and close prices. The Journal of Business, 73(3), 477–492. https://doi.org/10.1086/209650