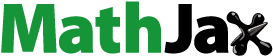
Abstract
The study aimed to identify factors that can be used in models that predict going concern of enterprises. The study employed the best-worst method of multi-criteria decision-making technique to evaluate and rank the factors. Twenty-one potential determinants were identified from the literature reviewed. Twelve decision-makers with rich experience and different professional backgrounds scrutinised and ranked the factors that affect going concern of organisations. A linear BWM solver was used to determine the optimal weights of each category. Liquidity and profitability ratios emerged as the two best determinants that are key in predicting going concern. The current ratio emerged as the overall critical determinant, while board independence measured as the ratio of non-executive board members to total board members popped up as the influential corporate governance variable for determining going concern. It is, therefore, recommended that going concern models should incorporate liquidity and profitability ratios as well as corporate governance issues.
1. Introduction
There are many concepts that guide the preparation and presentation of financial statements to the general public. However, one of the fundamental concepts that are crucial to accounting is the assumption of going concern concept (Gkouma et al., Citation2018). Going concern is described as the assertion that an entity will not cease operations but continue to operate for the foreseeable future or at least the next financial year (Sterling, Citation1968). It also refers to an enterprise’s capacity to generate enough resources to stay afloat or avoid insolvency. The concept of going concern thrives on the premise that the enterprise will continue to operate indefinitely and operate to achieve its objectives as well as accomplish its commitments and mission in the ensuing years (Kaczmarczyk, Citation2018). As indicated by Zéman and lentner (Citation2018) going concern assumption is designed to provide an indefinite and uninterrupted sequence of transactions, and goes to the heart in the preparation of the financial statement. These definitions show that the estimation and valuation of financial items such as revenue, expenses, assets and liabilities are affected by the going concern concept. It can also be deduced that financial statements are prepared based on this foundational concept and it gives assurance to the users that the organisation as an entity will be in existence for a long period to meet the aspirations and ambitions of the organisation. According to Wójcik-Jurkiewicz and Karczewska (Citation2019), when accounts are presented based on this assumption, it presupposes that the company has no intention neither to materially curtail its operations nor liquidate its assets. On the other hand, if there exists such intention of downscaling or liquidation, the financial report should be prepared on an appropriate basis and disclosed in the notes to the accounts.
The continuous existence of an entity as prescribed by the going concern assumption is of much concern to investors because previous experience has shown that even large and well-established companies can collapse. For instance, Enron Corp., WorldCom, Global Crossing Ltd., Kmart Corp., Pacific Gas and Electric Co., Reliance Group Holdings Inc., FINOVA Group Inc., and Federal-Mogul Corp are among the large firms (Obiyo & Ezenwa, Citation2012). Similarly, the financial crisis which started in 2007 resulted in countless wind-offs of businesses (Benita, Citation2019) and that has made investors and the general public alarmed by the continuity of business. The confidence of investors is boosted if the going concern status of the entity is guaranteed (Abdallah, Citation2018). Desai et al. (Citation2020) and Puspaningsih and Analia (Citation2020) have explained that enterprises that have going concern challenges are likely to default in the payment of debts when they fall due and to address this issue a number of studies have devoted to models that predict business failure and this has led to the development of many models. Aziz and Dar (Citation2006) and Altman, Citation2006) grouped the corporate failure prediction models into three broad classes; statistical models, artificial intelligence and expert systems (AIES), and theoretic models. Since different sectors require specialised insolvency forecast models, analysts utilize particular strategies and factors to develop models for each segment. Shi and Li (Citation2019) conducted a bibliometric study on predicting models and identified two groups of methods as statistical techniques and artificial intelligence/soft computing techniques. Yousaf et al. (Citation2021) have explained that the statistical techniques included Altman’s z-score, bankometer, logistic regression/logit, probit, discriminant analysis, hazard model, and partial least squares. The intelligent techniques, on the other hand, comprise data mining, support vector machine, neural network, decision tree, genetic algorithm, rough set, fuzzy logic, data envelopment analysis (DEA), Adaboost, K-nearest neighbours and Bayesian network. Other models applied more than one method. These methods are deemed as hybrid techniques. For instance, Wang et al. (Citation2021) employed a hybrid algorithm of Bayesian probabilistic networks and logistic regression to establish a bankruptcy prediction model and Sun et al. (Citation2021) used a hybrid method integrating principal component analysis with multivariate discriminant analysis and logit to develop a prediction model for financial distress.
Different models for predicting going concern and business failure have emerged over time. Balcaen and Ooghe (Citation2006) reviewed 43 predictors and classified them into 4 major groups: 1 univariate model, 2 risk index models; 21 multiple discriminant analysis models, and 19 conditional probability models. Moreover, Kumar and Ravi (Citation2007), analyzed 128 statistical and artificial intelligence models for predicting bankruptcy, concentrating on the methods employed in the different models. Jackson and Wood (Citation2013), also tabulated the occurrence and incidence of predicting tools in the previous literature. They identified five frequently used techniques as logit, univariate, multiple discriminant analysis, neural network and contingent claims. This means that researchers continue to develop models that can give a better prediction. However, the going concern predictors proposed so far have varying degrees of accuracy. This means more studies can be conducted to develop new models (Yousaf et al., Citation2021) to aid predictions.
Moreover, the variables for determining the going concerns which include ratios, such as profitability, liquidity, leverage, solvency, activity and corporate governance variables, vary from one researcher to another in terms of weight and importance (Altman, Citation2013). Different researchers employed different variables for their studies. The dominant variables are quite diverse and cannot be pinned down completely to a particular set and order. The relative importance of these identified factors is not in existence. Hence, there is a need to investigate and come out with the critical independent variables and be able to rank them in order of priority. Thus, this study tries to fill the gap by identifying factors that are useful in predicting going concern models.
This study, therefore, aims at identifying variables that can be helpful in developing models to predict going concern of entities by employing the best-worst multi-criteria method to accelerate decision-making. This paper will contribute to the literature on going concern by engaging experts in identifying the vital determinants of going concern and prioritizing in order of importance. The rest of the paper is organized as follows. Section 2 covers the theoretical framework. In section 3, the data and methods for the study are explained. Section 4 discusses the results, and section 5 concludes the study with recommendations.
2. Theoretical framework
The agency theory postulated by Jensen and Meckling (Citation1976) deals with the connection that exists between the principals and agents of the organization. The agent, that is, the management of the organization represents the principal being the owners or investors in business transactions (Benn & Bolton, Citation2011). Agency theory stems from an economic view of risk-sharing (Aaminou & Akin, Citation2020). At the very heart of the agency problem lies the concern of self-interest behaviour that may encourage an overzealous agent to not act and perform in a way that serves the best interest of the principal. As soon as the principal–agent relationship is mooted, the principal imagines the agency costs associated with it. However, when the agent takes an action contrary to the agreement, the risks assumed by the principal increase. Then emerged the first agency problem of shifts in risk sharing. The shift in risk sharing, whether actual or notion, makes it inherently difficult to create an ideal contract between the principal and the agent. This brings to a sharp focus those precarious governance mechanisms that limit the agent’s self-seeking behaviour.
The introduction of a corporate governance mechanism changes the rules under which the agent operates and reestablishes the principal interests (Lim et al., Citation2019). Based on the agency theory and its accompanying agency costs and problems, putting in place a proper mechanism to address the governance issue can help to align the divergent goals and aspirations of the principals and agents. The actions of agents are controlled by the application of the theory in the implementation of good corporate governance practices.
The institution of corporate governance results in enhanced transparency through disclosures. This in turn leads to the minimization of corporate misconduct among the management (Lim et al., Citation2019). Flowing from good governance is the proper management of companies that ensures transparency and accountability on the part of the management to the investors. This will end in the sustainability and going concern of the firm. Corporate governance is an integration of both internal and external mechanisms aimed primarily at establishing an effective governance structure and forming a balance of power between shareholders, directors, and management to better protect the interests of investors (Chen et al., Citation2020). The governance issues include shareholders concentration (Sánchez‐Ballesta & García‐Meca, Citation2007) board independence (Beekes et al., Citation2004), and director shareholding (Garcia-Meca & Sanchez-Ballesta, Citation2010), and auditor reputation (Akyol, Citation2020; Klai & Omri, Citation2011). Good corporate governance practices can yield benefits such as promoting financial transparency and accountability, attracting investment and promoting economic growth, safeguarding financial stability and creating societies that are fairer and more inclusive (Alhares et al., Citation2020; De Haes et al., Citation2019).
This study integrates financial ratios and corporate governance variables to identify factors that influence going concern of enterprises. There are many factors that affect the going concern of an organization. These factors may include variables such as profitability ratios, liquidity ratios, leverage ratios, solvency ratios, activity ratios and corporate governance variables.
3. Data and methods
3.1. Data collection
The data for the study was obtained through the administration of online questionnaires. The questionnaires were distributed to 15 experts with diverse backgrounds and experiences in assessing going concern of entities through email. The purpose of selecting participants with different expertise was to get different views from various angles for better analysis. The number of responses used for the analysis was 12 out of 15 questionnaires representing an 80% response rate. Three responses were rejected due to inconsistencies in their responses. The consistency rates of the response of the rejected questionnaires were closer to one which if added would affect the reliability of the result. Most multi-criteria decision-making studies use respondents between 4 and 10 participants (Bai et al., Citation2017; Gupta et al., Citation2020; Kusi-Sarpong et al., Citation2019). Therefore, a sample size of 12 high-quality and relevant decision-makers was enough to generate reliable results. The responses of the participants were subject to optimization and weighting to determine the best factors that predict going concern of an entity.
3.2. Background of expterts
The 12 experts who were finally used for the study were from the field of accounting, auditing, banking and finance. The majority of the respondents were male (75%) and most of them have more than ten years of experience (58.3%). The background data of the 12 participants that were used in the analysis are presented in Table .
Table 1. Background data of participants
3.3. Data analysis
The Best Worst Method (Rezaei, Citation2015, Citation2016) was used to evaluate and rank the factors that influence the going concern of enterprises. The key determining factors were ranked based on their weights. BWM developed by Rezaei (Citation2015, Citation2016) is one of the most popular and efficient multi-criteria decision analysis (MCDA) techniques used for obtaining criteria weights. BWM has the advantage over other mostly used MCDA techniques such as Analytic Hierarchy Process (AHP) and Analytic Network Process (ANP; Saaty, Citation2004) in that it requires relatively fewer pairwise comparisons for the same number of criteria with a more consistent result. It is simple but produces more reliable results. BWM has seen successful applications in various fields in recent times including Accounting (Bahadori et al., Citation2020) and Auditing (Hammond & Amissah, Citation2022).
The steps for BWM as given by Rezaei (Citation2015, Citation2016) are described below (steps 2, 3, and 4 steps are completed by the decision-makers/experts):
3.4. Step 1: Problem formulation
The first phase is to identify the set of relevant evaluation criteria. At this step, attention is given to the criteria [C1, C2 … Cn] that should be used to arrive at a decision. In this case, the criteria are the factors for determining going concern. Seventeen (17) financial ratios and four (4) corporate governance variables were identified from the literature and grouped into five main categories (criteria) with sub-criteria:
Liquidity ratios—current ratio, quick ratio, cash ratio and net working capital ratio.
Profitability ratios—gross profit margin, net profit margin and return on assets or capital employed.
Solvency/gearing ratios—debt-to-equity ratio, debt ratio, proprietary or equity ratio and interest cover ratio.
Efficiency/Activity ratios—inventory turnover, receivables turnover, payables turnover, working capital turnover and investment turnover.
Corporate Governance variables—board size, board independence, gender parity and CEO duality.
3.5. Step 2: Choosing the best and worst criteria
At this stage, the experts were asked to choose the best (B) (the most important, influential or desirable) and worst (W) (least important, influential or desirable) criteria from the set of evaluation criteria.
3.6. Step 3: Find the preference of the best criterion over all other criteria
The experts are asked to determine the preference of the best criterion over all other criteria to form a pairwise comparison between the best criterion (B) over all other criteria, using a scale of 1 to 9.
This will result in a vector as:
ABO = (aB1, aB2, …, aBn),
where aBj represents the preference of CB over C j and j = 1., 2, 3 …, n.
3.7. Step 4: Find the preference of all others over the worst criterion
Similar to the above, each of the decision-makers produces pairwise comparison ratings of all other criteria against the worst criterion (W) using the same scale of 1 to 9. This will also form a vector as:
AW = (a1W, a2W, …, anW).
where ajW represents the preference of Cj over CW and j = 1, 2, 3 …, n.
3.8. Step 5: Estimate optimal weights
Next is to obtain the optimized weights (w1*, w2*, …, wn*) for all criteria.
That is, the weights of the criteria are ascertained so that the maximum absolute differences for all j can be minimized for [|wB -aBjwj|,|wj—ajwww |]. The following minimax model will be obtained:
min max ]
s.t.
Model (Equationeqn. 1)(1)
(1) can be converted to a linear model and is indicated as:
min ξL
Model (2) can be solved to obtain the optimal weights (w1*, w2*, …, wn*) and optimal value ξL*. The consistency increases as (ξL*) approaches zero, comparisons become more reliable (Rezaei, Citation2016).
3.9. Step 6: Compute global weights
The final stage is to obtain the global weights. The global weights of each criterion are obtained by finding the product of the local weights of the main- and sub-criteria. The next step is to compute the overall score of alternatives using the additive value function (Bell et al., Citation1977)
where i is the index of any alternative, uij is the normalized score of alternative i with respect to criterion j. The value of uij can be obtained using expressions (2) and (3), where expression (4) is used for positive criteria (for benefit criteria/whose criterion value we want to increase) and expression (5) is used for negative criteria (for cost criteria/whose criterium value we want to decrease).
or
where xij is the actual score of alternative i with respect to criterion j.
4. Presentation of results
4.1. Possible determinants of going concern
After a thorough review of the relevant literature on the factors that influence going concern of an organisation, 17 financial ratios and four corporate governance variables were identified. These were grouped into five main categories (main) with subcriteria as presented below.
4.2. Choosing best and worst criteria
Following the methodology of BWM, the respondents were asked to select the best and worst criteria first from the main categories. Thereafter, they were asked to repeat the action by choosing the best and worst sub-criterion from each group of the main category. The best and worst main criteria and sub-criteria selected are presented in Table . The table indicates that most respondents chose liquidity as the important determinant of determining going concern. On the other hand, the worst criterion from the main category was solvency. The experts are asked to determine the preference of the best criterion over all other criteria to form a pairwise comparison between the best criterion (B) over all other criteria, using a scale of 1 to 9 as shown in Table . After a thorough review of the relevant literature on the factors that influence going concern of an organisation.These were grouped into five main categories (main) with subcriteria as presented in Table .
Table 2. Interpretation of BWM scale
Table 3. Determinants of going concern matrix
Table 4. Best and worst criteria
4.3. Pairwise comparison
The experts rated best-to-others and others-to-worst for the main category as well as the sub-category respectively, by applying a scale of 1–9. The pairwise comparisons for the main criteria for all participants are shown in Table and Table . The pairwise comparisons for all sub-criteria are performed.
Table 5. Pairwise comparison for main criteria—best against others
Table 6. Pairwise comparison of main criteria—others to worst
4.4. Optimal weights
The next step was to compute the optimal weights from pairwise scores attained for all categories of factors for determining going concern. The weights of each of the categories were calculated using a linear Chebyshev BWM solver. The detailed weights of each respondent as well as the consistency (ξL) are provided in Table for the main category.
Table 7. Optimal weights—main criteria
As indicated in Table , the consistency rates (ξL) for all experts were close to zero with an average of 0.099. This indicates that the model is reliable and desirable.
4.5. Global weights and ranks
This stage is to compute the global weights for the sub-criteria. The global weights of each criterion are obtained by multiplying the local weights of each sub-criterion by the weight of the main category. The sub-criteria were finally ranked based on the global weights. The weights and ranking of the criteria are presented in Table .
Table 8. Criteria weights and ranking
The global weight for each sub-criterion represents the product of the local weight of that sub-criterion and the weight of its parent main criterion. All criteria were evaluated regarding the findings using EquationEq. (3)(3)
(3) . These Vi values represent the ranking of challenges and strategies for the main criteria as shown in Table .
5. Discussion of results
There are a number of factors that affect the existence of an organization. These factors may be financial or non-financial in nature. The review of the extant literature revealed 17 financial ratios and four corporate governance indicators that influence the continuity of enterprise and as usually used in predicting going concern. These factors were captured under five main categories, namely profitability, liquidity, solvency, activity and corporate governance.
From Table , the average consistency rate (ξ = 0.099) of the rankings is close to zero. The fact that the consistency indicator is approximately zero indicates that the comparisons made by the decision makers were consistent and reliable (Rezaei, Citation2016).
The ranking by the experts in Table indicated that liquidity ratios are the most critical factors in determining going concern of entities. The ability of the firm to meet its financial obligations when they fall due has a great impact on the sustainability of the firm. If an organization fails consistently in paying its bills and payables at the right time, the firm risk of being liquidated. The second contender was profitability ratios, which signify the ability of the firm to earn enough return from operations. Profit is required for sustainability and expansion of business operations. Companies that are able to make profits are able to pay their debts, pay workers, retain and attract more qualified personnel as well as retain existing investors and attract potential investors, This finding supports the studies by Altman (Citation1968), Deakin (Citation1972), and Beaver (Citation1966) who stressed the importance of liquidity and profitability in developing bankruptcy models. Liquidity is so essential that entities with going concern challenges are likely to default in the payment of debts when they fall due (Desai et al., Citation2020; Puspaningsih & Analia, Citation2020). Thus, in determining going concern of any organization, liquidity and profitability ratios are the two most important variables to be concerned with.
In terms of the sub-criteria, the best five were current ratio (CACL), net working capital ratio (NWCL), cash ratio (CECL), net profit margin (NPMR) and return on capital employed (ROCE). The variables used by Altman (Citation1968) in developing his model christened z-score included current ratio and working capital ratio. Deakin (Citation1972) also singled out the cash ratio as a critical factor in determining going concern and business failure. Therefore, the findings collaborate well with their results.
The corporate governance variable that was found among the first 10 factors was a board independent variable. This is in line with resource dependence theory. According to the resource dependence theory, external sources offer opportunities to the organization to improve its performance, thereby enhancing its going concern status. In this regard, Khan et al. (Citation2017) stated that non-executive directors with more weighty abilities, in-depth knowledge, and immensely varied experience act as valuable assets to add value to the organization to boost operational capacity and performance. Hence, based on the theory of agency and resource dependence, there is a direct relationship between board independence and the organization’s performance. There is an expectation that the higher the number of non-executive members, the better they would manage the institution to avoid financial distress, thereby enhancing its going concern status.
6. Conclusion and recommendation
The survival of entities is essential to all stakeholders. Researchers continue to build models that can predict the fortunes of organisations with a high degree of accuracy. However, the efficacy of these models depends on the inputs. Hence, the importance of identifying the right variables has to be imputed to achieve the desired results. This study attempted to identify financial ratios and corporate governance variables that can be utilized in going concern models to enhance predictive prowess.
The study employed BWM approach to rank the factors. The finding of the study is reliable and consistent. First, the participants in the study were experienced professionals with diverse backgrounds. Moreover, it was ascertained that the mean consistency ratio was closer to 0 than to 1. This implies that the pairwise comparisons among criteria were reliable and robust.
Through an extensive literature review, 21 factors were identified. Using the linear BWM solver, the research recognized and ranked liquidity and profitability ratios as the most influential ratios that can be used to build going concern models. These two groups of ratios are really defined business success or failure. Managers of businesses are supposed to adopt strategies to boost the near-cash items while still focusing on making enough profit. Any attempt to focus on one at the expense of the other may affect the going concern of the firm.
It is recommended that further studies should focus on sector or industrial specifics to pinpoint indicators that affect the survival of that industry. More so, future research can build on this to assess the direction of influence of the factors identified. Finally, other methods such as partial least square structural equation modelling (PLS-SEM), multiple and logistic regression, nonlinear BWM, Fuzzy BWM and Bayesian BWM can be utilized to bring different dimensions to the study.
Informed consent
The study did not rely on data or participants that consent was needed.
Consent to publish
The study did not rely on data/methods/images/figures/participants that consent was needed to publish
Disclosure statement
The authors declare that they have no competing interests
Additional information
Funding
References
- Aaminou, M. W., & Akin, T. (2020). Risk-sharing finance and inequality in a benchmark agent-based model. In Iqbal, Z., Akin, T., El Maghrebi, N., & Mirakhor, A. (Eds.) Handbook of analytical studies in Islamic finance and economics. Walter de Gruyter GmbH & Co KG.
- Abdallah, S. (2018). External auditor type, discretionary accruals and investors’ reactions. Journal of Accounting in Emerging Economies, 8(3), 352–15. https://doi.org/10.1108/JAEE-10-2017-0098
- Akyol, A. C. (2020). Corporate governance and fraud. corporate fraud exposed. Emerald Publishing Limited.
- Alhares, A., Elamer, A. A., Alshbili, I., & Moustafa, M. W. (2020). Board structure and corporate R&D intensity: Evidence from Forbes global 2000. International Journal of Accounting & Information Management, 28(3), 445–463. https://doi.org/10.1108/IJAIM-11-2019-0127
- Altman, E. I. (1968). Financial ratios, discriminant analysis and the prediction of corporate bankruptcy. The Journal of Finance, 23(4), 589–609. https://doi.org/10.1111/j.1540-6261.1968.tb00843.x
- Altman, E. I. (2006). Corporate financial distress and bankruptcy: Predict and avoid bankruptcy, analyze and invest in distressed debt. Wiley.
- Altman, E. I. (2013). Predicting financial distress of companies: Revisiting the Z-score and ZETA® models. In Handbook of research methods and applications in empirical finance. Edward Elgar Publishing. Accessed on October 15 2022 from https://lemeunier.gilbert.free.fr/Investissement/DOCS/PDF/Zscores.pdf
- Aziz, M. A., & Dar, H. A. (2006). Predicting corporate bankruptcy: Where we stand? Corporate governance. The International Journal of Business in Society, 6(1), 18–33.
- Bahadori, M., Taleb Nia, G., Ranjbar, M. H., & Barkhordari Ahmadi, M. (2020). Implementation of performance-based budgeting using the combined technique of Best-Worst Method (BWM) and robust optimization. Advances in Mathematical Finance and Applications, 7(1), 263–288.
- Bai, C., Kusi-Sarpong, S., & Sarkis, J. (2017). An implementation path for green information technology systems in the Ghanaian mining industry. Journal of Cleaner Production, 164, 1105–1123. https://doi.org/10.1016/j.jclepro.2017.05.151
- Balcaen, S., & Ooghe, H. (2006). 35 years of studies on business failure: An overview of the classic statistical methodologies and their related problems. The British Accounting Review, 38(1), 63–93. https://doi.org/10.1016/j.bar.2005.09.001
- Beaver, W. H. (1966). Financial ratios as predictors of failure. Journal of Accounting Research, 4, 71–111. https://doi.org/10.2307/2490171
- Beekes, W., Pope, P., & Young, S. (2004). The link between earnings timeliness, earnings conservatism and board composition: Evidence from the UK. Corporate Governance: An International Review, 12(1), 47–59. https://doi.org/10.1111/j.1467-8683.2004.00342.x
- Bell, D. E., Keeney, R. L., & Raiffa, H. (1977). Conflicting objectives in decisions. John Wiley & Sons.
- Benita, F. (2019). Trade openness, economic growth and the global financial crisis of 2007–2009 in Latin America. Journal of International Development, 31(5), 411–431. https://doi.org/10.1002/jid.3411
- Benn, S., & Bolton, D. (2011). Key concepts in corporate social responsibility. Sage.
- Chen, C. C., Chen, C. D., & Lien, D. (2020). Financial distress prediction model: The effects of corporate governance indicators. Journal of Forecasting, 39(8), 1238–1252. https://doi.org/10.1002/for.2684
- Deakin, E. B. (1972). A discriminant analysis of predictors of business failure. Journal of Accounting Research, 10(1), 167–179. https://doi.org/10.2307/2490225
- De Haes, S., Huygh, T., Joshi, A., & Caluwe, L. (2019). National corporate governance codes and IT governance transparency in annual reports. Journal of Global Information Management (JGIM), 27(4), 91–118. https://doi.org/10.4018/JGIM.2019100105
- Desai, V., Desai, R., Kim, J. W., & Raghunandan, K. (2020). Are going‐concern issues disclosed in audit reports associated with subsequent bankruptcy? Evidence from the United States. International Journal of Auditing, 24(1), 131–144. https://doi.org/10.1111/ijau.12183
- Garcia-Meca, E., & Sanchez-Ballesta, J. P. (2010). The association of board Independence and ownership concentration with voluntary disclosure: A meta-analysis. European Accounting Review, 19(3), 603–627. https://doi.org/10.1080/09638180.2010.496979
- Gkouma, O., Filos, J., & Chytis, E. (2018). Financial crisis and corporate failure: The going concern assumption findings from Athens stock exchange. Journal of Risk & Control, 5, 141–170.
- Gupta, H., Kumar, S., Kusi-Sarpong, S., Jabbour, C. J. C., & Agyemang, M. (2020). Enablers to supply chain performance on the basis of digitization technologies. Industrial Management & Data Systems.
- Hammond, P., & Amissah, E. (2022). Examining the criteria for selecting external auditors. International Journal of Business & Management Studies, 3(1), 33–44.
- Jackson, R. H., & Wood, A. (2013). The performance of insolvency prediction and credit risk models in the UK: A comparative study. The British Accounting Review, 45(3), 183–202. https://doi.org/10.1016/j.bar.2013.06.009
- Jensen, M. C., & Meckling, W. H. (1976). Theory of the firm: Managerial behavior, agency costs and ownership structure. Journal of Financial Economics, 3(4), 305–360. https://doi.org/10.1016/0304-405X(76)90026-X
- Kaczmarczyk, A. (2018). Business restructuring in light of the going concern principle. Research insight in the selected enterprises. Transformations in Business & Economics, 17(2A), 466–480.
- Khan, I., Khan, M., & Tahir, M. (2017). Performance comparison of Islamic and conventional banks: Empirical evidence from Pakistan. International Journal of Islamic and Middle Eastern Finance and Management, 10(3), 419–433. https://doi.org/10.1108/IMEFM-05-2016-0077
- Klai, N., & Omri, A. (2011). Corporate governance and financial reporting quality: The case of Tunisian firms. International Business Research, 4, 158–166.
- Kumar, P. R., & Ravi, V. (2007). Bankruptcy prediction in banks and firms via statistical and intelligent techniques–A review. European Journal of Operational Research, 180(1), 1–28. https://doi.org/10.1016/j.ejor.2006.08.043
- Kusi-Sarpong, S., Gupta, H., & Sarkis, J. (2019). A supply chain sustainability innovation framework and evaluation methodology. International Journal of Production Research, 57(7), 1990–2008. https://doi.org/10.1080/00207543.2018.1518607
- Lim, A. L., Hashim, F., & Embong, Z. (2019). Board characteristics, investors’ confidence and firm value: Malaysian evidence. Asian Journal of Accounting and Governance, 12, (169–181).
- Obiyo, C. O., & Ezenwa, O. T. (2012). The effect of corporate reporting on public confidence; empirical evidence from quoted companies in Nigeria. Journal of Applied Management and Investments, 1, 187–194.
- Puspaningsih, A., & Analia, A. P. (2020). The effect of debt default, opinion shopping, audit tenure and company’s financial conditions on going-concern audit opinions. Review of Integrative Business and Economics Research, 9, 115–127.
- Rezaei, J. (2015). Best-worst multi-criteria decision-making method. Omega, 53, 49–57. https://doi.org/10.1016/j.omega.2014.11.009
- Rezaei, J. (2016). Best-worst multi-criteria decision-making method: Some properties and a linear model. Omega, 64, 126–130. https://doi.org/10.1016/j.omega.2015.12.001
- Saaty, T. L. (2004). Decision making—the analytic hierarchy and network processes (AHP/ANP). Journal of Systems Science and Systems Engineering, 13(1), 1–35. https://doi.org/10.1007/s11518-006-0151-5
- Sánchez‐Ballesta, J. P., & García‐Meca, E. (2007). Ownership structure, discretionary accruals and the informativeness of earnings. Corporate Governance: An International Review, 15(4), 677–691. https://doi.org/10.1111/j.1467-8683.2007.00596.x
- Shi, Y., & Li, X. (2019). A bibliometric study on intelligent techniques of bankruptcy prediction for corporate firms. Heliyon, 5(12), e02997. https://doi.org/10.1016/j.heliyon.2019.e02997
- Sterling, R. R. (1968). The going concern: An examination. The Accounting Review, 43, 481–502.
- Sun, J., Liu, X., Ai, W., & Tian, Q. (2021). Dynamic financial distress prediction based on class-imbalanced data batches. International Journal of Financial Engineering, 2150026.
- Wang, X., Pan, J., Ren, Z., Zhai, M., Zhang, Z., Ren, H., Song, W., He, Y., Li, C., & Yang, X. (2021). Application of a novel hybrid algorithm of Bayesian network in the study of hyperlipidemia related factors: A cross-sectional study. BMC Public Health, 21(1), 1–14. https://doi.org/10.1186/s12889-020-10013-y
- Wójcik-Jurkiewicz, M., & Karczewska, M. (2019). Assessment of the going concern value of a business entity by means of selected discriminative models. Scientific Journal of Bielsko-Biala School of Finance and Law, 23, 53–60.
- Yousaf, U. B., Jebran, K., & Wang, M. (2021). Can board diversity predict the risk of financial distress? Corporate Governance: The International Journal of Business in Society, 21(4), 663–684. https://doi.org/10.1108/CG-06-2020-0252
- Zéman, Z., & lentner, C. (2018). The changing role of going concern assumption supporting management decisions after financial crisis. Polish Journal of Management Studies, 18(1), 428–441. https://doi.org/10.17512/pjms.2018.18.1.32