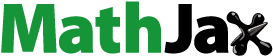
Abstract
The purpose of the study is to establish the effect of transport management practices on the performance of diamond mining companies. The study population was comprised of four authorized diamond mining companies in Zimbabwe. The sample size was comprised of 92 respondents drawn from logistics, procurement, and finance departments. Respondents were selected using stratified random sampling techniques. Data was collected using a structured 5-point Likert scale questionnaire. Regression analysis was used to test the relationship between study variables. The study found that vehicle scheduling, route planning, vehicle tracking, and fuel management positively influence the performance of diamond mining companies. This implies that the continued use of inefficient transport management practices has resulted in high transport costs, an increase in greenhouse gas emissions, and lower productivity. The study, therefore, recommended that diamond mining firms adopt contemporary transport technologies and implement safety measures such as regular vehicle maintenance, driver safety checks, and driver training. Furthermore, the study contributes to the existing body of literature on transport management and performance.
1. Introduction
The mining sector requires the movement of substantial amounts of materials, such as ore from the mine to the processing plant, and eventually to the market. Globally, the diamond mining sector plays a significant role in contributing to the gross domestic product (GDP) and employment creation. For instance, in Botswana, the diamond mining sector accounted for 33% of Botswana’s GDP in 2010 (Morgan & Hirsch, Citation2013), approximately 10% in Sierra Leone (Sesay & Mattia, Citation2015), about 14% in Namibia in 2017 (Snyman, Citation2019), and approximately 1.8% in Russia in 2019 (Alrosa, Citation2020). Despite its contribution to GDP, the mining sector incurs high transport costs in the movement of materials, equipment, and personnel within the industry (Ghebrihiwet, Citation2019). Likewise, van Jaarsveld et al., (Citation2013) have observed that transport costs account for a significant proportion of the total production costs in the mining sector. Similarly, Ngwenya and Busangavanye (Citation2016) found that high transport costs are a significant challenge for mining companies in Zimbabwe, particularly for small-scale mining operations.
Several studies conducted on transport management in the mining sector have focused on logistics planning, fleet management, supply chain optimization, and transportation safety (Hashemi & Sattarvand, Citation2015; Mhlanga et al., Citation2013; Yaping & Bossman, Citation2021). However, none of them have exposed the effect of transport management practices on performance, particularly in the diamond mining sector in Zimbabwe. This becomes a yawning research gap. The need to investigate whether transport management practices can reduce costs, improve ore production, and reduce environmental impact has therefore elicited interest in this direction. The mining sector has been a significant contributor to Zimbabwe’s economy, with minerals such as platinum, diamonds, gold, coal, and chrome being the main contributors. The sector accounts for approximately 10% of the country’s GDP (African Mining Market, Citation2021). However, despite the potential of the sector, it has faced various challenges such as inadequate transport infrastructure, a lack of capital investment, and weak regulatory frameworks (Gono et al., Citation2021). Similarly, Mhlanga et al. (Citation2013) have observed that the potential of the Zimbabwean mining sector has been hamstrung by inefficient transport management practices, which have severely affected production targets for the sector.
The continued use of inefficient transport management practices by diamond mining companies is contributing to increased operational costs, decreased productivity, and compromised safety, posing a serious threat to the performance of these mines. With skyrocketing fuel usage, poor driver management, high vehicle maintenance costs, and environmental regulations, the urgency of implementing effective transport management practices has never been greater. Failure to improve transport management practices not only undermines the firms’ bottom line but also threatens their ability to compete in an increasingly competitive business environment. Given these circumstances, this research is crucial because it addresses a critical knowledge gap that needs to be filled urgently to inform decision-making. The following objectives are sought by this study based on the arguments advanced: (1) to determine the effect of vehicle scheduling on performance; (2) to establish the influence of route planning on performance; (3) to understand the influence of fuel management on performance; and (4) to examine the effect of vehicle tracking on performance. The rest of the paper is organized as follows: Section 1 provides the introduction. Section 2 provides literature review. Section 3 outlines the research methodology adopted in this study. Section 4 presents the analysis and results. Section 5 presents a discussion of the findings. The paper ends with Section 6, which presents conclusions and implications.
2. Literature review
This section presents the theoretical framework and how hypotheses were developed.
2.1. Theoretical framework
The transportation model underpins the study. The model uses mathematical and analytical tools to optimize transport management decisions. It involves modeling transportation systems, analyzing data, and using optimization techniques to identify the most efficient and effective transportation strategies. In addition, it can be used to determine the optimal routes for deliveries, considering factors such as distance, traffic congestion, and delivery time windows. The model can also be used to determine the optimal inventory levels for fuel. Furthermore, the model was used in this study because it assists in making meaningful decisions on vehicle scheduling, allocation of vehicles to different routes, and optimization of fuel consumption.
2.2. Transport management practices
Transport management practices refer to the planning, execution, and control of the movement of goods and people from one location to another (Eisaeia et al., Citation2017). Likewise, Munuhuwa et al. (Citation2020) asserted that transport management encompasses all actions required to maintain and operate vehicles throughout their life cycles, from the initial stages of acquisition to the final stages of disposal. Transport management practices encompass all strategies and techniques used by organizations to manage the movement of goods and people efficiently and effectively from one location to another (Sirina & Zubkov, Citation2021). Transport management practices include route planning, vehicle scheduling, vehicle maintenance, freight consolidation, tracking and monitoring, safety management, fuel management, and driver management (Chiparo et al., Citation2022; Munuhuwa et al., Citation2020; Musau et al., Citation2017). The transport management practices considered in this study include vehicle scheduling, vehicle tracking, route planning, and fuel management.
2.2.1. Vehicle scheduling
Vehicle scheduling refers to the process of assigning vehicles to specific tasks or routes (Bredstrom & Ronnqvist, Citation2007). They added that vehicle availability ensures that there are an adequate number of vehicles available for scheduling. In addition, specific requirements such as the number of passengers, the amount of cargo, and the distance to be traveled should be considered when assigning vehicles to tasks (Shui et al., Citation2015). Likewise, Munuhuwa et al. (Citation2020) added that the most efficient route must be chosen for each task to minimize travel time and fuel consumption. Chiparo et al. (Citation2022) noted that drivers must be available for each scheduled trip, and their hours of service must be considered to ensure compliance with regulations. They added that vehicles must be regularly maintained to ensure that they are safe and reliable. In addition, the cost of fuel, maintenance, and labor must be considered when scheduling vehicles (Eglese et al., Citation2006).
2.2.2. Route planning
Route planning refers to the process of determining the most efficient and effective route between two or more points (Wang & Zhu, Citation2012). Van Zandt (Citation1992) observed that the starting and ending points of the journey are the most fundamental constructs in route planning as they help to identify the distance, time, and various modes of transport that can be used. Kaneko and Honda (Citation2016) pointed out that when selecting a mode of transport, it is important to consider factors such as distance, time, cost, and personal preferences. In addition, navigation tools such as maps, global positioning system (GPS) are essential constructs in route planning as they help identify the most efficient and effective routes. Furthermore, safety and security are important in route planning and include factors such as the safety of the mode of transportation, the safety of the route, and the availability of emergency services (Mathias & Foley, Citation2013).
2.2.3. Vehicle tracking
Li et al. (Citation2018) explained that vehicle tracking allows the owner or operator of a vehicle to keep track of its movements as well as gather other valuable information such as fuel consumption, engine performance, and driver behavior. A global positioning system (GPS)-based vehicle tracking system typically consists of a GPS device that is installed in the vehicle, which is then connected to a network or software application that can track its location and other data (Aloquili et al., Citation2009). This information can be accessed by the vehicle owner or operator through a web-based dashboard, mobile app, or other interface (Cui et al., Citation2019). Begashaw (Citation2018) asserted that vehicle tracking systems may significantly reduce accidents, improve fuel efficiency, and enhance the overall performance of fleets. Vehicle tracking systems help optimize logistics operations by reducing transportation costs, improving delivery times, and enhancing the overall efficiency of supply chains (Muchaendepi et al., Citation2018).
2.2.4. Fuel management
Fuel management is a function that involves planning, organizing, controlling, and monitoring the use of fuel resources to optimize their efficiency and minimize waste (Mohapatra & Tripathy, Citation2018). Fuel management involves fuel procurement, fuel monitoring and reporting, fuel quality management, fuel efficiency, and environmental considerations (Chiparo et al., Citation2022). Fuel procurement involves the process of sourcing and acquiring fuel for an organization’s needs. It includes negotiating contracts with suppliers, monitoring market trends and prices, and ensuring that the fuel meets quality standards. Organizations should keep track of fuel supplies, monitor usage levels, and maintain adequate inventory levels for fuel to meet operational needs (Chiparo et al., Citation2022). It is also important for organizations to monitor fuel quality, test fuel samples, and implement quality control measures to ensure that the fuel meets established standards. Effective fuel management requires continuous monitoring and reporting of fuel consumption, inventory levels, and other key metrics (Gitahi & Ogollah, Citation2014). This information can be used to identify trends, track performance, and make informed decisions about fuel management strategies. Furthermore, it is important to consider the environmental impact of fuel use. This includes reducing emissions, implementing environmentally friendly fuel alternatives, and complying with relevant regulations and standards.
2.3. Performance
Performance refers to the outcomes of a company’s internal and external systems (Karabulut, Citation2015). On the other hand, Al-Matari et al. (Citation2014) explained that an organization’s success is primarily determined by its performance over a specific period. The performance of a firm is usually determined using a set of non-financial and financial factors (De Loecker & Goldberg, Citation2014). Financial performance measures include profitability, which is measured using return on investment (ROI), working capital ratio, gross profit margin, and net profit after tax (Wijetunge & Pushpakumari, Citation2014). On the other hand, non-financial performance focuses on improving a company’s image, attracting new customers, and enhancing customer loyalty. Other aspects of non-financial performance include productivity, growth, market share, and customer satisfaction (Al-Matari et al., Citation2019). In addition, Sinha (Citation2020) explained that organizations should consider the environmental impact of their activities when assessing their performance. The measures for environmental impact include carbon emissions, energy consumption, land and ocean pollution, deforestation, natural resource depletion, land degradation, biodiversity, and global warming, among others (Manrique & Martí-Ballester, Citation2017; Younis et al., Citation2020). Mining is a capital-intensive industry that is prone to various risks and challenges, such as volatile commodity prices, geopolitical instability, environmental regulations, and social responsibility. Therefore, performance measures adopted by mining companies should align with their strategic objectives, stakeholder expectations, and industry standards (Arthur et al., Citation2017; Wibowo, Citation2013). Appendix 1 shows items that were used to measure performance.
2.4. Development of hypothesis and research model
Vehicle scheduling is a critical aspect of transport management that involves organizing and allocating vehicles to transport goods and people efficiently (Mendes & Iori, Citation2020; Xu & Kelly, Citation1996). Several studies have examined the impact of vehicle scheduling on organizational performance, and the findings suggest that efficient vehicle scheduling can lead to better performance in terms of cost savings, customer satisfaction, and productivity (Musau et al., Citation2017). They added that proper vehicle scheduling can lead to cost savings by reducing idle time and empty vehicle runs, optimizing fuel consumption, and minimizing maintenance costs. A study by Siddiqui and Verma (Citation2015) found that proper vehicle routing and scheduling can reduce transportation costs and improve customer satisfaction by ensuring that goods are delivered at the right time. Correspondingly, Ceselli et al. (Citation2009) found that a well-designed vehicle scheduling system can lead to a reduction in delivery times and improve customer satisfaction levels. In addition, Zaki et al. (2021) found that optimizing vehicle scheduling can lead to an increase in the number of deliveries per day, resulting in increased productivity levels. Furthermore, Erdoğan and Miller-Hooks (Citation2012) found that an optimized vehicle routing and scheduling system could reduce CO2 emissions. It is, therefore, hypothesized that:
H1:
Vehicle scheduling has a positive effect on performance.
Route planning is an essential aspect of transport management that involves the identification of the most efficient and effective routes to use in the transportation of goods or services from one location to another. A study conducted by Musau et al. (Citation2017) found that route planning positively influences organizational performance. Lindström and Fjeld (Citation2014) conducted a study on route planning problems and found that the major goal of vehicle route planning is to maximize transport capacity while reducing transportation costs. Likewise, Nha et al. (Citation2012) studied route planning in smart cities and found that inefficient route planning and optimization result in higher transport costs. According to Wang and Zhu (Citation2012), route planning can have a positive impact on the performance of organizations in terms of cost reduction and customer service improvement. They added that effective route planning can also help reduce transport costs by minimizing the distance traveled, fuel consumption, and vehicle repair and maintenance costs. Similarly, a study by Van Zandt (Citation1992) found that route planning can improve supply chain performance by reducing transportation costs, improving delivery reliability, and enhancing customer satisfaction. It is, therefore, hypothesized that:
H2:
Route planning has a positive effect on performance.
The overall agreement in transport literature is that vehicle tracking positively affects organizational performance (Begashaw, Citation2018; Muchaendepi et al., Citation2018; Waiyaki, Citation2013). A study by Ngumbi (Citation2016) concluded that vehicle tracking eliminates fleet inefficiencies like journey duplication and unscheduled journeys and ensures that drivers practice safe and economic driving styles that reduce wear and tear on the vehicles. Likewise, Aloquili et al. (Citation2009) explained that vehicle tracking improves productivity and business performance levels since managers would be able to monitor vehicles and control all fleet users. By the same token, Li et al. (Citation2018) revealed that vehicle tracking allows an organization to communicate with customers about the lead time and other possible delays in the delivery of the consignment. A study by Muchaendepi et al. (Citation2018) revealed that vehicle tracking technology allows organizations to monitor the location and status of their vehicles in real-time. They added that the information gathered by vehicle tracking systems can be used to optimize routes, reduce idle time, and improve fuel efficiency. Vehicle tracking can also help organizations improve fleet efficiency, reduce costs, enhance safety, improve customer service, and optimize asset utilization (Humphries et al., Citation2005; Kumar et al., Citation2022; Mounika et al., Citation2021). It is therefore hypothesized that:
H3:
Vehicle tracking has a positive effect on performance.
There is a general agreement in the literature that fuel management significantly influences organizational performance (Aflabo et al., Citation2020; Chiparo et al., Citation2022; Gitahi & Ogollah, Citation2014). A sizeable number of scholars have applauded fuel management as a key transport management component that facilitates the movement of vehicles at any point in time (Kamalanathsharma & Rakha, Citation2016; Zimon, Citation2016). Fuel management aspects such as monitoring fuel consumption rates, fuel procurement, allocations of fuel, and monitoring fuel usage rates influence the ability of an organization to respond to its customers (Gitahi & Ogollah, Citation2014). Similarly, research by Aflabo et al. (Citation2020) highlighted that proper fuel management can reduce vehicle fuel consumption, which in turn saves an organization’s financial resources. In addition, Chiparo et al. (Citation2022) found that fuel management aspects, such as fuel-efficient driving techniques and route optimization, can significantly reduce an organization’s carbon footprint. It is therefore hypothesized that:
H4:
Fuel management has a positive effect on performance.
Based on the foregoing discussion, the research model is shown in Figure .
4.Research Methodology
3. Methods
3.1. Sample
The study was conducted in Zimbabwe, a landlocked country located in southern Africa bordered by South Africa, Botswana, Zambia, and Mozambique. Zimbabwe is renowned for having a wealth of natural resources, including coal, iron ore, copper, diamonds, gold, platinum, and other minerals. Zimbabwe’s economy is heavily dependent on agriculture and mining, with the mining sector contributing 23% of the country’s total exports (Mapira, Citation2017). The context of this study is the country’s diamond mining industry, which is ranked among the top seven diamond producers in the world. The country is estimated to have produced over 14.5 million carats in 2012, including output from the River Ranch and Murowa mines (Centre for Natural Resources Governance, Citation2013). The target population for this study was composed of four authorized diamond mining companies in Zimbabwe (Mining Zimbabwe, Citation2021). A total of 120 people were drawn from the logistics, procurement, and finance departments of these mining companies. The sample size was determined using Krejcie and Morgan’s (Citation1970) formula, as shown below.
Where: X2= the table value of chi-square for one degree of freedom at the desired confidence level (3.841).
N = the population size.
P = the population proportion (assumed to be 0.50 since this would be the maximum sample size); and
d = the degree of accuracy expressed as a proportion (0.05)
According to Krejcie and Morgan’s (Citation1970) formula, 92 people participated in the quantitative survey. A quantitative survey design involves the collection of data from a representative sample of individuals through standardized questionnaires (Dillman et al., Citation2014). The aim of a survey design is to provide inferential statistics that are used to generalize findings to a larger population. In the current study, a survey design was implemented through a self-administered structured questionnaire. This allowed the researcher to remain objective during data collection, as the questions were standardized to reduce the potential for personal bias and interpretation of the data (Hickey & Kipping, Citation1996). The questionnaire was pilot tested to identify errors and make necessary adjustments to ensure valid and reliable data was collected. Creswell (Citation2014) recommended that when adopting a survey design, the common sampling techniques that should be used include random sampling, stratified sampling, and cluster sampling. Based on this recommendation, this study adopted stratified random sampling. Stratified random sampling involves dividing a population into homogeneous subgroups or strata and then selecting a sample from each stratum based on some predetermined criteria (Keskintürk & Er, Citation2007). A list of people working in the logistics, procurement, and finance departments was obtained from the relevant authorities of each mining company. Respondents were grouped into strata based on their management levels. As such, the strata were comprised of people from different levels of management, such as directors, managers, supervisors, and drivers. These were selected based on their understanding of research variables. Stratified sampling was used to improve the representativeness and precision of the sample due to the large differences that existed between subgroups in the population (Haruna et al., Citation2021).
Questionnaires were self-
administered to a total of 92 respondents. The researcher physically visited the diamond mining companies to collect data. The data collection process was carried out between January 27 and February 13, 2022, to give respondents ample time to complete the questionnaires. The researcher visited diamond mining companies between February 15 and February 18 to collect completed questionnaires. From the 92 questionnaires distributed, 83 were successfully completed, while 9 were unaccounted for, giving a response rate of 90%. To ensure a high response rate, the researcher used the following strategies: providing clear and concise instructions on how to complete questionnaires; using simple language; giving assurance to respondents that their responses will be treated with anonymity; conducting a pilot study prior to the actual study; and conducting a follow-up using the phone. Ponto (Citation2015) argues that a 60% response rate is good, while a response rate above 70% is considered excellent. This shows that the response obtained in this study was reliable and credible, and thus meaningful conclusions and implications were drawn. Responses obtained were validated using secondary data sources such as journal, reports, and textbooks on transport management. The basic characteristics of respondents, as depicted in Table , show that 73% of the respondents were males while 27% were females, suggesting that the study was dominated by males. This result implies that the diamond mining sector in Zimbabwe is a male-dominated sector. The most plausible explanation for the underrepresentation of women in the mining sector is that most mines are in remote areas and require a lot of physical work. Additionally, 88% of the respondents were aged between 20 and 50, indicating that the participants were economically active. Furthermore, 54% of the respondents were drawn from the logistics department, 33% from the procurement department, and 13% from the finance department, suggesting that the study was comprised of people with an understanding of transport management practices and performance issues.
Table 1. Demographic Information
3.2. Measurement
To measure all variables, 5-point Likert-type scales were used. Appendix 1 shows the measurement scale with items that were used to measure vehicle scheduling, route planning, fuel management, and vehicle tracking based on the Likert scale, which ranged from 1 (strongly disagree) to 5 (strongly agree). The study borrowed items from prior related studies and modified them to suit the current study (Aflabo et al., Citation2020; Begashaw, Citation2018; Chiparo et al., Citation2022; Gitahi & Ogollah, Citation2014; Lindström & Fjeld, Citation2014; Lu & Yang, Citation2019; Musau et al., Citation2017). The collected data was analyzed using the statistical package for social sciences (SPSS) version 23. The regression model was used to establish the influence of the study variables. The proposed regression model was presented as:
Where;
Y= Performance
X1= Vehicle scheduling
X2= Route Planning
X3= Vehicle Tracking
X4= Fuel Management
β1 and β2= Regression equation for each coefficient for the variables discussed
C = Constant.
4. Analysis and results
4.1. Data validation
Data were validated using reliability test, normality test and correlation matrix. The reliability test results in Table show that all the variables were reliable with a Cronbach’s alpha above 0.90. A Cronbach’s alpha of 0.80 or higher indicates that the gathered data is reliable, has relatively high internal consistency, and can be generalized to reflect the opinions of all respondents in the target population (Zinbarg et al., Citation2005). The Shapiro-Wilk test was used to determine whether there was any correlation in the sample data. The test produced a statistic of 0.865 and a p-value of 0.000, as shown in Table . The significance of p < 0.05 indicates that the data was extracted from a normally distributed sample (Arnastauskaitė et al., Citation2021). In addition, a multicollinearity test was performed using correlation statistics to check if the strength of the relationship between the variables would affect further statistical analysis. As recommended by Hair et al. (Citation2014), the correlation statistics should not exceed 0.7 for the method to be robust. As shown in Table , all the variables are within the ranges suggested by the literature.
Table 2. Reliability Test
Table 3. Normality Test
Table 4. Correlation Matrix
4.2. Inferential Statistics
The study used analysis of variance (ANOVA) and regression analysis of coefficients to establish the relationship between study variables.
4.2.1. Analysis of variance (ANOVA)
An analysis of variance (ANOVA) was used to establish the influence of transport management practices on the performance of diamond mining companies in Zimbabwe. Four variables (vehicle scheduling, route planning, vehicle tracking, and fuel management) were analyzed as independent variables, while performance was treated as the dependent variable. The results are as shown in Table .
Table 5. Analysis of Variance
Table shows that vehicle scheduling had an F-ratio of 103.425, indicating that it was the most influential factor on performance. The results also revealed that route planning was the second most influential factor with an F-ratio value of 61.414, indicating that it influenced the performance of diamond mining firms in Zimbabwe. This is supported by Bell et al. (Citation2018), who pointed out that the greater the F-ratio, the greater the influence. In addition, study results also indicated that vehicle tracking had an F-ratio of 46.761, indicating that it had the third influence on performance. Furthermore, fuel management had an F-ratio of 28.087, implying that it had the lowest influence on performance. All variables had a p-value below 0.05 to confirm a significant relationship with performance.
4.2.2. Regression analysis
The researcher conducted regression analysis to establish the influence of each transport management practice on performance. The results are shown in Table .
Table 6. Regression Analysis of Coefficients
Dependent Variable: Performance
The regression model is given by:
Vehicle scheduling has a positive unit score of 0.514, indicating that it significantly influences the performance of diamond mining companies in Zimbabwe. This result shows that key factors such as vehicle availability, driver availability, vehicle repair and maintenance, traffic conditions, capacity, and distance not considered in the transportation of personnel and mining ore, resulting in high transport costs. The score confirms that a unit increase of 0.514 in vehicle scheduling positively influences the performance of diamond mining companies by the same value. In addition, route planning had a positive unit score of 0.292, implying that the factor has a significant influence on performance. This result shows that route planning does not consider safety and security, resulting in increased fuel consumption. Therefore, a 0.292 increase in route planning would positively increase the performance of diamond mining companies with the same value.
Additionally, vehicle tracking had a unit score of 0.224, indicating that the factor has a significant influence on performance. This result shows that the vehicle tracking systems currently used by diamond mining companies provide delayed reports and inaccurate data. This entails that through effective tracking, diamond mining organizations can realize some positive outcomes in terms of return on investment, profitability, and productivity. Consequently, a unit increase of 0.224 in vehicle tracking would positively increase performance by the same value. Furthermore, fuel management showed a positive unit score of 0.039, indicating that the factor had a lower significance for the performance of diamond mining firms. Therefore, a unit increase of 0.039 in fuel management would positively influence performance with the same value. The constant was 0.145, indicating that the performance of diamond mining firms was significantly affected by vehicle scheduling, route planning, vehicle tracking, and fuel management.
5. Discussion
The research findings confirmed that vehicle scheduling positively influences the performance of diamond mining companies in Zimbabwe. These results are consistent with the theoretical proposals of Pak and Mun (Citation2022), who found that proper vehicle scheduling can lead to cost savings by minimizing the number of vehicles required to complete a given set of tasks. In a similar manner, Kleff (Citation2019) asserted that efficient vehicle scheduling can also help reduce the environmental impacts of transportation operations by minimizing fuel consumption and emissions. Similarly, Globisch et al. (Citation2018) alluded to the fact that vehicle scheduling can also help improve delivery times by ensuring that vehicles are deployed in the most efficient way possible. This can be achieved by considering factors such as traffic conditions and driver availability. The result suggests that the consideration of vehicle scheduling aspects such as vehicle availability, driver availability, vehicle repair and maintenance, traffic conditions, capacity, and distance enhance the firm’s profitability, reduces greenhouse emissions, and improves productivity. In addition, the results provide adequate confirmation that route planning positively affects the performance of diamond mining companies. This result shows that the distance between origin and destination, safety and security, terrain, and road conditions influence the total transport costs as well as improve a firm’s ability to reduce noise and air pollution. These results complement findings by Vareias et al. (Citation2019), who established that route planning can increase productivity and throughput by reducing the amount of time drivers spend on the road. Similarly, Chiparo et al. (Citation2022) pointed out that proper planning of routes improves the allocation of drivers, vehicles, and fuel, resulting in cost savings. In addition, proper route planning can help reduce the environmental impact of transportation by optimizing fuel usage and reducing greenhouse gas emissions.
Moreover, the results show that vehicle tracking contributes significantly to the performance of diamond mining companies in Zimbabwe. This suggests that the ability of diamond mining companies to monitor vehicle location and driver behavior reduces fuel costs, reduces repair and maintenance costs, and increases driver productivity. These finding ties in well with a theoretical study by Begashaw (Citation2018), who stated that vehicle tracking gives an accurate position of the vehicle and provides statistics on fuel consumption, driver behavior, and average speed of vehicles. Correspondingly, Ngumbi (Citation2016) pointed out that vehicle tracking eliminates fleet inefficiencies like journey duplication and unscheduled journeys. Furthermore, the study findings confirm that fuel management positively influences the performance of diamond mining companies. This implies that indicators of fuel management such as fuel consumption rates, fuel sourcing, inventory management, fuel monitoring, and the use of environmentally fuel sources influence profitability levels, productivity, and the production of greenhouse gas emissions. These results are consistent with the findings of Zimon (Citation2016), who asserts that the fuel management practices used by an organization could potentially affect motor running expenses.
6. Conclusion and Implications
From the findings, the study concludes that vehicle scheduling positively influences the performance of diamond mining companies in Zimbabwe. The study also concludes that aspects of vehicle scheduling such as vehicle availability, driver availability, vehicle repair and maintenance, traffic conditions, capacity, and distance influence the performance of diamond mining companies in Zimbabwe. The study also concludes that distance, cost, terrain, road conditions, safety, and security are aspects of route planning that influence the performance of diamond mining companies. Additionally, the study concludes that the ability to provide accurate data in real-time, the ability of the vehicle tracking system to report all necessary data, and the ease of sending and receiving signals are some of the aspects of vehicle tracking that influence the performance of diamond mining companies. Furthermore, the study concludes that fuel management significantly influences the performance of diamond mining companies. This implies that aspects of fuel management such as fuel consumption rates, fuel sourcing, monitoring and usage rates, and the use of environmentally friendly fuel alternatives influence the performance of diamond mining companies.
The study was motivated by the desire to gain a solid understanding of the influence of transport management practices on performance. To improve performance, diamond mining companies should consider vehicle scheduling, route planning, vehicle tracking, and fuel management in their business practices, as these play a pivotal role in their performance. Considering the above conclusions, the study recommends that firms in the diamond mining sector should invest massively in contemporary technologies such as global positioning systems (GPS) to track their vehicles, consignments, and drivers. In addition, firms should provide training (such as defensive driving and technology usage) to their drivers and other staff members to reduce costs associated with accidents, repairs, maintenance, and delays. Additionally, companies should implement safety measures such as regular vehicle maintenance, driver safety checks, and driver training to reduce accidents and the costs that emanate from poor vehicle maintenance. Firms should prioritize the use of data analytics tools to track key performance indicators such as fuel efficiency and delivery times. Likewise, firms should make use of route optimization software to reduce travel time, fuel consumption, and greenhouse gas emissions. Moreover, diamond mining companies should collaborate with their suppliers, logistics providers, and government agencies on key business issues, such as optimizing routes and creating a seamless flow of mineral ore, equipment, and other accessories. Furthermore, diamond mining firms should regularly review their performance metrics and implement necessary changes.
Furthermore, the study presents some limitations that suggest possibilities for future research. One limitation is that the study was conducted in Zimbabwe’s diamond mining sector, making it difficult to generalize the findings. Therefore, it is suggested that future studies of this type be conducted in other sectors of the economy and in different countries to generalize the findings. Furthermore, if different transport management practices are used, the study could yield more interesting results.
Disclosure statement
No potential conflict of interest was reported by the author.
Additional information
Notes on contributors
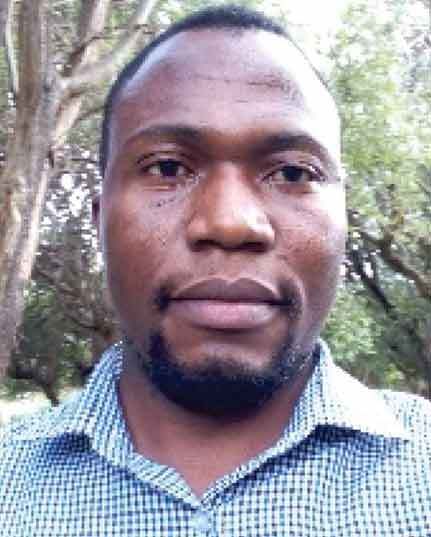
James Kanyepe
James Kanyepe is an academic, researcher and consultant. Among many educational qualifications, he holds a PhD in Supply Chain Management from Chinhoyi University of Technology, Zimbabwe. He is currently a Lecturer in the Department of Management at University of Botswana. His research area of interest is supply chain management.
References
- Aflabo, J., Kraa, J. J., & Agbenyo, L. (2020). Examining the effect of fleet management on competitive advantage in the transport industry. European Journal of Logistics, Purchasing and Supply Chain Management, 8(2), 7–17. https://doi.org/10.37745/ejlpscm/vol8.no2.pp7-23.2020
- African Mining Market. (2021). Mining sector in Zimbabwe. https://africanminingmarket.com/mining-sector-in-zimbabwe/
- Al-Matari, E. M., Al-Swidi, A. K., & Fadzil, F. H. B. (2014). Audit committee characteristics and executive committee characteristics and firm performance in Oman: Empirical study. Asian Social Science, 10(12), 98–113. https://doi.org/10.5539/ass.v10n12p98
- Al-Matari, E. M., Al-Swidi, A. K., & Fadzil, F. H. B. (2014). The measurements of firm performance’s dimensions. Asian Journal of Finance & Accounting, 6(1), 24–49. https://doi.org/10.5296/ajfa.v6i1.4761
- Aloquili, O., Elbanna, A., & Al-Azizi, A. (2009). Automatic vehicle location tracking system based on GIS environment. IET Software, 3(4), 255–263. https://doi.org/10.1049/iet-sen.2008.0048
- Alrosa. (2020). ALROSA diamond mining in Russia. Retrieved from https://www.alrosa.ru/press-releases-item/alrosa-diamond-mining-in-russia.
- Arnastauskaitė, J., Ruzgas, T., & Bražėnas, M. (2021). An Exhaustive Power Comparison of Normality Tests. Mathematics, 9(788), 1–20. https://doi.org/10.3390/math9070788
- Arthur, C. L., Obro-Adibo, G., & Tackie, G. (2017). Corporate Social responsibility and performance: A case study of mining companies in Ghana. Journal of Economics & Sustainable Development, 8(20), 48–57.
- Begashaw, M. (2018). The Effect of Fleet Management on Fleet Efficiency on Operational Efficiency. Thesis Submitted to the Department of Logistics and Supply Chain Management, University of Addis Ababa, Addis Ababa
- Bell, E., Bryman, A., & Harley, B. (2018). Business Research Methods. Oxford University Press.
- Bredstrom, D., & Ronnqvist, M. (2007). A branch and price algorithm for the combined vehicle routing and scheduling problem with synchronization constraints. SSRN Electronic Journal. ISSN 1556-5068, Elsevier BV. https://doi.org/10.2139/ssrn.971726
- Centre for Natural Resources Governance. (2013). Analysis of Zimbabwe’s Diamond Policy. http://swradioafrica.com/Documents/Zimbabwe%20Diamond%20Policy%20Analysis.pdf
- Ceselli, A., Righini, G., & Salani, M. (2009). A column generation algorithm for a rich vehicle-routing problem. Transportation Science, 43(1), 56–69. https://doi.org/10.1287/trsc.1080.0256
- Chiparo, J., Tukuta, M., & Musanzikwa, M. (2022). Vehicle Fleet management practices and service delivery in state owned enterprises in Zimbabwe. Journal of Transportation Technologies, 12(02), 159–171. https://doi.org/10.4236/jtts.2022.122010
- Creswell, J. W. (2014). Research Design: Qualitative, Quantitative and Mixed Methods Approaches (4th ed.). Sage.
- Cui, Y., Xu, H., Wu, J., Sun, Y., & Zhao, J. (2019). Automatic vehicle tracking with roadside LiDAR data for the connected-vehicles system. IEEE Intelligent Systems, 34(3), 44–51. https://doi.org/10.1109/MIS.2019.2918115
- De Loecker, J., & Goldberg, P. K. (2014). Firm performance in a global market. Annual Reviews Economics, 6(1), 201–227. https://doi.org/10.1146/annurev-economics-080113-104741
- Dillman, D. A., Smyth, J. D., & Christian, L. M. (2014). Internet, phone, mail, and mixed-mode surveys: The tailored design method. John Wiley & Sons.
- Eglese, R., Maden, W., & Slater, A. (2006). A road timetableTM to aid vehicle routing and scheduling. Computers & Operations Research, 33(12), 3508–3519. https://doi.org/10.1016/j.cor.2005.03.029
- Eisaeia, M., Moridpourb, S., & Tay, R. (2017). Heavy vehicle management: restriction strategies. Transportation Research Procedia, 21, 18–28. https://doi.org/10.1016/j.trpro.2017.03.074
- Erdoğan, S., & Miller-Hooks, E. (2012). A green vehicle routing problem. Transportation Research Part E: Logistics & Transportation Review, 48(1), 100–114. https://doi.org/10.1016/j.tre.2011.08.001
- Ghebrihiwet, N. (2019). FDI technology spillovers in the mining industry: Lessons from South Africa’s mining sector. Resources Policy, 62, 463–471. https://doi.org/10.1016/j.resourpol.2018.04.005
- Gitahi, P., & Ogollah, K. (2014). Influence of fleet management practices on service delivery to refugees in United Nations High Commissioner for Refugees Kenya programme. European Journal of Business Management, 2(1), 1–18.
- Globisch, J., Dütschke, E., & Wietschel, M. (2018). Adoption of electric vehicles in commercial fleets: Why do carpool managers campaign for BEV procurement? Transportation Research, Part D: Transport & Environment, 64, 122–133. https://doi.org/10.1016/j.trd.2017.10.010
- Gono, J., Osei Lartey, P., Amoah, S. K., & Appiah, A. (2021). An analysis of the mining sector in Zimbabwe: Current trends and future prospects. Resources Policy, 72, 102108. https://doi.org/10.1016/j.resourpol.2021.102108
- Hair, F. J., Sarstedt, M., Hopkins, L., & Kuppelwieser V, G. (2014). Partial least squares structural equation modeling (PLS-SEM) an emerging tool in business research. European Business Review, 26(2), 106–121. https://doi.org/10.1108/EBR-10-2013-0128
- Haruna, N. F., Singh, R. V. K., & Dahiru, S. (2021). A modified ratio type estimator of finite population mean under stratified random sampling scheme. Indian Journal of Applied Research, 58–60. https://doi.org/10.36106/4917049
- Hashemi, A. S., & Sattarvand, J. (2015). Simulation based investigation of different fleet management paradigms in open pit mines-a case study of Sungun copper mine. Archives of Mining Sciences, 60(1), 195–208. https://doi.org/10.1515/amsc-2015-0013
- Hickey, G., & Kipping, C. (1996). A multi-stage approach to the coding of data from open-ended questions. Nurse Researcher, 4(1), 81–91. https://doi.org/10.7748/nr.4.1.81.s9
- Humphries, M., Radev, P., & Shirvaikar, M. (2005). A realtime vehicle tracking system. In Proceedings of the Thirty-Seventh Southeastern Symposium on System Theory, 2005. SSST’05. 357–361. https://doi.org/10.1109/SSST.2005.1460936
- Kamalanathsharma, R. K., & Rakha, H. A. (2016). Leveraging connected vehicle technology and telematics to enhance vehicle fuel efficiency in the vicinity of signalized intersections. Journal of Intelligent Transportation Systems, 20(1), 33–44. https://doi.org/10.1080/15472450.2014.889916
- Kaneko, K., & Honda, S. (2016). A map database system for route navigation with multiple transit points and destination points. In 2016 5th IIAI International Congress on Advanced Applied Informatics 219–223. https://doi.org/10.1109/IIAI-AAI.2016.203.
- Karabulut, A. T. (2015). Effects of innovation strategy on firm performance: a study conducted on manufacturing firms in Turkey. Procedia - Social & Behavioral Sciences, 195, 1338–1347. https://doi.org/10.1016/j.sbspro.2015.06.314
- Keskintürk, T., & Er, Ş. (2007). A genetic algorithm approach to determine stratum boundaries and sample sizes of each stratum in stratified sampling. Computational Statistics & Data Analysis, 52(1), 53–67. https://doi.org/10.1016/j.csda.2007.03.026
- Kleff, A. (2019). Scheduling and Routing of Truck Drivers Considering Regulations on Drivers’ Working Hours (Doctoral dissertation, Karlsruher Institut für Technologie (KIT)).
- Krejcie, R. V., & Morgan, D. W. (1970). Determining sample size for research activities. Educational and Psychological Measurement, 30(3), 607–610. https://doi.org/10.1177/001316447003000308
- Kumar, T. V., Yeruva, A. R., Kumar, S., Gangodkar, D., Rao, A. L. N., & Chaturvedi, P. (2022). A new vehicle tracking system with R-CNN and Random Forest Classifier for Disaster Management Platform to Improve Performance. In 2022 2nd International Conference on Technological Advancements in Computational Sciences (ICTACS), 797–804, https://doi.org/10.1109/ICTACS56270.2022.9988024.
- Li, J., Cheng, H., Guo, H., & Qiu, S. (2018). Survey on artificial intelligence for vehicles. Automotive Innovation, 1, 2–14. https://doi.org/10.1007/s42154-018-0009-9
- Lindström, J., & Fjeld, D. (2014). A process perspective on the timber transport vehicle routing problem. Journal of Green Engineering, 4(4), 291–306
- Lu, E., & Yang, Y. (2019). A hybrid route planning approach for logistics with pickup and delivery. Expert Systems with Applications, 118, 482–492. https://doi.org/10.1016/j.eswa.2018.10.031
- Manrique, S., & Martí-Ballester, C. P. (2017). Analyzing the effect of corporate environmental performance on corporate financial performance in developed and developing countries. Sustainability, 9(11), 1957. https://doi.org/10.3390/su9111957
- Mapira, J. (2017). The Mining Industry in Zimbabwe: Challenges for Sustainable Development. European Journal of Social Sciences Studies, 2(8), 313–329.
- Mathias, H. D., & Foley, S. S. (2020). A parallel two-stage genetic algorithm for route planning. Proceedings of the 2020 Genetic and Evolutionary Computation Conference Companion, ACM, https://doi.org/10.1145/3377929.3398116
- Mendes, N., & Iori, M. (2020). A Decision Support System for a Multi-trip Vehicle Routing Problem with Trucks and Drivers Scheduling. Proceedings of the 22nd International Conference on Enterprise Information Systems. 1, 339–349. https://doi.org/10.5220/0009364403390349
- Mhlanga, S., Mbohwa, C., & Pretorius, J. H. (2013). Transportation demand analysis of coal from Hwange coal fields. In Proceedings of the World Congress on Engineering, London, United Kingdom, 1,1–6.
- Mining Zimbabwe. (2021). Diamond Mining in Zimbabwe. https://miningzimbabwe.com//diamond-mining-in-zimbabwe/
- Mohapatra, A., & Tripathy, S. (2018). A Critical Review of the use of Fuel Cells Towards Sustainable Management of Resources. IOP Conference Series: Materials Science and Engineering, 377, 12135. https://doi.org/10.1088/1757-899x/377/1/012135
- Morgan, W., & Hirsch, P. (2013). Botswana: Diamonds, development, and democracy. The Journal of Modern African Studies, 51(1), 109–132.
- Mounika, J., Charanjit, N., Saitharun, B., & Vashista, B. (2021). Accident Alert and Vehicle Tracking System using GPS and GSM. Asian Journal of Applied Science and Technology, 5(2), 81–89. https://doi.org/10.38177/ajast.2021.5211
- Muchaendepi, W., Mbohwa, C., & Kanyepe, J. (2018). Intelligent Transport Systems and its Impact on Performance of Road Freight Transport in Zimbabwe. 2018 IEEE International, https://doi.org/10.1109/IEEM.2018.8607409.
- Munuhuwa, S., Govere, E., Chibaro, M., Chikwere, D., & Kanyepe, J. (2020). Green Fleet Management Practices in Public Service Delivery by Urban Councils: Case of Makonde District in Mashonaland West Province of Zimbabwe. Journal of Economics & Sustainable Development, 11(10), 165–175. https://doi.org/10.7176/jesd/11-10-20
- Musau, E., Namusonge, G., & Ngeno, J. (2017). The Effect of Transport Management on Organizational Performance Among Textile Manufacturing Firms in Kenya. The International Journal of Academic Research in Business & Social Sciences, 7(11). https://doi.org/10.6007/ijarbss/v7-i11/3542
- Ngumbi, D. N. (2016). A model for vehicle tracking and monitoring systems adoption in the Kenya Police Service (Doctoral dissertation, Strathmore University).
- Ngwenya, B., & Busangavanye, W. (2016). Viability challenges and their effect on the performance of gold mining companies in Zimbabwe (2010-2014): A case study of Zimbabwe mining development corporation (ZMDC). International Journal of Original Research, 2(2), 76–90.
- Nha, V. T., Djahel, S., & Murphy, J. (2012). A comparative study of vehicles’ routing algorithms for route planning in smart cities. 2012 First International Workshop on Vehicular Traffic Management for Smart Cities (VTM), 1–6. pp. https://doi.org/10.1109/VTM.2012.6398701.
- Pak, Y. J., & Mun, K. H. (2022). A Practical Fuel Consumption Minimizing Vehicle Routing and Scheduling Problem in Small and Medium Cities and Variable Neighborhood Search. SSRN Electronic Journal. https://doi.org/10.2139/ssrn.4090731
- Ponto, J. (2015). Understanding and Evaluating Survey Research. Journal of the Advanced Practitioner in Oncology, 6(2), 168–171. https://doi.org/10.6004/jadpro.2015.6.2.9
- Sesay, S., & Mattia, G. (2015). Diamond mining in post-conflict Sierra Leone: Challenges and expectations. Resources Policy, 43, 98–104. https://doi.org/10.1016/j.resourpol.2014.11.002
- Shui, X., Zuo, X., Chen, C., & Smith, A. E. (2015). A clonal selection algorithm for urban bus vehicle scheduling. Applied Soft Computing, 36, 36–44. Elsevier BV. https://doi.org/10.1016/j.asoc.2015.07.001
- Siddiqui, A. W., & Verma, M. (2015). A bi-objective approach to routing and scheduling maritime transportation of crude oil. Transportation Research, Part D: Transport & Environment, 37, 65–78. https://doi.org/10.1016/j.trd.2015.04.010
- Sinha, A. K. (2020). Driving Business Performance [ Achieving Successful Business Outcomes, 129-144]. Productivity Press. https://doi.org/10.4324/9781003009399-10
- Sirina, N., & Zubkov, V. (2021). Transport Services Management on Transport and Logistic Methods. Transportation Research Procedia, 54, 263–273. https://doi.org/10.1016/j.trpro.2021.02.072
- Snyman, S. (2019). The political ecology of diamond mining in Namibia. The Extractive Industries and Society, 6(4), 1094–1102. https://doi.org/10.1016/j.exis.2019.06.010
- van Jaarsveld, L., Heyns, G., & Kilbourn, P. (2013). Logistics opportunity costs: A mining case study. Journal of Transport and Supply Chain Management, 7(1), 11. https://doi.org/10.4102/jtscm.v7i1.120
- Van Zandt, J. R. (1992). A genetic algorithm for search route planning. United States Air Force. ESD TR-92-262
- Vareias, A. D., Repoussis, P. P., & Tarantilis, C. D. (2019). Assessing Customer Service Reliability in Route Planning with Self-Imposed Time Windows and Stochastic Travel Times. Transportation Science, 53(1), 256–281. https://doi.org/10.1287/trsc.2017.0748
- Waiyaki, E. C. (2013). leveraging technology for business fleet applications: a case study of fleet management system implemented in Kenya power & lighting company limited ( Doctoral dissertation).
- Wang, X., & Zhu, D. (2012). A Fast Route Planning Algorithm. 2012 Spring Congress on Engineering and Technology, 1–4. https://doi.org/10.1109/SCET.2012.6341969
- Wibowo, S. (2013). A new sustainability index for evaluating the sustainability performance of mining companies. In 2013 Sixth International Symposium on Computational Intelligence and Design, Hangzhou, China,1, 185–188. https://doi.org/10.1109/ISCID.2013.53
- Wijetunge, W. A. D. S., & Pushpakumari, M. D. (2014). The Relationship between Strategic Planning and business Performance: An Empirical Study of Manufacturing SMEs in Western Province in Sri Lanka. Kelaniya Journal of Management, 3(1), 23–41. https://doi.org/10.4038/kjm.v3i1.7476
- Xu, J., & Kelly, J. P. (1996). A Network Flow-Based Tabu Search Heuristic for the Vehicle Routing Problem. Transportation Science, 30(4), 379–393. https://doi.org/10.1287/trsc.30.4.379
- Yaping, Q., & Bossman, M. (2021). Logistics and Supply Chain Management Efficiency Strategy for Ghana’s Mining Industry. European Journal of Business and Management Research, 6(2), 193–202. https://doi.org/10.24018/ejbmr.2021.6.2.779
- Younis, H., Sundarakani, B., & O’Mahony, B. (2020). Investigating the relationship between green supply chain management and corporate performance using a mixed method approach: Developing a roadmap for future research. IIMB Management Review, 32(3), 305–324. https://doi.org/10.1016/j.iimb.2019.10.011
- Zimon, G. (2016). Accounting tools vs. logistics costs control in a trading company. Logforum, 12(2), 155–162. https://doi.org/10.17270/J.LOG.2016.2.5
- Zinbarg, R. E., Revelle, W., Yovel, I., & Li, W. (2005). Cronbach’s α, Revelle’s β, and Mcdonald’s ωH: Their relations with each other and two alternative conceptualizations of reliability. Psychometrika, 70(1), 123–133. https://doi.org/10.1007/s11336-003-0974-7