Abstract
Many companies have encountered vagueness, uncertainty, complexity, and ambiguity in formulating strategies during the COVID-19 pandemic. Therefore, they urgently require a decision-making mechanism to determine the resources/capabilities to achieve a competitive advantage in external environmental change. This research aims to discover publications in RBT, particularly the decision-making mechanism. The review highlighted the novelty of the decision-making mechanism of dynamic capability in RBT that the researchers had never previously reviewed. This review research enabled a systematic literature review (SLR) method embedded with bibliometric and systematic mapping study (SMS) analysis. The results provided 27 final publications, highlighting three keywords (dynamic capabilities, decision making, and enterprise resource management) and Teece and colleagues’ paper as the center of reference. The publications revealed that the decision-making mechanism covered a complex context, big data analysis and multi-criteria decision-making, dynamic, updated characteristics mechanism, and subject fields. Moreover, the publications were generally conducted in common industries, and several decision-support tools were found in the RBT. In summary, publications still need to provide more information to present the decision-making mechanism in enterprise resource management. Therefore, the proposed theoretical framework and conceptual decision-making model should be developed to follow a dynamic environment.
PUBLIC INTEREST STATEMENT
The decision-making of resources becomes a significant role in strategic formulation. Firms must prepare their resources and capabilities to support their strategy. A dynamic environment forces firms to modify their strategy to win the competition. COVID-19 has reminded us that the directors must quickly decide to anticipate the complex situation. Hence, directors must have a mechanism to decide on resources and capabilities to support a firm’s strategy. The decision-making mechanism should be developed to become a decision-making model for directors. The decision-based resource should continuously be developed to strengthen the firm in dynamic environmental change.
1. Introduction
A company will strive to exploit its resources to implement a strategy to win a competition. A significant inquiry arises in selecting and configuring the best resources to achieve performance (James & Joseph, Citation2015). Determining resources is like managing the players in a squad team to prepare for a match. It needs managerial concept-based resources to approach the strategy plan (Sirmon et al., Citation2011). Building a team requires time and processes to accumulate and configure the players. Likely combining the firm’s resources, the manager has to scrutinize the characteristics of resources that will contribute to the firm’s performance. Decision-making for combining resources becomes significant in strategic planning to leverage a company’s core competencies to exceed its average return (Donnellan & Rutledge, Citation2019).
The COVID-19 pandemic has provided an important reminder for companies to be ready to make quick decisions to resolve the problem. For instance, banks encountered vagueness, uncertainty, complexity, and ambiguity when the end of the outbreak was unknown (Henry, Citation2020). Based on McKinsey’s Global Banking Annual Review, global banks have reserved $1.15 trillion for loan-loss provisions during the COVID-19 pandemic (McKinsey, Citation2020). The banks’ circumstances have occurred barely in the whole countries of the world, and the banks strive to overcome lending problems during the pandemic situation (Ҫolak & Öztekin, Citation2021). In another sample case in Indonesia, Bank Negara Indonesia (BNI) was late in anticipating the pandemic situation of COVID-19 that BNI recorded the most significant decline in earnings and suffered a loss of 41.54% compared with that in Semester 1 2019 (BNI, Citation2021). Based on interviews with strategic plan officers, they realized that BNI suffered the most significant losses in loan services. BNI lagged in preparing resources that human mobility restrictions, including quarantine, are obstacles for people to do in-person transactions while virtual transactions increased during the pandemic. Meanwhile, non-financial institutions faced this problem similarly, such as airline companies, which dropped revenue by 60 percent in 2020 (McKinsey, Citation2022).
The number of passengers declined dramatically when many countries applied the human mobility restriction policy. This situation has paralyzed the airlines. Resources become undervalued when core business competencies do not run well. With their available resources, airline companies struggled to maintain their operations even though the flight should tightly follow the health protocol standard. Airlines had to ensure sufficient resources to cover the new strategy during the pandemic. Hence, they acknowledged needing a decision-making mechanism to configure resources to anticipate rapid environmental change.
Teece (Citation2014) introduced the managerial decision constituting a dynamic decision-making mechanism to orchestrate a firm’s resources to achieve competitive advantage. Orchestrating resources is part of the dynamic capability where managers or bank directors are responsible for selecting resources (Barney & Hesterly, Citation2015) and developing their capabilities (Helfat & Peteraf, Citation2015) in environmental changes. In the case of banking, the bank should have a quick decision-making mechanism of resource strategy because the banking system tends to be sensitive to external environmental changes. However, banks need assistance adjusting resource configuration to follow the strategy changes.
Resource Based-View (RBV) is a theory that underlies the combining firm’s resources to achieve a competitive advantage (Barney & Hesterly, Citation2015). Many scholars have revealed the RBV concept in its early emergence, likely Dierickx and Cool (Citation1989), Lippman and Rumelt (Citation1982), Penrose (Citation1959), and Wernerfelt (Citation1984). However, Barney (Citation1991) prominently developed the RBV shape by defining the resource characteristics of competitive advantage. Hart (Citation1995) described the interconnect RBV-nature environment as a Natural-Resource-Based View (NRBV), and Grant (Citation1996) articulated a firm’s resources as a knowledge-based view. Therefore, Teece et al. (Citation1997) introduced dynamic capabilities based on RBV ideas to increase competitive advantage. RBV experiences evolvement until today, called resource-based theory (RBT). The focus of RBT elaborates on the resources that have the characteristics of valuable, rare, inimitable, and non-substitutable (VRIN), which will bring the firm to win the competition (Barney, Citation1991), revisited by Barney and Wright (Citation1998) to become valuable, rare, inimitable, and exploited by an organization (VRIO). Directors require these characteristics to determine the resources that support a bank’s strategy. When formulating a strategy, the bank’s directors must consider the resource’ features that will be exploited and combined. Hence, the director must have the micro-foundations of dynamic capability: to sense opportunities through constantly scanning, searching, and exploring the resources; seize the opportunities through resource investment decisions and allocation to increase return advantage and leverage bundle resources to create the product and services; and transform resources and capabilities configuration to create a competitive advantage for the product or services (Teece, Citation2007).
Decision-makers should focus on the context of environmental change. They can use the Cynefin Framework to help them choose an appropriate choice (Gorzeń-Mitka & Okręglicka, Citation2014; Snowden & Boone, Citation2007). The four contexts of the Cynefin Frameworks are simple, complicated, complex, and chaotic. Simple and complicated contexts generally consider an order in which facts can determine the correct answer and cause-effect relationship. Complex and chaotic are unordered contexts where no cause-effect relationship and an emergence pattern determine the decision-making process. The complex context still provides at least one answer in a significant change, likely a pandemic.
Meanwhile, the chaotic context assumes a rapid response to sudden environmental changes, there is no manageable pattern, and the correct answer is pointless. According to the COVID-19 pandemic, firms existed in a complex context where they could still find the emergence pattern but no cause-effect relationship. They must shape a new decision-making model to escape from complex and uncertain situations and make the right decisions. A decision-making model can draw the decision process flow in the decision-making mechanism of the resource configuration.
Furthermore, the overall operative context of the decision-making model is required to determine the appropriate action to make the correct decision. The Cynefin Framework divides the context of a decision-making model into four domains: Simple, Complicated, Complex, and Chaotic. Simple and Complicated are addressed for an ordered universal situation, cause-and-effect relationship, and correct answers based on facts. Whereas, Complex and Chaotic are contexts for unordered situations with no immediate apparent cause-and-effect relationship and correct answers determined by emerging patterns. A simple context addresses what the leader considers to conduct sense, categorize, and respond in decision-making. Decision-makers must carry out sense, analyze, and respond when the decision-making process is complicated. The Complex context requires probe, sense, and response from the decision-maker to overcome the situation change suddenly. Moreover, a chaotic context imposes the leader to act, sense, and respond to the situation immediately to determine the appropriate decision.
Priem and Butler’s critiques mentioned that RBT cannot report non-falsifiable, has no practical significance, neglects environmental factors, and has unclear mechanisms (Kraaijenbrink et al., Citation2010). Countering Priem and Butler’s critiques, Barney (Citation2001) refuted RBT as a tautology. He also explained RBT’s practical contributions and proposed using dynamic methods to conduct empirical studies. Concerning Barney’s disclaimers, there need to be more RBT implementations that neglect environmental factors and unclear mechanisms. Newbert (Citation2008) introduced the logical mechanism of RBT in which the resources-capabilities combination, undergirded by valuables and rareness, delivers a competitive advantage and increases performance.
Nevertheless, decision-making requires a precise mechanism to determine and combine resource capabilities for overcoming strategy changes. Teece is one of the scholars who developed managerial decisions in a dynamic capability framework. By observing the flow of the framework, Teece’s (Citation2014) dynamic capabilities framework still needs to be improved to draw on the decision-making mechanism of managerial decisions in resource orchestration. There is a space between resource capabilities and strategies that a resource-based decision process can bridge. Figure illustrates the lack of a decision-making mechanism to fill the gap in the dominant logic of the dynamic capability framework. According to Teece’s framework, a manager will conduct managerial decisions (sensing, seizing, and transforming) to organize dynamic capability and VRIN resources for strategy implementation. The dynamic capability framework’s problem is to organize the combination of resource capabilities to realize the strategy. A firm has ordinary capabilities, ordinary resources, VRIN resources, and dynamic capabilities that must be orchestrated to support the strategy until it provides a competitive advantage. Hence, resource management requires a decision-making mechanism to determine resources and capabilities.
However, the decision-making mechanism of the dynamic capability still needs to be found in RBT publications. Jay Barney, David Teece, Margaret Peteraf, Constance Helfat, and David Sirmon, prominent scholars in RBT and dynamic managerial capability, were inclined to focus on the framework and concept (Zhang et al., Citation2021)—nevertheless, the way of combining resources needed to be exhibited. Moreover, the banking context in RBT literature (Zhang et al., Citation2021) needs to be investigated. In contrast, banking needs resource management to anticipate strategic changes caused by a dynamic environment (Donnellan & Rutledge, Citation2019). The method of resource-based decision, which directors use to determine resources, still needs to be seen in the current literature. Hence, there is a gap regarding the decision-making mechanism of resources in the RBT literature.
This research aims to find the publication in RBT, particularly the decision-making mechanism of dynamic capability, through a literature review. Decision-making for resource configuration is significant for driving strategy implementation. Hence, this research strives to review the previous studies on the decision-making model of RBT research. Furthermore, the mapping literature on the decision-making of resources is essential to build a further model of the decision-making process involving big data analytics that can help banks select their resources for implementing strategies quickly. This literature study finds references to decision-making in banking through the status of decision-making in RBT literature, the decision-making model, dynamic capability in the decision-making process, the availability of big data analytics in decision support systems, and research areas in banking.
The review highlighted the novelty of the decision-making mechanism of dynamic capability in RBT, which has never been reviewed before. Although many scholars have conducted a literature review of decision-making in RBT, the decision-making mechanism constitutes a primary concern. The decision-making mechanism connects the gap between resources/capabilities and decision-making. The new findings expected from this review are the various decision-making mechanisms involved in RBT.
2. Related work
Identifying the decision-making model of RBT in the last three decades is to observe the literature study of Zhang et al. (Citation2021). Following Zhang et al. (Citation2021) study, the RBT evolved in several articles, topics, influential countries, and influential authors, articles, and journals between 1991 and 2020. Their research resulted in the status of RBT research, the evolution of topics in RBT research, and citation burst analysis of RBT research. These research findings described that RBT articles increased, the field of general management still dominated, both countries the United States and the United Kingdom are the epicenters of RBT, and Jay Barney, David Teece, and Birger Wernerfelt are the most influential scholars of RBT research. Nevertheless, the exciting finding exhibited that the most influential publication during the three decades referred to Teece et al. (Citation1997), followed by Priem and Butler (Citation2001) and Peteraf (Citation1993). These insights guide future scholars that RBT research will mainly focus on the dynamic capability topic, distinguishing the linkage of resources, capabilities, core competitiveness, and dynamic capabilities. Critiques of RBT by Priem and Butler (Citation2001) are still incomplete answers and have always been disputed by scholars.
Moreover, Peteraf’s thoughts (Peteraf, Citation1993) on the RBV framework have continually been discoursed by scholars. The insights and future research directions of Zhang et al.’s research are the guidelines to underlie the emergence of configuring resources as this literature review research topic. Table presents the topic formulation. The research agenda comprises five sections: objective, scope, methodology, results, and subsequent direction. The objective, scope, and methodology were developed based on this study’s background. Meanwhile, the results and further directions are utilized for formulating the research questions. This research agenda guides this systematic literature study to determine the criteria for the RBT literature.
Table 1. The insights of RBT evolution article and research agenda
3. Materials and methods
The research methodology of review adopts the procedure of the systematic literature review (SLR) introduced by Khan and Kitchenham (Khan et al., Citation2022; Kitchenham & Charters, Citation2007). The three phases of the systematic literature review procedure are planning, conducting, and reporting. The phases are detailed in the eight steps of the review protocol, which follow the systematic literature review process by Xiao and Watson (Citation2019). Figure shows the methodology of the review, which was developed by combining the methods of Khan et al. (Citation2022), Xiao and Watson (Citation2019), and Zhang et al. (Citation2021). The planning phase involves formulating the research questions and developing the review protocol. In the conducting phase, review planning is executed by searching for literature, screening, quality assessment, data extraction, and data synthesis and analysis. The reporting phase involves documenting the review process, implementation, synthesis, and future research.
According to the gap in RBT literature, this research combines bibliometric analysis and structured mapping study (SMS) to investigate the extent to which RBV research is conducting decision-making in the banking sector. The bibliometric analysis represents the structure of the research area based on essential characteristics (e.g., titles, authors, abstracts, keywords, and references) (Zupic & Čater, Citation2015). Using bibliometrics, researchers can identify RBT research’s dynamic status capability (as the origin of resource decision-making) through country location, prominent authors, and the most interlinked keywords. Country locations exhibit the most remarkable proliferation of dynamic capability and mention the potential place of conducting research. Influential authors portray scholars concerned with dynamic capabilities and potentially investigating decision-making in their research. The levels of interlinked keywords serve as the most influential keywords and determine the decision-making topics in RBT research.
Moreover, SMS generates structured evidence on relevant research topics (Khan et al., Citation2022). The topics of this study were used as criteria for delimiting appropriate literature on RBT. The relevant research in this literature study is in the RBT context, particularly the decision-making mechanism. The researcher manually sorted the final selection literature on RBT to enter the SMS analysis. The SMS analysis extracts the RBT literature regarding the decision-making basis, subject field, industry field, and decision support tools.
3.1. Planning phase
The planning phase consists of Steps 1 and 2, including formulating the research problem and developing and validating the review protocol (Xiao & Watson, Citation2019). Step 1, formulating the research question, was carried out by identifying the insights from previous studies on RBT research. This research chose the study by Zhang et al. (Citation2021) to obtain insights into RBT proliferation over the last three decades. The background of the choice study was that it could completely give the current position of RBT studies regarding research areas, topics, the spread of studies, influential authors, and future directions. Zhang et al.’s study guided RBT scholars to select the area that should be investigated. Step 1 also develops a methodology of review that adopts the systematic literature review (SLR) by Xiao and Watson (Citation2019). Figure describes the review methodology modified from Xiao and Watson’s SLR.
Step 2, developing and validating the review protocol, should reflect all the components of the review the following: (a) research question; (b) search strategy; (c) review criteria of primary study selection; (d) assessment of methodological quality; (e) sketching data extraction strategy; and (f) data synthesis and analysis (Khan et al., Citation2022).
Furthermore, the validation of the review protocol was organized by the research team to increase the rigor study in the decision-making field of RBT (Xiao & Watson, Citation2019). The review protocol must be developed carefully before the conducting phase for providing appropriate research questions. The components of the review protocol are as follows:
a. Research question
Formulating the appropriate research question can be aided by using a pre-mapping review to identify the subtopics of the research problem (Xiao & Watson, Citation2019). In the pre-mapping review, the researcher searched for the initial literature related to the research questions. After obtaining the literature, the researcher began to identify insights related to the research question. The pre-mapping review helps researchers obtain the most out of the topic, or they must be restricted to a particular research question. Table shows the development of the research questions using insight mapping.
Table 2. Research questions developed by Insight mapping
b. Search strategy
A search strategy must be predefined to provide the maximum result in the empirical literature (Khan et al. (Citation2022). The review strategy was adopted by Xiao and Watson (Citation2019) to search for primary studies. Following the research questions, the steps of the search strategy are as follows: (1) determining the channel of literature search, (2) keywords used for search, (3) downloading data and data cleaning, (4) sampling inclusion/exclusion criteria, (5) refining results with additional restrictions, and (6) stopping rule. The explanation of the review steps is exhibited by the following:
(1) Determine channel of literature search
This review utilized both electronic databases, namely SCOPUS and PROQUEST. SCOPUS is a credible database for academic articles: Elsevier, Science Direct, and Emerald. The SCOPUS database can broadly cover of the research areas (Zhang et al., Citation2021). Downloading the electronic database of SCOPUS is relatively easy to be obtained through the Scopus website. Simultaneously, the PROQUEST database can cover articles from the outside journal publisher SCOPUS.
(2) Keywords used for the search
Keywords were derived from research questions that should address the findings (Xiao & Watson, Citation2019). This research utilizes keywords related to RBV, RBT, dynamic capability, resource management, resource orchestration, and decision-making. The search strings use Boolean “AND” and “OR” to search the literature in the SCOPUS database (Xiao & Watson, Citation2019, p. 104).”AND” engages the main terms, whereas “OR” includes a synonym.
(3) Download data and data cleaning
SCOPUS and PROQUEST databases are derived from the SCOPUS website with the link www.scopus.com. Data mining of the SCOPUS repository was conducted by generating the keywords used for the search based on the article title, abstract, and keywords. Keywords for the search should be appropriate for the review (Xiao & Watson, Citation2019). The downloading database is an automated screening search using the keywords “resource”, “dynamic capability”, and “decision making”. The following process is data cleaning, ensuring the database contains the same meaning for different keywords. For example, “decision making” is the same word as “decision-making”, or “resource-based view is similar to “resource-based view”.
(4) Sampling inclusion/exclusion criteria
Sampling inclusion/exclusion criteria constitute the narrowing-down process to provide a repository corresponding to the research questions (Khan et al., Citation2022; Xiao & Watson, Citation2019). The sampling limitation was carried out in two ways: screening and eligibility. The screening process was article selection based on review criteria. The review criteria consist of period, area, keywords, and language. Eligibility is assessing the full text of the literature based on a review of specific topics. The eligibility process traces the literature on dynamic capability in RBT’s decision-making process. This method is conducted by manually selecting that scanning the literature using the keywords “dynamic capability”, “resource management”, and “resource orchestration”. The sampling inclusion/exclusion criteria provided the review results as primary studies.
(5) Refining results with additional restrictions
Refining the results for completing primary studies emits irrelevant articles and adds the potential article of excluding results. This step uses additional restrictions and complements the context of the review to address the research questions (Xiao & Watson, Citation2019).
(6) Stopping rule
The final step of the search strategy is the stopping rule, which mentions the ending of the search strategy (Xiao & Watson, Citation2019). The final results were obtained from a total of primary studies. The researcher states whether the primary studies have been confirmed for synthesis and analysis.
(c) Review criteria and primary studies selection
The inclusion and exclusion criteria determined the retrieved primary studies according to the relevant results. The review criteria were developed based on the purpose of the research. The first inclusion criteria were the title, abstract, and keywords relevant to research objectives. Exclusion criteria were applied when the studies were unsuitable for the research objectives. Table presents the inclusion and exclusion criteria for this review.
Table 3. Inclusion/Exclusion criteria
(d) Assessment of methodology quality
Quality assessment is not crucial for descriptive reviews but is essential for generalization (Xiao & Watson, Citation2019). There are no formal quality standards for most reviews; hence, the assessment methodology quality guideline is determined by these criteria. An independent quality assessment can be carried out by joint reviewers who set the criteria. The quality criteria that evaluate the selection of studies include rigor, credibility, and relevance (Khan et al., Citation2022). Reviewers work together to ensure that the methodology can provide relevant literature.
(e) Sketching data extraction strategy
Data extraction identifies article characteristics, including title, year of publication, source, authors, co-authors, keywords, countries, references, citations, funding agencies, and other details (Khan et al., Citation2022). This review uses two strategic approaches to sketch data extraction: narrative and scope. The narrative review identifies the key topics of the research questions and serves as a descriptive account of the evidence supporting the conclusions. In comparison, the scoping review extracts information on studies organized by year, country, field, and other areas.
(f) Data synthesis and analysis
The data synthesis and analysis were conducted using| quantitative and qualitative techniques. The quantitative technique performs the bibliometric analysis to synthesize the studies related to scoping reviews, such as period, location, influential authors, and other areas. Furthermore, the qualitative technique carries out the SMS to synthesize the studies regarding the relevant topics.
3.2. Conducting phase
The conducting phase constitutes six steps following the two steps of the planning phase. The conducting phase comprises from Step 3 to 7 of the review methodology. Steps 3 and 4 of the conducting phase were separated into four major sections: automated search, screening, eligibility, and inclusion (Khan et al., Citation2022; Xiao & Watson, Citation2019). In Step 3, the literature database was obtained by an automated search using the SCOPUS and PROQUEST. Figure illustrates the automated search process. The literature database was searched using periods, keyword strings, subject areas, and the English language. The search process used search strings of keywords, namely resource, dynamic capability, and decision making, typed in the search box. The strings of search directions were TITLE-ABS-KEY (“RESOURCE” AND “DYNAMIC CAPABILITY” AND “DECISION MAKING”) for SCOPUS. Meanwhile, the script direction is “RESOURCE” AND “DYNAMIC CAPABILITY” AND “DECISION MAKING” for the PROQUEST search. This process yielded 184 articles, which consisted of 98 articles from SCOPUS and 86 articles from PROQUEST.
Step 4 screened the results by removing duplication, review, outside reputable publishers, and not in English. Figure illustrates the conducting phase for searching the primary studies. The eligibility of Step 4 was sorting the literature with inclusion/exclusion criteria based on title, type, abstract, and resolution meeting of doubtful literature. The first inclusion/exclusion criteria, based on title, type, and abstract, obtained 20 articles, removed 107 articles, and gained 45 doubtful articles. Subsequently, the following selection assessed 45 doubtful articles and included two articles. Step 4 resulted in the primary studies with a total of 22 articles.
Meanwhile, Steps 5, 6, and 7 were conducted into three sections, namely manual search, screening, and eligibility, and included (Khan et al., Citation2022; Xiao & Watson, Citation2019). The manual search process is exhibited in . Step 5 was the quality assessment of the primary studies conducted by a manual search. The manual search strategy is divided into snowballing and author searching. The snowballing technique conducted a backward process to search the references of articles and used a forward process for articles that cited primary studies. The results of the snowballing technique were obtained from 2,992 articles.
In comparison, the author searched and browsed articles published by the authors of the primary studies. A total of 5,027 articles were identified. Step 6 elaborates on the data extraction process, including inclusion/exclusion criteria based on the title, type, abstract, and full text. The screening and eligibility sections examined both snowballing and author search results, which provided three articles, and two articles, respectively.
The final process of the conducting phase is Step 7, which synthesizes 22 primary study articles, three snowballing search articles, and two author search articles. The total number of articles included in the final selection was 27. The final selection consisted of 6 articles published in 2022; 5 articles published in 2021; 4 articles published in 2019; 3 articles published in 2020 and 2017; and 1 article published in 2018, 2016, 2014, 2011, 2010, and 2007. This statistical number indicates that research on decision-making based on a dynamic capability view has relatively increased from 2007 to 2022. The analysis of the publication number trend indicates that researchers are interested in beginning the investigation of the decision-making mechanism on the dynamic capability and RBV.
Furthermore, the number of publishers of the final article selection was found: 8 articles from Elsevier; 7 articles from Emerald; 3 articles from Inderscience; and 1 article from each Springer, MDPI, De Gruyter Open, Allied Business Academies, University of Minnesota, the University of California Press, Decision Sciences Institute, and Oxford University Press. This finding elaborates that the final selection comprised articles published by reputable publishers.
3.3. Reporting phase
The reporting phase denotes the final stage of SLR, which encompasses documenting the review process, implementation, synthesis, and future research. Step 8 of the methodology manifests the reporting phase, which employs bibliometric analysis and SMS to document the review process. The reporting phase also provides a discussion of the review results.
4. Results and discussions
The two analyses for reporting the review are as follows:
a. Bibliometric analysis
The bibliometric analysis provided the number of documents in each country, the country’s citation concerning the topic, co-occurrence, and link strength of the keyword, and the number of articles that cited the final selection. The results of the bibliometric analysis are leveraged to answer RQ1 regarding the status of the literature on the decision-making mechanism of dynamic capability. The bibliometric analysis also assists in answering RQ2 concerning publication coverage in the value creation area. This research conducted four terms of the bibliometric analysis, as follows:
(1) The number of documents in each country
The highest number of documents in the decision-making mechanism topic om the RBT literature was from the United Kingdom, with eight articles. The United States was in the second position with six articles. Germany, India, and Italy placed third, fourth, and fifth positions with 5, 4, and 3 articles, respectively. Turkey and Iran provided two documents. Single documents were found in Pakistan, Ireland, Norway, Denmark, France, Australia, Czech Republic, Poland, Finland, Latvia, Taiwan, Brazil, and Chile. Figure depicts the spread of documents in the decision-making topic of the RBT literature worldwide.
(2) Country’s citation with regard on the topic
The United States was the most-cited country, with 1176. This number of citations was dominant among all countries. The other positions were the United Kingdom and Pakistan, ranking second and third, with 276 and 125 citations, respectively. The next cluster was placed by Turkey, Ireland, and Norway, with 98, 88, and 88 citations, respectively. Italy, Iran, and India were clustered with 77, 77, and 75 citations, respectively. Whereas Denmark, France, Australia, and Germany achieved under sixty citations. The fewest citations were found in the Czech Republic, Poland, Finland, Latvia, Taiwan, Brazil, and Chile. The number of countries’ citations is exhibited in Figure . This finding is not surprising as the United Kingdom and the United States have become the reference for RBT research, particularly the decision-making mechanism topic in the dynamic capability subject area. Surprisingly, Pakistan and Turkey emerged as countries with many citations. These insights indicate that research on decision-making mechanisms has developed exceed Europe and America. India dominates research on RBT in Asia, and emerging countries, such as Pakistan and Turkey, have become evidence of RBT proliferation.
(3) Co-occurrence and link strength of keywords
Co-occurrence keywords point out the number of documents in which keywords occur together. The analysis used a minimum of two keyword occurrences. Co-occurrence analysis provided 198 co-occurrences of keywords and 22 thresholds. The results mentioned that “dynamic capabilities” and “decision making” were the dominant co-occurrence keywords. Whereas “enterprise resource management” emerged as the third keyword with seven times in co-occurrences. “resource-based view” was the other keyword six times. The keywords with three times co-occurrences were big data, competitive advantage, COVID-19, industrial management, and micro-foundations. The twice co-occurrences of keywords consisted of agility, analytical hierarchy process, big data analysis, data analytics, debate, emerging economies, emerging markets, entrepreneur, information management, multi-criteria decision-making, strategy, supply chains, and uncertainty. Table lists the number of co-occurrences and the link strength keywords.
Table 4. Co-occurrence and link strength of keywords
Meanwhile, the link strength of the RBT literature in decision-making topics showed that “dynamic capabilities” had the strongest interconnection among keywords. The keyword “decision making” was the second most vital link, followed by “enterprise resource management”, “industrial management”, “big data”, big data analytics, and “analytical hierarchy process, as different sequences. An interesting keyword was found, namely “enterprise resource management”, which had high co-occurrences and many inter-keyword links. A visualization of the link strength is shown in Figure .
(4) The number of citations of the final selection
The number of citations indicates that an article influences the burst analysis of decision-making mechanisms in the value-creation area. Table lists the number of final selection citations. The statistical data of citations are leveraged to answer RQ2 regarding articles covering resource management decision-making mechanisms. Teece et al. (Citation2016) paper is the most cited RBT literature publication, with 800 citations. Teece et al.‘s paper, “Dynamic Capabilities and Organizational Agility: Risk, Uncertainty and Entrepreneurial Management in the Innovation Economy,” strongly influences researchers to develop a decision-making mechanism topic of dynamic capability. This paper provides fundamental knowledge for many researchers to investigate dynamic capability from various points of view.
Table 5. The number of article’s citations
In addition, Allred et al. (Citation2011), Conboy et al. (Citation2020), Shamim et al. (Citation2019), and Yasmin et al. (Citation2020) are the other primary references for researchers. Allred et al. (Citation2011) pointed out how firm and supply chain resources can be configured to achieve unique advantages and superior performance. Conboy et al. (Citation2020); Shamim et al. (Citation2019), and Yasmin et al. (Citation2020) highlighted the role of big data analytics in assisting the decision-making process. Based on previous influenced papers, the decision-making mechanism of dynamic capability frequently discusses the micro-foundations of dynamic capability, supply chain, and big data analytics.
Meanwhile, the other articles on the final selection, which have fewer than 50 citations, elaborate on the specific context of decision-making, such as supply chain (Bathke et al., Citation2022; Čirjevskis & Tvaronavičienė, Citation2017; Mathivathanan et al., Citation2017), IT (Information Technology) capability (Ayabakan et al., Citation2017; Collan et al., Citation2014; Kozak et al., Citation2021; Yadav et al., Citation2021); the role of dynamic capability (Agostini & Nosella, Citation2021; Bucak et al., Citation2022; Hasegan et al., Citation2018; Kay, Citation2010); micro-foundations of dynamic capability (Bhardwaj et al., Citation2022; Farago et al., Citation2019; Kunc, Citation2007; Möller & McCaffrey, Citation2021), big data analytics (Dahiya et al., Citation2022; van Rijmenam et al., Citation2019; Yan et al., Citation2022; Zeng & Khan, Citation2019), SMEs (Jafari-Sadeghi et al., Citation2021, Citation2022), and decision support tool (Singh & Samuel, Citation2020).
b. Systematic mapping study of decision making mechanism
The review used the SMS to identify the final selection of papers that addressed the decision-making mechanism. The SMS analysis provided the results regarding publication year, context of decision-making, processing strategy, decision-making characteristics, decision mechanism in the subject field, industry field, and decision-making tool. The context, processing strategy, and mechanism characteristics were analyzed to determine the answer to RQ3. The investigation of decision mechanisms based on the subject field was leveraged to answer RQ4. The industry field analysis provided the findings for answering RQ5. Subsequently, RQ6 was answered by investigating the decision support tool. Table describes the SMS of the decision-making mechanism in the RBT literature.
Table 6. SMS analysis of paper selection
Based on the Cynefin Framework, the decision-making mechanism is highlighted regarding the situation, decision approach, and decision support (Snowden & Boone, Citation2007). Hence, this review focuses on three main aspects of decision-making: the approach to context (Snowden & Boone, Citation2007), processing strategy (Yazdi et al., Citation2020), and characteristics of the mechanism (Roberts & Wernstedt, Citation2019)—the findings of decision-making identification aimed to answer RQ3. The context of the decision-making mechanism was found in 2 papers for simple, 9 for complicated, and 16 for the complex. These findings indicate that most decision-making mechanism literature on dynamic capability addresses complex contexts. The complex context parallels the tenets of dynamic capability in that quick decision-making capability based on resources is required to respond to the dynamics of the environment (Barney & Hesterly, Citation2015; Helfat & Peteraf, Citation2015; Sirmon et al., Citation2011; Teece, Citation2014). These results also answer Eisenhardt and Martin’s critiques that RBT’s dynamic capabilities come from managers’ abilities to allocate valuable resources in moderately dynamic and high-velocity market contexts (Eisenhardt & Martin, Citation2000).
Furthermore, the processing strategy shows that the decision-making mechanism utilizes BDA, MCDM, and system dynamics to provide a decision. BDA was leveraged by Ayabakan et al. (Citation2017), Conboy et al. (Citation2020), Dahiya et al. (Citation2022), Kozak et al. (Citation2021), van Rijmenam et al. (Citation2019), Shamim et al. (Citation2019), Yan et al. (Citation2022), and Zeng and Khan (Citation2019). They optimized the data-driven role to select many alternative decisions for a firm’s strategy. Ten papers generated MCDM in the decision-making mechanism investigation. One article used content analysis as a qualitative mechanism to identify a manager’s dynamic decision capability (Farago et al., Citation2019). Hasegan et al. (Citation2018) leveraged the system dynamically for a decision-making mechanism for operational decisions, namely Dynamic Performance Measurement System (DPMS). At the same time, seven articles did not describe researchers’ processing strategies. The results show that the processing strategy of decision-making mechanisms in RBT literature commonly utilizes MCDA and BDA.
Table lists the characteristics of real-time, dynamic, adaptive, and iterative mechanisms. Several articles adopt all the characteristics of the decision-making process; that is, Agostini and Nosella (Citation2021), Conboy et al. (Citation2020), Kozak et al. (Citation2021), van Rijmenam et al. (Citation2019), Yan et al. (Citation2022) and Zeng and Khan (Citation2019). Dahiya et al. (Citation2022), Möller and McCaffrey (Citation2021), and Shamim et al. (Citation2019) have all the natures of a mechanism unless an iterative process. Meanwhile, the other papers have incomplete natures of the decision-making process with three natures, such as Kay’s (Citation2010) article (natures: dynamic, adaptive, and iterative) and Dahiya et al. (Citation2022) article (natures: real-time, dynamic, and non-iterative). Bhardwaj et al. (Citation2022) and Yadav et al. (Citation2021) carried out two characteristics of the mechanism (dynamic, adaptive). Eleven papers had only one nature (adaptive) (Allred et al., Citation2011; Ayabakan et al., Citation2017; Bathke et al., Citation2022; Bucak et al., Citation2022; Čirjevskis & Tvaronavičienė, Citation2017; Farago et al., Citation2019; Jafari-Sadeghi et al., Citation2021, Citation2022; Mathivathanan et al., Citation2017; Singh & Samuel, Citation2020; Yasmin et al., Citation2020). These results denote that the characteristics of the decision mechanisms are dominated by an adaptive nature instead of real-time, dynamic, and iterative. Therefore, dynamic capability decision-making must address all characteristics to anticipate environmental changes.
This review examined the decision-making mechanism of dynamic capability in the subject field in response to Priem and Butler’s critiques (Priem & Butler, Citation2001), particularly regarding the unclear mechanism of RBT. The subject field addressed the context in which dynamic capability was enabled in decision-making. Industry field items have been utilized to analyze the flexibility of decision-making mechanisms in various sectors (Helfat & Winter, Citation2011). Dynamic capability’s decision-making mechanism can manifest as a decision-support tool for practical significance (Sirmon et al., Citation2011).
Subsequently, the decision-making mechanism of dynamic capability in the subject field shows that the findings are related to the decision-making processing strategy. The findings of the subject field analysis were used to answer RQ4. For instance, BDA capability has been commonly used to seek a source of competitive advantage (Dahiya et al., Citation2022), such as resource configuration (Conboy et al., Citation2020; Zeng & Khan, Citation2019), organizational ability (van Rijmenam et al., Citation2019), and data-driven decision-making (Ayabakan et al., Citation2017; Collan et al., Citation2014; Kozak et al., Citation2021; Möller & McCaffrey, Citation2021; Shamim et al., Citation2019). The dynamic system model and MCDM emphasized decision-making in operational management, such as the hybrid mental-computer model (Yan et al., Citation2022), supply chain (Bathke et al., Citation2022; Bucak et al., Citation2022; Yadav et al., Citation2021), managerial capabilities (Farago et al., Citation2019; Jafari-Sadeghi et al., Citation2021 & Citation2011), strategy barriers (Singh & Samuel, Citation2020), and organizational capabilities (Collan et al., Citation2014; Mathivathanan et al., Citation2017; Yasmin et al., Citation2020). In contrast, the other papers that did not describe processing strategies addressed the subject fields: logical structure of competitive advantage (Čirjevskis & Tvaronavičienė, Citation2017), organizational agility (Teece et al., Citation2016), interfirm resources (Allred et al., Citation2011), critiques of dynamic capability (Kay, Citation2010), and dynamic managerial capabilities (Kunc, Citation2007). These findings show that a decision-making mechanism of dynamic capability exists in subject fields. Hence, the decision-making mechanism in RBT research must clarify the standard process that accommodates the view of dynamic capability.
Industry field analysis assists this review in revealing which industries have been involved in the decision-making of dynamic capability. According to the results, maritime, technology, social enterprises, agri-food, research, apparel retail, cinema, and manufacturing sectors have generated decision-making regarding dynamic capability in RBT publications. Decision-making research is mainly carried out in a common field, not specified in specific industries. Thus, the decision-making topic of RBT research covers the common industries that firms can adopt in their decisions. The findings of the industry field analysis can be leveraged to answer RQ5.
Moreover, the last analysis of SMS is a decision-support tool to assist in the decision-making mechanism of dynamic capability. The result shows the decision support tools that researchers used in their works as well as IBM’s COGNOS, SAP Business Objects, Oracle Hyperion, System Dynamics Modelling, Analytical Hierarchy Process (AHP), Variable Returns to Scale (VRS) Integrated Fuzzy AHP and TOPSIS, Mann—Whitney U-test, Integrated DEMATEL and Analytical Network Process (ANP), Decision-Making Trial and Evolution Laboratory (DEMATEL), Integrated Interpretative Structural Modelling (ISM) and Fuzzy DEMATEL (F-DEMATEL), Integrated ISM and Matrix-based Multiplication Applied to a Classification (MICMAC), Machine Learning & Swarm Algorithms, Integrated Intuitionistic F-DEMATEL (IF-DEMATEL) and ANP, Simple Additive Weighting (SAW), System Dynamics Modelling, and Variable Returns to Scale (VRS). The researchers mostly leveraged DEMATEL as a decision support tool based on the results. In contrast, Kozak et al. (Citation2021) have only utilized machine learning to make prescriptive recommendations for current and future actions. Database applications and IBM’s COGNOS, SAP Business Objects, and Oracle Hyperion constitute decision-support tools provided by third-party companies (Dahiya et al., Citation2022). AHP and ANP are commonly used as decision support tools to make firm decisions. These results indicate that decision-support tools still use data-driven tools to make decisions. The findings of the decision support tool answered RQ6.
c. Implementation and synthesis
Implementation of the review involves executing the phases and steps of methodology to search the literature (Xiao & Watson, Citation2019). The review methodology consisted of three phases and eight steps. The implementation of this review is as follows:
4.8. Planning phase
The planning phase is a review preparation that starts by formulating the problem to develop and validate the review protocol. The planning phase consisted of two steps: Step 1 and Step 2. Step 1 identifies the research questions and selects the methodology for the review, and Step 2 develops and validates the review protocol. The execution of Step 1 provided six research questions obtained from the previous review’s insights and the review’s methodology. Zhang et al. (Citation2021) paper was used as a reference to assist in formulating the problem. Tables show the formulation of the problem, from investigating the insights to developing the research questions. Step 1 provided two findings: (1) the problem of the review was the decision-making mechanism of dynamic capability in the RBT literature, and (2) the methodology of the review was modified from SLR by Xiao and Watson (Citation2019). Step 2 resulted in a review protocol, which became the review rule for searching the literature. Review protocols constitute the research method used in this review. Six components of the review protocol were included in this review. The researcher leverages the protocols to conduct a review and restricts the review topic.
4.1. Conducting phase
This review was conducted according to the review protocol. The conducting phase from Steps 3 to 7, provided the final selection of as many as 27 papers with data analysis and synthesis. Search and selection of the final selection of literature consisted of two techniques: automated search and manual search. Subsequently, the final selection was analyzed using bibliometric and SMS analyses. The results of the bibliometric analysis indicate the following:
The United Kingdom and the United States have dominated the country’s documents.
The United States has the highest number of citations.
The prominent co-occurrences and link strengths of keywords were dynamic capabilities, decision-making, and enterprise resource management.
Teece et al.‘s (2016) paper was the highest number of citations.
Meanwhile, SMS analysis produced findings regarding the decision-making mechanism of dynamic capability in RBT. The six findings are as follows:
Complex situations dominate the decision-making context.
The decision-making processing strategy prominently pointed out BDA and MCDM.
Few publications have covered the decision mechanism with the following characteristics: real-time, dynamic, adaptive, and iterative.
Decision mechanisms were implemented in the subject fields: resource configuration, organizational ability, data-driven decision-making, hybrid mental-computer model, supply chain, managerial capabilities, strategy barriers, and organizational capabilities.
The publication of decision-making mechanisms was mainly conducted in the common industry, although few publications covered maritime, technology, social enterprises, agri-food, research, apparel retail, cinema, and manufacturing.
DEMATEL, AHP, and ANP were the most used decision support tools, and machine learning was still used less in the decision-making process.
4.2. Reporting phase
The final phase of the review methodology is the reporting phase, in which all implementations are documented. The results of each step of the SLR revealed the implementation of the reporting phase. Step 1 delivers the research question list and the flowchart methodology of the review, and Step 2 provides the review protocol as a guideline for review. Steps 3, 4, 5,6, and 7 serve as the flowchart for searching the literature. The results of bibliometric analysis and SMS were exhibited as a country’s citation table, document number map, table and visualization of co-occurrence and link strength keywords, article citation table, and table of SMS analysis. All the results of the bibliometric analysis and SMS were constituted in Step 8.
Meanwhile, the review synthesis constitutes a means to seek the convergence of all findings to answer six research questions (RQ1, RQ2, RQ3, RQ4, RQ5, and RQ6). This review provided four insights from bibliometric analysis and six from SMS analysis. Subsequently, all insights were synthesized into convergent answers to the research questions. The synthesis of the findings provides the following answers:
1. RQ1 mentions an inquiry regarding the status of decision-making mechanism research in the RBT literature.The status of RBT publications on decision-making mechanisms shows that the United States and the United Kingdom are still research centers on decision-making mechanisms with dynamic capability. The three main keywords in decision-making mechanism research are dynamic capabilities, decision-making, and enterprise resource management.
2. RQ2 represents the question with the most influential paper on the decision-making mechanism of dynamic capability.The review found that Teece et al. (Citation2016) publication was a barometric reference for many researchers. “Dynamic Capabilities and Organizational Agility: Risk, Uncertainty and Entrepreneurial Management in the Innovation Economy” by Teece et al. is a powerful publication that many researchers refer to theirs.
3. RQ3 elaborates on the inquiry regarding decision-making publications based on context, process, and characteristics.The review outline in RBT shows that the decision-making mechanism of dynamic capability conducted in a complex context utilizes the BDA and MCDM processes, and few papers with complete characteristics of the mechanism.
4. RQ4 declares the question of dealing with the decision-making mechanism implementation in the RBT literature based on the subject field.The decision-making mechanism of RBT encompasses the following subject fields: resource configuration, organizational ability, data-driven decision-making, hybrid mental-computer model, supply chain, managerial capabilities, strategy barriers, and organizational capabilities.
5. RQ5 is accompanied by an inquiry regarding on the industry view on publications of decision-making mechanisms in the RBT literature.In general, RBT publications on decision-making mechanisms cover common industries, even though several papers cover particular industries such as maritime, technology, social enterprises, agri-food, research, apparel retail, cinema, and manufacturing.
6. RQ6 addresses the decision support tool of publications that assists in the decision mechanism of dynamic capability.Many researchers have generally used DEMATEL, AHP, and ANP as decision support tools, even though several scientists have conducted machine learning and data-driven methods.
According to the synthesis of the review, critiques have emerged to address the apparent mechanism of decision-making in RBT. First, decision-making regarding dynamic capability in the RBT did not perform enterprise resource management. It involves the system approach as a bridge between RBT and decision-making. This critique is relevant to Priem and Butler’s criticism (Kraaijenbrink et al., Citation2010). The decision-making of dynamic capability in RBT should point out processes, elements, and purposes. No publications in a review illustrate the implementation of decision-making mechanisms in the system approach. Hence, decision-making mechanisms in RBT need to be detailed in system components.
Second, the decision-making mechanism in the current literature needs to be revised by Sirmon to expose resource orchestration (Sirmon et al., Citation2011). By contrast, RBT’s dynamic capability prompts the firm’s flexibility to integrate, build, and reconfigure internal and external resources or competencies (Teece et al., Citation1997). The decision-making mechanism should reveal real-time, adaptive, dynamic, and iterative processes to overcome the moderately dynamic and high-velocity market context (Eisenhardt & Martin, Citation2000).
Third, no particular conceptual model of decision-making in RBT indicates the mechanism of resource selection from delivering resource/capability alternatives to determining resource/capability configuration, as well as Sirmon et al. (Citation2007, Citation2011) proposed model. This critique states that the decision-making mechanism needs to design a conceptual model to provide a decision-making system in RBT. This model development involves the micro-foundations of dynamic capability, the primary process of dynamic capability, resource management, and decision support systems.
4.3. Future research
Based on the insights of the review, further work on the decision-making mechanism in RBT is needed to develop a theoretical framework to underlie the concept of the decision-making model. A theoretical framework is built using RBT, the dynamic capability view, decision-making theory, bounded rationality, Cynefin’s context, big data analytic capability, and resource orchestration. The development of a theoretical framework focuses on how firms can identify, integrate, configure, leverage, and reconfigure resources and capabilities to achieve a competitive advantage in a dynamic environment Barney, Citation1991, Eisenhardt & Martin, Citation2000; Sirmon et al., Citation2007, Citation2011; Teece, Citation2007; Teece et al., Citation1997.
Building a conceptual decision-making model for RBT is a topic for future research. The model must be accompanied by resource quality (valuable, rare, inimitable, exploited by an organization), a clear decision-making mechanism, resource orchestration, and a decision support system. The development of a conceptual model refers to the underlying theory. Hence, future research should focus on developing a model to determine the resource configuration to achieve a competitive advantage.
Eventually, review improvement is conducted continuously in further works besides the two agendas. The updated review seeks the focal subject to explore the decision-making mechanism of dynamic capability in RBT. Conducting review improvements will upscale the decision-making model of RBT.
5. Conclusions
Researchers in several countries have investigated the decision-making mechanism of dynamic capability in RBT. The United States and the United Kingdom are prominent countries that provide documents and citations. Based on the review results, a new finding is that “enterprise resource management” is the highlighted keyword in the decision-making mechanism. The review also shows that Teece et al. (Citation2016) paper was the most referenced by researchers when they conducted decision-making research in RBT.
The last decision-making research on RBT generally pointed out the complex context, generated the BDA and MCDM processes, and need to characterize the characteristics of dynamic capability fully. Publications need to be more comprehensive to present the decision-making mechanism in enterprise resource management. Thus, the proposed theoretical framework and conceptual decision-making model must be developed to follow the dynamic environment in real-time, dynamically, adaptively, and iteratively.
Previous studies on decision-making mechanisms covered the following subject fields: resource configuration, organizational ability, data-driven decision-making, hybrid mental-computer model, supply chain, managerial capabilities, strategy barriers, and organizational capabilities. Subject field coverage must focus more on strategic decisions regarding resource configuration to support the firm’s strategy. The firm can optimally exploit the resources/capabilities aggregate to evoke a competitive advantage and elevate performance.
Furthermore, the decision-making mechanism of RBT is mainly discussed in common industries rather than in specific sectors. Particular industries indeed have specific resources and capabilities, but the decision-making mechanism generally helps the manager determine the resource configuration for the firm’s strategy. Hence, a precise decision-making mechanism in RBT is the integral process of dynamic capability.
Finally, the decision-making mechanism constitutes a part of the decision-making system, and execution requires a decision-support tool. Researchers have created various devices to assist in the decision-making process by providing decisions in real-time, dynamic mechanisms, and iterative processes. The decision support tool should help the decision-making mechanism adapt to dynamically moderate and high-velocity market contexts.
6. Limitation
This review used the SCOPUS and PROQUEST databases, which caused the limitation of the literature number. The availability of journal access limits the vast amount of literature data. Subsequently, the topic of the review only revolves around the decision-making mechanism but has expanded to the decision-making model of the RBT. An in-depth analysis of the review requires more enhancement and enrichment of the materials.
7. Recommendations
Several recommendations make some improvements to this review for other researchers concerning the availability of the literature database, quality of literature assessment, and in-depth analysis of the final selection of literature. The availability of database literature can be enhanced to other databases, such as Web of Science, Springer, DOAJ, and Science Direct. The additional database for the search literature can convince and strengthen the review regarding the decision-making mechanism topic in RBT.
The quality of literature assessment encompasses the methodology of the review. This review refers to the SLR methodology proposed by Kitchenham and Charters (Citation2007) and Xiao and Watson (Citation2019). Therefore, the modified SLR can be improved using other methodologies. The solid methodology provides robust results for the review.
Eventually, the depth analysis of the final selected papers uses both the bibliometric and SMS analyses. Other analyses can be conducted as meta-analysis reviews, critical analysis reviews, or narrative reviews. A combination of analyses will enrich the quality of the review and provide a wider perspective.
Acknowledgments
We thank the reviewers for their fruitful comments regarding the development of this manuscript. We also thank the School of Business and Management of Bandung Institute of Technology for supporting this research.
Disclosure statement
The author(s) declared no potential conflicts of interest with respect to the research, authorship, and/or publication of this article.
Additional information
Funding
Notes on contributors
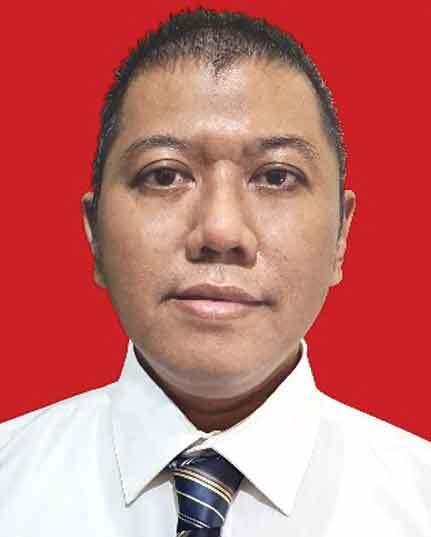
Mochammad Ridwan Ristyawan
Mochammad Ridwan Ristyawan is a Lecturer at Universitas Tanjungpura, Indonesia. He is accomplishing a Doctor of Sains Management in the School of Business Management, Institut Teknologi Bandung, Indonesia. His research interest focus on decision-making, data analytics, strategic management, and banking.
Utomo Sarjono Putro
Utomo Sarjono Putro is a Professor at School of Business and Management, Institut Teknologi Bandung, Indonesia. He specializes in decision science, system thinking, and strategic negotiation.
Manahan Siallagan
Manahan Siallagan is a Senior Lecturer at the School of Business and Management, Institut Teknologi Bandung, Indonesia. He has specialized in big data analytics, agent-based modelling, system dynamics, and system thinking.
References
- Agostini, L., & Nosella, A. (2021). Industry 4.0 and business models: A bibliometric literature review. Business Process Management Journal, 27(5), 1633–28. https://doi.org/10.1108/BPMJ-03-2021-0133
- Allred, C. R., Fawcett, S. E., Wallin, C., & Magnan, G. M. (2011). A dynamic collaboration capability as a source of competitive advantage. Decision Sciences, 42(1), 129–161. https://doi.org/10.1111/j.1540-5915.2010.00304.x
- Ayabakan, S., Bardhan, I. R., & Zheng, Z. (2017). A data envelopment analysis approach to estimate IT-enabled production capability. MIS Quarterly, 41(1), 189–206. https://doi.org/10.25300/MISQ/2017/41.1.09
- Barney, J. (1991). Firm resources and sustained competitive advantage. Journal of Management, 17(1), 99–120. https://doi.org/10.1177/014920639101700108
- Barney, J. B. (2001). Resource-based theories of competitive advantage: A ten-year retrospective on the resource-based view. Journal of Management, 27(6), 643–650. https://doi.org/10.1177/014920630102700602
- Barney, J. B., & Hesterly, W. S. (2015). Strategic management and competitive advantage: Concepts and cases (5th ed.). Pearson.
- Barney, J. B., & Wright, P. M. (1998). On becoming a strategic partner: The role of human resources in gaining competitive advantage. Human Resource Management, 37(1), 31–46. https://doi.org/10.1002/(SICI)1099-050X(199821)37:1<31:AID-HRM4>3.0.CO;2-W
- Bathke, H., Münch, C., von der Gracht, H. A., & Hartmann, E. (2022). Building resilience through Foresight: The case of Maritime Container Shipping firms. IEEE Transactions on Engineering Management, 1–23. https://doi.org/10.1109/TEM.2021.3137009
- Bhardwaj, R., Srivastava, S., Taggar, R., & Bindra, S. (2022). Exploring micro-foundations of dynamic capabilities for social enterprises. Social Enterprise Journal, 18(3), 451–469. https://doi.org/10.1108/SEJ-08-2021-0071
- BNI. (2021). Annual report 2021: Jumping higher for the country. https://www.bni.co.id/Portals/1/BNI/Perusahaan/HubunganInvestor/Docs/AR-BNI-TB-2021-ENG-v2.pdf
- Bucak, U., Mollaoğlu, M., & Dinçer, M. F. (2022). Port personnel recruitment process based on dynamic capabilities: Port managers’ priorities vs customer evaluations. Maritime Business Review. https://doi.org/10.1108/MABR-01-2022-0003
- Čirjevskis, A., & Tvaronavičienė, M. (2017). Acquisition based dynamic capabilities and reinvention of business models: Bridging two perspectives together. Entrepreneurship & Sustainability Issues, 4(4), 516. https://doi.org/10.9770/jesi.2017.4.4(9)
- Collan, M., Freiling, J., Kyläheiko, K., & Roemer, E. (2014). Entrepreneurship and the art of tackling technological crises: A strategic real options framework. International Journal of Technology Intelligence and Planning, 10(2), 166–185. https://doi.org/10.1504/IJTIP.2014.068021
- Conboy, K., Mikalef, P., Dennehy, D., & Krogstie, J. (2020). Using business analytics to enhance dynamic capabilities in operations research: A case analysis and research agenda. European Journal of Operational Research, 281(3), 656–672. https://doi.org/10.1016/j.ejor.2019.06.051
- Dahiya, R., Le, S., Ring, J. K., & Watson, K. (2022). Big data analytics and competitive advantage: The strategic role of firm-specific knowledge. Journal of Strategy and Management, 15(2), 175–193. https://doi.org/10.1108/JSMA-08-2020-0203
- Dierickx, I., & Cool, K. (1989). Asset stock accumulation and the sustainability of competitive advantage: Reply. Management Science, 35(12). https://doi.org/10.1287/mnsc.35.12.1514
- Donnellan, J., & Rutledge, W. L. (2019). A case for resource-based view and competitive advantage in banking. Managerial and Decision Economics, 40(6), 728–737. https://doi.org/10.1002/mde.3041
- Eisenhardt, K. M., & Martin, J. A. (2000). Dynamic capabilities: What are they?. Strategic Management Journal, 21(10–11), 1105–1121. https://doi.org/10.1002/1097-0266(200010/11)21:10/11<1105::AID-SMJ133>3.0.CO;2-E
- Farago, F. E., Denkewski, W., Lourenço, M. L., & Fernandes, J. M. F. (2019). Dynamic capabilities, new business creation and the entrepreneur: An analysis about the La La Land film. International Journal of Entrepreneurship, 23(1), 1–14. https://www.proquest.com/scholarly-journals/dynamic-capabilities-new-business-creation/docview/2238481542/se-2?accountid=31562
- Gorzeń-Mitka, I., & Okręglicka, M. (2014). Improving decision making in complexity environment. Procedia Economics and Finance, 16, 402–409. https://doi.org/10.1016/S2212-5671(14)00819-3
- Grant, R. M. (1996). Toward a knowledge-based theory of the firm. Strategic Management Journal, 17(S2), 109–122. https://doi.org/10.1002/smj.4250171110
- Hart, S. L. (1995). A Natural-resource-based view of the firm. Academy of Management Review, 20(4), 986–1014. https://doi.org/10.2307/258963
- Hasegan, M. F., Nudurupati, S. S., & Childe, S. J. (2018). Predicting performance – a dynamic capability view. International Journal of Operations & Production Management, 38(11), 2192–2213. https://doi.org/10.1108/IJOPM-10-2016-0601
- Helfat, C. E., & Winter, S. G. (2011). Untangling dynamic and operational capabilities: Strategy for the (N)ever changing world. Strategic Management Journal, 32(11), 1243–1250. https://doi.org/10.1002/smj.955
- Helfat, C. E., & Peteraf, M. A. (2015). Managerial cognitive capabilities and the microfoundations of dynamic capabilities. Strategic Management Journal, 36(6), 831–850. https://doi.org/10.1002/smj.2247
- Henry, J. (2020). Banking system stress testing and COVID-19: A first summary appraisal. Journal of Risk Management in Financial Institutions, 14(1), 7–24. https://EconPapers.repec.org/RePEc:aza:rmfi00:y:2020:v:14:i:1:p:7-24
- Jafari-Sadeghi, V., Amoozad Mahdiraji, H., Bresciani, S., & Pellicelli, A. C. (2021). Context-specific micro-foundations and successful SME internationalisation in emerging markets: A mixed-method analysis of managerial resources and dynamic capabilities. Journal of Business Research, 134, 352–364. https://doi.org/10.1016/j.jbusres.2021.05.027
- Jafari-Sadeghi, V., Amoozad Mahdiraji, H., Busso, D., & Yahiaoui, D. (2022). Towards agility in international high-tech SMEs: Exploring key drivers and main outcomes of dynamic capabilities. Technological Forecasting and Social Change, 174, 121272. https://doi.org/10.1016/j.techfore.2021.121272
- James, B. J., & Joseph, C. (2015). Corporate governance mechanisms and bank performance: Resource-based view. Procedia Economics and Finance, 31, 117–123. https://doi.org/10.1016/S2212-5671(15)01138-7
- Kay, N. M. (2010). Dynamic capabilities as context: The role of decision, system and structure. Industrial and Corporate Change, 19(4), 1205–1223. https://doi.org/10.1093/icc/dtq028
- Khan, F., Siddiqui, M. A., & Imtiaz, S. (2022). Role of financial literacy in achieving financial inclusion: A review, synthesis and research agenda. Cogent Business & Management, 9(1), 2034236. https://doi.org/10.1080/23311975.2022.2034236
- Kitchenham, B., & Charters, S. (2007). Guidelines for performing systematic literature reviews in software engineering. Technical Report, Ver. 2.3 EBSE Technical Report. EBSE.
- Kozak, J., Kania, K., Juszczuk, P., & Mitręga, M. (2021). Swarm intelligence goal-oriented approach to data-driven innovation in customer churn management. International Journal of Information Management, 60, 102357. https://doi.org/10.1016/j.ijinfomgt.2021.102357
- Kraaijenbrink, J., Spender, J.-C., & Groen, A. J. (2010). The resource-based view: A review and assessment of its critiques. Journal of Management, 36(1), 349–372. https://doi.org/10.1177/0149206309350775
- Kunc, M. (2007). Portraying managerial dynamic capabilities: A case study in the fast-moving consumer goods industry. International Journal of Learning and Intellectual Capital, 4(1–2), 92–110. https://doi.org/10.1504/IJLIC.2007.013825
- Lippman, S. A., & Rumelt, R. P. (1982). Uncertain imitability: An analysis of Interfirm differences in efficiency under competition. The Bell Journal of Economics, 13(2), 418–438. https://doi.org/10.2307/3003464
- Mathivathanan, D., Govindan, K., & Haq, A. N. (2017). Exploring the impact of dynamic capabilities on sustainable supply chain firm’s performance using grey-analytical hierarchy process. Journal of Cleaner Production, 147, 637–653. https://doi.org/10.1016/j.jclepro.2017.01.018
- McKinsey. (2020). McKinsey’s global banking annual review. Retrieved November 12, 2021. https://www.mckinsey.com/industries/financial-services/our-insights/global-banking-annual-review.
- McKinsey. (2022). COVID-19: Briefing note #100, April 13, 2022. McKinsey Global Publishing. Retrieved November 2, 2022. https://www.mckinsey.com/capabilities/risk-and-resilience/our-insights/covid-19-implications-for-business
- Möller, U., & McCaffrey, M. (2021). Entrepreneurship and firm strategy: Integrating resources, capabilities, and judgment through an Austrian framework. Entrepreneurship Research Journal, https://doi.org/10.1515/erj-2020-0519
- Newbert, S. L. (2008). Value, rareness, competitive advantage, and performance: A conceptual-level empirical investigation of the resource-based view of the firm. Strategic Management Journal, 29(7), 745–768. https://doi.org/10.1002/smj.686
- Penrose, R. (1959). The apparent shape of a relativistically moving sphere. Mathematical Proceedings of the Cambridge Philosophical Society, 55(1), 137–139. https://doi.org/10.1017/S0305004100033776
- Peteraf, M. A. (1993). The cornerstones of competitive advantage: A resource-based view. Strategic Management Journal, 14(3), 179–191. https://doi.org/10.1002/smj.4250140303
- Priem, R. L., & Butler, J. E. (2001). Is the resource-based “view” a useful perspective for strategic research? Academy of management Review, 26(1), 22–40. https://doi.org/10.5465/amr.2001.4011928
- Roberts, P. S., & Wernstedt, K. (2019). Herbert Simon’s forgotten legacy for improving decision processes. International Public Management Journal, 22(4), 591–616. https://doi.org/10.1080/10967494.2018.1502223
- Shamim, S., Zeng, J., Shariq, S. M., & Khan, Z. (2019). Role of big data management in enhancing big data decision-making capability and quality among Chinese firms: A dynamic capabilities view. Information & Management, 56(6), 103135. https://doi.org/10.1016/j.im.2018.12.003
- Singh, A. K., & Samuel, C. (2020). Positioning strategy implementation barriers: A decision making approach based on resource-based theory. International Journal of Management & Decision Making, 19(4), 473–504. https://doi.org/10.1504/IJMDM.2020.110884
- Sirmon, D. G., Hitt, M. A., & Ireland, R. D. (2007). Managing firm resources in dynamic environments to create value: Looking inside the black box. Academy of Management Review, 32(1), 273–292. https://doi.org/10.5465/amr.2007.23466005
- Sirmon, D. G., Hitt, M. A., Ireland, R. D., Gilbert, B. A., Barney, J. B., Ketchen, D. J., & Wright, M. (2011). Resource orchestration to create competitive advantage: Breadth, depth, and life cycle effects. Journal of Management, 37(5), 1390–1412. https://doi.org/10.1177/0149206310385695
- Snowden, D. J., & Boone, M. E. (2007). A leader’s framework for decision making. Harvard Business Review, 85(11). https://hbr.org/2007/11/a-leaders-framework-for-decision-making
- Teece, D. J. (2007). Explicating dynamic capabilities: The nature and microfoundations of (sustainable) enterprise performance. Strategic Management Journal, 28(13), 1319–1350. https://doi.org/10.1002/smj.640
- Teece, D. J. (2014). The foundations of enterprise performance: Dynamic and ordinary capabilities in an (economic) theory of firms. Academy of Management Perspectives, 28(4), 328–352. https://doi.org/10.5465/amp.2013.0116
- Teece, D., Peteraf, M., & Leih, S. (2016). Dynamic capabilities and organizational agility: Risk, uncertainty, and strategy in the innovation economy. California Management Review, 58(4), 13–35. https://doi.org/10.1525/cmr.2016.58.4.13
- Teece, D. J., Pisano, G., & Shuen, A. (1997). Dynamic capabilities and strategic management. Strategic Management Journal, 18(7), 509–533. https://doi.org/10.1002/(SICI)1097-0266(199708)18:7<509:AID-SMJ882>3.0.CO;2-Z
- van Rijmenam, M., Erekhinskaya, T., Schweitzer, J., & Williams, M.-A. (2019). Avoid being the Turkey: How big data analytics changes the game of strategy in times of ambiguity and uncertainty. Long Range Planning, 52(5), 101841. https://doi.org/10.1016/j.lrp.2018.05.007
- Wernerfelt, B. (1984). A resource-based view of the firm. Strategic Management Journal, 5(2), 171–180. https://doi.org/10.1002/smj.4250050207
- Xiao, Y., & Watson, M. (2019). Guidance on conducting a systematic literature review. Journal of Planning Education and Research, 39(1), 93–112. https://doi.org/10.1177/0739456X17723971
- Yadav, S., Luthra, S., & Garg, D. (2021). Modelling Internet of things (IoT)-driven global sustainability in multi-tier agri-food supply chain under natural epidemic outbreaks. Environmental Science and Pollution Research, 28(13), 16633–16654. https://doi.org/10.1007/s11356-020-11676-1
- Yan, M.-R., Hong, L.-Y., & Warren, K. (2022). Integrated knowledge visualization and the enterprise digital twin system for supporting strategic management decision. Management Decision, 60(4), 1095–1115. https://doi.org/10.1108/MD-02-2021-0182
- Yasmin, M., Tatoglu, E., Kilic, H. S., Zaim, S., & Delen, D. (2020). Big data analytics capabilities and firm performance: An integrated MCDM approach. Journal of Business Research, 114, 1–15. https://doi.org/10.1016/j.jbusres.2020.03.028
- Yazdi, M., Khan, F., Abbassi, R., & Rusli, R. (2020). Improved DEMATEL methodology for effective safety management decision-making. Safety Science, 127, 104705. https://doi.org/10.1016/j.ssci.2020.104705
- Zeng, J., & Khan, Z. (2019). Value creation through big data in emerging economies. Management Decision, 57(8), 1818–1838. https://doi.org/10.1108/MD-05-2018-0572
- Zhang, Y., Hou, Z., Yang, F., Yang, M. M., & Wang, Z. (2021). Discovering the evolution of resource-based theory: Science mapping based on bibliometric analysis. Journal of Business Research, 137, 500–516. https://doi.org/10.1016/j.jbusres.2021.08.055
- Zupic, I., & Čater, T. (2015). Bibliometric methods in management and organization. Organizational Research Methods, 18(3), 429–472. https://doi.org/10.1177/1094428114562629
- Ҫolak, G., & Öztekin, Ö. (2021). The impact of COVID-19 pandemic on bank lending around the world. Journal of Banking & Finance, 133, 106207. https://doi.org/10.1016/j.jbankfin.2021.106207