Abstract
Online shopping has grown in popularity over the past few years, particularly in the context of COVID-19. Gamification is also progressively emerging as an ideal means to boost motivation and encourage community engagement. This study attempts to identify the variables influencing young Vietnamese people’s willingness to adopt gamification and its impact on their buying habits in order to suggest practical solutions for business marketing initiatives. The study uses a quantitative research approach with simple random sampling (n = 230). The number of valid samples officially included in the study is 288 as a consequence of the survey results. The results suggest that the following elements significantly impact the intention to adopt gamification: favorable circumstances, effort expectations, social influence, and performance expectations. These have a 54.1 percent impact on gamification acceptance intent and a 29.6 percent impact on online shopping behavior. Finally, the study’s findings are used to make various suggestions to improve gamification’s effectiveness in marketing activities.
1. Introduction
Gamification is currently being used in a wide range of industries, including education, sports, human resource management, and online marketing. The gamification of e-commerce applications is growing (Tobon et al., Citation2020). E-commerce platforms like Alipay and Taobao have utilized gamified MiniApps to attract more customers by offering game-like experiences (Meeker, Citation2019). Numerous successful video games inspired by movies, such as Payday: The Heist, Marvel’s Spider-Man, Star Wars Jedi: Fallen Order, etc., indicate that gamification is becoming more widely adopted. Retailers in India have identified it as an upcoming trend in social media marketing, and their customer-centric initiatives include gamification in their core process in order to “drive engagement and participation” (Archana, Citation2012). Gamification aims to enhance usability and satisfaction, create more enjoyable experiences and produce a positive business impact (Liu et al., Citation2017; Saha et al., Citation2012).
Furthermore, in the context of the global COVID-19 pandemic, some countries quickly responded and succeeded in early containment of the disease. However, many other countries opted for prevention measures and only intervened when the major impacts of the pandemic emerged. Consequently, social distancing measures made online shopping more popular and important than ever (Sahin et al., Citation2020). As a result, gamification not only makes participants feel that they love the brand but also develops a sense of belonging with the brand (Ruengaramrut et al., Citation2020). This outcome adds to the reason why the application of gamification in marketing deserves further active attention.
Several research studies have been conducted in many countries investigating and evaluating the impact of gamification on online shopping behavior. Some popular studies include whether gamification encourages online buying through elements of focused attention, perceived usability, felt involvement and novelty (García-Jurado et al., Citation2021); or are developing countries ready for gamification or not? From the study of Ofosu-Ampong et al. (Citation2020) has shown that attitude plays an important role in students’ intention to use, however, the level of understanding and access to gamification in learning at universities is still limited (Ofosu-Ampong et al., Citation2020); another study indicates that anchoring gamification in the service marketing literature for enhancing the service with the affordability of the game experience to support the overall user value creation (Huotari & Hamari, Citation2017); gamification and mobile marketing effectiveness is lifelong as it has the potential to affect the customer experience (Hofacker et al., Citation2016); gamification design for increasing customer purchase intention in a mobile marketing campaign app through four phases: attract, engage, retain, and monetize to increase customer purchase intent in mobile marketing campaigns (Wen et al., Citation2014). In addition, gamification was proved that it can affect brand engagement and equity. A study in online brand communities suggested that its feature is associated with a positive association in social interaction and a positive influence on brand engagement and increased more brand value, and also to brand management (Xi & Hamari, Citation2020); and the gamification systems application elements in the marketing perspective requires more involvement of consumers in the buying decision process (Teotónio & Reis, Citation2018). The above studies are fundamental materials for preparing this paper to apply gamification to Vietnamese youth and its impact on online shopping habits. The majority of the aforementioned articles use the Technology Acceptance Model (TAM), the Theory of Reasoned Action (TRA), or the Theory of Planned Behavior (TPB), to analyze consumer online shopping behavior. According to this approach, customers’ perceptions and attitudes toward technology impact their intention to utilize it. In this article, the Unified Theory of Acceptance and Use of Technology (UTAUT) model will be used to explain how customers behave when they shop online in order to provide a more thorough understanding of all the aspects that can influence their technology-using behavior. UTAUT is a combined model based on eight previous models studying user acceptance of a new information system, including the Theory of Reasoned Action (TRA), the Technology Acceptance Model (TAM), the Motivation Model (MM), the Theory of Planned Behavior (TPB), a model combining TAM and TPB -C-TAM-TPB), the Model of PC Utilization (MPCU), Innovation Diffusion Theory (IDT), and Social Cognitive Theory—SCT; as a result, UTAUT has been tested and proven to outperform other models (Venkatesh & Zhang, Citation2010).
The application of gamification is a fruitful avenue for marketing research when it is realized that the global economy is increasing significantly based on the development of digital platforms. As a result, businesses that operate on e-commerce platforms are always looking for new methods to attract, retain, and drive customers to engage with their brands in various ways. This study provides an in-depth look at the potential for gamification applications to affect online shopping behavior, particularly among young people who are considered digital consumption-explosive factors. In particular, the first and foremost objective is to show the relationship between independent and dependent variables; after that, it will examine the mediating role of gamification adoption attention in regulating the relationship between the independent and dependent variables; and finally, it will then provide managerial implications for applying gamification effectively in marketing activities, improving customer experience, and increasing online shopping behavior.
2. Literature review
2.1. Unified Theory of Acceptance and Use of Technology (UTAUT)
The UTAUT model was established in 2003. It is a hybrid model built on eight previous models that looked at how users reacted to a new information system. Performance expectancy, effort expectancy, social influence, and facilitating conditions are the four fundamental elements that determine adoption and use. Performance expectancy is defined as the degree to which an individual believes that adopting new systems would assist them in achieving improved task performance; effort expectancy is defined as the system’s ease of use. The extent to which an individual feels the importance of being influenced by the beliefs of people around him that the individual should use the new system is referred to as social influence. The degree to which an individual believes the organization’s or individual’s technical infrastructure is qualified to support the system is referred to as facilitation (Venkatesh et al., Citation2003). External elements, such as gender, age, the voluntariness of use, and experience, are also mentioned in the UTAUT model as contributing to the adjustment of intention to use. UTAUT is believed to combine the fundamental aspects of previous models and weighs up the impact of these factors on the desire to use and the user behavior differentiated by peripheral factors. UTAUT has also been tested and found to be superior to other models (Venkatesh & Zhang, Citation2010). Nowadays, researchers use this model more commonly. Research topics that apply UTAUT include consumer health technology, E-health, user acceptance, virtual reality, technology acceptance, integration, innovation, technical advice, UTAUT2, mobile learning, consumer perception and behavior (by analyzing the keyword co-word network map of UTAUT) (Wang et al., Citation2021). Thereby, the UTAUT model explains user intention is measured with performance expectancy, effort expectancy, social influence, and facilitating conditions. In other words, the UTAUT model provides a more explicit picture of user behavior. Likewise, this paper’s purpose studies to influence customer behavioral intentions through perceived benefits in perceived usefulness and perceived ease of use of new technology adoption and how this behavioral prediction process can be moderated by online consumer attitudes. Thus, the elements in the structure of UTAUT are very suitable to determine the variables affecting the readiness of Vietnamese youth to accept gamification and usage behavior (Figure ).
2.2. Gamification
The term “gamification” is not to be confused with the term “game.” Gamification is using game design components and techniques to tackle problems unrelated to games (Kevin & Dan, Citation2012). Some activities are vital to performing many different facets of life. However, only some people enjoy them, such as learning, doing repetitive tasks at work, and exercising…yet we still have to do it, and sometimes our displeasure reduces the effectiveness of our work. As a result, the goal of gamified application design should be to encourage playful and reflective behaviors (Drugău-Constantin, Citation2019). In addition, gamification will encourage the process of completing those tedious activities. The following ideas can be derived from the above foundations: gamification is a method of increasing stakeholder motivation, participation, and contribution based on the psychology and internal motivation of the target audience, resulting in the desired outcomes (Mohammadi, Citation2014). Gamification has emerged as an innovative marketing strategy that effectively attracts and motivates online consumer behavior through interactive immersion features (Xi & Hamari, Citation2020). Additionally, the utilization of gamification through mobile applications has the potential to augment the consumption of intentional behaviors (Hofacker et al., Citation2016). In higher education, integrating gamification has a relationship with attitudes, favorable conditions, and familiarity with technology to create a trend of gamification application in teaching and research at the high level (Ofosu-Ampong et al., Citation2020). Therefore, it can be concluded that gamification has a close relationship with consumer intention and behavior consumption.
In general, acceptance and access to the internet are increasingly commonplace globally, and every year the number of people shopping online grows. In 2020, more than two billion people purchased goods or services online, and in the same year, e-retail sales surpassed US$4.2 trillion worldwide. This remarkable increase is partly due to the significant impact of the COVID-19 pandemic, which has affected e-commerce and online consumer behavior worldwide. At the beginning of 2020, social distancing measures were in place, and since then, millions of people worldwide have had to stay at home to prevent the spread of the virus, so digital channels have become the ubiquitous solution. The most popular way to avoid crowds is to shop online. In particular, in June 2020, global retail e-commerce traffic reached a record level, with 22 billion monthly visits for everyday items, such as groceries, clothing, and other categories of retail technology (Clement, Citation2019). In terms of online shopping, it is a type of e-commerce that allows users to buy goods or services directly from a vendor over the internet (Liang & Lai, Citation2000). Consumers benefit from online shopping because they do not have to move when they shop, do not take time to go to stores, and it is easy to find items that fulfill their needs and can be purchased online (Forsythe et al., Citation2006). Furthermore, online shopping offers a wide range of products, a pleasant shopping experience, and a sales website that can fully meet the needs of consumers at clear, transparent, and fair rates (Chowdhury & Ahmad, Citation2012).
2.3. Research model & hypothesis
After the secondary data collection, it is easy to see that there are precedents for using the UTAUT theory in the study of gamification. This is the basis to prove the feasibility of applying the UTAUT theory to this topic. The research for “Does gamification engage users in online shopping?” (García-Jurado et al., Citation2021) provided solid evidence to confirm the direct impact of gamification on user engagement. In addition, the study Enhancing consumer online purchase intention through gamification in China: Perspective of cognitive evaluation theory (Xu et al., Citation2020) uses cognitive evaluation theory to explain the impact of gamification factors on consumer purchase intention. The results showed that factors such as reward, attraction, and autonomy of gamification enhance the customer’s sense of enjoyment and satisfaction of psychological needs, influencing online consumer buying satisfaction. In addition, gamification is widely used in studies on intention, brand awareness, and loyalty in tourism (Abou-Shouk & Soliman, Citation2021) and learning industries (Bui & Bui, Citation2018; Ofosu-Ampong et al., Citation2020). In short, the factors in the UTAUT model were deployed in this study’s research model as outlined below (Figure ):
Performance Expectancy is defined as the degree to which a person believes that employing a system would help them be more effective (Venkatesh et al., Citation2003). In this area of research, Performance Expectation is understood to be the level of efficiency in online shopping when users participate in gamification. For instance, it encourages, improves, and motivates online shopping and benefits gamers. Furthermore, it can directly influence on intention and buying behavior (Abou-Shouk & Soliman, Citation2021; Davis et al., Citation1989; Ofosu-Ampong et al., Citation2020). Consequently, the hypotheses are presented as follows:
H1.
Performance Expectancy positively influences Gamification Adoption Intention.
Effort Expectancy is described as the degree to which people quickly join and employ a technological system (Venkatesh et al., Citation2003). This study emphasizes the ease with which consumers participate in gamification (Abou-Shouk & Soliman, Citation2021; Ofosu-Ampong et al., Citation2020; Venkatesh & Zhang, Citation2010; Venkatesh et al., Citation2003). In addition, there are connections between the individual’s effort in tasks, the achievements that result from that effort, and the benefits that the individual receives as a consequence of that effort (Ghalandari, Citation2012). According to the findings of prior studies, this is a factor that significantly impacts the willingness to gamify adoption intention. As a result, the following is the second hypothesis:
H2.
Effort Expectancy positively influences Gamification Adoption Intention.
Social Influence is defined as the degree to which an individual believes others should use the new system (Venkatesh et al., Citation2003). It is the degree to which people believe others should participate in gamification. For example, a user may express a liking for playing a game on an e-commerce platform because all of his friends play it. Since the impact of family, friends, and coworkers is simple to perceive, it plays an essential role in shaping consumer intent and behavior, as evidenced by linked studies (Abou-Shouk & Soliman, Citation2021; Ofosu-Ampong et al., Citation2020; Venkatesh & Zhang, Citation2010). Therefore, the third hypothesis is:
H3.
Social Influence positively influences Gamification Adoption Intention.
Facilitating Conditions are defined as the degree to which an individual believes that organizational support and physical conditions will enable him or her to use the system easily (Venkatesh et al., Citation2003). Additionally, Facilitating Conditions as a concept within UTAUT refers to the degree to which an individual feels that the organizational and technical infrastructures necessary to use the intended system are present (Ghalandari, Citation2012). Therefore, if favorable facilitating conditions exist, consumers will be more likely to apply technology (Abou-Shouk & Soliman, Citation2021; Im et al., Citation2011; Ofosu-Ampong et al., Citation2020). Based on these evidence, the next hypothesis included in the study is:
H4.
Facilitating Conditions positively influences Gamification Adoption Intention.
Gamification Adoption Intention is the extent to which a user intends to perform, or not engage in gamification in the future. Gamification is appealing to online shoppers (García-Jurado et al., Citation2021). Gamification factors such as reward, attraction, and autonomy will enhance enjoyment and meet customers’ psychological needs, affecting online purchases (Xu et al., Citation2020). Moreover, several empirical studies have confirmed that behavioral intention plays an important role in predicting behavior, such as Sheppard et al. (Citation1988) who found strong support for using intention to predict behavior in a meta-analysis of behavioral intention to behavior; Taylor and Todd (Citation1995) who similarly found strong evidence in testing disaggregated TAM, TPB and TRA that the path from behavioral intention to behavior is significant in all models, or the research results of Kulviwat et al. (Citation2006) that showed intention to accept online shopping has a positive relationship with online shopping behavior. To put it another way, in this study, the willingness to adopt gamification had a favorable impact on online shopping behavior. Therefore, the following final hypothesis is:
H5.
Gamification Adoption Intention positively influences Online Buying Behavior.
3. Method
The research scale was formed by selectively using the approaches of previous typical studies. This study also selected scales from other studies to achieve a relevant and reliable level. Specifically, the online shopping behavior scale is inherited from (Huang et al., Citation2017; Xu et al., Citation2020). The remaining scales, in addition to the reference from the father of the unified theory of acceptance and use of technology (UTAUT), Venkatesh et al., also use scales from other groups of authors (Bui & Bui, Citation2018; Foon & Fah, Citation2011; Ofosu-Ampong et al., Citation2020). In addition, a five-point Likert scale was used for measurement in this study. Since this is an interval scale, the collected data can be used for processing and quantitative analysis to determine the correlation and linear relationships between the variables. This is a scale used by many people/research groups and has been acknowledged for its effectiveness and relevance. Therefore, the authors decided to use the Likert scale with the above five levels for their research survey. The survey questionnaire was based on the observed variables corresponding to each scale.
Gamification has become increasingly prominent on a global scale, with Vietnam serving as a notable example. Businesses have been using gamification in their marketing campaigns to draw in more customers, yet since this concept is still in its infancy, the priority of this study is on investigating gamification practices in the context of Vietnam. Younger people (aged 16–30) are the study’s target audience as they have the legal status to shop online independently. Young people should be viewed as a group of people transitioning from childhood to adult independence. In psychophysiology, this group of people enjoys a modern lifestyle, does not adhere to rigid frameworks, is always innovative, and is always looking for new trends to adopt. The traits, age, and age groupings from the Vietnam Young Generation Research Report were used in this study to understand young people better and target the correct audience (British, Citation2020). At the same time, Article 1 of the Youth Law (SRVN, Citation2020) states, “Youth are Vietnamese nationals between the ages of 16 and 30.” As a result, the entire sample of young people in Ho Chi Minh City who participate in gamification on e-commerce platforms is aged between 16 and 30.
In this study, the simple random sampling approach was applied, giving each member of the population an equal chance of being chosen (Berndt, Citation2020). This approach was selected since it is simple to understand and considered fair because anyone can be chosen. However, this approach still has its limitations, all members of the population must be included in a comprehensive and current list, yet this data is frequently unavailable. According to Hair et al. (Citation2010) the sample-to-observed variable ratio should be 5:1 or 10:1. The sample-to-observed variable ratio in this study has been set at 10:1 to ensure that the research findings are reliable. In this study, the total number of items is 23, so the minimum number of samples needed is N = 23 × 10 = 230 (Hair et al., Citation2010). Because the COVID-19 pandemic was still underway at the time of this investigation, direct access was limited. Therefore, the survey form (questionnaire) was sent to the candidates via email or social media sites such as Facebook, Zalo, and other similar platforms. Candidates participating in the survey were required to meet two criteria: (i) be between the ages of 16 and 30, and (ii) participate in gamification on e-commerce sites. Initially, 300 candidates participated in the survey. After the first screening question, “How old are you?” the number of participants retained for the survey was 295. The second screening question, “Have you or are you participating in any gamification on e-commerce sites?” excluded seven further participants from the survey. Therefore, after sample cleaning, the final number of samples included in the study was n = 288 (consistent with the previous estimate of n = 230). As a result, the number of samples above met Roscoe’s principle of sample sizes being larger than 30 and fewer than 500 (Roscoe, Citation1975).
Their data was then returned and processed. To evaluate the relationships between latent variables in the structural model proposed, the Covariance-Based-Structural Equation Modeling (CB-SEM) was adopted using AMOS 24.0 software. CB-SEM supports or refutes theories through hypothesis testing when the sample size is large, the data is normally distributed, and when most significantly, the model is precisely defined. In the process of converting the theory into a structural equation model, the right variables are chosen and connected (Hair et al., Citation2011; Hwang et al., Citation2010; Reinartz et al., Citation2009). This technique is ideal for investigations that support the research theory because it accurately compares the model to the theory by assessing how well the whole model fits with numerous significant statistical indicators. Additionally, CB-SEM is the best option because this is a heritage study from a prior study.
4. Results
4.1. Reliability and validity of the scale
The internal consistency approach, which uses Cronbach’s Alpha coefficient, is used to measure the scale’s dependability. This test aims to look at the observed variables using the total variable’s correlation coefficient (Corrected Item-Total Correlation). If the total variable’s correlation coefficient (Corrected Item-Total Correlation) is larger than 0.3, the observed variable fits the criteria (Nunnally, Citation1978). The scale can be employed especially when Cronbach’s Alpha reliability coefficients are in the range of 0.6 to less than 0.9 (Nunnally & Bernstein, Citation1994). Then, all observed variables are acceptable (more than 0.6, less than 0.9) and qualified for factor analysis based on the scale’s reliability analysis results by Cronbach’s Alpha coefficient (Table ).
Table 1. Result of Cronbach’s Alpha reliability coefficient
The Sig value = 0.000 and Kaiser-Meyer-Olkin (KMO) value = 0.892 are shown in the analytical findings of the KMO coefficient and Bartlett statistical value (Table ). This demonstrates that the observed variables are correlated. As a result, exploratory factor analysis (EFA) is recommended.
Table 2. Result of KMO coefficient and Bartlett’s statistical value
PAF (Principal Axis Factoring) extraction and POR (Promax Oblique Rotation) were used to conduct the EFA analysis. After analysis, the number of extracted factors is six, exactly as the study model proposed. However, the two detected variables, NL3 and XH5, were found to be invalid and were eliminated. Furthermore, because the load factor (Factor Loading) is greater than 0.5, the remaining 21 observed variables are acceptable. The overall value of variance discovered was 58.464 percent (> 50%), implying that these six factors account for 58.464 percent of the data variation. Therefore, when extracting six elements, the stopping point with an Eigenvalue = 1.023 (higher than 1) is acceptable.
Using the above exploratory factor analysis (EFA) results, confirmatory factor analysis (CFA) was performed on AMOS 24.0 software, and the following results were obtained (Figure ):
The indices must achieve a particular threshold to assess the model’s fit (Hair et al., Citation2010). The Chi-square/df (Chi-squared/degrees of freedom) = 0.893 (less than 3); GFI, CFI, and TLI indices are 0.953, 1,000, and 1.009, respectively (higher than 0.9); RMSEA = 0.000 (less than 0.08); PCLOSE = 1,000 (greater than 0.05). Furthermore, the p-value for each construct is less than 0.05, indicating that the correlation coefficient for each concept construct is different from 1 at the 95 percent confidence level. As a result, the indicators are satisfactory, and the study model is consistent with the survey results.
Convergent Validity, Discriminant Validity, and Reliability are required to guarantee that the factors do not create variations in the analytical results in the structural equation modeling (SEM), as well as to reflect the meaning of the data and reality. According to the test findings, the indicators all meet the standards in terms of value and dependability. As a result, this scale has been determined to be accurate and can be utilized for further structural equation modeling (SEM) studies.
4.2. Model & hypothesis testing
To test the posed hypotheses, two models were run. Model 1 had the direct effect of PE, EE, SI, and FC on online shopping behavior; Model 2 was an augmentation of Model 1 with GAI added as the mediating variable; this study calculated the indirect impact of GAI on the PE, EE, SI, FC→GAI →OSB path.
Structural equation modeling (SEM) analysis was performed with 288 samples, 95% confidence intervals, and 1000 repetitions. From that, based on the estimation coefficient (β), the degree of factors affecting the intention to accept gamification is: Facilitating Conditions (β = 0.268), Effort Expectancy (β = 0.257), Social Influence (β = 0.224), and Performance Expectancy (β = 0.202). Furthermore, the estimated coefficient (β = 0.544) is also important proof that the Gamification Adoption Intention factor positively influences Online Shopping Behavior. Thus, it can be concluded that this is a highly correlated and positive relationship. In addition, the findings of the Squared Multiple Correlation (R-squared) value also suggest that the model fits 54.1 percent of the data.
5. Discussion & implications
5.1. Theoretical implications
In general, the objectives put forth at the outset have been met. The research findings are a foundation for bolstering the rationality and viability of employing the UTAUT model. Based on the results of testing the research hypotheses, all four factors, Performance Expectancy, Effort Expectancy, Social Influence, and Facilitating Conditions, significantly impact the Gamification Adoption Intention, and this intention directly affects Online Shopping Behavior. This result confirms that the UTAUT theory is correct and can be used for various research topics.
It is noted that Facilitating Conditions are found to have the most significant positive effect on the Gamification Adoption Intention because it has the highest standardized beta coefficient of 0.2688. This finding discovery aligns with prior study that the intention to utilize mobile applications for online booking is influenced by the facilitating conditions established by institutions (Abou-Shouk & Soliman, Citation2021). Nevertheless, Ofosu-Ampong et al. (Citation2020) show mixed results regarding the impact of facilitating conditions on technology does not guarantee the acceptance of gamification in learning. This phenomenon can arise due to variations in the levels of technology adoption across different countries and sectors.
The present study reveals that there is a significant relationship between Effort Expectancy and Gamification Adoption Intention in the context of online shopping, with a correlation coefficient of 0.257. This result is also consistent with Venkatesh and Zhang (Citation2010), whose study indicated that effort expectancy has a stronger response to intention for women, large experienced age workers, and those with limited experience. Whereas, effort expectancy is one of the least predictors of consumption behavior and is derived from performance expectations in brand awareness and customer engagement (Abou-Shouk & Soliman, Citation2021). Overall, the results suggest that customers are encouraged to shop online when they find it easy to join the system.
Social Influence also positively affects the Gamification Adoption Intention and gets 0.224. This result suggests that if consumers are encouraged to participate by those who influence their behavior, such as family and friends, as well as supported by their significant others to use the service, there is more likelihood that they will adopt gamification. This result coincides with prior studies, such as indicating the relationship between social influence and the acceptance of gamification in education (Ofosu-Ampong et al., Citation2020), and the positive link between socializing and motivating individuals to use gamification apps in Egypt (Abou-Shouk et al., 2020).
The relationship between Performance Expectancy and Gamification Adoption Intention gets a 0.202 score in the standardized beta coefficient, which implies measuring consumer trust with gamification in their intention to shop online. In other words, information received from gamification will engage users and influence their intentions. In addition, this result is similar to the learner’s attributes on gamification adoption (Ofosu-Ampong et al., Citation2020); or in promoting perceived benefits achieved by consumers when engaged mainly male younger workers when improving performance (Venkatesh et al., Citation2003). This finding suggests that consumers will have the tension to adopt gamification if they recognize the gamified useful in spans enhancing their performance.
Generally, the above characteristics have a 54.1 percent impact on the willingness to adopt gamification. This helps confirm the close relationship between the factors affecting Gamification Adoption Intention and reinforces the proposed theory.
Moreover, the study’s findings also confirmed a link between Gamification Adoption Intention and Online Shopping Behavior. At the same time, they have a 29.6 percent impact on each other. This demonstrates that a variety of other factors continue to influence Online Shopping Behavior. It also agrees that gamification in marketing is currently popular but should not necessarily be used because it is new or to copy rivals. Gamification will only have the desired effect on businesses if the benefits are clearly articulated and there is a detailed implementation plan. Therefore, businesses should decide on the desired socio-economic benefits and carefully plan implementation before applying gamification.
Last but not least, this study proposes that game components and narratives be used to completely utilize users’ deep-rooted incentives, thereby motivating or modifying their behavior. This is supported by up to 98 people agreeing with the premise that “gaming will promote motivation and boost online purchasing,” and 82 people absolutely agree. The game’s components and mechanisms will stimulate people’s psychological factors. However, on the other hand, businesses are not obligated to include a specific number of ingredients in their gamification. Which components to utilize is determined by the emotions and behaviors businesses want their customers to have. As for narratives, these should include a sense of gradual progress, increasing difficulty, or a reaction to a cumulative sequence of events (Torres-Toukoumidis et al., Citation2022).
5.2. Managerial implications
In addition to its theoretical implications, this study has several practical implications that offer interested parties efficient, measurable gamification. First and foremost, businesses should examine gamification’s potential to interact with various technological devices before implementing it since Facilitating Conditions have the greatest impact on Gamification Adoption Intention. The fact that the game may be played on nearly any device creates several favorable situations for players. Businesses should resolve compatibility issues to ensure it works consistently with a range of devices and does place excessively large capacity or high internet access speed demands on devices. Second, there should be a means to raise and resolve technical issues because this is helpful to players and businesses in identifying game bugs. This functionality will enable businesses to deal with faults or technical issues more swiftly.
In terms of Social Influence, this study suggests that when adopting gamification solutions, businesses should promote them widely. If the majority of consumers are unaware of the business’s gamification, it will not attract the expected number of participants or gain viral traction. According to the study, 64 people highly agree, and 77 people agree that “the mass media (such as television, radio, newspapers, and social media) will influence my decision to join in games.” Consequently, excellent communications and marketing will help ensure a successful gamification strategy.
Next, the study suggests the following three options for Effort Expectancy. First, the game implementation mechanism, or, in other words, the game rules, should be thoroughly stated because this will have a direct impact on the simplicity with which gamification can be implemented. Users will understand what they need to do to fulfill the given tasks if the game’s rules are well explained. Second, the difficulty of gamification should be evaluated. Because the primary purpose of a game is to amuse, a difficult game will detract from the participant’s enjoyment. Third, aesthetics and user interface should be considered when creating games because these two factors will influence the user’s attention and retention from the start. Finally, it will influence user involvement with gamification if it is insufficiently developed or generates confusion and difficulties in use.
Moreover, gamers must be persuaded that gamification enhances their online purchasing experience and rewards them in order to increase the Performance Expectation factor. Studies showed that using game elements, including achievements, avatars, badges, boss fights, collections, combat, content unlocking, gifting, leaderboards, and levels, fully exploits players’ inherent motives and subsequently motivates or changes their behavior.
Finally, gamification’s ultimate purpose is influencing people to alter their online shopping behavior. Therefore, businesses must decide the behavior they want customers to express in accordance with the socio-economic benefits the business has outlined to convert gamification adoption intentions. For instance, sign in to the app or website each day, watch a brief video (which may be a TV commercial), purchase a specific item, receive a certain number of orders within a certain amount of time, and suggest the service to others. If these calls to action are cleverly incorporated into gamification, brand loyalty or lavishly spending money on a product unintentionally becomes the user’s “task.” That makes it entirely possible for the organization to achieve its goals (benefits) as outlined at the outset.
6. Conclusion
In conclusion, fully improving Performance Expectancy, Effort Expectancy, Social Influence, and Facilitating Conditions will also help boost the Gamification Adoption Intent. As a result, online shopping behavior improves.
However, in terms of content, this study has the following limitations. First, due to the short research time available, weak financial capacity, and the negative impacts of the COVID-19 pandemic, the study survey is limited by the number of samples. Second, the survey only focused on young people between the ages of 16 and 30. Therefore, the research results are not sufficiently inclusive. Third, this study only explains 29.6% of the impact of Gamification Adoption Intention on Online Shopping Behavior. This proves that many other factors affect Online Shopping Behavior, which further research still needs to clarify.
Disclosure statement
No potential conflict of interest was reported by the author(s).
Additional information
Funding
Notes on contributors
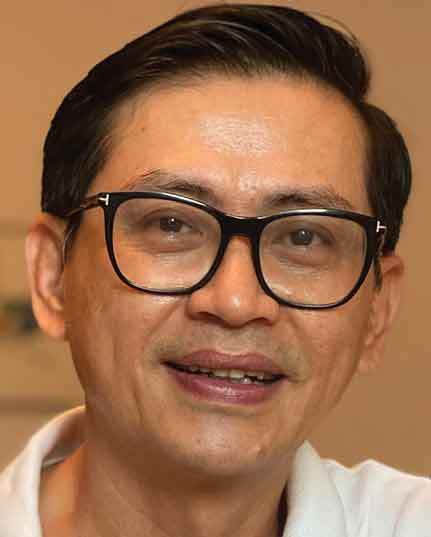
Dinh Tien Minh
Dinh Tien Minh holds PhD degree in Management at University of Economics Ho Chi Minh City and Master degree in Corporate Management at Université Lumière Lyon II, France. Started with doing research projects in education, Dr. Minh has widened his research fields by conducting a variety of empirical studies in marketing. He was the author and co-author of seven books on marketing and made more than twenty scientific publications such as textbook principle of marketing, international marketing and consumer behaviours as reference book. Dr. Minh is also known as a dynamic lecturer in marketing with more than twenty five years of lecturing at School of International Business – Marketing, University of Economics Ho Chi Minh City. During that period of time, he has involved in developing and teaching internal training courses for numerous enterprises ranging from entertainment, education, banking, advertising, communication, construction to pharmaceutical industry within Vietnam. Research interests: Retail, Commerce, Online, Customer Behavior, Media, Digital Transformation, Tourism. Email: [email protected].
Le Vu Lan Oanh
Le Vu Lan Oanh, a Solvay Brussels School - Université Libre de Bruxelles (ULB) Masters graduate, is currently pursuing a Ph.D. in Commerce at University of Economics Ho Chi Minh City, Vietnam. She is lecturer in Marketing major, focuses on Marketing Communications, Advertising, and Creative Thinking. Her research interest is in ethical practice, sustainability, consumer characteristics and marketing innovation. She is looking forward to collaborating, exchanging ideas, and learning from other scholars. University of Economics Ho Chi Minh City email: [email protected]; Van Lang University email: [email protected].
References
- Abou-Shouk, M., & Soliman, M. (2021). The impact of gamification adoption intention on brand awareness and loyalty in tourism: The mediating effect of customer engagement. Journal of Destination Marketing & Management, 20, 100559. https://doi.org/10.1016/j.jdmm.2021.100559
- Archana, B. (2012). A case study on retailing in India. EXCEL International Journal of Multidisciplinary Management Studies, 2(7), 178–13. .
- Berndt, A. E. (2020). Sampling methods. Journal of Human Lactation, 36(2), 224–226. https://doi.org/10.1177/0890334420906850
- British, C. (2020). Next generation Vietnam. https://www.britishcouncil.vn/en/programmes/society/next-generation-vietnam
- Bui, T. T. H., & Bui, H. T. (2018). Gamification impact on the acceptance of mobile payment in Ho Chi Minh City, Vietnam. International Journal of Social Science and Economic Research, 3(9), 4822–4837. .
- Chowdhury, M. S., & Ahmad, N. (2012). Factors affecting consumer participation in online shopping in Malaysia: The case of university students. European Journal of Business and Economics, 5. https://doi.org/10.12955/ejbe.v5i0.171
- Clement, J. (2019). E-commerce worldwide-statistics & facts. Statista Recuperado el, 5. https://www.statista.com/topics/779/mobile-internet
- Davis, F. D., Bagozzi, R. P., & Warshaw, P. R. (1989). User acceptance of computer technology: A comparison of two theoretical models. Management Science, 35(8), 982–1003. https://doi.org/10.1287/mnsc.35.8.982
- Drugău-Constantin, A. L. (2019). Is consumer cognition reducible to neurophysiological functioning? Economics, Management, & Financial Markets, 14(1), 9–15. https://doi.org/10.22381/EMFM14120191
- Foon, Y. S., & Fah, B. C. Y. (2011). Internet banking adoption in Kuala Lumpur: An application of UTAUT model. International Journal of Business & Management, 6(4), 161. https://doi.org/10.5539/ijbm.v6n4p161
- Forsythe, S., Liu, C., Shannon, D., & Gardner, L. C. (2006). Development of a scale to measure the perceived benefits and risks of online shopping. Journal of Interactive Marketing, 20(2), 55–75. https://doi.org/10.1002/dir.20061
- García-Jurado, A., Torres-Jim’enez, M., Leal-Rodríguez, A. L., & Castro-Gonzalez, P. (2021). Does gamification engage users in online shopping? Electronic Commerce Research and Applications, 48, 101076. https://doi.org/10.1016/j.elerap.2021.101076
- Ghalandari, K. (2012). The effect of performance expectancy, effort expectancy, social influence and facilitating conditions on acceptance of e-banking services in Iran: The moderating role of age and gender. Middle-East Journal of Scientific Research, 12(6), 801–807. https://doi.org/10.5829/idosi.mejsr.2012.12.6.2536
- Hair, J. F., Black, W. C., Babin, B. J., & Anderson, R. E. (2010). Multivariate Data Analysis (7th ed.). Prentice Hall.
- Hair, J. F., Ringle, C. M., & Sarstedt, M. (2011). PLSSEM: Indeed a Silver Bullet. Journal of Marketing Theory & Practice, 18(2), 139–152. https://doi.org/10.2753/MTP1069-6679190202
- Hofacker, C. F., De Ruyter, K., Lurie, N. H., Manchanda, P., & Donaldson, J. (2016). Gamification and mobile marketing effectiveness. Journal of Interactive Marketing, 34, 25–36. https://doi.org/10.1016/j.intmar.2016.03.001
- Huang, T., Bao, Z., & Li, Y. (2017). Why do players purchase in mobile social network games? An examination of customer engagement and of uses and gratifications theory. Program, 51(3), 259–277. https://doi.org/10.1108/PROG-12-2016-0078
- Huotari, K., & Hamari, J. (2017). A definition for gamification: Anchoring gamification in the service marketing literature. Electronic Markets, 27(1), 21–31. https://doi.org/10.1007/s12525-015-0212-z
- Hwang, H., Malhotra, N. K., Kim, Y., Tomiuk, M. A., & Hong, S. (2010). A comparative study on parameter recovery of three approaches to structural equation modeling. Journal of Marketing Research, 47, 699–712. https://doi.org/10.1509/jmkr.47.4.699
- Im, I., Hong, S., & Kang, M. S. (2011). An international comparison of technology adoption: Testing the UTAUT model. Information & Management, 48(1), 1–8. https://doi.org/10.1016/j.im.2010.09.001
- Kevin, W., & Dan, H. (2012). For the win: How game thinking can revolutionize your business. Wharton Digital Press.
- Kulviwat, S., Thaku, R., & Guo, C. (2006). An exploratory study of consumer adoption of online shipping: Mediating effect of online purchase intention. International Journal of E-Business Research (IJEBR), 2(2), 68–82. https://doi.org/10.4018/jebr.2006040105
- Liang, T.-P., & Lai, H.-J. (2000). Electronic store design and consumer choice: An empirical study. Proceedings of the 33rd Annual Hawaii International Conference on System Sciences. IEEE.
- Liu, D., Santhanam, R., & Webster, J. (2017). Toward meaningful engagement: A framework for design and research of gamified information systems. MIS Quarterly, 41(4), 1011–1034. https://doi.org/10.25300/MISQ/2017/41.4.01
- Meeker, M. (2019). Internet trends. (Retrieved June 11, 2019), from https://www.bondcap.com/#view/1
- Mohammadi, D. (2014). How online gamers are solving sciences biggest problems. The Guardian, 25.
- Nunnally, J. C. (1978). Psychometric theory. McGraw-Hill.
- Nunnally, J. C., & Bernstein, I. H. (1994). Psychometric theory (3rd ed.). McGraw-Hill.
- Ofosu-Ampong, K., Boateng, R., Anning-Dorson, T., & Kolog, E. A. (2020). Are we ready for gamification? An exploratory analysis in a developing country. Education and Information Technologies, 25(3), 1723–1742. https://doi.org/10.1007/s10639-019-10057-7
- Reinartz, W. J., Haenlein, M., & Henseler, J. (2009). An empirical comparison of the efficacy of covariance-based and variance-based SEM. International Journal of Market Research, 26(4), 332–344. https://doi.org/10.1016/j.ijresmar.2009.08.001
- Roscoe, D. (1975). New methods for the derivation of stable difference representations for differential equations. IMA Journal of Applied Mathematics, 16(3), 291–301. https://doi.org/10.1093/imamat/16.3.291
- Ruengaramrut, V., Ribiere, V., & Mariano, S. (2020). The moderating effect of gamification on the relationship between customer engagement and new service development process involvement. International Journal of Innovation and Learning, 27(1), 93–119. https://doi.org/10.1504/IJIL.2020.103895
- Saha, R., Manna, R., & Geetha, G. (2012). Captchino-a gamification of image-based captchas to evaluate usability issues. Proceedings of the 2012 International Conference on Computing Sciences. IEEE.
- Sahin, O., Salim, H., Suprun, E., Richards, R., MacAskill, S., Heilgeist, S., Rutherford, S., Stewart, R. A., & Beal, C. D. (2020). Developing a preliminary causal loop diagram for understanding the wicked complexity of the COVID-19 pandemic. Systems, 8(2), 20. https://doi.org/10.3390/systems8020020
- Sheppard, B. H., Hartwick, J., & Warshaw, P. R. (1988). The theory of reasoned action: A meta-analysis of past research with recommendations for modifications and future research. Journal of Consumer Research, 15(3), 325–343. https://doi.org/10.1086/209170
- SRVN, S. R. O. V. N. (2020). Youth Law. Socialist Republic of Viet Nam. https://thuvienphapluat.vn/van-ban/Van-hoa-Xa-hoi/Luat-thanh-nien-so-57-2020-QH14-416260.aspx
- Taylor, S., & Todd, P. (1995). Decomposition and crossover effects in the theory of planned behavior: A study of consumer adoption intentions. International Journal of Research in Marketing, 12(2), 137–155. https://doi.org/10.1016/0167-8116(94)00019-K
- Teotónio, N., & Reis, J. L. (2018). The gamification systems application elements in the marketing perspective. Proceedings of the World Conference on Information Systems and Technologies. Springer International Publishing.
- Tobon, S., Ruiz-Alba, J. L., & García-Madariaga, J. (2020). Gamification and online consumer decisions: Is the game over? Decision Support Systems, 128, 113167. https://doi.org/10.1016/j.dss.2019.113167
- Torres-Toukoumidis, A., León, D. V., De Santis, A., & López-López, P. C. (2022). Gamification in ecology-oriented mobile applications—typologies and purposes. Societies, 12(2), 42. https://doi.org/10.3390/soc12020042
- Venkatesh, V., Morris, M. G., Davis, G. B., & Davis, F. D. (2003). User acceptance of information technology: Toward a unified view. MIS Quarterly, 27(3), 425–478. https://doi.org/10.2307/30036540
- Venkatesh, V., & Zhang, X. (2010). Unified theory of acceptance and use of technology: US vs. China. Journal of Global Information Technology Management, 13(1), 5–27. https://doi.org/10.1080/1097198X.2010.10856507
- Wang, J., Li, X., Wang, P., Liu, Q., Deng, Z., & Wang, J. (2021). Research trend of the Unified theory of acceptance and use of technology theory: A bibliometric analysis. Sustainability, 14(1), 10. https://doi.org/10.3390/su14010010
- Wen, D. M. H., Chang, D. J. W., Lin, Y. T., Liang, C. W., & Yang, S. Y. (2014). Gamification design for increasing customer purchase intention in a mobile marketing campaign app. Proceedings of the International Conference on HCI in Business. Springer International Publishing.
- Xi, N., & Hamari, J. (2020). Does gamification affect brand engagement and equity? A study in online brand communities. Journal of Business Research, 109, 449–460. https://doi.org/10.1016/j.jbusres.2019.11.058
- Xu, Y., Chen, Z., Peng, M. Y.-P., & Anser, M. K. (2020). Enhancing consumer online purchase intention through gamification in China: Perspective of cognitive evaluation theory. Frontiers in Psychology, 11. https://doi.org/10.3389/fpsyg.2020.581200