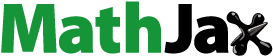
Abstract
Credit risk in financial institutions has been widely debated among experts and legislators as a substantial risk to the financial industry’s health. In addition to examining bank-specific and macroeconomic reasons, the study examines the impacts of political institutions and macroprudential regulations on credit risk in Ethiopia. Using panel data from 2011–2019 and employing dynamic ordinary least squares, the findings imply that political institutions have significant positive links to credit risk. It also found that macroprudential policy helps to control bank risk and reduce exposure in the banking industry. The results indicate that banks with larger sizes and profitability have less credit risk. The findings support the’skimping’ and “dark side of diversification” hypotheses; retaining a higher loan-to-asset ratio, cost-effectiveness, and diversity of the banking commercial model reduces credit quality by promoting credit risk. A higher inflation rate reduces credit risk by increasing borrowers’ potential to service their debts when lending rates are fixed. GDP and the exchange rate have little influence on credit risk. The outcomes of this study provide significant implications for authorities and bankers in developing countries such as Ethiopia in the understanding of credit risk-determining elements to implement prudential rules and remedial management policies.
Public Interest Statement
Credit risk in financial institutions has been widely debated among experts and legislators as a substantial risk to the financial industry’s health. The banks experience lower profit margins due to increasing nonperforming loans, and if the issue worsens, a crisis may result. The banking sector’s nonperforming loans are still at the forefront of severe bank failures in developing and developed countries. This study insight on the determinants of credit risk, how the political structure of the country and macroprudential policy affect the credit risk. This helps for authorities and bankers to implement prudential rules and remedial management policies.
1. Introduction
The banking industry plays an important role in the growth and stability of the global economy. Significant crises in finance in recent years have affected the banking industry worldwide, particularly within emerging nations, causing financial shocks and volatility in financial markets (Khan et al., Citation2020). Credit risk, which can be quantified by nonperforming loans (hereafter NPLs), is primarily what causes the banking industry to fail. Loans that have been missing repayments for at least three months are referred to as nonperforming loans or bad loans (Freeman & Bloem, Citation2005). An increasing NPL ratio indicates an unstable banking system (Khan et al., Citation2020). An ongoing increase in NPLs has a negative impact on the financial system’s liquidity, hinders interest earnings, reduces investment opportunities, and increases the risk of a financial meltdown (Louzis et al., Citation2012; Nkusu, Citation2011), limiting the ability of the bank to raise capital (Boussaada et al., Citation2020, Citation2020).
For the stability of finances and the efficient operation of an economy, it is therefore vital to determine the parameters that influence NPLs to reduce the volume of NPLs. Because the financial crisis of 2008 showed that microprudential regulation is ineffective at averting banking crises, financial stability and regulations continue to be major priorities for legislators across the globe (Allen & Gu, Citation2018; Atellu et al., Citation2021). The Basel Committee harmonises critical credit quality management standards (Adeolu, Citation2014). However, the 2007–2008 global financial crisis highlighted inadequate credit risk management, resulting in a wave of credit risk that affected banks in developed and developing countries (Ghosh, Citation2015). Thus, macroprudential regulation has emerged as the spotlight in both developing and advanced nations (Akinci & Olmstead Rumsey, Citation2018; Atellu et al., Citation2021).
Macroprudential measures increase the risk-adjusted performance of financial firms or creditors, while various of these measures are related to the credit cycle (such as the countercyclical capital buffer), which aims to establish safeguards during periods of strong economic growth to mitigate the economic downturn (Tatarici et al., Citation2020). However, the assessment of macroprudential regulations’ attainment presents an initial obstacle, mainly when many tools are in use. The second aspect is the variety of macroprudential objectives and procedures. The regulators’ perception of the exposures involved and their level of confidence in their assessment will determine which instruments to employ, how to set up properly, and when to utilise them (Altunbas et al., Citation2018).
Furthermore, there may be significant policy compromises between stability and financial regulations. More stringent financial restrictions are required to support financial security. As a result, institutions will be equipped to build substantial buffering to handle any financial turmoil (Delis, Citation2015). On the other hand, strict regulations may trigger the banking sector to become unstable. Regulations’ burdens might result in increased implicit costs for the expansion of financial firms (Atellu et al., Citation2021). These results indicate that appropriate policies continue to be a controversial subject (Carreras et al., Citation2018), and our comprehension of the impact of macroprudential policy on credit risks remains limited (Akinci & Olmstead Rumsey, Citation2018). Insufficient evidence supports the usefulness of MPPs for addressing financial risk factors (Atellu et al., Citation2021; Carreras et al., Citation2018; Dutta & Saha, Citation2020).
Formal and informal entities, especially those that vary throughout nations, will also have an impact on the outside and inside working environments of banks. In this regard, previous research has highlighted legal institutions and the banking industry’s rules as major factors influencing bank risk-taking practices (Ashraf, Citation2017). The Center for the Study of Financial Innovation (CSFI) and PricewaterhouseCoopers (PwC) worked together to conduct the Banking Banana Skins survey, which supported the worth of political structures towards financial institutions. They assessed “political interference” in financial institutions as the most significant risk by hurting banks and distorting the market (Liu & Ngo, Citation2014).
Additionally, building on recent political economy literature, Ashraf (Citation2017) provides evidence that stronger political institutions put banking at greater risk by raising competition in loan markets from additional funding sources and creating moral hazard issues because people expect government bailouts in the worst economic times. Furthermore, Papanikolaou (Citation2018) illustrates that larger and more complicated banks are more inclined to qualify for bailouts owing to lower failure rates and that regulators are more likely to help a banking institution in trouble if it has strong ties to political figures and parties. How political institutions have an influence on the banking sector’s credit risk areas is notably scarce within the previous study. The objective of the current study is to address this important knowledge gap.
In this setting, utilising political institutions to address the gaps missing from prior investigations is important. A number of drivers thus support the significance of this study on the Ethiopian financial system. First, because most past research has focused on advanced and emerging banking sectors, the findings from developed countries cannot be applied to developing countries. This is because banks differ in terms of legal systems and other country-specific requirements (Adem, Citation2022a). Second, Ethiopia’s financial regulatory framework is weaker and less established than its neighbours in the region. Although the central bank of Ethiopia has several existing macroprudential regulatory tools, there is a lack of macroprudential monitoring systems to address mitigating local and international credit risks. Certain additional Basel III (the capital surcharge) instrument components are also not being utilised (World Bank, Citation2019). Therefore, it is unclear how credit risk and macroprudential regulation interact to ensure the banking system’s resilience, prompting the need for the present investigation. Third, the Ethiopian economy has been dominated by banks and has a small, undeveloped financing sector (World Bank, Citation2019). As a result, any disruption that affects the banking industry might have an impact on the financial system as a whole. Thus, this study aimed to identify the influence of macroprudential policy and political institutions on the Ethiopian banking sector’s credit risk from 2011 to 2019.
This paper contributes to the existing body of knowledge in the following aspects. First, to the best of the researcher’s knowledge, no other research has investigated the influence of political institutions and macroprudential policy on credit risk in Ethiopia. Second, because the number of studies on credit risk determinants considers bank-specific or macroeconomic factors, this article analyses both factors and macroprudential policy with political institutions as other determinant factors. Finally, it contributes to the present literature by investigating the effects of macroprudential policies on credit risk minimisation.
This paper is organised as follows. First, the relevant literature is reviewed in section 2. Then, section 3 presents the data and methodology. Section 4 provides the empirical results and discussion. Finally, section 5 presents concluding remarks and policy implications.
2. Literature review
When the principal and interest payments are 90 days or longer overdue, the loan is considered nonperforming (NPL) (Freeman & Bloem, Citation2005). Banks experience lower profit margins due to more NPL, and if the issue worsens, a crisis may result. The banking sector’s nonperforming loans are still at the forefront of severe bank failures in developing and developed nations (Ghosh, Citation2015). Therefore, for banks to effectively manage this role, it is crucial to look at the main factors influencing credit risk. This study describes four substantial sets of factors that explain credit risk: bank specific, macroeconomic, political institution, and macroprudential policy.
As discussed by (Bernanke et al., Citation1998) financial accelerator theory provides a framework to connect NPLs with macroeconomic indicators. Bernanke et al. (Citation1998) introduced the notion of the financial accelerator. They argued that credit markets are typically cyclical and, therefore, that asymmetric information between lenders and borrowers and financial statement influence serves to magnify and transmit credit market disruptions to the economy. Relaxing lending rules and robust credit growth are also caused by competitive pressure and confidence in macroeconomic prospects, laying the seeds of future financial disasters for both borrowers and lenders. The current legal and supervisory structure affects how lending criteria are relaxed during economic expansions. Increased caution among lenders and a tightening of loan extension during downturns cause higher anticipated NPL percentages as well as a decrease in the value of collaterals. These factors negatively influence domestic demand (Nkusu, Citation2011).
The factors that affect the NPL ratio have received much theoretical and empirical study. The specific bank features make up most of the factors determining a bank’s NPL ratio. Decisions and actions made by shareholders and managers may directly affect these traits (Lee et al., Citation2020). Numerous bank management theories about loan quality, cost-effectiveness, and bank capital were put to the test by Berger and DeYoung (Citation1997). They proposed moral hazard and poor management theories as possible explanations for NPLs. As substitutes for poor management, bank-level variables could be utilised, including bank size, loan quality, and cost-effectiveness or profitability (Kuzucu & Kuzucu, Citation2019).
The currently available research identifies political institutions as important determinants of bank risk (Ashraf, Citation2017). Political structures serve as compliance checks determining the government’s deliberate functional arrangement. If it has consistently bailed out banks, it will undoubtedly continue to do so within the bounds of the law. Political institutions can influence bank risk in various ways that have first-order consequences. The first way is via how they affect the “risk of government expropriation.” For example, politicians may demand bribes, establish bank monopolies that split rent with them, award licences to favoured parties, or compel banks to lend money to businesses with political links (Ashraf, Citation2017). They may utilise predatory taxing practices, maintain board seats, or support banks to obtain a position in these institutions later. They may also influence contract enforcement when some important organisation is involved. In addition, increasing expropriation may result in higher bank risk due to increased loan defaults to politically connected businesses and the inefficiencies associated with monopolies or favored groups. Reduced “adverse selection” is a second way political institutions might affect bank risk. Adverse selection occurs when borrowers with bad credit are more likely than those with good credit to be approved for loans (i.e., firms with less inherent risk and better investment opportunities). Adverse selection is a severe credit issue since lenders and borrowers have asymmetrical knowledge.
By enhancing the information environment, political institutions may reduce informational asymmetries. A more open atmosphere would make it easier for bank management to assess borrowers’ creditworthiness, reducing the possibility of discriminatory hiring. The degree of autonomous government entities that significantly impact the degree of restrictions on a policy formulation decision is determined using the Political Constraints Index (Henisz, Citation2015), which was developed to measure political institutions. The index of the political constraint ranges from 0 to 1. In political systems that constrain the ruling body’s decision, higher ratings suggest significant political restrictions, more autocratic political institutions, and separate ministries with legislative authority (Ashraf, Citation2017). Other political institution indicators, such as political rights, have been applied to fully evaluate the dependability of the results. Political rights, which stand in for political risk and probable outcomes of political discussions that affect borrowers’ and lenders’ future potential costs and benefits considerations, are also used to assess integrity and competitiveness. Information on political rights was gathered from the Freedom-house (Citation2020) Political Rights Index based on the study of (Adem, Citation2022b). This study depends on Ashraf’s (Citation2017) research and fills a gap on whether political structures affect bank credit risk. As a result, the research proposed the following:
H1:
Political institutions have a significant impact on credit risk.
According to a generally used definition, “macroprudential policies are intended to detect and reduce threats to systemic stability, therefore lowering the cost to the economy from a disruption in financial services that support the operation of the financial sector” (Altunbas et al., Citation2018). Thus, the vigorous application of macroprudential regulations might reduce banks’ risk-taking behaviour. For example, capital-based tools such as risk-based capital cushions could enable institutions to generate resources during prosperous times, which may subsequently be utilised to absorb losses during stressful times (Altunbas et al., Citation2018). Together with capital control, MPPs have been proven to be helpful in preventing financial vulnerabilities brought on by domestic and/or foreign financing. MPPs can also help to enhance stability and lessen the likelihood of a financial crisis and its effects (Korinek & Sandri, Citation2016). By lowering systemic risk, strict MPPs support the viability of the banking industry. The risk of increased lines of credit entering a bank’s financial statements during economic expansion and the threat of lending to businesses with good investment prospects during distress can both be avoided by MPPs (Zhang et al., Citation2018). According to Dutta and Saha (Citation2020), macroprudential policies are more effective in emerging economies and in normal times. In contrast, Dutta and Saha (Citation2020) stated that extra regulations and business constraints may limit economic growth and impede the banking industry’s profitability. The study of (Rubbaniy et al., Citation2022) demonstrates significant insights regarding how to maintain a viable and effective financial system by reducing regulatory constraints and altering the policy rate amid the business cycle’s economic contraction phases.
Kodongo (Citation2018) asserts that MPPs might “unknowingly impair the effective financial intermediary operation” of banking institutions. Due to its thorough inclusion, which records the usages of twelve macroprudential tools in 160 countries, the study employed Cerutti et al. (Citation2017) macroprudential policy dataset to measure MPPs per earlier literature (Altunbas et al., Citation2018, Zhang et al., Citation2018; Saha & Dutta, Citation2020). The tools include the general countercyclical capital buffer/requirement, the leverage ratio for banks, the loan-to-value ratio, the debt-to-income ratio, the limits on domestic and foreign currency loans, the reserve requirement ratios, the tax on financial institutions, the capital surcharges on systemically important financial institutions, the limits on interbank exposures, and the concentration limits (Saha & Dutta, Citation2020). The dataset is built using simple binary measurements of whether the instruments were detected in a certain country-year case based on these instruments (Altunbas et al., Citation2018). As a result, the research proposed the following:
H2:
Macroprudential policy has a significant negative impact on credit risk.
The literature considers that the share of loans is a significant source of NPL. Naili and Lahrichi (Citation2022) suggested that loan expansion was one of the primary causes of the recent financial crisis. According to previous studies, excessive lending (measured by the loan-to-asset ratio) contributes to higher NPLs due to asymmetric information problems and adverse selection (Alhassan et al., Citation2014; Baselga-Pascual et al., Citation2015; Ghosh, Citation2015; Klein, Citation2013). Mishra et al. (Citation2020) and Nikolaidou and Vogiazas (Citation2017) find that the loan-to-asset ratio and NPL are going in the same direction. Therefore, to confirm or reject the previous empirical evidence, the study formulates the following hypothesis:
H3:
The loan-to-asset ratio has a significant positive impact on banks’ credit risk.
Following the “bad management” hypothesis, Berger and DeYoung (Citation1997) argued that profitable banks have less incentive to participate in high-risk investments, thus reducing their NPL to improve the quality of loans (Abusharbeh, Citation2020; Chaibi & Ftiti, Citation2015; Klein, Citation2013; Louzis et al., Citation2012). In contrast, Ali et al. (Citation2022) pointed out that inadequate performance in the banking industry is connected with low liquidity generation since a larger number of loans raises potential loan losses and a higher volume of deposits increases vulnerability to financial institution collapses. Moreover (Abbas et al., Citation2021), found a significant relationship between credit risk and commercial banks’ profitability in Asian emerging economies. Due to these contradicting claims, it is necessary to conduct a more thorough investigation and formulate the following hypotheses regarding how return on assets affects the amount of NPLs:
H4:
Bank profitability has a significant negative impact on banks’ credit risk.
The cost-to-income ratio measures operating efficiency (Louzis et al., Citation2012; Swami et al., Citation2019). A positive correlation between low-cost productivity and NPLs is supposed to be a “bad management” hypothesis (Berger & DeYoung, Citation1997). This is because bad managers with poor credit rating skills and monitoring defaulters increase costs. At the other extreme, the “skimping hypothesis” argues that high-cost efficiency could hurt NPLs. This is because banks that choose never to spend adequate resources to maintain loan quality may appear to be cost-effective; in turn, there may be a significant level of credit risk in the long run. The study by Swami et al. (Citation2019), Louzis et al. (Citation2012), Baselga-Pascual et al. (Citation2015), and Chaibi and Ftiti (Citation2015) provided evidence to support poor operational efficiencies going to increase NPLs by decreasing the quality of loans.
H5:
Bank operational inefficiency significantly impacts banks’ credit risk.
The natural logarithm of the total bank assets was used to calculate the bank’s size. Another pathway connecting bank-specific characteristics with NPLs is the moral hazard of too-big-to-fail banks. Policymakers are worried that large banks could use too much leverage and give loans to customers with bad credit (Louzis et al., Citation2012). Empirical studies have found a positive affiliation between credit risk and bank size (Abid et al., Citation2014; Chaibi & Ftiti, Citation2015; Louzis et al., Citation2012; Swami et al., Citation2019). These studies argued that larger banks are subject to a moral hazard problem and diseconomies of scale and appear to be more volatile and thus have more NPLs. Hence, the study hypothesised the following:
H6:
Bank size has a significant impact on banks’ credit risk.
Diversification comprises noninterest income through trading, contracts, service charge activities, and portfolio management. As per supporters of portfolio theory, banks can reduce firm-specific risk by diversifying their portfolios. Accordingly, diversifying a bank’s revenue reduces collateral risk and improves the profitability of loans (Alhassan et al., Citation2014; Ghosh, Citation2017). On the other hand, some studies explain their findings by citing the “dark side” of diversifying. This holds that banks that grow into new products seem to be more likely to suffer larger loan losses owing to the increased risk and do not always ensure a lower percentage of nonperforming loans (Ghosh, Citation2015; Louzis et al., Citation2012; Salas & Saurina, Citation2002; Stiroh, Citation2004). Consequently, the association listed below is anticipated based on previous studies.
H7:
Bank diversification has a significant negative impact on credit risk.
From macroeconomic determinants, a growing economy is likely to be associated with rising incomes and reduced financial distress (Nkusu, Citation2011). This is because throughout the stimulative phase of the economy’s prosperity, debtors will have a steady stream of adequate income to pay back their debts (Louzis et al., Citation2012). However, due to the decline in asset values used as collateral during difficult times, borrowers are more likely to fail on their obligations, which results in a rise in nonperforming loans. Furthermore, the empirical findings of many studies confirm an adverse effect of real GDP on NPLs (Salas & Saurina, Citation2002; Fofack, Citation2005; Alhassan et al., Citation2014; Amuakwa-Mensah et al., Citation2017; Aswini K; Mishra et al., Citation2020; Twum et al., Citation2021). Hence, based on these findings, the study hypothesises the following:
H8.
GDP growth has a significant negative impact on banks’ credit risk
A price spiral for goods and services over a specific period in a given economy is referred to as inflation. Since earnings in developing markets are often sticky and the quantity of NPLs rises, central bankers’ foremost worry is inflation (Naili & Lahrichi, Citation2022). While low inflation promotes economic growth, excessive inflation reduces the borrower’s real income and capacity to repay the loan. Empirically, many studies find a positive effect of inflation on NPLs (Aswini K Mishra et al., Citation2020, Boussaada et al., Citation2020b; Twum et al., Citation2021). Other pieces of literature support opposing views. Nkusu (Citation2011) and Hajja (Citation2020) document that inflation decreases the value of outstanding debts, improving borrowers’ repayment capacity. Thus, the relationship between NPLs and inflation is hypothesised as follows:
H9.
Inflation has a positive impact on banks’ credit risk
In developing economies, exchange rate volatility creates economic instability. The recent research conducted by (Umar & Sun, Citation2018) found that the exchange rate is one of the critical and significant determinants of NPLs. An exchange rate appreciation may weaken the competitiveness of export-oriented companies and adversely affect their ability to repay their debts (Fofack, Citation2005). On the other hand, if the home currency appreciates, it becomes easier for consumers who have loans in foreign currencies to service debt (Amuakwa-Mensah et al., Citation2017). These mixed findings further motivate the need for more empirical work on the macroeconomic determinants of credit risk.
H10:
The exchange rate has a significant impact on credit risk.
A large number of studies have identified macroeconomic and bank-industry-specific elements that affect NPLs. However, the relationship between NPLs and these factors is not clear. Some researchers concluded that these factors have positive relationships, while others rejected their results. In addition, there is a great dearth of research initiatives considering the effect of political institutions. Considering this empirical gap, this study aims to explore the effectiveness or adverse effect of political institutions and macroprudential policy on credit risk in the Ethiopian context.
3. Data and methodology
3.1. Population and data
According to the national banks of Ethiopia (2021), by the end of 2020/21, the number of banks increased to 19, including the newly opened interest-free bank (ZamZam bank). They are not listed in the stock market because there is no stock market. However, in 2021, Ethiopia passed a new Proclamation on establishing Financial Markets. In terms of banking industry advancements, the banking system’s deposit balances were Birr 187.3 billion. Private bank participation in financial intermediation decreased somewhat from 33.3 percent to 31.9 percent this year. Nevertheless, deposit holdings for the banking industry reached Birr 1 trillion, representing a yearly increase of 15.8 percent for 2019–2020.
At the end of 2011/12, the banking sector mobilised a total of Birr 89.2 billion from deposits, loan repayments, and lending. At the end of 2019–20, it reached Birr 333.4 billion. In addition, the banking industry collected Birr 35.2 billion in loans, accounting for 42.5 percent of all loans disbursed. Banks collected 183.3 billion in loans in 2019–20, with private banks accounting for 50.7 percent of all loans given.
Secondary data were gathered from the respective 12 commercial banks and national banks of Ethiopia from 2011–2019. For sample banks, it was necessary to have information for at least nine consecutive years during the period considered for the variables analysed. Therefore, banks with a record of fewer than nine years are not included in the sample. This is because they either lack complete data for the sample period or may not be enough to affect the outputs significantly. As long as it includes approximately 75% of the whole population, the sample size is adequate to draw reliable conclusions about populations from samples. The selection of variables relies on the literature review and data availability. Therefore, the collected panel data were analysed by using descriptive statistics and panel model regression.
3.2. Econometric model specification
The investigator seeks to identify macroeconomic, bank specific, macroprudential policy, and political institution determinants of credit risk in Ethiopia. The models are expressed mathematically by the following equation:
Y= represents the dependent variable, where i = entity and t = time. X=represents one independent variable, β=is the coefficient for that independent variable, and uit is the error term.
Estimating EquationEquation (1)(1)
(1) using traditional regression techniques such as OLS estimates could suffer from serial correlation and heteroskedasticity. Estimating EquationEquation (1)
(1)
(1) using fixed and random effects also fails to address endogeneity issues (Adem, Citation2022a). There may be an issue with endogeneity or reverse causality between political institutions in the government and credit risk. Governments are more inclined to respond to a worsening economic shock by offering bailouts to prevent the worst economic conditions and maintain popular support for the government among the people and depositors. Meanwhile, these government initiatives will probably cause moral hazard issues and push banks to take on greater risk when circumstances are good (Adem, Citation2022b; Ashraf, Citation2017).
Additionally, reverse causation could occur if nations with greater risk design MPP policies to reduce that risk (Saha & Dutta, Citation2020). Second, omitted variable bias would arise because additional factors might be used to explain credit risk. Third, endogeneity induced by measurement error could exist (Adem, Citation2022a). Therefore, panel Fully Modified Ordinary Least Square (FMOLS) and panel Dynamic Ordinary Least Square (DOLS) are better than OLS and other static panel models for a variety of reasons: OLS estimates may suffer from serial correlation and heteroskedasticity; OLS does not account for endogeneity; OLS estimator has a nonnegligible bias in small samples for estimating the cointegrated panels (Tugcu, Citation2018). However, the FMOLS and DOLS estimation techniques can avoid endogeneity in the regression coefficients and serial correlation in the error terms. FMOLS is an estimator that (Phillips & Hansen, Citation1990) devised to solve the issues brought on by the long-run connection between the cointegrating equation and the stochastic regressor innovations.
While the DOLS estimator to a panel dataset was expanded by (Kao & Chiang, Citation2000), they realised that DOLS is a superior predictor for both homogeneous and heterogeneous panels. This is due to the FMOLS estimator’s overall lack of improvement over the OLS estimator. The significant failure of the nonparametric correction for FMOLS could be severe (Lau et al., Citation2019). These results suggest that when the covariates are nonstationary (I(1)) and cointegrated, the DOLS model may be more effective than the OLS or FMOLS estimators (Kao & Chiang, Citation2000). The dynamic ordinary least squares (DOLS) model corrects endogeneity by including the regressor’s leads and lags in the static regression (Kao & Chiang, Citation2000). In addition, panel DOLS captures cross-sectional dependence’s influence through common time effects (Pedroni, Citation2001). Hence, the study made use of the panel dynamic ordinary least squares (DOLS) estimation techniques provided by Kao and Chiang (Citation2000) to estimate EquationEquation (2)(2)
(2) .
where Yit denotes the credit risk proxies by NPL for bank i in annual t, γit denotes the augmented lag and lead differences coefficients, which account for potential endogeneity and serial correlation problems. Xit is a 2 × 1 vector of independent variables, and εit is the error term.
where NPLs denotes nonperforming loans, LTA represents loan-to-asset, BS is the size of the bank, ROA is the ratio of return on assets, OE is operational efficiency, INDIV is the ratio of noninterest income to total income, GDP is the annual GDP growth rate, INF represents the inflation rate, EXR denotes the annual exchange rate, POI represents political institutions, MPP represents macroprudential policy, α represents the time-invariant unobserved effect, and Єi is the time-variant error term for bank i at time t.
Before applying DOLS, checking whether the data series of every dependent and predictor variable are stationary at the level is mandatory. Regression models for nonstationary variables generally offer spurious estimation results unless the variables are cointegrated, whereby the model eliminates stochastic trends to produce stationary residuals. It is necessary to confirm that all data series for dependent and explanatory parameters are stationary at the level prior to performing DOLS (Kao & Chiang, Citation2000). To check panel unit root tests, cross-sectional dependency defines a next step to be taken to verify panel unit root testing. The common panel unit root test, which ignores cross-sectional interdependence, may be skewed if there is considerable cross-sectional dependence (Tugcu, Citation2018). Therefore, cross-sectional dependency in the data could be tested using the second generation of unit root tests, such as the cross-sectionally augmented IPS (CIPS) and the cross-sectionally augmented Dickey-Fuller (CADF) unit root test (Lau et al., Citation2019; Pesaran, Citation2007). Cointegration in the model, intended to be created using DOLS, is a second crucial requirement (Kao & Chiang, Citation2000). The panel cointegration test should be used to determine whether there is a long-term link between the variables once the unit root in the panel has been validated (Pedroni, Citation1999) and (Kao, Citation1999) cointegration tests are the best option for studies with cross-section dependency to determine whether there is a long-term connection between variables (Lau et al., Citation2019).
4. Empirical results and discussion
4.1. Descriptive statistics
Table shows that the average nonperforming loan of the sampled banks is approximately 0.043, with the highest value of the reported NPL being 0. 602. This indicates that the sampled banks sought to increase their loaned assets by a maximum of 60.2% over the study period. The operational efficiency ranges with a deviation of 0.131 from a minimum of 0.005 and a maximum of 0.832. Among the bank-specific variables, the standard deviation recorded in the diversification of bank revenue was 0.113. This shows that there is more variance in terms of diversifying their source of income. The size of banks revealed the highest standard deviation (4.099).
Table 1. Descriptive statistics
Another finding was the loan-to-asset ratio of banks, which ranged between 0.015 and 0.424. Conversely, the average ROA was.039. This suggests that the banking sector gained an average of 3.09 cents of profit before tax for a single birr investment in their assets throughout the sampled period. The average GDP growth in Ethiopia in the last 9 years was 10.04, with a standard deviation of 0.069. This means that the dollar in terms of the Ethiopian birr suggests less variability in the time under deliberation. The average values for political constraint and macroprudential policy indexes are 0.43 and 0.77, respectively. Macroeconomic variables were also constant over the sample period relative to bank-specific variables, except inflation rate fluctuations.
4.2. Estimation results of credit risk determinants
The study examines whether the cross sections are interconnected to choose the appropriate unit root and cointegration tests. The study conducted heterogeneity tests recommended by Pesaran (Citation2007) to validate the results of the cross-sectional dependency test. The findings in Table demonstrate the interdependence of the data cross-sections. It denotes the transfer of an economic shock from one bank to the others. Next, CIPS and CADF unit root tests were used to evaluate the order of integration level of the model parameters while allowing for cross-sectional dependency in the data. The results from Table indicate that the variables bank size, exchange rate, macroprudential policy, and political institutions are nonstationary at level, while they are stationary at the first difference.
Table 2. Cross section dependence and second generation unit root
The existence of a long-term link between variables was then examined using cointegration tests from Pedroni (Citation1999) and Kao (Citation1999) (see Table ). The findings of the Pedroni and Kao cointegration tests indicate that the null hypothesis—no cointegration between variables—is rejected. As a result, it may be assumed that the model’s parameters are cointegrated, which supports the factors’ long-term connection. This suggests that the model satisfies the second requirement for cointegration, making the use of dynamic ordinary least squares (DOLS) viable. The outcome of the DOLS estimation demonstrates that the residuals have a normal distribution, as the Jarque-Bera statistics are insignificant. Multicollinearity problems can be detected using the variance inflation factor (VIF) with a cut-off threshold of 10 and a tolerance value of 0.1. Table shows that the variables have no large VIF values, so none of the variables were excluded.
Table 3. Cointegration Test
Table shows the estimation results of DOLS. The political restrictions index results in statistically significant positive coefficients on credit risk, indicating that countries with more significant political restraints positively impact banks’ risk tendencies. This explains how moral hazard occurs when the government bails out institutions, increasing banks’ risk by fostering competition from financial markets (Adem, Citation2022b; Ashraf, Citation2017). Officials may also demand bribery through the “government expropriation risk,” establish bank monopolies that pool earnings with them, provide licences to preferred parties, and pressure banks to lend money to politically linked businesses (Ashraf, Citation2017). The political rights index measures of political institutions also do not have sensitivity to the favorable influence of political structures on bank credit risk.
Table 4. Regression results for credit risk determinants with DOLS and GMM estimations
The coefficients on MPP_index are negative and significant at a 1% and 5% significance level, indicating that with the strengthening of MPP, bank risk is reduced and benefits the development of the banking system and overall financial stability. It also suggested that a tightening (easing) of macroprudential policies reduces (increases) bank risk; in fact, macroprudential policies seem more effective in a tightening than in an easing phase (Altunbas et al., Citation2018). This infers that policymakers can design MPPs to achieve specific goals according to the different effects of macroprudential tools. This outcome is consistent with the research of (Dutta & Saha, Citation2020) and (Altunbas et al., Citation2018).
Following the results, it was observed that the loan-to-asset ratio was statistically significant and positively associated with credit risk. This explains that to develop their credit portfolios, banks may be interested in boosting their short-term profits by lowering their credit requirements. However, as slow credit growth and lower credit standards exacerbate moral hazard, the likelihood of future problematic loans rises. In addition, when banks change their supply, the resources allocated to loan screening and monitoring become overburdened. This results in inadequate risk analysis, which in turn causes a decline in loan processing and evaluation, which raises the number of NPLs (Naili & Lahrichi, Citation2022). This result is consistent with the prior hypothesis and the findings of recent studies by (Abbas & Ali, Citation2022) and (Abbas et al., Citation2021), who found that an increase in the loan ratio increases the risk of banks.
According to Naili and Lahrichi (Citation2022), banks with poor performance seem more inclined to take on additional risk and implement a flexible lending policy to make up for prior losses and maintain present profitability. This may be accomplished at the price of larger future NPLs. In comparison, the current research’s coefficient estimates show a negative relationship—significant at the 5% level—between bank profitability and credit risk. This may be explained by the fact that highly profitable banks are less inclined to make hazardous loans, which reduces their credit risk, than their rivals since they are under less pressure to earn more earnings. The finding confirms the bad management arguments put out by Louzis et al. (Citation2012), which contends that an increased degree of NPLs and low profitability are both indicators of weak management abilities. This outcome is consistent with Klein (Citation2013) and Abusharbeh (Citation2020). However, it is in contrast to the results of Boussaada et al. (Citation2020), who have demonstrated that bank profitability is positively linked to NPLs.
The results demonstrate that operational efficiency positively and substantially impacts NPLs. This is explained by the fact that banks with lower costs typically employ fewer resources for credit screening and cut back on expenses, which raises the amount of NPLs they have. This finding contradicts Berger and DeYoung’s (Citation1997) “bad management” argument, which claims that banks’ inefficiency is an indication of poor management of loan processing and supervision (Klein, Citation2013; Louzis et al., Citation2012). While it corroborates the “skimping theory,” which contends that institutions with insufficient resources for credit assessment and review procedures may seem to be cost-effective, they are more likely to have a greater proportion of nonperforming loans (Berger & DeYoung, Citation1997).
Although the results of previous research are unclear, this study shows a negative correlation between bank size and NPLs. This suggests that larger banks, typically well resourced, enhance one’s lending evaluation, which lessens the level of information asymmetries, affiliated adverse selection and moral hazard issues, and consequently, the number of NPLs. At the same time, smaller banks are more inclined to spend their limited funds on credit assessment, surveillance, and risk management procedures, contributing to the rise in NPLs (Naili & Lahrichi, Citation2022). As a result, the findings offer compelling support for the diversification assumption, which holds that larger banks often have greater levels of diversity than their smaller counterparts, which lowers their risk exposure and, consequently, the amount of problematic credit (Louzis et al., Citation2012).
Furthermore, the research indicates a substantial positive link between income diversification and nonperforming loans at a 1% level. The results of this study are consistent with the “dark side” of the diversification school of philosophy (Stiroh, Citation2004), which contends that income diversification and NPLs are positively correlated. This explains why bankers who enter new industries are more likely to have enormous loan losses since the risk increases (Louzis et al., Citation2012; Stiroh, Citation2004), which lowers bank efficiency (Adem, Citation2022b).
In terms of macroeconomic factors, a noteworthy result of the study is the substantial and inverse link observed between inflation and NPLs at the 1% level, suggesting that an increase in inflation reduces the actual value of the existing loans and the number of NPLs. The effect of inflation on the number of NPLs is determined by whether money is lent at fixed or adjustable interest rates. Banks that employ a variable lending rate face greater NPLs if interest rates rise due to monetary activities to control inflation (Fofack, Citation2005; Klein, Citation2013). A rise (fall) in interest rates caused by a rise (fall) in inflation could impair (enhance) borrowers’ capacity to fulfil existing loan commitments. This finding is similar to the results of Nkusu (Citation2011) and Hajja (Citation2020), who found that inflation reduces the value of outstanding debts, improving borrowers’ repayment capabilities. This, however, contradicts the findings of Mishra et al. (Citation2020), and Twum et al. (Citation2021). In practice, economic growth has long been regarded as the fundamental measure of the country’s economic process. Studies have shown that in intense economic times, consumers and companies are more prone to fulfil their obligations, lowering the number of default loans (Naili & Lahrichi, Citation2022). However, the result reveals that the coefficient estimates of the exchange rate and GDP indicate insignificant effects on credit risk. This implies that GDP growth is insufficient to manage the reduction of credit processes in the Ethiopian banking sector. The findings contradict those of Chaibi and Ftiti (Citation2015) and Ghosh (Citation2017). This finding is consistent with Abusharbeh (Citation2020) and Ghosh (Citation2015).
4.3. Robustness check
To confirm those primary inferences, additional robustness assessments were undertaken. First, an alternative estimation technique was conducted using a two-step system generalised method of moments estimator (GMM) proposed by Arellano and Bover (Citation1995). Reliability checks were performed to gauge the studies’ assertions and assess the model’s feasibility. The Arellano‒Bond AR (2) test reveals that there is no second-order autocorrelation. The Hansen and Sargan tests further support the model’s validity and the absence of instrument overidentification. The coefficient of the lagged dependent variable is statistically significant, providing credibility for the validity of GMM modelling techniques and indicating that credit risks persist over time in the Ethiopian banking industry. The primary robust estimation for credit risk parameters in the baseline DOLS results appeared to be trustworthy. The prior sign and significance levels are likewise retained by the additional predictive factors in (Table , Model 7 & 8).
Second, the study utilised an alternate proxy for measuring political institutions. As an extra indicator, the Freedom-house (Citation2020) political right index is employed. This index assesses electoral integrity and competition, as well as the ability of rival party and minority factions to restrain the administration. Because lenders and borrowers evaluate anticipated rewards and risks during investment choices, this proactive measurement is useful to corroborate key findings. Model 5 & 6 replaces Political Constraints with the Freedom-house (Citation2020) Political Rights Index. Consistent with Model 2 & 4, the Political Right index has positive and significant (at the 1% level) coefficients, indicating that banking credit risk stands much greater in nations with greater levels of political rights. Overall, the robustness of the findings indicates that macroprudential policy and political institutions have an enormous direct influence on bank credit risks. Thus, the likelihood of banking collapse is much greater within nations that have established political institutions, while utilising MPPs helps to control bank risk and reduce exposure in the banking industry.
5. Conclusions and managerial implication
Credit risk in financial institutions has been widely debated among experts and legislators as a substantial risk to the financial industry’s health (Naili & Lahrichi, Citation2022). Nonetheless, empirical research has concentrated on bank-specific and macroeconomic drivers of nonperforming loans. This study attempts to address a gap in the literature by investigating how a nation’s political institutions and macroprudential policies influence credit risk, in addition to bank-specific and macroeconomic causes. The research used panel data from 2011 to 2019 and employed the dynamic ordinary least squares (DOLS) estimation approach for data analysis. The result is robust to alternative estimation techniques and alternative proxies for political institutions. According to the findings, the primary factors of credit risk in Ethiopia include bank size, operational efficiency, profitability, inflation, loan-to-asset ratio, political institution, income diversity, and macroprudential policy. In contrast, GDP and the exchange rate have little influence on credit risk. The results, in particular, imply that political institutions have significant, direct impacts that are positively linked to credit risk. The negative association between MPPs and bank credit risk suggests that policymakers may utilise MPPs to control bank risk and reduce exposure in the banking industry. The results indicate that banks with larger sizes and profitability have comprehensive risk management processes to minimise loan defaults. The findings also provide indications that retaining a greater loan-to-asset ratio, cost-effectiveness, and diversity of the banking commercial model reduces credit quality by promoting bad debt. When lending rates are steady, a greater inflation rate reduces the actual worth of existing debt, increasing the borrower’s potential to service their debts.
The outcomes of this study provide significant implications for authorities and bankers in developing countries such as Ethiopia, who need a comprehensive understanding of credit risk-determining elements to implement prudential rules and remedial management policies. More precisely, as preserving better resources and performance influences combating loan losses, banks must have adequate resources and actively improve risk management protocols. Bank executives must thrive on economies of scale by handling information asymmetry when they diversify their source of income. As cost-cutting may increase the number of nonperforming loans, dedicating sufficient means by banks, a set of regulations that promote rigorous risk-based surveillance, and adequate resource allocation can assist in mitigating the risk of loan defaults. Additionally, it may be inferred that high loan-to-asset ratios promote nonperforming loans; thus, banks and regulators should place more emphasis on keeping a data mine for the management of defaulter repayment capacities that allow banks to prevent adverse selection and evaluate the borrower’s ability to repay. Furthermore, central banks should develop plans to reduce the systemic risk problems caused by superior political arrangements. Finally, fiscal policy should be focused on enhancing economic growth and implementing procedures to adjust inflation.
While the study has a lot to contribute to banks and regulators, it has shortcomings as it only concentrates on one country and excludes elements such as competitiveness and cultural and behavioral characteristics. Future studies may thus look at additional factors influencing credit risk, such as cultural and behavioral factors. Again, more investigation may reveal how current factors affect NPLs in cross-country analyses.
Disclosure statement
No potential conflict of interest was reported by the author.
Additional information
Funding
Notes on contributors
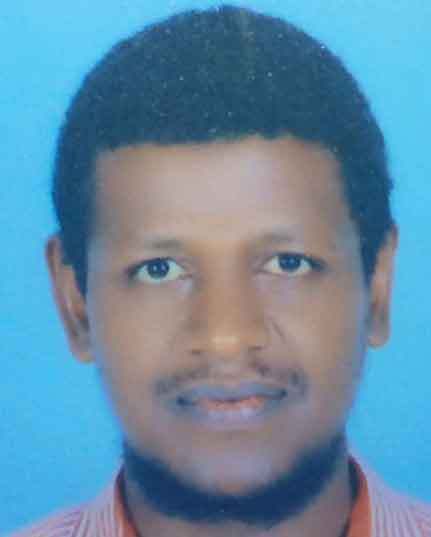
Mohammed Adem
Mohammed Adem Endris is working as a lecturer and researcher in Arba Minch University, Departments of Accounting and Finance. He has publications in well-reputed national & International journals. His research interest includes the broad area on Behavioral Finance, Islamic Finance, Corporate Finance, Auditing, Taxation, Fintech, Environmental Economics and so on. He has several publications in leading and well-reputed journals like Global Business Review, Asian Journals of Accounting Research, Discrete of Dynamics in Nature and Society, etc.
References
- Abbas, F., & Ali, S. (2022). Economics of loan growth, credit risk and bank capital in Islamic banks. Kybernetes, 51(12), 3591–20. https://doi.org/10.1108/K-03-2021-0232
- Abbas, F., Ali, S., & Ahmad, M. (2021). Does economic growth affect the relationship between banks’ capital, liquidity and profitability: Empirical evidence from emerging economies. Journal of Economic and Administrative Sciences. https://doi.org/10.1108/JEAS-03-2021-0056
- Abid, L., Ouertani, M. N., & Zouari-Ghorbel, S. (2014). Macroeconomic and bank-specific determinants of household’s nonperforming loans in Tunisia: A dynamic panel data. Procedia Economics and Finance, 13, 58–68. https://doi.org/10.1016/s2212-5671(14)00430-4
- Abusharbeh, M. T. (2020). Determinants of credit risk in Palestine: Panel data estimation. International Journal of Financial Economics, (October), 1–10. https://doi.org/10.1002/ijfe.2329
- Adem, M. (2022a). Determinants of credit risk in Ethiopian banking industry: Does political stability matter?. https://Do.Org/10.1177/09721509221104244, 097215092211042.
- Adem, M. (2022b). Impact of income diversification on bank stability: A cross-country analysis. Asian Journal of Accounting Research, 8(2), 133–144. https://doi.org/10.1108/AJAR-03-2022-0093
- Adeolu, M. (2014). Asset quality and bank performance: A study of commercial banks in Nigeria. Research Journal of Finance & Accounting, 5(18), 39–44. https://doi.org/10.7176/RJFA
- Akinci, O., & Olmstead Rumsey, J. (2018). How effective are macroprudential policies? An empirical investigation. Journal of Financial Intermediation, 33, 33–57. https://doi.org/10.1016/J.JFI.2017.04.001
- Alhassan, A. L., Kyereboah-Coleman, A., & Andoh, C. (2014). Asset quality in a crisis period: An empirical examination of Ghanaian banks. Journal of Advanced Research, 4(1), 50–62. https://doi.org/10.1016/j.rdf.2014.03.001
- Ali, S., Yousaf, I., Chughtai, S., & Ali Shah, S. Z. (2022). Role of bank competition in determining liquidity creation: Evidence from GCC countries. Journal of Applied Economics, 25(1), 242–259. https://doi.org/10.1080/15140326.2022.2043114
- Allen, F., & Gu, X. (2018). The interplay between regulations and financial stability. Journal of Financial Services Research, 53(2–3), 233–248. https://doi.org/10.1007/s10693-018-0296-7
- Altunbas, Y., Binici, M., & Gambacorta, L. (2018). Macroprudential policy and bank risk. Journal of International Money and Finance, 81, 203–220. https://doi.org/10.1016/j.jimonfin.2017.11.012
- Amuakwa-Mensah, F., Marbuah, G., & Ani-Asamoah Marbuah, D. (2017). Re-examining the determinants of non-performing loans in Ghana’s banking industry: Role of the 2007–2009 financial crisis. Journal of African Business, 18(3), 357–379. https://doi.org/10.1080/15228916.2017.1308199
- Arellano, M., & Bover, O. (1995). Another look at the instrumental variable estimation of error-components models. Journal of Econometrics, 68(1), 29–51. https://doi.org/10.1016/0304-4076(94)01642-D
- Ashraf, B. N. (2017). Political institutions and bank risk-taking behavior. Journal of Financial Stability, 29, 13–35. https://doi.org/10.1016/J.JFS.2017.01.004
- Atellu, A. R., Muriu, P., & Sule, O. (2021). Do bank regulations matter for financial stability? Evidence from a developing economy. Journal of Financial Regulation & Compliance, 29(5), 514–532. https://doi.org/10.1108/JFRC-12-2020-0114
- Baselga-Pascual, L., Trujillo-Ponce, A., & Cardone-Riportella, C. (2015). Factors influencing bank risk in Europe: Evidence from the financial crisis. The North American Journal of Economics & Finance, 34, 138–166. https://doi.org/10.1016/j.najef.2015.08.004
- Berger, A. N., & DeYoung, R. (1997). Problem loans and cost efficiency in commercial banks. Journal of Banking and Finance, 21(6), 849–870. https://doi.org/10.1016/S0378-4266(97)00003-4
- Bernanke, B. S., Gertler, M., & Gilchrist, S. B. (1998). The financial accelerator in a quantitative business cycle framework. Handbook of Macroeconomics, 1, 1341–1393. https://doi.org/10.1016/S1574-0048(99)10034-X
- Boussaada, R., Hakimi, A., & Karmani, M. (2020). Is there a threshold effect in the liquidity risk–non-performing loans relationship? A PSTR approach for MENA banks. International Journal of Finance & Economics, 27(2), 1886–1898. https://doi.org/10.1002/ijfe.2248
- Carreras, O., Davis, E. P., & Piggott, R. (2018). Assessing macroprudential tools in OECD countries within a cointegration framework. Journal of Financial Stability, 37, 112–130. https://doi.org/10.1016/J.JFS.2018.04.004
- Cerutti, E., Claessens, S., & Laeven, L. (2017). The use and effectiveness of macroprudential policies: New evidence. Journal of Financial Stability, 28, 203–224. https://doi.org/10.1016/J.JFS.2015.10.004
- Chaibi, H., & Ftiti, Z. (2015). Credit risk determinants: Evidence from a cross-country study. Research in International Business and Finance, 33, 1–16. https://doi.org/10.1016/j.ribaf.2014.06.001
- Delis, M. (2015). Bank risk, financial stability, and the role of regulation. Journal of Banking & Finance, 61, S1–S2. https://doi.org/10.1016/J.JBANKFIN.2015.11.015
- Dutta, K. D., & Saha, M. (2020). Nexus of governance, macroprudential policy and financial risk: Cross-country evidence. Economic Change and Restructuring, 54(4), 1253–1298. https://doi.org/10.1007/s10644-020-09301-9
- Fofack, H. L. (2005). Nonperforming loans in Sub-Saharan Africa: Causal analysis and macroeconomic implications. Working Paper of World Bank Policy Research , WPS3769, 1–36. https://doi.org/10.1596/1813-9450-3769
- Freedom-house. (2020). The Annual Survey of Political Rights and Civil Liberties. https://rowman.com/ISBN/9781538151815/Freedom-in-the-World-2020-The-Annual-Survey-of-Political-Rights-and-Civil-Liberties
- Freeman, R., & Bloem, A. M. (2005). The treatment of nonperforming loans. International Monetary Fund. https://www.imf.org/external/pubs/ft/bop/2005/18.htm
- Ghosh, A. (2015). Banking-industry specific and regional economic determinants of nonperforming loans: Evidence from US states. Journal of Financial Stability, 20, 93–104. https://doi.org/10.1016/j.jfs.2015.08.004
- Ghosh, A. (2017). Sector-specific analysis of nonperforming loans in the US banking system and their macroeconomic impact. Journal of Economics and Business, 93, 29–45. https://doi.org/10.1016/j.jeconbus.2017.06.002
- Hajja, Y. (2020). Impact of bank capital on nonperforming loans: New evidence of concave capital from dynamic panel-data and time series analysis in Malaysia. International Journal of Finance & Economics. (2019) 1–28. https://doi.org/10.1002/ijfe.2305
- Henisz, W. (2015). The Political Constraint Index (POLCON) Dataset. https://mgmt.wharton.upenn.edu/faculty/heniszpolcon/polcondataset/
- Kao, C. (1999). Spurious regression and residual-based tests for cointegration in panel data. Journal of Econometrics, 90(1), 1–44. https://doi.org/10.1016/S0304-4076(98)00023-2
- Kao, C., & Chiang, M. H. (2000). On the estimation and inference of a cointegrated regression in panel data. Advances in Econometrics, 15, 179–222. https://doi.org/10.1016/S0731-9053(00)15007-8/FULL/XML
- Khan, M. A., Siddique, A., & Sarwar, Z. (2020). Determinants of nonperforming loans in the banking sector in developing state. Asian Journal of Accounting Research, 5(1), 135–145. https://doi.org/10.1108/ajar-10-2019-0080
- Klein, N. (2013). Non-performing loans in CESEE: Determinants and impact on macroeconomic performance. IMF Working Papers, 13(72), 1. https://doi.org/10.5089/9781484318522.001
- Kodongo, O. (2018). Financial regulations, financial literacy, and financial inclusion: Insights from Kenya. Emerging Markets Finance and Trade, 54(12), 2851–2873. https://doi.org/10.1080/1540496X.2017.1418318
- Korinek, A., & Sandri, D. (2016). Capital controls or macroprudential regulation? Journal of International Economics, 99, S27–S42. https://doi.org/10.1016/J.JINTECO.2016.02.001
- Kuzucu, N., & Kuzucu, S. (2019). What drives non-performing loans? Evidence from emerging and advanced economies during pre- and PostGlobal financial crisis. Emerging Markets Finance and Trade, 55(8), 1694–1708. https://doi.org/10.1080/1540496X.2018.1547877
- Lau, L.-S., Ng, C.-F., Cheah, S.-P., & Choong, C.-K. (2019). Panel data analysis (Stationarity, cointegration, and causality). Environmental Kuznets Curve, EKC, 101–113. https://doi.org/10.1016/B978-0-12-816797-7.00009-6
- Lee, J., Chang, I., Chen, C., & Chen, C.-C. (2020). Determinants of non-performing loans, firm’s corporate governance and macroeconomic factors. International Journal of Finance and Economics, 27(1), 88–98. https://doi.org/10.1002/ijfe.2139
- Liu, W. M., & Ngo, P. T. H. (2014). Elections, political competition and bank failure. Journal of Financial Economics, 112(2), 251–268. https://doi.org/10.1016/J.JFINECO.2014.02.005
- Louzis, D. P., Vouldis, A. T., & Metaxas, V. L. (2012). Macroeconomic and bank-specific determinants of nonperforming loans in Greece: A comparative study of mortgage, business and consumer loan portfolios. Journal of Banking and Finance, 36(4), 1012–1027. https://doi.org/10.1016/j.jbankfin.2011.10.012
- Mishra, A. K., Abid, M., Jain, S., & L, M. R. (2020). Macroeconomic determinants of nonperforming assets in the Indian banking system: A panel data analysis. International Journal of Finance & Economics. (2019) 1–16. https://doi.org/10.1002/ijfe.1989
- Naili, M., & Lahrichi, Y. (2022). Banks’ credit risk, systematic determinants and specific factors: Recent evidence from emerging markets. Heliyon, 8(2), 1–16. https://doi.org/10.1016/j.heliyon.2022.e08960
- Nikolaidou, E., & Vogiazas, S. (2017). Credit risk determinants in sub-saharan banking systems: Evidence from five countries and lessons learnt from central east and South east European countries. Review of Development Finance, 7(1), 52–63. https://doi.org/10.1016/j.rdf.2017.01.003
- Nkusu, M. (2011). Nonperforming loans and macrofinancial vulnerabilities in advanced economies. International Monetary Fund.
- Papanikolaou, N. I. (2018). To be bailed out or to be left to fail? A dynamic competing risks hazard analysis. Journal of Financial Stability, 34, 61–85. https://doi.org/10.1016/J.JFS.2017.11.005
- Pedroni, P. (1999). Critical values for cointegration tests in heterogeneous panels with multiple regressors. Oxford Bulletin of Economics and Statistics, 61(SUPPL), 653–670. https://doi.org/10.1111/1468-0084.61.s1.14
- Pedroni, P. (2001). Purchasing power parity tests in cointegrated panels. The Review of Economics and Statistics, 83(4), 727–731. https://doi.org/10.1162/003465301753237803
- Pesaran, M. H. (2007). A simple panel unit root test in the presence of cross-section dependence. Journal of Applied Econometrics, 22(2), 265–312. https://doi.org/10.1002/jae.951
- Phillips, Peter C. B. & Hansen, B. E. (1990). Statistical inference in instrumental variables regression with I(1) processes. The Review of Economic Studies, 57(1), 99–125. https://doi.org/10.2307/2297545
- Rubbaniy, G., Khalid, A. A., Ali, S., & Polyzos, E. (2022). Cyclicality of liquidity creation: Nonlinear evidence from US bank holding companies. SSRN Electronic Journal. https://doi.org/10.2139/SSRN.4139760
- Saha, M., & Dutta, K. D. (2020). Nexus of financial inclusion, competition, concentration and financial stability: Cross-country empirical evidence. Competitiveness Review, 31(4), 669–692. https://doi.org/10.1108/CR-12-2019-0136
- Salas, V., & Saurina, J. (2002). Credit risk in two institutional regimes: Spanish commercial and savings banks. Journal of Financial Services Research, 22(3), 203–224. https://doi.org/10.1023/A:1019781109676
- Stiroh, K. J. (2004). Do community banks benefit from diversification? Journal of Financial Services Research, 25(2–3), 135–160. https://doi.org/10.1023/b:fina.0000020657.59334.76
- Swami, O. S., Nethaji, B., & Sharma, J. P. (2019). Determining risk factors that diminish asset quality of Indian commercial banks. Global Business Review, 23(2), 372–384. https://doi.org/10.1177/0972150919861470
- Tatarici, L. R., Kubinschi, M. N., & Barnea, D. (2020). Determinants of non-performing loans for the EEC Region. A financial stability perspective. Management & Marketing, 15(4), 621–642. https://doi.org/10.2478/MMCKS-2020-0036
- Tugcu, C. T. (2018). Panel data analysis in the energy-growth Nexus (EGN). In The economics and econometrics of the Energy-Growth Nexus (pp. 255–271). Elsevier Inc. https://doi.org/10.1016/B978-0-12-812746-9.00008-0
- Twum, A. K., ZhongMing, T., Agyemang, A. O., Ayamba, E. C., & Chibsah, R. (2021). The impact of internal and external factors of credit risk on businesses: An empirical study of Chinese commercial banks. Journal of Corporate Accounting & Finance, 32(1), 115–128. https://doi.org/10.1002/JCAF.22482
- Umar, M., & Sun, G. (2018). Determinants of nonperforming loans in Chinese banks. Journal of Asia Business Studies, 12(3), 273–289. https://doi.org/10.1108/JABS-01-2016-0005
- World Bank. (2019). Ethiopia financial sector development: The path to an efficient stable and inclusive financial sector. World Bank.
- Zhang, X., Li, F., Li, Z., & Xu, Y. (2018). Macroprudential policy, credit cycle, and bank risk-taking. Sustainability, 10(10), 3620. https://doi.org/10.3390/su10103620